What are Your Pronouns? Examining Gender Pronoun Usage on Twitter
Abstract
The increasing awareness of nonconforming gender identities puts discussions of gender inclusivity at the forefront. Using people’s preferred pronouns is a primary way to develop gender-inclusive language. This work presents the first empirical research on the self-disclosure of gender pronouns on social media. Leveraging a Twitter dataset with over 2 billion tweets collected over two years, we find that the public self-disclosure of gender pronouns is on the rise. The disclosure of gender pronouns is particularly popular among users employing she series pronouns, followed by he series pronouns, and a smaller but sizable amount of non-binary pronouns. By analyzing Twitter messages and users’ sharing activities, we identify the users who choose to disclose their gender pronouns and additionally distinguish users of various gender pronoun categories. Analyzing the relationship between social network exposure to gender pronouns and gender pronoun adoption, we show that those who frequently interact with users who disclose gender pronouns are also more likely to adopt gender pronouns in the future. This work carries implications for research on gender inclusivity.
1 Introduction
Discussions and disclosures of gender pronouns are becoming ubiquitous at schools, workplaces, and especially on social media (University of Waterloo n.d.; Chen 2021; Goldberg 2020). Online platforms such as Twitter, Instagram, and LinkedIn are rolling out designated fields for users to add their preferred pronouns in their biographies (Elks 2021). Many proactively ask, “What are your pronouns?”, a question that symbolizes “an invitation to declare, to honor, or to reject, not just a pronoun, but a gender identity” (Baron 2020). Encouraging gender pronoun sharing fosters gender-inclusive language, which is especially important for people whose genders do not fit neatly into the stereotypical binary gender roles. Non-binary gender pronouns are constantly evolving as gender identity awareness grows (Berger 2019; Gustafsson Sendén, Renström, and Lindqvist 2021). The Swedish gender-inclusive, third-person pronoun hen achieved widespread usage and increasing acceptance since its inception in the Swedish vocabulary in the early 2010s (Gustafsson Sendén, Renström, and Lindqvist 2021). In English, the singular they is the most widely accepted gender-neutral pronoun (Hekanaho 2020), followed by popular picks such as xe and ze (Devin-Norelle 2020). Preferred pronouns can also shift for those who are gender fluid, moving across the spectrum of gender identities over time (Katz-Wise 2020; Diamond 2020).
Acceptance of minority gender identities faces many challenges (Smith 2017; Brown 2017, 2022b; Parker, Horowitz, and Brown 2022), with 54% of the current US population believing that gender identity is determined by physiological attributes (Parker, Horowitz, and Brown 2022; Lipka and Tevington 2022). However, the share of the population who publicly use non-binary pronouns or state themselves as gender-nonconforming is undeniably rising (Geiger and Graf 2019; Brown 2022a): the proportion of people who personally know someone who uses gender-neutral pronouns grew from 18% in 2017 to 26% in 2021 (Geiger and Graf 2019). In 2020, an estimated 5% of US young adults identify as gender-nonconforming (Brown 2022a).
In light of the increasing awareness of gender nonconformity, this paper offers an empirical and analytical approach to provide an initial understanding of gender pronoun disclosure on social media. In particular, we seek to answer the following research questions:
-
•
RQ1: What is the prevalence of gender pronoun usage in Twitter user biographies over time? We show that though the proportion of users who use gender pronouns remains a minority at less than 8% at any given time, this number is rising over time, up 33% from 2020 to 2021. Twice as many tweets are posted by she series (6M) compared to those shared by he series users (3M). A notable 1.6M tweets are posted by users with non-binary pronouns.
-
•
RQ2: Can we automatically identify the presence and type of gender pronouns in a user’s biography? We can detect a user’s gender pronoun category, or lack thereof, from their Twitter usage and sharing activities.
-
•
RQ3: Can we automatically identify gender pronoun adoption from prior social network exposure? We show that users who adopted gender pronouns, especially non-binary pronouns, experienced higher social network exposure to gender pronouns than those who did not.
To address these RQs, we leverage a large recent longitudinal Twitter dataset on COVID-19 collected over two years (2020-2021) (Chen et al. 2020). As discussions on COVID-19 are not primarily focused on gender disclosure, this dataset grants us access to a large sample of users who may or may not use gender pronouns. We also acknowledge that similar conclusions were found in a parallel work (Tucker and Jones 2022), strengthening the validity of our findings. Our findings provide actionable insights toward a principled understanding of gender identity and gender inclusivity on social media and stimulate further research.
2 Background
Gender Identity and Pronouns
Gender is a deeply rooted societal concept that many believe to be determined by sex at birth (Parker, Horowitz, and Brown 2022; Lipka and Tevington 2022). But while sex is a biological state, gender is an identity that can be either male or female, neither male nor female, somewhere in between, or something else altogether (Marwick 2013). Gender identity also need not be immutable or singular (Keyes 2018; Keyes, May, and Carrell 2021). The common social understanding of gender maps directly from physiology and dictates how people of each sex should behave, conventionally landing either in masculinity or femininity (Deaux 1985; Marwick 2013; Goffman 1977; Wolf 2000; Witt and Wood 2010). The existence of other arrangements of gender threatens this notion of well-defined boundaries of sex and gender (Stryker and Whittle 2013), defying gender norms deeply reinforced from popular culture to everyday discourse (Gauntlett 2008; Cameron 1998). As such, gender nonconforming people face discrimination and stigma. One of the most devastating social phenomena is the high rates of suicide and depression among the marginalized LGBTQ+ community (Haas et al. 2010; Rotondi et al. 2012; Budge, Adelson, and Howard 2013; Toomey, Syvertsen, and Shramko 2018), aggravated by targeted hate crimes and systematic violence against gender-nonconforming people (HRC Foundation 2020; Andrew 2020; Gyamerah et al. 2021). Recognizing non-binary gender pronouns is a crucial step towards gender equity and inclusivity (Hosking 2021; Brown et al. 2020; Knutson, Koch, and Goldbach 2019; Moser and Devereux 2019; Parks, O’Connor, and Parrish 2016). Using a person’s correct pronouns shows respect and validation for their chosen gender identity and self-representation (Parks, O’Connor, and Parrish 2016; Knutson, Koch, and Goldbach 2019). In this work, we utilize users’ self-disclosed gender pronouns as a proxy for expressions of gender identity.
Gender Pronouns on Social Media
Most empirical gender research on social media treats gender as a binary variable (Ciot, Sonderegger, and Ruths 2013; Alowibdi, Buy, and Yu 2013; Liu and Ruths 2013; Bamman, Eisenstein, and Schnoebelen 2014; Zheng et al. 2016; Fink, Kopecky, and Morawski 2012). This is harmful in and of itself for the gender-nonconforming people we address in this work, perpetuating biases rooted in misgendering based on sexism and cisgenderism (Ansara and Hegarty 2014; Keyes 2018). A 2021 study by Fosch-Villaronga et al. (2021) found that Twitter’s automatic, binary gender classifier misgendered 19% of the people surveyed. Other studies focus exclusively on trans or other LGBTQ+ people (Krueger and Young 2015; Karami, Webb, and Kitzie 2018; Tuah and Mazlan 2020), neglecting the wider cisgender society and those who have not openly declared their gender.
There are two gender-related studies on Twitter COVID-19 discourse. Thelwall, Thelwall, and Fairclough (2021) conducted a thematic analysis of the Twitter biographies of male, female, and non-binary people who tweeted about COVID-19 in the UK, demonstrating variations in their interests, jobs, relationships, sexuality, and linguistic styles. Al-Rawi et al. (2021) explored the differences in gendered COVID-19 discourse co-occurring with men, women, and non-binary keywords, suggesting that women disproportionately experienced domestic violence and that non-binary people had concerns about blood donation. Building on past literature, we carry out a large-scale analysis of not only users who disclosed gender identity but also those who did not, as well as to analyze their Twitter activities beyond biography descriptions and text.
A recent concurrent preprint by Tucker and Jones (2022) replicates several of our key findings. Instead of a focused COVID-19 dataset, they collected a longitudinal Twitter dataset of US users using the 1% streaming API. They also find that the use of gender pronouns is on the rise and that there are substantially more she series users. Further, users with gender pronouns tend to cluster together in the following network. In this work, by focusing on users who appear several times throughout the dataset, we further contribute by showing how the social network is linked to the adoption of gender pronouns over time.
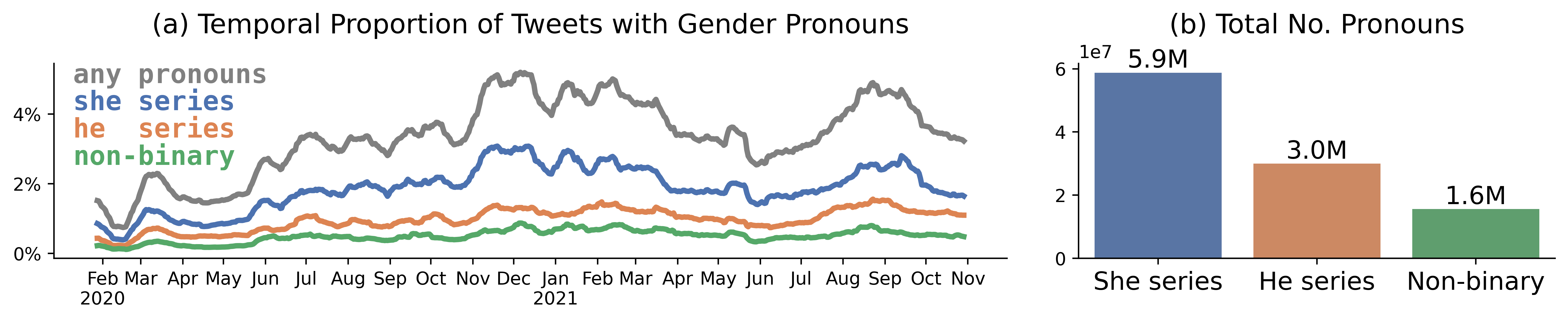
3 Data and Definitions
We use a public collection of nearly two years of Twitter data by Chen et al. (2020) (release v2.72)111https://github.com/echen102/COVID-19-TweetIDs from Jan 21, 2020, to Nov 5, 2021. The dataset was collected using keywords related to the COVID-19 pandemic and consists of over 2 billion tweets. It is important to note that the data was collected in real-time; therefore we can track changes in a user’s gender pronoun status in their biography over time.
Definitions
To maintain consistency throughout this paper, we define the following terms and phrases. Each user is categorized either as a GP (gender pronoun) user who disclosed any gender pronouns or a NP (no pronoun) user who did not disclose gender pronouns. Importantly, we infer a user’s gender from their disclosure of pronouns—we consider users to be women if they only use she series pronouns and men if they only use he series pronouns—but we caveat the gender and gender pronouns are not necessarily equivalent. Someone who uses she series pronouns can be a cis-gendered woman, trans, non-binary, or gender-diverse person. Further, within the context of this paper, we use the umbrella term non-binary to refer to all those who use gender-nonconforming pronouns (e.g., they), gender expansive pronouns (e.g., he/she), or gender fluid pronouns (transitions from using she to they, he to she, they to he, etc.). Though we have no intentions of diminishing the complex and multiplicitous arrangements of gender identity (Keyes, May, and Carrell 2021), we acknowledge the limitations of our rigid categorization. The rationale behind this choice is to initiate a high-level empirical analysis that can shed light on explicit self-disclosures of gender on social media at scale.
Gender Pronoun Categorization
We build a regular expression to match gender pronouns that appeared in users’ biography descriptions or display names. We do not match gender pronouns from the tweet texts because pronouns are parts of speech frequently used in normal discourse. To be matched as a GP user, the user’s biography must contain a substring with more than one gender pronoun separated by either forward slashes or commas, with or without additional blank spaces. We limit our scope to three categories of gender pronouns, which are the he series pronouns: {‘he’, ‘him’, ‘his’}, she series pronouns: {‘she’, ‘her’, ‘hers’}, and non-binary pronouns: {‘they’, ‘them’, ‘theirs’, ‘their’, ‘xe’, ‘xem’, ‘ze’, ‘zem’}. We further match the substring ‘any pronouns’ as an additional indication of gender nonconformity. The non-binary pronouns are chosen due to their relatively widespread usage in society and in previous literature (Hekanaho 2020).
4 Gender Pronouns on Twitter
To address RQ1, we begin by looking at the overall gender pronoun usage on Twitter over time. Fig. 2(a) charts the temporal patterns of the daily proportion of tweets by GP users over almost two years. Tweets by GP users represent a minority of all users’ tweets (mean = 3.3%, median = 3.3%, maximum = 7.3%). That said, we observe a rising proportion of tweets by GP users. Using a Mann-Kendall trend test, we find this growth to be statistically significant . In particular, the average proportion of tweets by GP users had a 33% increase, growing from 2.86% in 2020 to 3.82% in 2021 (-test, ). We also see a consistent pattern of she series users (5.9M) dominating the GP user pool, followed by he series users (3M) and then a smaller but considerable amount of non-binary pronouns users (1.6M), as illustrated in Fig. 2(b). The fact that there are twice as many tweets by she series users as by he series users stands in stark contrast with data collected via third-party Twitter surveys, which showed that Twitter users consist of 50% women and 50% men (Wojcik and Hughes 2019). Given that is it not likely to have twice as many women as men in this Twitter dataset, our work suggests that women are more prone to declare their gender pronouns than men or that women tweet more often about COVID-19.
5 Detecting Gender Pronouns
In this section, we test if we can identify a user’s gender pronoun status from their tweets, Twitter account metadata, and sharing activities. This analysis will help us understand the characteristics separating these users.
Method
Feature Sets.
We use the full span of the collected data and consider only users who had at least 10 posts to ensure we have sufficient data points per user. For each user, we build a series of feature sets as follows:
-
•
Text (): the BERTweet embeddings (Nguyen, Vu, and Tuan Nguyen 2020) of their most recent 20 tweets, zero-padded if they had less than 20 tweets. We use this value since most (60%) of the users had less than 20 tweets. BERTweet is the state-of-the-art language model developed specifically for Twitter, producing 768-dimensional embeddings per tweet.
-
•
Text Pronouns (): the number of times I, you, we, he, she, it, they, and other gender-nonconforming pronouns appeared in their tweets (see Appendix).
-
•
User (): The last entry of user-level metadata including whether they are verified and their number of friends, followers, favorites, statuses, and lists.
-
•
Activities (): The number of original tweets, retweets, quoted tweets, and replies the user posted as well as the number of hashtags, URLs, mentions, characters, and tokens used.
-
•
Mentions (): We gather the top 1K most frequently mentioned users in our dataset and build a vector representing how frequently they were mentioned. Note that we treat retweets as a subset of mentions.
We purposely omit user biography as a feature because it is our source of ground truth labeling of gender pronouns.
Modeling.
We run two experiments. Experiment (1) is the binary classification task of detecting whether a user discloses gender pronouns or not; experiment (2) is the multiclass classification task of detecting the category of gender pronoun disclosed. To address data imbalance, we sample 10K users from each of the gender pronoun groups (he series, she series, and non-binary) followed by 30K NP users, totaling 60K users. We gather two such random samples of 60K users, one for hyperparameter-tuning and one for final testing via 5-fold CV. For experiment (2), the 30K NP users are not included. As we will show below, this decision is in part driven by the relative ease in which the models are able to distinguish users with from users without pronouns in experiment (1). In this way, we can investigate the factors that separate users of each gender pronoun group. We train and test a deep neural network (DNN) for each prediction task, using each feature set in isolation as ablation studies, as well as all feature sets in combination. For every input scenario, we fine-tune the model using randomized grid search to test over 40 combinations of hyperparameters, fixing 20% of the data for validation. The exact model architecture and hyperparameters searched can be found in the Appendix. We use the macro-F1222Since the data is balanced, macro-F1 is equivalent to micro-F1. score as the classification metric and use two baseline methods for comparison: Random, which predicts random labels based on the distribution of labels in the training set, and Majority, which predicts the majority label.
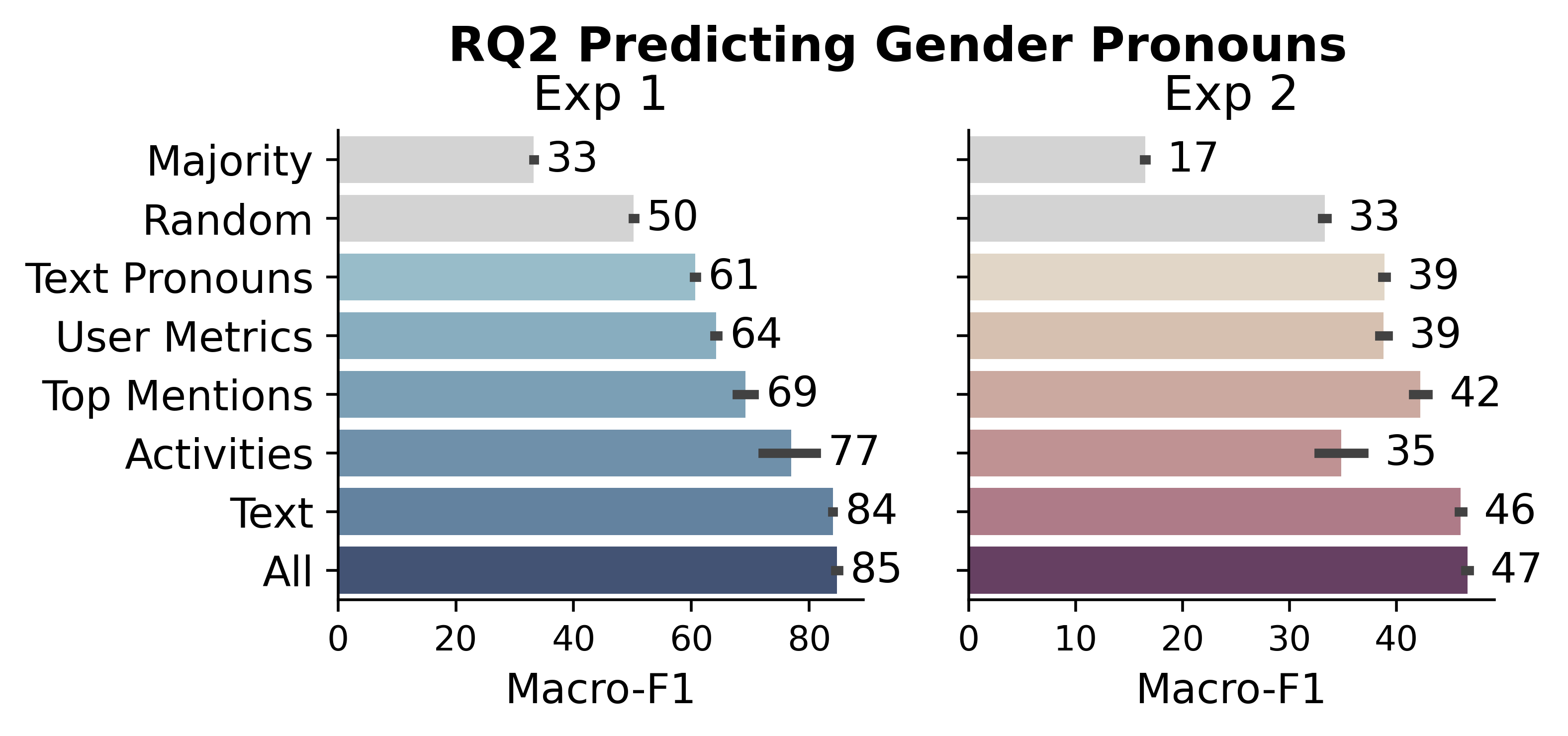
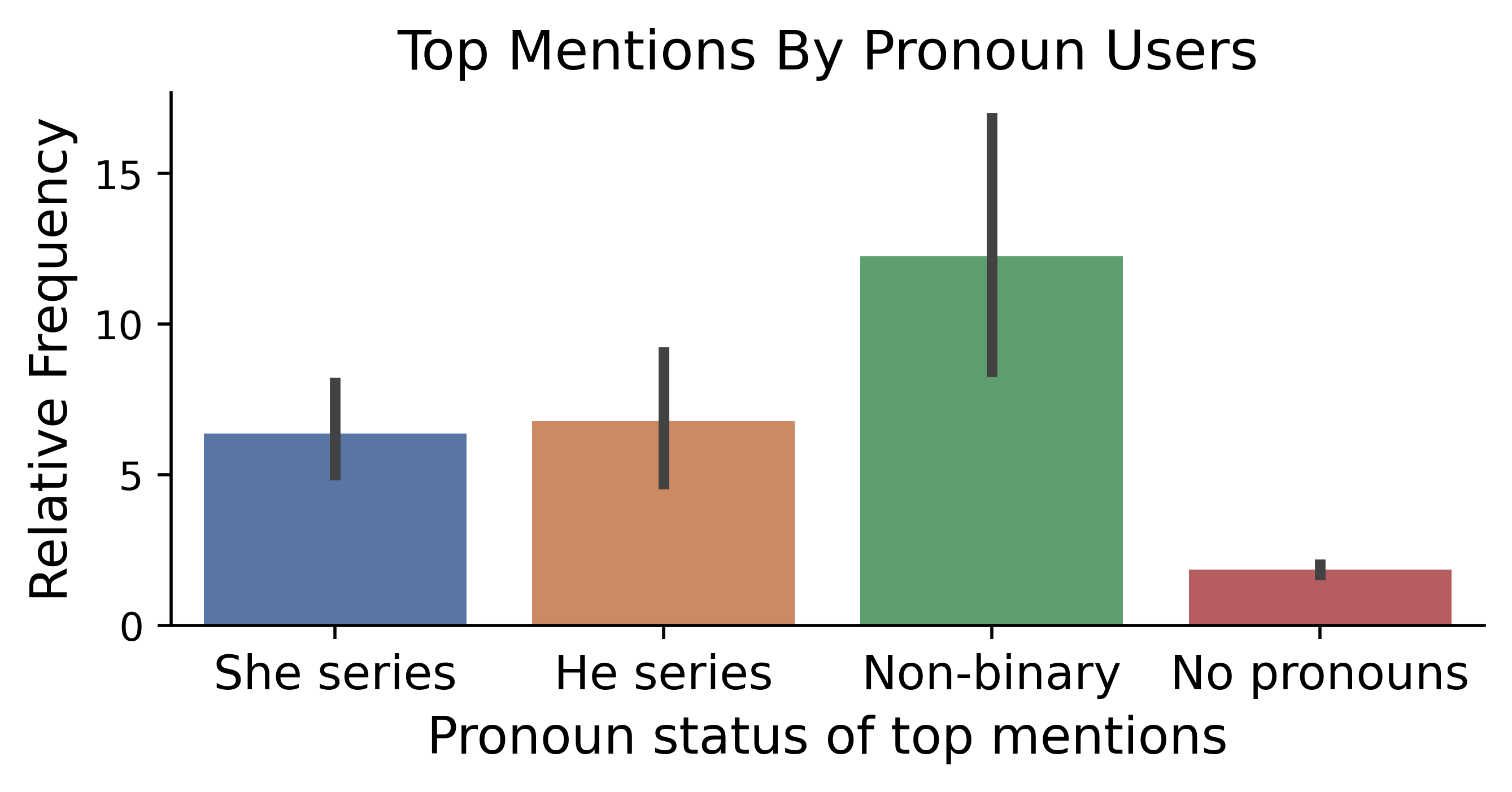
Results
Classification Performance.
For experiment (1), the binary classification distinguishing GP users from NP users, we find that using all of the feature sets improves predictive performance over the baseline Random and Majority models (Fig. 3). Embeddings of the textual data are the best single predictors of gender pronouns, achieving a macro-F1 of 84% (). User activities are the second best predictors at 77% macro-F1 (), albeit with a much larger variance. The feature set on top mentions achieves an impressive score of 69% macro-F1 (). Including all features yields a slightly better score of 85% (). These results indicate that users’ sharing activities encode substantial signals to predict whether they disclose gender pronouns.
For experiment (2), the multiclass classification of gender pronoun category, we note a similar improvement in classification performance over the baselines (Fig. 3). However, the improvement is more modest than what was achieved in experiment (1), The model using all feature sets attained only a macro-F1 of 47% (), indicating that discerning a user’s gender pronoun category is more challenging. Textual features remain the best predictors of gender pronoun category at 46% macro-F1 (), whereas activities features are the worst predictors at 35% macro-F1 (). The feature set of top mentions is the best single set of features after the textual features, yielding a score of 42% ().
Highly Mentioned Users.
Since using the feature set of highly mentioned users alone achieves a non-negligible improvement over the baselines in both experiments, we further explore whether there is a link between gender pronoun disclosure and the gender pronoun categories of the 1K highly mentioned users. To this end, we first check if the 1K highly mentioned users belong to any gender pronoun group. 12% of the highly mentioned users have gender pronouns (she series: 6%, he series: 4%, and non-binary: 2%). We then compute the relative frequency of them getting mentioned by GP users as opposed to NP users. The result is depicted in Fig 4. Across the board, all highly mentioned GP users are mentioned significantly more by GP users (Mann-Whitney U test, . These differences are also significant across all four groups of users using a Kruskal-Wallis H test (). Highly mentioned users who use non-binary pronouns, in particular, are 10 times more likely to be mentioned by GP users than by NP users ().
6 Gender Pronoun Adoption
Previously, we saw that the overall proportion of tweets with gender pronouns is rising (§4) and that the highly mentioned GP users are more likely mentioned by GP users. In this section, we want to better understand the mechanisms underpinning gender pronoun adoption, with a focus on social network effects.
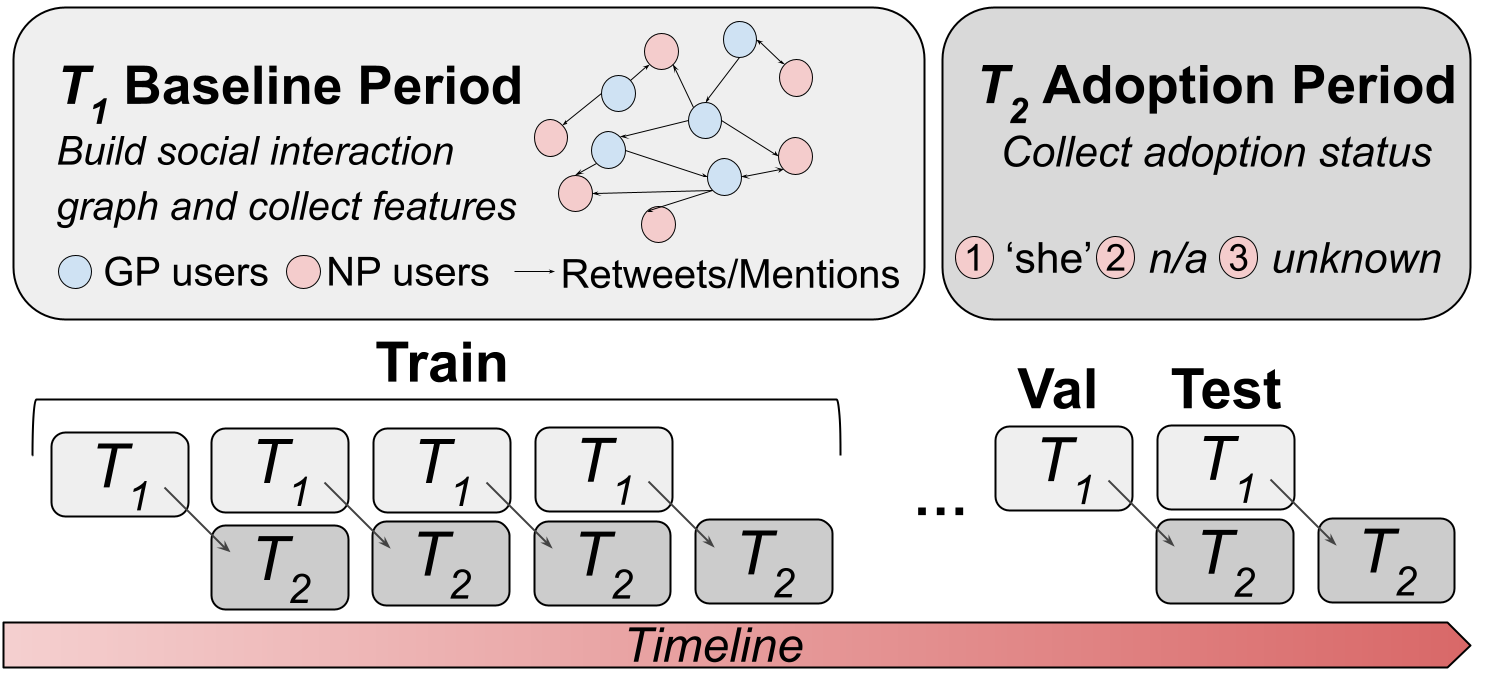
Method
Network Effects on Gender Pronouns.
In any social network, either online or offline, ideas, emotions, and behaviors can diffuse from one to another, a process known as social contagion (Christakis and Fowler 2013; Ferrara and Yang 2015a; Kramer, Guillory, and Hancock 2014; Rosenquist, Fowler, and Christakis 2011; Tsvetkova and Macy 2014; Pachucki, Jacques, and Christakis 2011; Hodas and Lerman 2014; Mønsted et al. 2017; Ferrara and Yang 2015b). Prior works have also shown that people who congregate in social communities share similar traits, interests, and tendencies, resulting in homophilic communities (McPherson, Smith-Lovin, and Cook 2001; Kossinets and Watts 2009; Bisgin, Agarwal, and Xu 2012). Putting network homophily and contagion together results in the social network effect (VanderWeele and An 2013). In this work, we elicit social network effects from observable sharing activities, such as retweeting and mentioning other users who disclose gender pronouns. While it is preferable to use the follower/following relationships among users (Hodas and Lerman 2014; Ferrara and Yang 2015a), this is not computationally feasible in practice given Twitter API’s rate limits. In lieu of the follower/following network, we use the retweet and mention networks under the assumption that the act of retweeting or mentioning can be treated as a form of a stronger, more explicit signal of social network exposure to peer influence as well as social endorsement (Boyd, Golder, and Lotan 2010; Metaxas et al. 2015).
Network | Time Span | No. Users | Pronouns (% of Total) | |||
---|---|---|---|---|---|---|
(Wks) | Total | Pronouns | She Series | He series | Non-binary | |
Retweet | Four | 11,435,974 | 165,590 (1.4%) | 0.9% | 0.3% | 0.3% |
Retweet | Eight | 4,106,182 | 101,023 (2.5%) | 1.6% | 0.5% | 0.4% |
Mention | Four | 19,533,731 | 278,084 (1.4%) | 0.9% | 0.3% | 0.2% |
Defining Measurement Periods.
To measure the network effects on gender pronoun adoption, we restructure our data into temporally disjointed subsets. Consider two time windows of data and , where occurred prior to . We build a directed, weighted, and attributed network from tweets posted in as follows. We first identify the set of GP users who appeared in our dataset with gender pronouns during . Then we extract the network interactions that occurred in , where each edge is a directed edge where at least one of the users involved in the interaction is a GP user, i.e., or . The weight of the edge represents the frequency of the mention or retweet interactions from u to v. In the context of this paper, retweeting a user is one way to mention them, therefore the retweet network edges are a strict subset of the mention network edges. We then retain the largest weakly connected component of the network. The NP users in the network compose the set , where . In the ensuing period , we check if any of the users in adopted gender pronouns. Note that a substantial amount of users would have unknowable pronoun status if we do not see their tweets in .
We repeatedly build and data using sliding windows of discretized time periods starting from Feb 1, 2020. We experiment with spans of four weeks for graphs built on both retweet edges and mention edges. This produces twenty-one and pairs, ending the last period on Oct 9, 2021. For graphs with retweet edges, we further explore a larger span of eight weeks to control for data biases due to time span selection. This produces ten and pairs, ending the last period on Oct 23, 2021.
Data Statistics.
The adoption experiment data sets used in this section are described in Table 1. We show the number of target users included in the experiments and how many of those eventually adopted pronouns. These users are all part of , that is, users who were connected to a GP user () in but who themselves did not have gender pronouns in . Overall, the proportion of gender pronoun adoption is very low at around 2%, rendering the data extremely imbalanced. This comes as no surprise given the relatively low gender pronoun usage we have seen so far in this paper, even though, by design, every user is connected to at least one GP user through retweeting or mentioning them. Of all the gender pronouns adopted, she series is the most popular pick. He series and non-binary pronoun adoptions follow closely behind, with he series pronouns slightly more frequently adopted.
Features Sets.
To explore the relationship between data collected from and the adoption of gender pronoun in of all users in , we use the following sets of features:
-
•
Text (): the BERTweet embeddings of the tweets each user posted in , concatenated as a single string.
-
•
User (): the BERTweet embedding of the user’s biography and user-level metadata including friends count, followers count, favorites count, statuses count, listed count, and whether they are verified. We use the last entry in if a user appeared more than once.
-
•
Network (): the number of neighbors they have (i.e., they retweeted or mentioned), the number of GP neighbors, and the number of neighbors with she series, he series, or non-binary pronouns. We also compute all weighted versions of the previous numbers of neighbors. Additionally, we include the node2vec (Grover and Leskovec 2016) graph embeddings learned from the retweet or mention network (see Appendix).
-
•
Activities (): the number of original tweets, retweets, quoted tweets, or replies the user posted during . We also count the number of hashtags, URLs, mentions, characters, and tokens used. Finally, we include the number of first-person singular pronouns used in the tweets, which convey individualism, and the number of first-person plural pronouns, which convey collectivism (Twenge, Campbell, and Gentile 2013).
Modeling.
We conduct two experiments. Experiment (1) is the binary classification of whether the user will adopt gender pronouns in and experiment (2) is the multiclass classification of which of the three gender pronoun categories the user adopts in . Users who did not adopt any gender pronouns are excluded from experiment (2), as was done in §5. Our experiments are repeated for all data scenarios: retweet networks over four- and eight-week time spans and mention networks over four-week time spans (i.e., is either four or eight weeks). For each data scenario, we use the last – pair as the test set, the second to last – pair as the validation set, and the remaining data as the training set. Similar to §5, we built a deep neural network (DNN) for these tasks. We first tune the model hyperparameters via randomized grid search using the training set. Please see the Appendix for the exact model architecture and hyperparameter search space. Macro-F1 is used as the evaluation metric. Following §5, we explore the results using each feature set in isolation as well as in combination, and we use the Random and Majority models as baselines.
Results
Classification Performance.
We present our results to address RQ3 in Fig. 6. The experimental results of the four-week and eight-week time spans of the retweet network are averaged due to the similarity in their results. Differently from Section 5.2.1, we recognize lower classification performances in experiment (1), the binary classification of predicting whether a user will adopt any type of gender pronouns in given the features in , probably due to the limited observation period leveraged in the training phase. Using all of the features sets in features, our approach performs slightly better than the Random and Majority baselines on the retweet network, achieving 56% macro-F1 compared to the baseline score of 50%. The mention network prediction tasks achieve better performance at scores of 59% macro-F1. Combining all features yields very little improvement over using features in isolation. All singular categories of features perform relatively equivalently. User-level features are the most predictive, while network features are some of the lowest. We note that this could be an artifact of our study design limited by computational constraints, forsaking the larger social network of users who are not directly connected to a GP user. Therefore, we do not know how many other NP users they were connected to. The ineffectiveness of the network features becomes even more apparent using the mention network. One explanation is the underlying difference between retweets and mentions: retweets are often seen as endorsements (Boyd, Golder, and Lotan 2010; Metaxas et al. 2015) whereas mentions can represent social conversations (Leavitt et al. 2009).
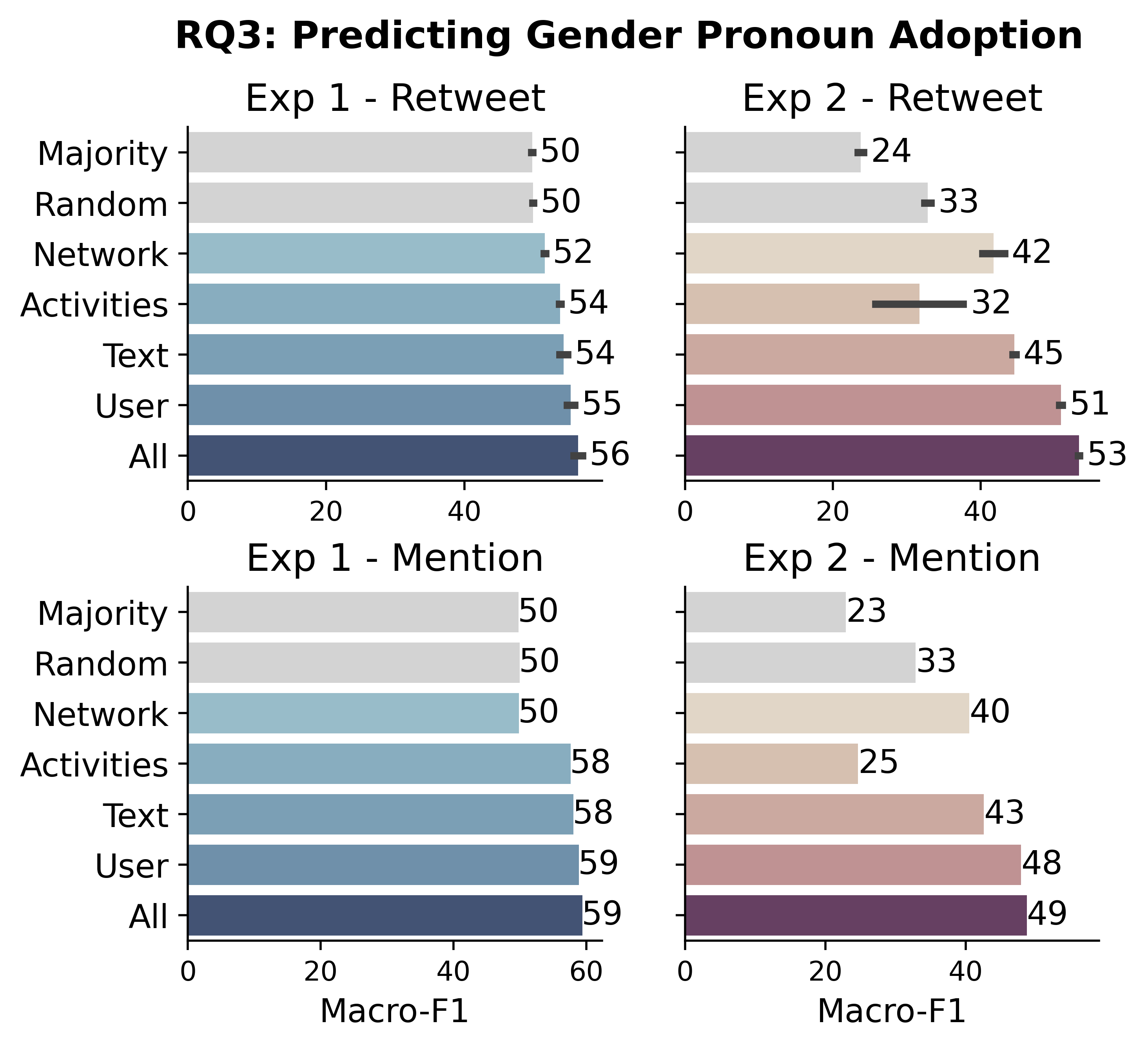
The predictive performance of experiment (2), which is the multiclass classification of which of the three gender pronoun category a GP adoptee chooses, yields further insights. While user-level features remain the singularly most predictive set of features, we now see a large improvement gained from using network features. This shows that network features contain cues as to which gender pronoun a user will adopt. We also see that the performance is better in general on the retweet network than on the mention network, suggesting that the retweet network signals encode more cues as to which gender pronoun category a user will adopt. This process could unfold via either social homophily or social contagion or both (Shalizi and Thomas 2011). Social networks are mediums for online communities of people with shared interests and similar backgrounds, forming homophilic clusters of users. It can also be a pathway for social influence, wherein one exerts behavioral changes on another by exposure. While we cannot disentangle the intertwining effects of the two forces, we provide additional analyses on the social network effects below.
Relative Number of Pronoun Neighbors.
To deepen our understanding of the link between the social network and gender pronoun adoption, we analyze how gender pronoun adoption in is linked to the relative number of GP neighbors they had in . A user’s neighbor is someone they retweeted in a retweet network and someone they mentioned in a mention network. We begin by establishing the expected number of type -gendered neighbors any NP user would be connected to in , given by:
(1) |
where denotes the number of -gendered neighbors a user had in , . The relative number of -gendered neighbors for users who adopted gender pronouns in is thus given by:
(2) |
where represents the set of users with pronoun status in , . If , then users who adopted pronoun status in had less -gendered pronoun neighbors in . Alternatively, if , then users who adopted pronoun status in had more -gendered pronoun neighbors in .
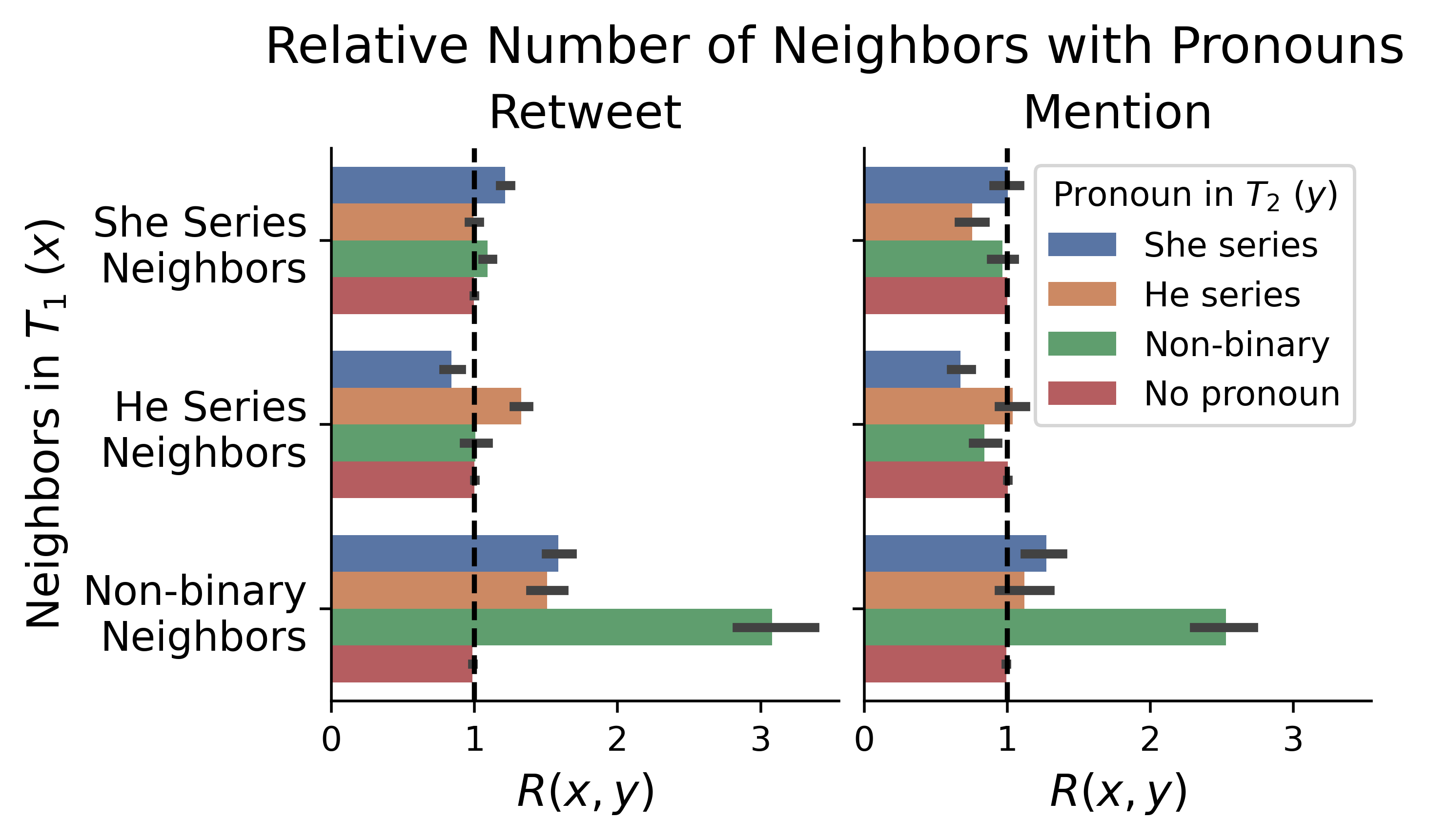
Fig. 7 displays the relative number of neighbors separately for the retweet and mention networks. We aggregate the statistics for each individual in every timeframe. The retweet networks of four-week and eight-week time spans are combined in this figure due to the similarity of their results. There are several key insights. First, we observe that users who did not adopt pronouns (red bars) retweeted or mentioned an expected number of GP users. That is, . This is both a sanity check and a result that shows that users who remained without gender pronouns had network exposures that did not deviate from the norm. Interestingly, users who adopted she and he series pronouns retweeted and mentioned slightly more neighbors of the same gender pronoun category but slightly fewer neighbors of the opposite gender pronoun category.
Importantly, we see that users who adopted any gender pronouns at all had substantially more neighbors who use non-binary pronouns. Those who adopted she and he series pronouns had 1.5 times non-binary neighbors in the retweet network. This number is particularly sizeable for non-binary pronoun adoptees, who retweeted over 3 times as many and mentioned over 2.5 times as many non-binary neighbors as the expected baseline.
7 Discussion
This paper provides a preliminary understanding of the landscape of gender pronoun usage on Twitter in recent years. We demonstrate the rising prevalence of gender pronoun usage and illustrate the differences between users with and without explicit gender pronoun disclosure, as well as between users who use she series, he series, and non-binary pronouns. We explain how social network influence leads to the adoption of gender pronouns. This work represents a critical initial step toward understanding the evolving landscape of gender pronoun use on social media. We discuss below the important findings and implications of this paper.
RQ1: Gender Pronouns on Twitter.
Our results show that gender pronoun usage is considerable on Twitter, with a rising number of tweets composed by users with gender pronouns over time. The most prevalent gender pronoun category is the she series, followed by he series and non-binary pronouns. She series users overwhelmingly dominate he series users at a ratio of 2:1, which could suggest that those who identify more as women are more prone to publicly disclose their gender pronouns than those who identify more as men. While there are fewer non-binary gender pronoun users, they nonetheless occupy a sizable proportion of the total Twitter population.
RQ2: Predicting Gender Pronouns.
We show that there are cues from a user’s Twitter usage and sharing activities that can inform whether a user uses any gender pronouns and also the type of gender pronoun they use. The implications are two-fold. On one hand, with textual data being the best predictor of gender pronoun status or lack thereof, there could be a difference in discourse revolving COVID-19 among men, women, non-binary people, and people who do not disclose gender pronouns. While previous Twitter work indeed showed that each gender identity group grapples with different COVID-19 concerns (Al-Rawi et al. 2021), we further provide evidence that discourse also differs between users with explicit gender pronoun disclosure and those who do not. On the other hand, non-textual features can also inform predictive modeling, suggesting that there are distinguishing patterns of social media usage that could help us discern users’ proclivity to gender pronoun disclosure and users of various gender pronoun categories. We hope this work sheds light on future research directions on this matter.
RQ3: Gender Pronoun Adoption.
We show that gender pronoun adoption is linked to social network effects. There are two theories. The social contagion theory suggests that gender pronoun adoption is causally impacted by observing other users using gender pronouns (Christakis and Fowler 2013). The homophily theory suggests that users with similar propensities of disclosing gender pronouns naturally attract one another (McPherson, Smith-Lovin, and Cook 2001). Though it is virtually impossible to disentangle these theories in observational data (Shalizi and Thomas 2011), we believe that gender pronoun adoption is a much more complex process. Gender pronoun usage is acquiring increasing exposure at work (Chen 2021), at school (University of Waterloo n.d.), and elsewhere in our lives (Thornhill 2019; Levietes 2022; Longman 2022; Kolodziej 2019). It is therefore futile to decouple the effects of the world around us on the adoption of gender pronouns on Twitter.
With these precautions in mind, we make two conclusions regarding RQ3. The first is that we find different gender pronoun groups respond differently to social network stimuli, possibly indicating the users of each gender identity have distinct responses to influence. The second conclusion is that each category of gender pronoun users exerts varying levels of influence on gender pronoun adoption. Having non-binary pronoun users in the social network is linked to the adoption of any gender pronouns, but is particularly linked to the adoption of non-binary pronouns. In other words, non-binary individuals stand out as being both the most likely to adopt gender pronouns after seeing others use gender pronouns and also the most related to others adopting gender pronouns in subsequent periods.
Finally, we must stress that our conclusions illustrate mechanisms of gender pronoun adoption, not gender or gender identity adoption. Our results should be not misconstrued to imply that gender identity is infectious, but rather that there is a link between a user’s social network interactions and their willingness to disclose gender pronouns.
Limitations
There are several limitations of our work. First, we consider only public-facing Twitter users, which presents unavoidable self-selection and platform biases in the users included in this study. Second, we use a COVID-19 dataset, which offers a wide variety of users who may or may not use gender pronouns but may arguably bear biases. We note that a similar, parallel study on a random Twitter also reached similar conclusions (Tucker and Jones 2022). Finally, our definition of social influence is self-selected exposure rather than involuntary exposure. Notwithstanding these limitations, we believe that our results carry actionable insights and crucial implications that could inform additional research in this area.
Broader Impact
Openly declaring one’s gender nonconformity can be daunting (Levin 2018). Yet we witness an increasing number of people publicly sharing their non-binary identities. This paper underscores how observing gender pronoun usage can affect gender pronoun adoption. Even the active declaration of gender pronouns by cisgender people, however self-evident, is an expression of solidarity with those whose genders lie beyond the dichotomous sociocultural perceptions of gender. Thus, our work provides actionable insights toward improving gender inclusivity in online spaces by encouraging gender pronoun disclosure.
By focusing on more generalizable aspects of network dynamics and Twitter usage, our results also carry transferable implications to other situations, contexts, and mediums beyond strictly COVID-19 discussions. The omnipresence of the COVID-19 pandemic motivated us to use this dataset as a tool to study a heterogeneous sample of the Twitter population, including not only the marginalized LGBTQ+ community but also the wider cisgender society.
Ethical Consideration
We emphasize the ethical impact of our work. First and foremost, while we simplified the categories of gender pronouns to holistically analyze the landscape the gender pronoun usage on Twitter, we recognize the complexity and multiplicity of gender identities (Keyes, May, and Carrell 2021). In this work, we also assume that a person’s gender pronoun choice is a reflection of their gender identity, which may not be the case in reality. Furthermore, since we demonstrate the possibility to detect genders on social media, we are aware of how our research could be misused and abused, endangering the LGBTQ+ community (United Nations Human Rights n.d.; HRC Foundation 2020; Andrew 2020; Gyamerah et al. 2021; Reid 2015). As such, we avoid exposing harmful personal details and our analyses are carried out only at an aggregated, high level. We will also not share the raw data or our pronoun labels, which could be exploited to identify individual users. We also strongly encourage future studies to consider the ethical aspects of online gender-related studies from the initial study design to the final research output.
Note: This study and the data used are IRB-approved.
8 Acknowledgments
The authors are indebted and grateful to people from the LGBTQ+ community who offered their feedback on this work, especially Os Keyes (University of Washington) for their generous and helpful advice. We also thank Prof. Jonathan May (USC) for his helpful insights. This work was supported by DARPA (award number HR001121C0169).
References
- Al-Rawi et al. (2021) Al-Rawi, A.; Grepin, K.; Li, X.; Morgan, R.; Wenham, C.; and Smith, J. 2021. Investigating public discourses around gender and COVID-19: a social media analysis of Twitter data. Journal of Healthcare Informatics Research, 5(3): 249–269.
- Alowibdi, Buy, and Yu (2013) Alowibdi, J. S.; Buy, U. A.; and Yu, P. 2013. Language independent gender classification on Twitter. In Proceedings of the 2013 IEEE/ACM international conference on advances in social networks analysis and mining, 739–743.
- Andrew (2020) Andrew, S. 2020. Florida observes Pulse Remembrance Day on the fourth anniversary of the Pulse nightclub shooting. CNN.
- Ansara and Hegarty (2014) Ansara, Y. G.; and Hegarty, P. 2014. Methodologies of misgendering: Recommendations for reducing cisgenderism in psychological research. Feminism & Psychology, 24(2): 259–270.
- Bamman, Eisenstein, and Schnoebelen (2014) Bamman, D.; Eisenstein, J.; and Schnoebelen, T. 2014. Gender identity and lexical variation in social media. Journal of Sociolinguistics, 18(2): 135–160.
- Baron (2020) Baron, D. 2020. What’s Your Pronoun?: Beyond He and She. Liveright Publishing.
- Berger (2019) Berger, M. 2019. A guide to how gender-neutral language is developing around the world. The Washington Post.
- Bisgin, Agarwal, and Xu (2012) Bisgin, H.; Agarwal, N.; and Xu, X. 2012. A study of homophily on social media. World Wide Web, 15(2): 213–232.
- Boyd, Golder, and Lotan (2010) Boyd, D.; Golder, S.; and Lotan, G. 2010. Tweet, tweet, retweet: Conversational aspects of retweeting on twitter. In 2010 43rd Hawaii International Conference on System Sciences, 1–10. IEEE.
- Brown (2017) Brown, A. 2017. Republicans, Democrats have starkly different views on transgender issues. Pew Research Center.
- Brown (2022a) Brown, A. 2022a. About 5% of young adults in the U.S. say their gender is different from their sex assigned at birth. Pew Research Center.
- Brown (2022b) Brown, A. 2022b. Deep partisan divide on whether greater acceptance of transgender people is good for society. Pew Research Center.
- Brown et al. (2020) Brown, C.; Frohard-Dourlent, H.; Wood, B. A.; Saewyc, E.; Eisenberg, M. E.; and Porta, C. M. 2020. “It makes such a difference”: An examination of how LGBTQ youth talk about personal gender pronouns. Journal of the American Association of Nurse Practitioners, 32(1): 70–80.
- Budge, Adelson, and Howard (2013) Budge, S. L.; Adelson, J. L.; and Howard, K. A. 2013. Anxiety and depression in transgender individuals: the roles of transition status, loss, social support, and coping. Journal of Consulting and Clinical Psychology, 81(3): 545.
- Cameron (1998) Cameron, D. 1998. Gender, language, and discourse: A review essay. Signs: Journal of Women in Culture and Society, 23(4): 945–973.
- Chen et al. (2020) Chen, E.; Lerman, K.; Ferrara, E.; et al. 2020. Tracking social media discourse about the COVID-19 pandemic: Development of a public coronavirus Twitter data set. JMIR public health and surveillance, 6(2): e19273.
- Chen (2021) Chen, T.-P. 2021. Why gender pronouns are becoming a big deal at work. The Wall Street Journal.
- Christakis and Fowler (2013) Christakis, N. A.; and Fowler, J. H. 2013. Social contagion theory: examining dynamic social networks and human behavior. Statistics in medicine, 32(4): 556–577.
- Ciot, Sonderegger, and Ruths (2013) Ciot, M.; Sonderegger, M.; and Ruths, D. 2013. Gender inference of Twitter users in non-English contexts. In EMNLP ’13, 1136–1145.
- Deaux (1985) Deaux, K. 1985. Sex and gender. Annual Review of Psychology.
- Devin-Norelle (2020) Devin-Norelle. 2020. Gender-neutral pronouns 101: Everything you’ve always wanted to know.
- Diamond (2020) Diamond, L. M. 2020. Gender fluidity and nonbinary gender identities among children and adolescents. Child Development Perspectives, 14(2): 110–115.
- Elks (2021) Elks, S. 2021. Why Twitter and Instagram are inviting people to share their pronouns. Thomas Reuters Foundation News.
- Ferrara and Yang (2015a) Ferrara, E.; and Yang, Z. 2015a. Measuring emotional contagion in social media. PloS one, 10(11): e0142390.
- Ferrara and Yang (2015b) Ferrara, E.; and Yang, Z. 2015b. Quantifying the Effect of Sentiment on Information Diffusion in Social Media. PeerJ Computer Science, 1: e26.
- Fink, Kopecky, and Morawski (2012) Fink, C.; Kopecky, J.; and Morawski, M. 2012. Inferring gender from the content of tweets: A region specific example. In Proceedings of the International AAAI Conference on Web and Social Media, volume 6, 459–462.
- Fosch-Villaronga et al. (2021) Fosch-Villaronga, E.; Poulsen, A.; Søraa, R. A.; and Custers, B. 2021. A little bird told me your gender: Gender inferences in social media. Information Processing & Management, 58(3): 102541.
- Gauntlett (2008) Gauntlett, D. 2008. Media, gender and identity: An introduction. Routledge.
- Geiger and Graf (2019) Geiger, A.; and Graf, N. 2019. About one-in-five U.S. adults know someone who goes by a gender-neutral pronoun. Pew Research Center.
- Goffman (1977) Goffman, E. 1977. The arrangement between the sexes. Theory and society, 4(3): 301–331.
- Goldberg (2020) Goldberg, B. 2020. Singular ‘they’ is voted Word of the Decade by U.S. linguists.
- Grover and Leskovec (2016) Grover, A.; and Leskovec, J. 2016. node2vec: Scalable feature learning for networks. In ACM SIGKDD ’16, 855–864.
- Gustafsson Sendén, Renström, and Lindqvist (2021) Gustafsson Sendén, M.; Renström, E.; and Lindqvist, A. 2021. Pronouns beyond the binary: The change of attitudes and use over time. Gender & Society, 35(4): 588–615.
- Gyamerah et al. (2021) Gyamerah, A. O.; Baguso, G.; Santiago-Rodriguez, E.; Sa’id, A.; Arayasirikul, S.; Lin, J.; Turner, C. M.; Taylor, K. D.; McFarland, W.; Wilson, E. C.; et al. 2021. Experiences and factors associated with transphobic hate crimes among transgender women in the San Francisco Bay Area: comparisons across race. BMC Public Health, 21(1): 1–15.
- Haas et al. (2010) Haas, A. P.; Eliason, M.; Mays, V. M.; Mathy, R. M.; Cochran, S. D.; D’Augelli, A. R.; Silverman, M. M.; Fisher, P. W.; Hughes, T.; Rosario, M.; et al. 2010. Suicide and suicide risk in lesbian, gay, bisexual, and transgender populations: Review and recommendations. Journal of Homosexuality, 58(1): 10–51.
- Hekanaho (2020) Hekanaho, L. 2020. Generic and nonbinary pronouns: Usage, acceptability and attitudes. Ph.D. thesis, University of Helsinki.
- Hodas and Lerman (2014) Hodas, N. O.; and Lerman, K. 2014. The simple rules of social contagion. Scientific Reports, 4(1): 1–7.
- Hosking (2021) Hosking, G. 2021. What are gender pronouns and why is it important to use the right ones? The Conversation.
- HRC Foundation (2020) HRC Foundation. 2020. An epidemic of violence: Fatal violence against transgender and gender non-conforming people In the U.S. in 2020. Human Rights Campaign.
- Karami, Webb, and Kitzie (2018) Karami, A.; Webb, F.; and Kitzie, V. L. 2018. Characterizing transgender health issues in Twitter. Proceedings of the Association for Information Science and Technology, 55(1): 207–215.
- Katz-Wise (2020) Katz-Wise, S. L. 2020. Gender fluidity: What it means and why support matters. Harvard Health Publishing.
- Keyes (2018) Keyes, O. 2018. The misgendering machines: Trans/HCI implications of automatic gender recognition. PACM HCI, 2(CSCW): 1–22.
- Keyes, May, and Carrell (2021) Keyes, O.; May, C.; and Carrell, A. 2021. You keep using that word: Ways of thinking about gender in computing research. PACM HCI, 5(CSCW1): 1–23.
- Knutson, Koch, and Goldbach (2019) Knutson, D.; Koch, J. M.; and Goldbach, C. 2019. Recommended terminology, pronouns, and documentation for work with transgender and non-binary populations. Practice Innovations, 4(4): 214.
- Kolodziej (2019) Kolodziej, C. 2019. The Importance of trans-sensitive police policies and practices. Policing Project.
- Kossinets and Watts (2009) Kossinets, G.; and Watts, D. J. 2009. Origins of homophily in an evolving social network. American journal of sociology, 115(2): 405–450.
- Kramer, Guillory, and Hancock (2014) Kramer, A. D.; Guillory, J. E.; and Hancock, J. T. 2014. Experimental evidence of massive-scale emotional contagion through social networks. Proceedings of the National Academy of Sciences, 111(24): 8788–8790.
- Krueger and Young (2015) Krueger, E. A.; and Young, S. D. 2015. Twitter: a novel tool for studying the health and social needs of transgender communities. JMIR Mental Health, 2(2): e4113.
- Leavitt et al. (2009) Leavitt, A.; Buchard, E.; Fischer, D.; and Gilbert, S. 2009. The influentials: New approaches for analyzing influence on twitter. The Web Ecology Project.
- Levietes (2022) Levietes, M. 2022. Is the word ‘women’ being erased from the abortion rights movement? NBC News.
- Levin (2018) Levin, R. N. 2018. The problem with pronouns. Inside Higher Ed.
- Lipka and Tevington (2022) Lipka, M.; and Tevington, P. 2022. Attitudes about transgender issues vary widely among Christians, religious ‘nones’ in U.S. Pew Research Center.
- Liu and Ruths (2013) Liu, W.; and Ruths, D. 2013. What’s in a name? Using first names as features for gender inference in Twitter. In 2013 AAAI Spring Symposium Series.
- Longman (2022) Longman, J. 2022. Sport is again divided over inclusiveness and a level playing field. The New York Times.
- Marwick (2013) Marwick, A. 2013. Gender, sexuality, and social media. In The social media handbook, 67–83. Routledge.
- McPherson, Smith-Lovin, and Cook (2001) McPherson, M.; Smith-Lovin, L.; and Cook, J. M. 2001. Birds of a feather: Homophily in social networks. Annual Review of Sociology, 415–444.
- Metaxas et al. (2015) Metaxas, P.; Mustafaraj, E.; Wong, K.; Zeng, L.; O’Keefe, M.; and Finn, S. 2015. What do retweets indicate? Results from user survey and meta-review of research. In Proceedings of the international AAAI conference on web and social media, 658–661.
- Mønsted et al. (2017) Mønsted, B.; Sapieżyński, P.; Ferrara, E.; and Lehmann, S. 2017. Evidence of complex contagion of information in social media: An experiment using Twitter bots. PloS one, 12(9): e0184148.
- Moser and Devereux (2019) Moser, C.; and Devereux, M. 2019. Gender neutral pronouns: A modest proposal. The International Journal of Transgenderism, 20(2-3): 331.
- Nguyen, Vu, and Tuan Nguyen (2020) Nguyen, D. Q.; Vu, T.; and Tuan Nguyen, A. 2020. BERTweet: A pre-trained language model for English Tweets. In EMNLP ’20, 9–14. Association for Computational Linguistics.
- Pachucki, Jacques, and Christakis (2011) Pachucki, M. A.; Jacques, P. F.; and Christakis, N. A. 2011. Social network concordance in food choice among spouses, friends, and siblings. American Journal of Public Health, 101(11): 2170–2177.
- Parker, Horowitz, and Brown (2022) Parker, K.; Horowitz, J. M.; and Brown, A. 2022. Americans’ complex views on gender identity and transgender issues. Pew Research Center.
- Parks, O’Connor, and Parrish (2016) Parks, R.; O’Connor, M.; and Parrish, J. 2016. Gender pronouns: Recommendations from an institution with solutions. College and University, 91(4): 83.
- Reid (2015) Reid, G. 2015. Outlawed: Legal discrimination against gays and lesbians. Human Rights Watch.
- Rosenquist, Fowler, and Christakis (2011) Rosenquist, J. N.; Fowler, J. H.; and Christakis, N. A. 2011. Social network determinants of depression. Molecular Psychiatry, 16(3): 273–281.
- Rotondi et al. (2012) Rotondi, N. K.; Bauer, G. R.; Travers, R.; Travers, A.; Scanlon, K.; and Kaay, M. 2012. Depression in male-to-female transgender Ontarians: results from the Trans PULSE Project. Canadian Journal of Community Mental Health, 30(2): 113–133.
- Shalizi and Thomas (2011) Shalizi, C. R.; and Thomas, A. C. 2011. Homophily and contagion are generically confounded in observational social network studies. Sociological Methods & Research, 40(2): 211–239.
- Smith (2017) Smith, G. A. 2017. Views of transgender issues divide along religious lines. Pew Research Center.
- Stryker and Whittle (2013) Stryker, S.; and Whittle, S. 2013. The Transgender Studies Reader. Routledge.
- Thelwall, Thelwall, and Fairclough (2021) Thelwall, M.; Thelwall, S.; and Fairclough, R. 2021. Male, female, and nonbinary differences in UK Twitter self-descriptions: A fine-grained systematic exploration. Journal of Data and Information Science, 6(2): 1–27.
- Thornhill (2019) Thornhill, T. 2019. ‘I identify as a suitcase. Can I fly for free now?’ United Airlines is mocked after becoming first in the world to allow passengers to identify as gender neutral Mx. DailyMail.com.
- Toomey, Syvertsen, and Shramko (2018) Toomey, R. B.; Syvertsen, A. K.; and Shramko, M. 2018. Transgender adolescent suicide behavior. Pediatrics, 142(4).
- Tsvetkova and Macy (2014) Tsvetkova, M.; and Macy, M. W. 2014. The social contagion of generosity. PloS ONE, 9(2): e87275.
- Tuah and Mazlan (2020) Tuah, K. M.; and Mazlan, U. S. 2020. Twitter as safe space for self-disclosure among Malaysian LGBTQ Youths. Jurnal Komunikasi: Malaysian Journal of Communication, 36(1): 436–448.
- Tucker and Jones (2022) Tucker, L.; and Jones, J. J. 2022. Pronoun Lists in Profile Bios Display Increased Prevalence, Systematic Co-Presence with Other Keywords and Network Tie Clustering among US Twitter Users 2015-2022.
- Twenge, Campbell, and Gentile (2013) Twenge, J. M.; Campbell, W. K.; and Gentile, B. 2013. Changes in pronoun use in American books and the rise of individualism, 1960-2008. Journal of Cross-Cultural Psychology, 44(3): 406–415.
- United Nations Human Rights (n.d.) United Nations Human Rights. n.d. The struggle of trans and gender-diverse persons. United Nations.
- University of Waterloo (n.d.) University of Waterloo. n.d. Gender pronouns and teaching.
- VanderWeele and An (2013) VanderWeele, T. J.; and An, W. 2013. Social networks and causal inference. Handbook of Causal Analysis for Social Research, 353–374.
- Witt and Wood (2010) Witt, M. G.; and Wood, W. 2010. Self-regulation of gendered behavior in everyday life. Sex Roles, 62(9): 635–646.
- Wojcik and Hughes (2019) Wojcik, S.; and Hughes, A. 2019. Sizing up Twitter users. Pew Research Center.
- Wolf (2000) Wolf, A. 2000. Emotional expression online: Gender differences in emoticon use. Cyberpsychology & Behavior, 3(5): 827–833.
- Zheng et al. (2016) Zheng, W.; Yuan, C.-H.; Chang, W.-H.; and Wu, Y.-C. J. 2016. Profile pictures on social media: Gender and regional differences. Computers in Human Behavior, 63: 891–898.
Appendix A Appendix
All Pronoun Keywords
We use the following list of keywords to match pronouns used in the texts for the RQ2 experiments conducted in §5:
-
•
I: I, me, mine, my, myself
-
•
You: you, your, yours yourself, yourselves
-
•
We: we, us, our, ours, ourself, ourselves
-
•
He: he him his himself,
-
•
She: she, her, hers, herself
-
•
It: it, its, itself
-
•
They: they, them, their, theirs, xe, xem, ze, zem
Node2vec
We apply the node2vec algorithm (Grover and Leskovec 2016) on the networks generated for the RQ3 experiments conducted in §6, treating networks as weighted and directed. We use the default parameters, which fixes the dimension size to 128, the length of the walk per source to 80, the number of walks per source to 10, the context size to 10, the return hyperparameter to 1, the in-out hyperparameter to 1, and the number of epochs to 1.
Deep Neural Network Architecture
The model architecture includes an input normalization layer, followed by a variable number of hidden layers. Each hidden layer is preceded by a dropout layer and succeeded by a normalization layer. The activation functions are ReLU. We use the Adam optimizer. The data is trained with batch sizes of 4096. All models are trained for 100 epochs with early stopping imposed if the validation loss stops reducing.
The binary classification models use the sigmoid activation function as the final layer activation and are optimized via binary cross-entropy loss. The multiclass classification models use the softmax activation as the final layer activation and are optimized via categorical cross-entropy loss.
Hyperparameter Tuning
For every prediction task, we conduct hyperparameter tuning using 40 randomized combinations of the following hyperparameters:
-
•
Number of layers: {2, 4, 16}
-
•
Hidden units: {32, 128, 768}
-
•
L2 regularization: {, , }
-
•
Learning rates: {, , }
-
•
Dropout: {0.1, 0.2, 0.3}