VoLTA: Vision-Language Transformer with
Weakly-Supervised Local-Feature Alignment
Abstract
Vision-language pre-training (VLP) has recently proven highly effective for various uni- and multi-modal downstream applications. However, most existing end-to-end VLP methods use high-resolution image-text-box data to perform well on fine-grained region-level tasks, such as object detection, segmentation, and referring expression comprehension. Unfortunately, such high-resolution images with accurate bounding box annotations are expensive to collect and use for supervision at scale. In this work, we propose VoLTA (Vision-Language Transformer with weakly-supervised local-feature Alignment), a new VLP paradigm that only utilizes image-caption data but achieves fine-grained region-level image understanding, eliminating the need for expensive box annotations. VoLTA adopts graph optimal transport-based weakly-supervised alignment on local image patches and text tokens to germinate an explicit, self-normalized, and interpretable low-level matching criterion. In addition, VoLTA pushes multi-modal fusion deep into the uni-modal backbones during pre-training and removes fusion-specific transformer layers, further reducing memory requirements. Extensive experiments on a wide range of vision- and vision-language downstream tasks demonstrate the effectiveness of VoLTA on fine-grained applications without compromising the coarse-grained downstream performance, often outperforming methods using significantly more caption and box annotations. Code and pre-trained model are available at https://github.com/ShramanPramanick/VoLTA. ††footnotetext: ∗Equal technical contribution. ††footnotetext: †Part of this work was done during an internship at Meta.
1 Introduction
Inspired by the escalating unification of transformer-based modeling in vision (Dosovitskiy et al., 2021; Liu et al., 2021; Chen et al., 2021a) and language (Devlin et al., 2019; Liu et al., 2019) domains, coupled with readily available large-scale image-caption pair data, vision-language pre-training (VLP) (Lu et al., 2019; Li et al., 2020a; Kim et al., 2021; Kamath et al., 2021; Zhang et al., 2021) has recently been receiving increasing attention. VLP has not only been proven the de-facto for several vision-language tasks, but it has also been beneficial for traditional vision-only tasks, such as image classification and object detection. Such wide-range applications of VLP can broadly be categorized into two groups: tasks requiring image-level understanding, e.g., image classification, image & text retrieval (Plummer et al., 2015), image captioning (Zhou et al., 2020), visual question answering (Antol et al., 2015), and tasks requiring region-level understanding, e.g., object detection, instance segmentation, and referring expression comprehension (Kazemzadeh et al., 2014; Yu et al., 2016). Most existing VLP methods address only one group of application, leaving the question of a generalizable and unified VL framework under-explored.
Traditional VLP methods with image-level understanding (Li et al., 2021a; Wang et al., 2021b; Dou et al., 2022b) utilize large-scale image-caption pair datasets and are commonly trained with image-text contrastive objectives computed on global features. Hence, it is not trivial to extend such methods to region-level applications. On the other hand, VLP methods with region-level understanding (Kamath et al., 2021; Li et al., 2022c; Zhang et al., 2022) use image-text-box grounding data and are designed to predict bounding boxes during pre-training. Consequently, they do not support image-level tasks. Furthermore, accurate bounding box annotations require high-resolution input images, which are often expensive to collect, annotate and use for pre-training at scale. Recently, FIBER (Dou et al., 2022a) addressed the problem of such unified VLP and proposed a two-stage pre-training algorithm requiring fewer box annotations than previous region-level pre-training methods. Moving a step forward, as shown in Figure 1, we aim to eliminate the use of costly box annotations and ask the challenging but natural question: Can we attain region-level understanding from global image-caption annotations and unify image- and region-level tasks in a single VL framework?
Subsequently, we focus on achieving region-level fine-grained understanding by weakly-supervised alignment of image patches and text tokens. Previous VLP methods (Chen et al., 2020d; Kim et al., 2021) in this direction use Wasserstein distance (WD) (Peyré et al., 2019), a.k.a Earth Mover’s distance (EMD)-based optimal transport (OT) algorithms for such alignment problems. However, we argue that WD is not optimum for images with multiple similar entities. Thus, we propose to jointly utilize Gromov-Wasserstein distance (GWD) (Peyré et al., 2016) and Wasserstein distance (WD) in a setup known as graph optimal transport (Chen et al., 2020a). Moreover, instead of using a commonly deployed contrastive objective, we propose to use redundancy reduction from Barlow Twins (Zbontar et al., 2021), which is less data-intensive and does not require hard-negative mining. We also follow Dou et al. (2022a) and incorporate deep multi-modal fusion into the uni-modal backbones, removing the need for costly fusion-specific transformer layers. These steps when integrated yield VoLTA, Vision-Language Transformer with weakly-supervised local-feature Alignment, a unified VLP paradigm only utilizes image-caption annotations but achieves fine-grained region-level image understanding, eliminating the need for expensive box annotations. Figure 4 visualizes the feature-level image-text alignment generated by VoLTA, which can attend text tokens to the corresponding visual patches without relying on low-level supervision.
In summary, our contributions are three-fold. We propose to use graph optimal transport for weakly-supervised feature-level patch-token alignment in VLP. We introduce VoLTA, a unified VLP paradigm for image-level and region-level applications, but pre-trained only using image-caption pairs. VoLTA is memory, compute, and time-efficient and can easily be scaled up with readily available large-scale image-caption data harvested from the web. We present the results of a wide range of vision- and vision-language coarse- and fine-grained downstream experiments to demonstrate the effectiveness of VoLTA compared to strong baselines pre-trained with significantly more caption and box annotations.
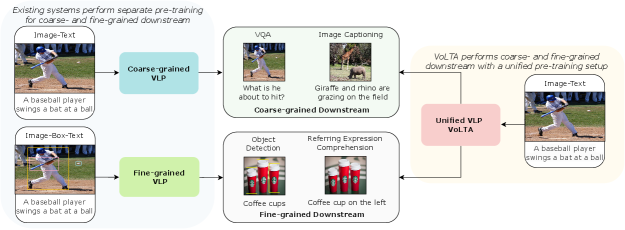
2 Related Works
Uni-modal Self-supervised Pre-training: In recent years, the machine learning community has observed a boom in self-supervised pre-training. In the language domain, representations learned by BERT (Devlin et al., 2019), RoBERTa (Liu et al., 2019) have become the default setting for many downstream tasks. Generative models such as GPT (Radford et al., 2019; Brown et al., 2020) have also achieved impressive few-shot/zero-shot performances on novel applications. SimCSE (Gao et al., 2021) uses contrastive learning to help learn useful sentence representations.
In the vision domain, several contrastive/joint-embedding methods (He et al., 2020; Chen et al., 2020c; 2021b; b; Grill et al., 2020; Chen & He, 2021; Caron et al., 2021; Zbontar et al., 2021; Bardes et al., 2022; Shah et al., 2022; Assran et al., 2022) have outperformed supervised counterparts. Recently, generative models such as BEiT (Bao et al., 2021) and MAE (He et al., 2022) have also achieved impressive performances with much more scalable potential.
Vision-Language Pre-training (VLP): Vision-language pre-training mainly relies on image-text pair datasets to learn joint visual-language representations. One line of work is to train separate vision and language encoders and only fuse in the representation space. CLIP (Radford et al., 2021), UniCL (Yang et al., 2022a), and ALIGN (Jia et al., 2021) use the image-text contrastive loss to learn aligned representations. SLIP (Mu et al., 2021) combines self-supervised visual representation learning and contrastive multi-modal learning. M3AE (Geng et al., 2022), FLAVA (Singh et al., 2022) combines masked image modeling and masked language modeling. Another line of work uses cross attention to fuse vision and language information in the early stage (Kamath et al., 2021; Dou et al., 2022b; Lu et al., 2019; Li et al., 2020b; Kiela et al., 2019; Kim et al., 2021; Zhang et al., 2021; Li et al., 2022b; Wang et al., 2022c; Pramanick et al., 2023; Park & Han, 2023; Li et al., 2023a; Jang et al., 2023; Wang et al., 2023a). These works focus on learning semantic-level aligned vision-language representations. In addition, UniTAB (Yang et al., 2022c), OFA (Wang et al., 2022b), GLIP (Li et al., 2022c), and FIBER (Dou et al., 2022a) use expensive grounding image-text-box annotations to learn the fine-grained aligned representations. Our work uses representation space alignment and cross-attention fusion, but we do not use any box annotation to learn robust feature-level alignments.
Unsupervised Representation Alignment: Unsupervised multi-modal alignment typically relies on specific metrics. Wasserstein distance (Peyré et al., 2019), a.k.a EMD-based optimal transport (OT) algorithms have been widely adopted to various domain alignment tasks, including sequence-to-sequence learning (Chen et al., 2019), few-shot learning (Zhang et al., 2020), knowledge distillation (Balaji et al., 2019), unsupervised domain adaptation (Balaji et al., 2019), generative networks (Han et al., 2015; Genevay et al., 2018; Mroueh et al., 2018; 2019), and multi-modal learning (Yuan et al., 2020; Chen et al., 2020d; Kim et al., 2021; Li et al., 2022d; Pramanick et al., 2022). Previous VLP methods (Chen et al., 2020d; Kim et al., 2021), which use OT-based patch-word alignment, only utilize the Wasserstein distance. However, we argue that jointly modeling GWD (Peyré et al., 2016) and WD results in a superior multi-modal alignment for intricate images. To the best of our knowledge, this is the first work to apply WD and GWD-based optimal transport for feature-level alignment in VLP.
3 Proposed System - VoLTA
In this section, we present our proposed approach, VoLTA, which contains three broad modules - intra- and inter-modality redundancy reduction, weekly-supervised cross-modal alignment of local features, and cross-modal attention fusion (CMAF). Next, we introduce the fine-tuning strategies for various uni- and multi-modal downstream tasks as supported by VoLTA. An overview of the different modules of VoLTA is presented in Figure 2.
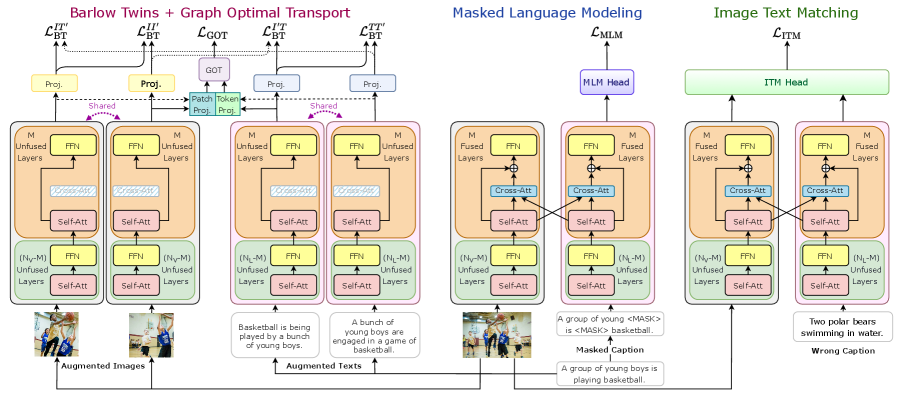
3.1 Intra- & Inter-modality Redundancy Reduction
We use Barlow Twins (BT) (Zbontar et al., 2021), a non-contrastive covariance regularization as the foundational objective of VoLTA. The recent success of contrastive vision-language pre-training (Radford et al., 2021; Li et al., 2021b; Jia et al., 2021; Kim et al., 2021; Yang et al., 2022a; Dou et al., 2022a; b) has already shown that, compared to a single modality, image-caption pairs offer a significantly higher-level of abstractive and semantic concepts about the training samples. However, common contrastive VLP objectives, like InfoNCE (Oord et al., 2018), are data-hungry, as they require large batch sizes and well-mined hard negatives. On the other hand, the BT objective operates on the dimensions of the embeddings across the two views of training samples. Hence, it is more robust to batch size and can be trained using lower memory resources. In this work, we extend the BT objective for a multi-modal setup.
The original BT algorithm, which operates on joint embeddings of distorted samples, was proposed only for image modality. Specifically, for each image of a batch , two distorted views are obtained using a distribution of data augmentation with disparate probabilities. These distorted images are then fed into a shared image encoder containing a feature extraction network (e.g., ResNet (He et al., 2016)) cascaded with trainable linear projection layers, producing a batch of parallel embeddings and . The BT loss computed using the encoded embeddings can be denoted as:
(1) |
is a positive weighting factor; is the cross-correlation matrix computed between and along the batch dimension; stands for sample indices in a batch; refers to the dimension indices of and . The first term in Equation 1 is the invariance term which attempts to equate the diagonal elements of the cross-correlation matrix to , whereas the second term is the redundancy reduction term which pushes the off-diagonal elements of matrix to .
In this work, we use BT for image-caption pairs. Specifically, we use stochastic data augmentations for both images and text111Augmentation details are provided in Appendix D.1., and directly apply the BT objective for all the pairs, resulting in additional supervision. Note this simple, straightforward, and instinctive extension enables us to apply redundancy reduction in between and across modalities, which intuitively results in superior visual representation. Moreover, in this bi-modal setting, we can pre-train a text encoder in parallel with the image encoder and, thus, can generalize our system to a broader range of uni- and multi-modal downstream applications.
Intra-modal Objective: Intra-modal objective refers to applying the BT loss in-between pairs of image and text embeddings. Given an image-caption pair, we first have two augmented views for each image, and two augmented views for each text. Then, we resort to Equation 1 individually for the image and text pairs.
(2) |
Inter-modal Objective: Inter-modal objective refers to applying the BT loss across image and text embeddings. Since the image and text encoders can output features with different shapes, we design the projector layers with same output dimension. Hence, in addition to the original BT loss between in Zbontar et al. (2021), we get three more loss terms - , , , leading to diverse and high-quality additional supervision. The inter-modal BT losses can be directly computed following Equation 1.
(3) |
The resulting bi-modal BT loss is , .
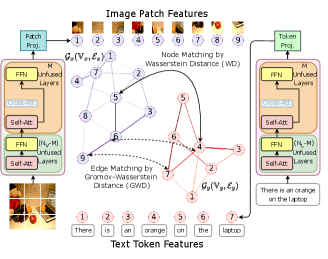
3.2 Alignment of Local Features
Though the inter-modal redundancy reduction provides high-quality semantic supervision, it is computed on the global image- and text features and, thus, only simulates implicit and non-interpretable multi-modal alignment. However, fine-grained region-level downstream applications like detection, segmentation, and reference expression comprehension require local visual feature descriptors with specific spatial information. To achieve this, most existing top-performing VLP methods, including UniTAB (Yang et al., 2022c), OFA (Wang et al., 2022b), GLIP (Li et al., 2022c), and FIBER (Dou et al., 2022a), use high-resolution image-text-box data for fine-grained pre-training. However, bounding box annotations are expensive to collect and use for supervision. Hence, we seek an alternate weekly-supervised solution for local feature-level alignment using global image-caption annotations.
Recently, WD (Peyré et al., 2019), a.k.a EMD-based OT algorithms have been used for weakly-supervised patch-word alignment in VLP (Chen et al., 2020d; Kim et al., 2021). Such OT-based learning methods are optimized for distribution matching by minimizing the cost of a transport plan. We pose the patch-word alignment as a more structured graph-matching problem and use the graph optimal transport (GOT) algorithm, which utilizes GWD (Peyré et al., 2016) in conjunction with WD to ensure the preservation of topological information during cross-modal alignment. More specifically, we obtain the patch- and token-level features from the last layers of corresponding visual and textual transformer encoders, and use these encoded local-feature vectors to construct modality-specific dynamic graphs - for image patches and for text tokens. Each node in these graphs is represented by corresponding feature vectors, and intermediate edges by thresholded cosine similarity.
Importance of GOT in Patch-Word Alignment: As mentioned previously, GOT adopts two types of OT distances - WD for node matching and GWD for edge matching. In contrast, previous vision-language pre-training algorithms using OT for patch-word alignment only considered WD (Chen et al., 2020d; Kim et al., 2021). However, we argue that intricate images with multiple objects with similar shapes and colors require both WD and GWD for accurate, fine-grained matching. For example, in Figure 3, there are multiple “orange" present in the image. WD can only match nodes in the graph, and will treat all “orange" entities as identical and will ignore neighboring relations like “on the laptop". However, by using proper edge matching with GWD, we can preserve the graph’s topological structure. We can correctly identify which “orange" in the image the sentence is referring to. Hence, we couple WD and GWD mutually beneficially and use a joint transport plan for accurate patch-word matching.
Once and are computed, we follow Chen et al. (2020a) to compute WD and GWD.
Wasserstein Distance calculates the pairwise distances between two sets of cross-domain node embeddings. Consider two discrete distributions, and , where and ; and being the Delta-Dirac function centered on . Since and are both probability distributions, sum of weight vectors is , . The WD distance between and is defined as:
(4) |
where , is cosine distance metric, and is the transport plan, interpreting the amount of mass shifted from to .
Gromov-Wasserstein Distance assists in edge matching and preserves graph topology by calculating distances between pairs of nodes in each domain and measuring how these distances compare to the counter domain. In the same discrete graph matching setting, GWD between and can be mathematically represented as:
(5) |
where intra-graph structural similarity between two node pairs and is represented as , being cosine similarity between a node pair in any graph . Transport plan is periodically updated to align the edges in different graphs belonging to disparate modalities.
We further follow Chen et al. (2020a) to combine WD and GWD transport plans, leading to a unified GOT objective given as:
(6) |
where regulates the importance of two loss terms.
3.3 Cross-Modal Attention Fusion (CMAF)
BT and GOT losses are computed in a dual encoder setting, which does not contain cross-modal interactions and is not suitable for complex multi-modal feature representation. Most existing methods, including UNITER (Chen et al., 2020d), ViLT (Kim et al., 2021), METER (Dou et al., 2022b), and GLIP (Li et al., 2022c) design cross-modal fusion by stacking additional transformer layers on top of uni-modal encoders, introducing a large number of added parameters during pre-training. We follow a more efficient solution proposed by FIBER (Dou et al., 2022a), which inserts cross-modal fusion into the uni-modal backbones with a gating mechanism. Specifically, at the top transformer layers in the vision and language backbone, cross-attention signals, weighted by a gating scalar , are added to self-attention:
(7) | ||||
where is a trainable parameter initialized to . Following existing literature (Li et al., 2021a; Wang et al., 2021a; Dou et al., 2022b; a), we use masked language modeling (MLM) and image-text matching (ITM) to pre-train the cross-attention parameters. For MLM, we randomly mask text tokens, and the loss aims to reconstruct the masked tokens. We feed the network with randomly sampled image-caption pairs for ITM, and the loss predicts whether they are matched. The gating mechanism is a good choice for CMAF because cross-attention parameters can easily be switched off by setting the gating scalar to when computing the BT and GOT losses. Thus, we can learn the cross-attention parameters without affecting the original computational flow of uni-modal backbones. gating mechanism is more lightweight and memory-efficient than adding fusion-specific layers (GLIP and METER use 4 more fusion parameters than VoLTA).
Overall, VoLTA training pipeline can be summarized in the following three steps:
-
•
BT & GOT: CMAF is switched off , VoLTA acts as dual encoder, and are computed.
-
•
MLM & ITM: CMAF is switched on , VoLTA now acts as fusion encoder, and losses are computed.
-
•
Back-propagation: the four losses are added, giving , and back-propagated into the model end-to-end. An ablation on different pre-training objectives of VoLTA and values of is given in Section 4.7.
3.4 Finetuning For Downstream Tasks
We adopt VoLTA to various vision- and vision-language downstream tasks. We switch off the inserted cross-attention modules for the vision-only tasks and use the image encoder. We utilize the learned cross-attention parameters as required for the vision-language tasks, following Dou et al. (2022a). For example, VQA and visual reasoning employ all cross-attention modules, whereas captioning requires only image-to-text cross-attention. Again, during IRTR, we switch off all cross-attentions and use VoLTA in a dual encoder setting. We keep all cross-attention parameters during multi-modal object detection and referring expression comprehension and train an object detection head from scratch using the language-aware image features.
4 Experiments, Results, and Analysis
4.1 Pre-training & Downstream datasets
Following Chen et al. (2020d) and Huang et al. (2021), we perform pre-training by appending the VG dataset (Krishna et al., 2017) with COCO (Lin et al., 2014), together consisting of k images. We divide our downstream tasks into three categories - Uni-modal tasks such as image classification on ImageNet (Deng et al., 2009), VOC (Everingham et al., 2010), COCO; object detection on VOC, COCO, and instance segmentation on COCO. Multi-modal fine-grained tasks such as region-level VL tasks - referring expression comprehension (REC) on RefCOCO, RefCOCO, RefCOCOg (Kazemzadeh et al., 2014; Yu et al., 2016), and language-conditioned object detection on COCO and LVIS (Gupta et al., 2019). Multi-modal coarse-grained tasks such as image-level VL tasks - visual question answering on VQAv (Antol et al., 2015), visual reasoning on NLVR2 (Suhr et al., 2019), image- and text retrieval on Flickerk (Plummer et al., 2015) and captioning on COCO. We exclude any overlap between our pre-training and downstream validation/test splits. Detailed statistics of all downstream datasets are given in Appendix C.
4.2 Network Architectures
Following FIBER (Dou et al., 2022a), we adopt Swin-Base (Liu et al., 2021) and RoBERTa-Base (Liu et al., 2019) as our vision and text encoders, which are initialized with weights from uni-modal pre-training. We collect patch- and token features from the last transformer layers, feed them into the local projector network, and compute GOT loss. Furthermore, we apply AvgPool on patch and token features, feed them into the global projector network, and compute BT loss. Both local and global projector networks have three linear layers with dimensions --, with batch normalization and ReLU after the first two layers. Section 4.7 gives an ablation on projector dimension. We use the image and text features after the AvgPool layer during downstream tasks. For CMAF, we insert the cross-attention into the top 6 blocks of the vision and text encoders. Moreover, for direct comparison with existing uni-modal baselines, we re-train VoLTA with ResNet (He et al., 2016) and Swin-Tiny image encoders.
Linear probing on ImageNet Validation Set | Linear probing on VOC and COCO | |||||||||
Method | Pre-train | Arch. | Pre-training Supervision | Top- | Method | Pre-train | Arch. | VOC | COCO | |
SVM | MLP | MLP (PC/O) | ||||||||
Sup. | IN-K | RN | Label | 76.5 | Sup. | IN-K | RN | 87.5 | 90.8 | 55.2/60.8 |
Sup. | IN-100 | RN | Label | 53.3† | BYOL | IN-K | RN | 86.6 | ||
MoCo | COCO | RN | NA | 44.5† | BT | IN-K | RN | 86.2 | 91.9‡ | 56.1/63.0‡ |
MoCo-v2 | COCO | RN | NA | 49.3† | VICReg | IN-K | RN | 86.6 | 91.1‡ | 51.0/57.9‡ |
CAST | COCO | RN50 | Caption | 48.7 | CAST | COCO | RN50 | 74.0 | 51.0/57.9 | |
VirTex | COCO | RN | Caption | 52.8 | VirTex | COCO | RN50 | 88.7 | ||
ICMLM | COCO | RN | Caption | 51.9 | ICMLM | COCO | RN50 | 87.5 | ||
MCT | COCO | RN | Caption | 54.9 | LocTex | COCO | RN50 | 88.4 | ||
MCT | COCO | RN | CaptionTag | 55.3 | LocTex | COCOOpenIm | RN50 | 92.6 | ||
VoLTA(w/o MLM, ITM) | COCO | RN | Caption | 55.3 | VoLTA(w/o MLM, ITM) | COCO | RN | 89.6 | 94.3 | 71.4/74.3 |
VoLTA(w/o MLM, ITM) | COCO | Swin-T | Caption | 56.3 | VoLTA(w/o MLM, ITM) | COCO | Swin-T | 88.2 | 93.5 | 73.4/75.7 |
VoLTA(w/o MLM, ITM) | COCO | Swin-B | Caption | 62.5 | VoLTA(w/o MLM, ITM) | COCO | Swin-B | 88.5 | 93.9 | 74.1/76.1 |
VoLTA | COCO | Swin-B | Caption | 62.5 | VoLTA | COCO | Swin-B | 89.7 | 95.0 | 74.5/76.4 |
Method | Pre-train | Arch. | Pre-training Supervision | VOC+ det | COCO det | COCO instance seg | ||||||
---|---|---|---|---|---|---|---|---|---|---|---|---|
APall | AP50 | AP75 | APbb | AP | AP | APmk | AP | AP | ||||
Sup. | IN-K | RN | Label | 53.5 | 81.3 | 58.8 | 38.2 | 58.2 | 41.2 | 33.3 | 54.7 | 35.2 |
MoCo-v† | IN-K | RN | NA | 57.4 | 82.5 | 64.0 | 39.3 | 58.9 | 42.5 | 34.4 | 55.8 | 36.5 |
MoCo | COCO | RN | NA | 47.5 | 75.4 | 51.1 | 38.5 | 58.5 | 42.0 | 35.0 | 55.6 | 37.5 |
MoCo-v | COCO | RN | NA | 48.4 | 75.5 | 52.1 | 39.8 | 59.6 | 43.1 | 35.8 | 56.9 | 38.8 |
CAST | COCO | RN50 | Caption | 54.2 | 80.1 | 59.9 | 39.4 | 60.0 | 42.8 | 35.8 | 57.1 | 38.6 |
VirTex | COCO | RN50 | Caption | 55.6 | 81.4 | 61.5 | 40.9 | 61.7 | 44.8 | 36.9 | 58.4 | 39.7 |
LocTex | COCO | RN50 | Caption | 53.9 | 80.9 | 59.8 | 40.6 | 60.6 | 44.1 | 35.2 | 57.0 | 37.4 |
MCT | COCO | RN50 | Caption | 56.1 | 82.1 | 62.4 | 41.1 | 61.8 | 44.9 | 36.9 | 58.2 | 40.0 |
VoLTA | COCO | RN50 | Caption | 56.6 | 84.4 | 62.7 | 41.9 | 61.8 | 44.8 | 36.5 | 58.5 | 40.8 |
Sup.‡ | IN-1K | Swin-T | Label | 50.5 | 69.3 | 54.9 | 43.7 | 66.6 | 47.1 | |||
MoBY‡ | IN-1K | Swin-T | NA | 50.2 | 68.8 | 54.7 | 43.5 | 66.1 | 46.9 | |||
VoLTA | COCO | Swin-T | Caption | 50.9 | 69.6 | 55.5 | 43.8 | 66.9 | 47.5 | |||
Sup.‡ | IN-1K | Swin-B | Label | 51.9 | 70.9 | 56.5 | 45.0 | 68.4 | 48.7 | |||
ViTDet | IN-1K | ViT-B | NA | 51.6 | 45.9 | |||||||
CLIP | LAION-20M | ViT-B | Caption | 45.2 | 40.4 | |||||||
SLIP | LAION-20M | ViT-B | Caption | 44.7 | 41.0 | |||||||
MaskCLIP | LAION-20M | ViT-B | Caption | 46.6 | 41.7 | |||||||
VoLTA | COCO | Swin-B | Caption | 52.1 | 71.3 | 56.6 | 45.2 | 68.5 | 49.0 |
4.3 Implementation Details
We perform pre-training for epochs with batch-size on V GPUs. Following Zbontar et al. (2021), we use the LARS optimizer (You et al., 2017) with a learning rate of for the weights and for the biases and batch normalization parameters. We use a learning rate warm-up period of epochs, after which we reduce the learning rate by a factor of using a cosine decay schedule (Loshchilov & Hutter, 2016). We use e weight decay, excluding the biases and batch normalization parameters. We conduct a grid search for the GOT loss hyperparameter (), and we empirically found the best value to be . Appendix D explains other necessary pre-training and downstream hyper-parameters details.
4.4 Results on Vision-only tasks
We first experiment on three uni-modal tasks - classification, object detection, and instance segmentation. For a direct comparison with existing ResNet and Swin-T baselines, we re-train identical encoders with VoLTA pipeline. Furthermore, since the uni-modal tasks do not utilize cross-attention parameters, we perform an ablation by dropping the MLM and ITM objectives from VoLTA.
Image Classification: Table 1 presents the linear probing results of uni-label classification on ImageNet and multi-label classification on VOC and COCO. For all uni-modal tasks, we report results with COCO pre-training for a fair comparison with existing baselines. For ImageNet, we adopt all COCO baselines from Yuan et al. (2021). Even without the MLM and ITM objectives, VoLTA achieves better performance than all baselines across three datasets with ResNet backbone. The Swin backbones and cross-attention module further improve the performance. For VOC, we report the results for both SVM and MLP-based linear classifiers. VoLTA with ResNet backbone achieves state-of-the-art results on VOC SVM evaluation, beating the nearest baseline, SwAV, by mAP score. These results indicate the ability of VoLTA to learn effective image-level visual features.
Method | #Pre-train Data | RefCOCO | RefCOCO+ | RefCOCOg | ||||||
I-T | I-T-B | val | testA | testB | val | testA | testB | val | test | |
MAttNet | 76.4 | 80.4 | 69.3 | 64.9 | 70.3 | 56.0 | 66.7 | 67.0 | ||
VLBERT | M | 71.6 | 77.7 | 61.0 | ||||||
ViLBERT | 3M | 72.3 | 78.5 | 62.6 | ||||||
Ernie-VL-L | M | 75.9 | 82.4 | 66.9 | ||||||
Rosita | M | 84.8 | 88.0 | 78.3 | 76.1 | 82.0 | 67.4 | 78.2 | 78.3 | |
UNITER-L | M | 81.4 | 87.0 | 74.2 | 75.9 | 81.5 | 66.7 | 74.9 | 75.8 | |
VILLA-L | M | 82.4 | 87.5 | 74.8 | 76.2 | 81.5 | 66.9 | 76.2 | 76.7 | |
Models pre-trained on Im-Txt-Box data | ||||||||||
MDETR-B | M | 87.5 | 90.4 | 82.7 | 81.1 | 85.5 | 73.0 | 83.4 | 83.3 | |
UniTAB | M | 86.3 | 88.8 | 80.6 | 78.7 | 83.2 | 69.5 | 80.0 | 80.0 | |
X-VLM | M | M | - | - | - | 80.2 | 86.4 | 71.0 | - | - |
OFA-L | M | M | 90.1 | 92.9 | 85.3 | 84.5 | 90.1 | 77.8 | 84.5 | 85.2 |
FIBER-B | M | M | 90.7 | 92.6 | 87.3 | 85.7 | 90.1 | 79.4 | 87.1 | 87.3 |
VoLTA-B | k | 86.1 | 88.6 | 81.8 | 77.0 | 82.7 | 67.8 | 78.3 | 78.3 |
Method | COCO Val 2017 | LVIS MiniVal | |||
---|---|---|---|---|---|
AP | APr | APc | APf | AP | |
Models pre-trained on Im-Txt-Box and/or with larger size | |||||
Mask R-CNN | 26.3 | 34.0 | 33.9 | 33.3 | |
MDETR | 20.9 | 24.9 | 24.3 | 24.2 | |
GLIP-B | 57.0 | 31.3 | 48.3 | 56.9 | 51.0 |
GLIP-L | 60.8 | ||||
FIBER-B | 58.4 | 50.0 | 56.9 | 58.1 | 56.9 |
VoLTA-B | 51.6 | 34.4 | 43.1 | 43.8 | 42.7 |
Object Detection & Instance Segmentation: Next, we perform two uni-modal region-level tasks - object detection on VOC and COCO, and instance segmentation on COCO. As shown in Table 2, VoLTA yields the state-of-the-art performance in both tasks across the majority of metrics. The fine-grained region-level understanding helps VoLTA to perform well on detection and segmentation tasks.
4.5 Results on Fine-grained Vision-Language tasks
Next, we perform region-level multi-modal downstream tasks - referring expression comprehension (REC) and language-guided object detection.
REC: This task aims to localize target objects in an image described by a referring expression phrased in natural language and, thus, perfectly evaluates the fine-grained feature representation capability of VoLTA. As depicted in Table 3, VoLTA beats larger-sized UNITER-L and VILLA-L models on the challenging testB split of both RefCOCO and RefCOCO. Moreover, VoLTA performs comparably with MDETR and UniTAB, even without being trained on grounding data. These results indicate our model’s efficacy in learning fine-grained local visual features.
Object Detection: We evaluate VoLTA on two challenging language-conditioned object detection benchmarks - COCO and LVIS. Note that, all existing baselines for this tasks are pre-trained on fine-grained image-text-box data, whereas VoLTA only utilizes image-caption pairs. Table 4 shows that VoLTA performs comparatively with these strong baselines. Note that VoLTA beats Mask R-CNN, MDETR, and GLIP-B on LVIS APr, which denotes average precision on rare objects. Thus, we conclude that VoLTA achieves impressive localization ability and robustness, even without utilizing any grounding annotations.
Method | #Pre-train Data | VQAv | NLVR2 | Fk IRTR | Method | #Pre-train Data | COCO Captioning | ||||||
dev | std | dev | test-P | IR@ | TR@ | B | M | C | S | ||||
Models pre-trained on COCO (k) and/or VG (k) | Models fine-tuned without CIDEr optimization | ||||||||||||
SCAN | k | 48.6 | 67.4 | VirTex | k | 95.5 | 18.1 | ||||||
SCG | k | 49.3 | 71.8 | VL-T5 | k | 34.5 | 28.7 | 116.5 | 21.9 | ||||
PFAN | k | 50.4 | 70.0 | VL-BART | k | 35.1 | 28.7 | 116.6 | 21.5 | ||||
MaxEnt | k | 54.1 | 54.8 | VoLTA-B | k | 38.2 | 30.7 | 126.6 | 22.5 | ||||
VisualBERT | k | 70.8 | 71.0 | 67.4 | 67.0 | VoLTA-GOLD-B | k | 38.9 | 30.5 | 128.5 | 23.4 | ||
LXMERT | k | 72.4 | 72.5 | 74.9 | 74.5 | Models fine-tuned with CIDEr optimization | |||||||
SOHO | k | 73.2 | 73.4 | 76.3 | 77.3 | 72.5 | 86.5 | VoLTA-B | k | 39.7 | 30.5 | 133.6 | 23.7 |
VoLTA-B | k | 74.6 | 74.6 | 76.7 | 78.1 | 72.7 | 83.6 | VoLTA-GOLD-B | k | 40.2 | 30.9 | 137.5 | 23.7 |
VoLTA | RefCOCO | RefCOCO | RefCOCOg | |||||||||
val | testA | testB | val | testA | testB | val | test | |||||
✓ | 81.7 | 84.1 | 77.8 | 71.2 | 76.6 | 62.2 | 71.7 | 71.7 | ||||
✓ | ✓ | 82.0 | 84.5 | 77.8 | 71.5 | 77.1 | 62.2 | 71.4 | 71.8 | |||
✓ | ✓ | ✓ | 82.7 | 85.2 | 78.1 | 72.0 | 77.7 | 62.5 | 72.8 | 72.7 | ||
✓ | ✓ | ✓ | ✓ | 83.9 | 86.6 | 80.5 | 73.9 | 79.5 | 64.1 | 74.6 | 74.3 | |
✓ | ✓ | ✓ | ✓ | 82.8 | 85.5 | 78.5 | 72.2 | 77.8 | 62.8 | 72.9 | 72.8 | |
✓ | ✓ | ✓ | ✓ | ✓ | 86.1 | 88.6 | 81.8 | 77.0 | 82.7 | 67.8 | 78.3 | 78.3 |
4.6 Results on Coarse-grained Vision-Language tasks
Next, we perform image-level multi-modal downstream tasks - visual question answering (VQA), visual reasoning, retrieval, and captioning.
VQA & Visual Reasoning: As reported in Table 5, VoLTA achieves the best performance on VQA and visual reasoning across the baselines pre-trained with a comparable amount of data. Moreover, on VQA, VoLTA beats LXMERT, which is trained with more data. These results demonstrate the efficacy of our method even when utilizing a mid-scale pre-training corpus.
Retrieval: Most existing VLP methods use a fusion encoder for image and text retrieval and feed every image-text pair into the model. Though such fine-tuning often results in higher performance, it introduces quadratic time cost and is not scalable. Following Dou et al. (2022a), we adopt a more efficient strategy. We drop the cross-attention parameters for this task and compute the dot product of image and text features extracted separately in the dual-encoder setting. As shown in Table 5, even with such an approach, VoLTA produces superior performance among the baselines trained with a similar amount of data, beating all three baselines by a significant margin.
VOC | COCO | ||||
MLP | MLP (PC/O) | ||||
✓ | 90.8 | 72.2/71.7 | |||
✓ | ✓ | 91.8 | 74.0/73.7 | ||
✓ | ✓ | 86.5 | 69.0/69.8 | ||
✓ | ✓ | ✓ | ✓ | 94.0 | 74.5/76.4 |
VOC | COCO | |
---|---|---|
MLP | MLP (PC/O) | |
50 | 93.4 | 74.1/76.0 |
100 | 94.0 | 74.5/76.4 |
200 | 93.2 | 73.1/75.5 |
500 | 93.1 | 72.8/75.3 |
Projector Config. | VOC | COCO |
---|---|---|
MLP | MLP (PC/O) | |
8192-8192-128 | 91.3 | 71.3/73.0 |
8192-8192-256 | 91.9 | 72.2/73.3 |
2048-2048-512 | 93.4 | 73.9/76.1 |
2048-2048-1024 | 94.0 | 74.5/76.4 |
Captioning: We perform captioning on the COCO dataset to evaluate if VoLTA can adopt a generation task. We integrate GOLD (Pang & He, 2021) into VoLTA during fine-tuning as it produces significant improvements. As shown in Table 5, our approach maintains superior captioning performance across all baselines pre-trained with comparable data. Using CIDEr optimization further improves performance.
It is worth mentioning that besides achieving a superior result than all baselines using a comparable amount of data on multi-modal coarse-grained tasks, VoLTA also outperforms multiple methods pre-trained using magnitude more data. These results, shown in Table F.1, indicate the effectiveness and generalizability of VoLTA across these tasks.
4.7 Ablation Study
We perform ablation studies on the pre-training objectives, GOT loss weight, and the dimension of projectors.
Pre-training Objectives: We ablate the effectiveness of different pre-training objectives and evaluate the pre-trained models on fine-grained downstream tasks. First, we pre-train VoLTA only with the multi-modal BT loss. In this setup, VoLTA only acts as a dual encoder; thus, the cross-attention parameters are not pre-trained. Next, we add MLM and ITM loss which helps the model to learn cross-modal information via attention fusion. Next, we add the GOT pre-training objective. Note that GOT adopts two types of OT distances WD for node matching and GWD for edge matching. As shown in table 6, applying WD and GWD together improves the performance of reference expression comprehension across RefCOCO, RefCOCO, and RefCOCOg datasets. Specifically on RefCOCOg, adding to yields a significant boost in the challenging test set. Since this dataset contains intricate images with multiple similar objects with different shapes and colors, GWD is crucial in distinguishing between them. However, we see that adding GWD without WD is not helpful. This is because though GWD can capture the edge similarity between graphs, it cannot directly address graph alignment since it does not consider node information. For example, the word pair (boy, girl) has a similar cosine similarity as the pair (football, basketball). Still, the semantic meanings of the two pairs are different and should not be matched. But GWD will treat these two pairs as the same since it only considers the cosine similarity between nodes. Hence, when applied together, WD and GWD objectives only result in an effective region-word alignment.
We also verify the effectiveness of the multi-modal BT objective by ablating the intra- and inter-modal terms. The first row of Table 7(a) is identical to the original image-only BT objective. Next, we introduce the text branch and add the same BT objective between the two views of the caption. Afterward, we add the inter-modal BT objectives. As shown in Table 7(a), each loss term improved the image classification performance, demonstrating the importance of intra- and inter-modal objectives. Overall, this set of experiments demonstrates that all objectives are necessary for our model to perform well on different fine-grained multi-modal tasks.
GOT Loss Weight: In our loss formulation, we introduce a GOT loss weight which regulates the alignment of local features through GOT loss. By conducting a grid search on uni-modal downstream classification tasks, we assessed the impact of as shown in Table 7(b) and experimentally found its best value to be 100 in our case. It is to be noted that a very high value of considerably degrades the performance of downstream tasks.
Ablation on Projector Dimension: The design of the projector head plays a pivotal role in the downstream performance of the model (Garrido et al., 2022). To investigate the impact of hidden and feature (projector output) dimensions, we have tested different configurations on uni-modal downstream classification tasks. It can be observed (see Table 7(c)) that an increase in the number of parameters in the projector head does not necessarily lead to an increase in performance. For example, a projector configuration of -- has roughly eight times more parameters than --. However, the latter performs better in downstream tasks (Table 7(c)), indicating that the output dimension of the projector plays a crucial role in the final performance of the model.
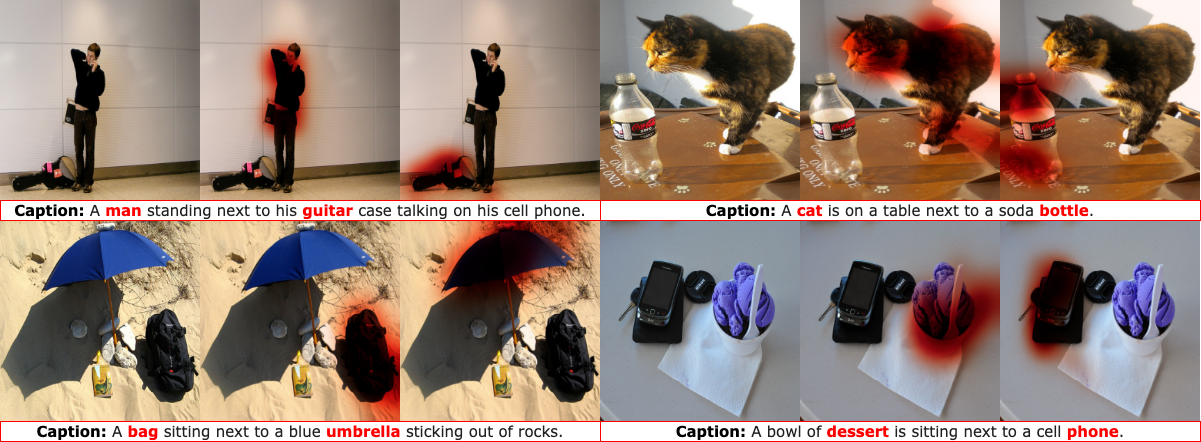
4.8 Qualitative Results & Error Analysis
Figure 4 shows the fine-grained alignment of image regions and caption words achieved by the pre-trained VoLTA system. The transport plan from GOT module outputs the similarity across every image patch and caption token. To obtain the visualizations in Figure 4, we choose the similarity scores between the red words in the caption with every image patch. Next, we apply bilinear interpolation to these similarity scores to convert them to the same dimension as the input image. Finally, we superimpose these interpolated similarity maps on the input images to obtain Figure 4 as the outcome. In most cases, the pre-trained model accurately learns to localize various objects using only global image-caption data. However, objects in extremely cluttered scenarios are occasionally not focused. We show such error cases in Section E.
5 Conclusion
We present VoLTA, a unified VLP paradigm that utilizes image-caption data but achieves fine-grained region-level image understanding, eliminating the use of expensive box annotations. VoLTA adopts graph optimal transport-based weakly supervised patch-token alignment and produces an explicit, self-normalized, and interpretable low-level matching criterion. Extensive experiments demonstrate the effectiveness of VoLTA on a wide range of coarse- and fine-grained tasks.
Acknowledgement
The codebase for this work is built on the Barlow Twins (Zbontar et al., 2021), GOT (Chen et al., 2020a), and FIBER (Dou et al., 2022a) repository. We would like to thank the respective authors for their contribution, and the Meta AI team for discussions and feedback. Shraman Pramanick and Rama Chellappa were partially supported by an ONR MURI Grant N00014-20-1-2787.
References
- Anderson et al. (2016) Peter Anderson, Basura Fernando, Mark Johnson, and Stephen Gould. Spice: Semantic propositional image caption evaluation. In European Conference on Computer Vision, pp. 382–398. Springer, 2016.
- Antol et al. (2015) Stanislaw Antol, Aishwarya Agrawal, Jiasen Lu, Margaret Mitchell, Dhruv Batra, C Lawrence Zitnick, and Devi Parikh. Vqa: Visual question answering. In International Conference on Computer Vision, pp. 2425–2433, 2015.
- Assran et al. (2022) Mahmoud Assran, Mathilde Caron, Ishan Misra, Piotr Bojanowski, Florian Bordes, Pascal Vincent, Armand Joulin, Michael G. Rabbat, and Nicolas Ballas. Masked siamese networks for label-efficient learning. arXiv, abs/2204.07141, 2022.
- Balaji et al. (2019) Yogesh Balaji, Rama Chellappa, and Soheil Feizi. Normalized wasserstein for mixture distributions with applications in adversarial learning and domain adaptation. In International Conference on Computer Vision, pp. 6500–6508, 2019.
- Banerjee & Lavie (2005) Satanjeev Banerjee and Alon Lavie. Meteor: An automatic metric for mt evaluation with improved correlation with human judgments. In Proceedings of the ACL Workshop on Intrinsic and Extrinsic Evaluation Measures for Machine Translation and/or Summarization, pp. 65–72, 2005.
- Bao et al. (2021) Hangbo Bao, Li Dong, Songhao Piao, and Furu Wei. Beit: Bert pre-training of image transformers. In International Conference on Learning Representations, 2021.
- Bardes et al. (2022) Adrien Bardes, Jean Ponce, and Yann LeCun. Vicreg: Variance-invariance-covariance regularization for self-supervised learning. In International Conference on Learning Representations, 2022.
- Brown et al. (2020) Tom Brown, Benjamin Mann, Nick Ryder, Melanie Subbiah, Jared D Kaplan, Prafulla Dhariwal, Arvind Neelakantan, Pranav Shyam, Girish Sastry, Amanda Askell, et al. Language models are few-shot learners. Advances in Neural Information Processing Systems, 33:1877–1901, 2020.
- Cai & Vasconcelos (2018) Zhaowei Cai and Nuno Vasconcelos. Cascade r-cnn: Delving into high quality object detection. In Proceedings of the IEEE/CVF Conference on Computer Vision and Pattern Recognition, pp. 6154–6162, 2018.
- Caron et al. (2021) Mathilde Caron, Hugo Touvron, Ishan Misra, Herv’e J’egou, Julien Mairal, Piotr Bojanowski, and Armand Joulin. Emerging properties in self-supervised vision transformers. International Conference on Computer Vision, pp. 9630–9640, 2021.
- Chen et al. (2021a) Chun-Fu (Richard) Chen, Quanfu Fan, and Rameswar Panda. CrossViT: Cross-Attention Multi-Scale Vision Transformer for Image Classification. In International Conference on Computer Vision, 2021a.
- Chen et al. (2019) Liqun Chen, Yizhe Zhang, Ruiyi Zhang, Chenyang Tao, Zhe Gan, Haichao Zhang, Bai Li, Dinghan Shen, Changyou Chen, and Lawrence Carin. Improving sequence-to-sequence learning via optimal transport. In International Conference on Learning Representations, 2019. URL https://openreview.net/forum?id=S1xtAjR5tX.
- Chen et al. (2020a) Liqun Chen, Zhe Gan, Yu Cheng, Linjie Li, Lawrence Carin, and Jingjing Liu. Graph optimal transport for cross-domain alignment. In International Conference on Machine Learning, pp. 1542–1553. PMLR, 2020a.
- Chen et al. (2020b) Ting Chen, Simon Kornblith, Mohammad Norouzi, and Geoffrey Hinton. A simple framework for contrastive learning of visual representations. In International Conference on Machine Learning, pp. 1597–1607. PMLR, 2020b.
- Chen & He (2021) Xinlei Chen and Kaiming He. Exploring simple siamese representation learning. Proceedings of the IEEE/CVF Conference on Computer Vision and Pattern Recognition, pp. 15745–15753, 2021.
- Chen et al. (2020c) Xinlei Chen, Haoqi Fan, Ross Girshick, and Kaiming He. Improved baselines with momentum contrastive learning. arXiv preprint arXiv:2003.04297, 2020c.
- Chen et al. (2021b) Xinlei Chen, Saining Xie, and Kaiming He. An empirical study of training self-supervised vision transformers. International Conference on Computer Vision, pp. 9620–9629, 2021b.
- Chen et al. (2020d) Yen-Chun Chen, Linjie Li, Licheng Yu, Ahmed El Kholy, Faisal Ahmed, Zhe Gan, Yu Cheng, and Jingjing Liu. Uniter: Universal image-text representation learning. In European Conference on Computer Vision, pp. 104–120. Springer, 2020d.
- Cho et al. (2021) Jaemin Cho, Jie Lei, Hao Tan, and Mohit Bansal. Unifying vision-and-language tasks via text generation. In International Conference on Machine Learning, pp. 1931–1942. PMLR, 2021.
- Dai et al. (2021) Xiyang Dai, Yinpeng Chen, Bin Xiao, Dongdong Chen, Mengchen Liu, Lu Yuan, and Lei Zhang. Dynamic head: Unifying object detection heads with attentions. In Proceedings of the IEEE/CVF Conference on Computer Vision and Pattern Recognition, pp. 7373–7382, 2021.
- Deng et al. (2009) Jia Deng, Wei Dong, Richard Socher, Li-Jia Li, Kai Li, and Li Fei-Fei. Imagenet: A large-scale hierarchical image database. In Proceedings of the IEEE Conference on Computer Vision and Pattern Recognition, pp. 248–255. Ieee, 2009.
- Devlin et al. (2019) Jacob Devlin, Ming-Wei Chang, Kenton Lee, and Kristina Toutanova. Bert: Pre-training of deep bidirectional transformers for language understanding. In Proceedings of the 2019 Conference of the North American Chapter of the Association for Computational Linguistics: Human Language Technologies, Volume 1 (Long and Short Papers), pp. 4171–4186, 2019.
- Dosovitskiy et al. (2021) Alexey Dosovitskiy, Lucas Beyer, Alexander Kolesnikov, Dirk Weissenborn, Xiaohua Zhai, Thomas Unterthiner, Mostafa Dehghani, Matthias Minderer, Georg Heigold, Sylvain Gelly, Jakob Uszkoreit, and Neil Houlsby. An image is worth 16x16 words: Transformers for image recognition at scale. In International Conference on Learning Representations, 2021. URL https://openreview.net/forum?id=YicbFdNTTy.
- Dou et al. (2022a) Zi-Yi Dou, Aishwarya Kamath, Zhe Gan, Pengchuan Zhang, Jianfeng Wang, Linjie Li, Zicheng Liu, Ce Liu, Yann LeCun, Nanyun Peng, et al. Coarse-to-fine vision-language pre-training with fusion in the backbone. Advances in Neural Information Processing Systems, 2022a.
- Dou et al. (2022b) Zi-Yi Dou, Yichong Xu, Zhe Gan, Jianfeng Wang, Shuohang Wang, Lijuan Wang, Chenguang Zhu, Pengchuan Zhang, Lu Yuan, Nanyun Peng, et al. An empirical study of training end-to-end vision-and-language transformers. In Proceedings of the IEEE/CVF Conference on Computer Vision and Pattern Recognition, pp. 18166–18176, 2022b.
- Everingham et al. (2010) Mark Everingham, Luc Van Gool, Christopher KI Williams, John Winn, and Andrew Zisserman. The pascal visual object classes (voc) challenge. International Journal of Computer Vision, 88(2):303–338, 2010.
- Gao et al. (2021) Tianyu Gao, Xingcheng Yao, and Danqi Chen. Simcse: Simple contrastive learning of sentence embeddings. In Proceedings of the 2021 Conference on Empirical Methods in Natural Language Processing, pp. 6894–6910, 2021.
- Garrido et al. (2022) Quentin Garrido, Yubei Chen, Adrien Bardes, Laurent Najman, and Yann Lecun. On the duality between contrastive and non-contrastive self-supervised learning. arXiv preprint arXiv:2206.02574, 2022.
- Genevay et al. (2018) Aude Genevay, Gabriel Peyré, and Marco Cuturi. Learning generative models with sinkhorn divergences. In International Conference on Artificial Intelligence and Statistics, pp. 1608–1617. PMLR, 2018.
- Geng et al. (2022) Xinyang Geng, Hao Liu, Lisa Lee, Dale Schuurams, Sergey Levine, and P. Abbeel. Multimodal masked autoencoders learn transferable representations. arXiv, abs/2205.14204, 2022.
- Goyal et al. (2017) Priya Goyal, Piotr Dollár, Ross Girshick, Pieter Noordhuis, Lukasz Wesolowski, Aapo Kyrola, Andrew Tulloch, Yangqing Jia, and Kaiming He. Accurate, large minibatch sgd: Training imagenet in 1 hour. arXiv preprint arXiv:1706.02677, 2017.
- Grill et al. (2020) Jean-Bastien Grill, Florian Strub, Florent Altché, Corentin Tallec, Pierre Richemond, Elena Buchatskaya, Carl Doersch, Bernardo Avila Pires, Zhaohan Guo, Mohammad Gheshlaghi Azar, et al. Bootstrap your own latent-a new approach to self-supervised learning. Advances in Neural Information Processing Systems, 33:21271–21284, 2020.
- Gupta et al. (2019) Agrim Gupta, Piotr Dollar, and Ross Girshick. Lvis: A dataset for large vocabulary instance segmentation. In Proceedings of the IEEE Conference on Computer Vision and Pattern Recognition, pp. 5356–5364, 2019.
- Han et al. (2015) Song Han, Jeff Pool, John Tran, and William Dally. Learning both weights and connections for efficient neural network. Advances in Neural Information Processing Systems, 28, 2015.
- Han et al. (2023) Xiao Han, Xiatian Zhu, Licheng Yu, Li Zhang, Yi-Zhe Song, and Tao Xiang. Fame-vil: Multi-tasking vision-language model for heterogeneous fashion tasks. In Proceedings of the IEEE/CVF Conference on Computer Vision and Pattern Recognition, pp. 2669–2680, 2023.
- He et al. (2016) Kaiming He, Xiangyu Zhang, Shaoqing Ren, and Jian Sun. Deep residual learning for image recognition. In Proceedings of the IEEE Conference on Computer Vision and Pattern Recognition, pp. 770–778, 2016.
- He et al. (2017) Kaiming He, Georgia Gkioxari, Piotr Dollár, and Ross Girshick. Mask r-cnn. In International Conference on Computer Vision, pp. 2961–2969, 2017.
- He et al. (2020) Kaiming He, Haoqi Fan, Yuxin Wu, Saining Xie, and Ross Girshick. Momentum contrast for unsupervised visual representation learning. In Proceedings of the IEEE/CVF Conference on Computer Vision and Pattern Recognition, pp. 9729–9738, 2020.
- He et al. (2022) Kaiming He, Xinlei Chen, Saining Xie, Yanghao Li, Piotr Dollár, and Ross Girshick. Masked autoencoders are scalable vision learners. In Proceedings of the IEEE/CVF Conference on Computer Vision and Pattern Recognition, pp. 16000–16009, 2022.
- Huang et al. (2020) Zhicheng Huang, Zhaoyang Zeng, Bei Liu, Dongmei Fu, and Jianlong Fu. Pixel-bert: Aligning image pixels with text by deep multi-modal transformers. arXiv preprint arXiv:2004.00849, 2020.
- Huang et al. (2021) Zhicheng Huang, Zhaoyang Zeng, Yupan Huang, Bei Liu, Dongmei Fu, and Jianlong Fu. Seeing out of the box: End-to-end pre-training for vision-language representation learning. In Proceedings of the IEEE/CVF Conference on Computer Vision and Pattern Recognition, pp. 12976–12985, 2021.
- Jang et al. (2023) Jiho Jang, Chaerin Kong, Donghyeon Jeon, Seonhoon Kim, and Nojun Kwak. Unifying vision-language representation space with single-tower transformer. In Proceedings of the AAAI Conference on Artificial Intelligence, 2023.
- Jia et al. (2021) Chao Jia, Yinfei Yang, Ye Xia, Yi-Ting Chen, Zarana Parekh, Hieu Pham, Quoc Le, Yun-Hsuan Sung, Zhen Li, and Tom Duerig. Scaling up visual and vision-language representation learning with noisy text supervision. In International Conference on Machine Learning, pp. 4904–4916. PMLR, 2021.
- Kamath et al. (2021) Aishwarya Kamath, Mannat Singh, Yann LeCun, Gabriel Synnaeve, Ishan Misra, and Nicolas Carion. Mdetr-modulated detection for end-to-end multi-modal understanding. In International Conference on Computer Vision, pp. 1780–1790, 2021.
- Kazemzadeh et al. (2014) Sahar Kazemzadeh, Vicente Ordonez, Mark Matten, and Tamara Berg. Referitgame: Referring to objects in photographs of natural scenes. In Proceedings of the 2014 Conference on Empirical Methods in Natural Language Processing, pp. 787–798, 2014.
- Kiela et al. (2019) Douwe Kiela, Suvrat Bhooshan, Hamed Firooz, and Davide Testuggine. Supervised multimodal bitransformers for classifying images and text. arXiv, abs/1909.02950, 2019.
- Kim et al. (2021) Wonjae Kim, Bokyung Son, and Ildoo Kim. Vilt: Vision-and-language transformer without convolution or region supervision. In International Conference on Machine Learning, pp. 5583–5594. PMLR, 2021.
- Krishna et al. (2017) Ranjay Krishna, Yuke Zhu, Oliver Groth, Justin Johnson, Kenji Hata, Joshua Kravitz, Stephanie Chen, Yannis Kalantidis, Li-Jia Li, David A Shamma, et al. Visual genome: Connecting language and vision using crowdsourced dense image annotations. In International Journal of Computer Vision, volume 123, pp. 32–73. Springer, 2017.
- Li et al. (2022a) Juncheng Li, Xin He, Longhui Wei, Long Qian, Linchao Zhu, Lingxi Xie, Yueting Zhuang, Qi Tian, and Siliang Tang. Fine-grained semantically aligned vision-language pre-training. In Advances in Neural Information Processing Systems, 2022a.
- Li et al. (2021a) Junnan Li, Ramprasaath Selvaraju, Akhilesh Gotmare, Shafiq Joty, Caiming Xiong, and Steven Chu Hong Hoi. Align before fuse: Vision and language representation learning with momentum distillation. Advances in Neural Information Processing Systems, 34:9694–9705, 2021a.
- Li et al. (2022b) Junnan Li, Dongxu Li, Caiming Xiong, and Steven Hoi. Blip: Bootstrapping language-image pre-training for unified vision-language understanding and generation. In International Conference on Machine Learning, 2022b.
- Li et al. (2023a) Junnan Li, Dongxu Li, Silvio Savarese, and Steven Hoi. Blip-2: Bootstrapping language-image pre-training with frozen image encoders and large language models. In International Conference on Machine Learning, 2023a.
- Li et al. (2020a) Liunian Harold Li, Mark Yatskar, Da Yin, Cho-Jui Hsieh, and Kai-Wei Chang. What does bert with vision look at? In Proceedings of the 58th Annual Meeting of the Association for Computational Linguistics, pp. 5265–5275, 2020a.
- Li et al. (2022c) Liunian Harold Li, Pengchuan Zhang, Haotian Zhang, Jianwei Yang, Chunyuan Li, Yiwu Zhong, Lijuan Wang, Lu Yuan, Lei Zhang, Jenq-Neng Hwang, et al. Grounded language-image pre-training. In Proceedings of the IEEE/CVF Conference on Computer Vision and Pattern Recognition, pp. 10965–10975, 2022c.
- Li et al. (2022d) Manling Li, Ruochen Xu, Shuohang Wang, Luowei Zhou, Xudong Lin, Chenguang Zhu, Michael Zeng, Heng Ji, and Shih-Fu Chang. Clip-event: Connecting text and images with event structures. In Proceedings of the IEEE/CVF Conference on Computer Vision and Pattern Recognition, pp. 16420–16429, 2022d.
- Li et al. (2020b) Xiujun Li, Xi Yin, Chunyuan Li, Pengchuan Zhang, Xiaowei Hu, Lei Zhang, Lijuan Wang, Houdong Hu, Li Dong, Furu Wei, et al. Oscar: Object-semantics aligned pre-training for vision-language tasks. In European Conference on Computer Vision, pp. 121–137. Springer, 2020b.
- Li et al. (2021b) Yangguang Li, Feng Liang, Lichen Zhao, Yufeng Cui, Wanli Ouyang, Jing Shao, Fengwei Yu, and Junjie Yan. Supervision exists everywhere: A data efficient contrastive language-image pre-training paradigm. In International Conference on Learning Representations, 2021b.
- Li et al. (2023b) Yanghao Li, Haoqi Fan, Ronghang Hu, Christoph Feichtenhofer, and Kaiming He. Scaling language-image pre-training via masking. In Proceedings of the IEEE/CVF Conference on Computer Vision and Pattern Recognition, pp. 23390–23400, 2023b.
- Lin et al. (2014) Tsung-Yi Lin, Michael Maire, Serge Belongie, James Hays, Pietro Perona, Deva Ramanan, Piotr Dollár, and C Lawrence Zitnick. Microsoft coco: Common objects in context. In European Conference on Computer Vision, pp. 740–755. Springer, 2014.
- Liu et al. (2019) Yinhan Liu, Myle Ott, Naman Goyal, Jingfei Du, Mandar Joshi, Danqi Chen, Omer Levy, Mike Lewis, Luke Zettlemoyer, and Veselin Stoyanov. Roberta: A robustly optimized bert pretraining approach. arXiv preprint arXiv:1907.11692, 2019.
- Liu et al. (2021) Ze Liu, Yutong Lin, Yue Cao, Han Hu, Yixuan Wei, Zheng Zhang, Stephen Lin, and Baining Guo. Swin transformer: Hierarchical vision transformer using shifted windows. In International Conference on Computer Vision, pp. 10012–10022, 2021.
- Loshchilov & Hutter (2016) Ilya Loshchilov and Frank Hutter. Sgdr: Stochastic gradient descent with warm restarts. arXiv preprint arXiv:1608.03983, 2016.
- Lu et al. (2019) Jiasen Lu, Dhruv Batra, Devi Parikh, and Stefan Lee. Vilbert: Pretraining task-agnostic visiolinguistic representations for vision-and-language tasks. Advances in Neural Information Processing Systems, 32, 2019.
- Ma et al. (2022) Zhiyuan Ma, Jianjun Li, Guohui Li, and Kaiyan Huang. Cmal: A novel cross-modal associative learning framework for vision-language pre-training. In Proceedings of the 30th ACM International Conference on Multimedia, pp. 4515–4524, 2022.
- Mroueh et al. (2018) Youssef Mroueh, Chun-Liang Li, Tom Sercu, Anant Raj, and Yu Cheng. Sobolev gan. In International Conference on Learning Representations, 2018.
- Mroueh et al. (2019) Youssef Mroueh, Tom Sercu, and Anant Raj. Sobolev descent. In International Conference on Artificial Intelligence and Statistics, pp. 2976–2985. PMLR, 2019.
- Mu et al. (2021) Norman Mu, Alexander Kirillov, David A. Wagner, and Saining Xie. Slip: Self-supervision meets language-image pre-training. arXiv, abs/2112.12750, 2021.
- Oord et al. (2018) Aaron van den Oord, Yazhe Li, and Oriol Vinyals. Representation learning with contrastive predictive coding. arXiv preprint arXiv:1807.03748, 2018.
- Pang & He (2021) Richard Yuanzhe Pang and He He. Text generation by learning from demonstrations. In International Conference on Learning Representations, 2021.
- Papineni et al. (2002) Kishore Papineni, Salim Roukos, Todd Ward, and Wei-Jing Zhu. Bleu: a method for automatic evaluation of machine translation. In Proceedings of the 40th Annual Meeting of the Association for Computational Linguistics, pp. 311–318, 2002.
- Park & Han (2023) Jaeyoo Park and Bohyung Han. Multi-modal representation learning with text-driven soft masks. In Proceedings of the IEEE/CVF Conference on Computer Vision and Pattern Recognition, pp. 2798–2807, 2023.
- Peyré et al. (2016) Gabriel Peyré, Marco Cuturi, and Justin Solomon. Gromov-wasserstein averaging of kernel and distance matrices. In International Conference on Machine Learning, pp. 2664–2672. PMLR, 2016.
- Peyré et al. (2019) Gabriel Peyré, Marco Cuturi, et al. Computational optimal transport: With applications to data science. Foundations and Trends® in Machine Learning, 11(5-6):355–607, 2019.
- Plummer et al. (2015) Bryan A Plummer, Liwei Wang, Chris M Cervantes, Juan C Caicedo, Julia Hockenmaier, and Svetlana Lazebnik. Flickr30k entities: Collecting region-to-phrase correspondences for richer image-to-sentence models. In International Conference on Computer Vision, pp. 2641–2649, 2015.
- Pramanick et al. (2022) Shraman Pramanick, Aniket Roy, and Vishal M Patel. Multimodal learning using optimal transport for sarcasm and humor detection. In Proceedings of the IEEE/CVF Winter Conference on Applications of Computer Vision, pp. 3930–3940, 2022.
- Pramanick et al. (2023) Shraman Pramanick, Yale Song, Sayan Nag, Kevin Qinghong Lin, Hardik Shah, Mike Zheng Shou, Rama Chellappa, and Pengchuan Zhang. Egovlpv2: Egocentric video-language pre-training with fusion in the backbone. arXiv preprint arXiv:2307.05463, 2023.
- Radford et al. (2019) Alec Radford, Jeff Wu, Rewon Child, David Luan, Dario Amodei, and Ilya Sutskever. Language models are unsupervised multitask learners. 2019.
- Radford et al. (2021) Alec Radford, Jong Wook Kim, Chris Hallacy, Aditya Ramesh, Gabriel Goh, Sandhini Agarwal, Girish Sastry, Amanda Askell, Pamela Mishkin, Jack Clark, et al. Learning transferable visual models from natural language supervision. In International Conference on Machine Learning, pp. 8748–8763. PMLR, 2021.
- Ren et al. (2015) Shaoqing Ren, Kaiming He, Ross Girshick, and Jian Sun. Faster r-cnn: Towards real-time object detection with region proposal networks. Advances in Neural Information Processing Systems, 28, 2015.
- Shah et al. (2022) Anshul Shah, Suvrit Sra, Rama Chellappa, and Anoop Cherian. Max-margin contrastive learning. In Proceedings of the AAAI Conference on Artificial Intelligence, volume 36, pp. 8220–8230, 2022.
- Singh et al. (2022) Amanpreet Singh, Ronghang Hu, Vedanuj Goswami, Guillaume Couairon, Wojciech Galuba, Marcus Rohrbach, and Douwe Kiela. Flava: A foundational language and vision alignment model. In Proceedings of the IEEE/CVF Conference on Computer Vision and Pattern Recognition, pp. 15638–15650, 2022.
- Su et al. (2019) Weijie Su, Xizhou Zhu, Yue Cao, Bin Li, Lewei Lu, Furu Wei, and Jifeng Dai. Vl-bert: Pre-training of generic visual-linguistic representations. In International Conference on Learning Representations, 2019.
- Suhr et al. (2019) Alane Suhr, Stephanie Zhou, Ally Zhang, Iris Zhang, Huajun Bai, and Yoav Artzi. A corpus for reasoning about natural language grounded in photographs. In Proceedings of the Annual Meeting of the Association for Computational Linguistics, 2019.
- Tan & Bansal (2019) Hao Tan and Mohit Bansal. Lxmert: Learning cross-modality encoder representations from transformers. In Proceedings of the 2019 Conference on Empirical Methods in Natural Language Processing and the 9th International Joint Conference on Natural Language Processing, pp. 5100–5111, 2019.
- Vedantam et al. (2015) Ramakrishna Vedantam, C Lawrence Zitnick, and Devi Parikh. Cider: Consensus-based image description evaluation. In Proceedings of the IEEE Conference on Computer Vision and Pattern Recognition, pp. 4566–4575, 2015.
- Wang et al. (2023a) Jianfeng Wang, Zhengyuan Yang, Xiaowei Hu, Linjie Li, Kevin Lin, Zhe Gan, Zicheng Liu, Ce Liu, and Lijuan Wang. Git: A generative image-to-text transformer for vision and language. Transactions of Machine Learning Research, 2023a.
- Wang et al. (2023b) Jinpeng Wang, Pan Zhou, Mike Zheng Shou, and Shuicheng Yan. Position-guided text prompt for vision-language pre-training. In Proceedings of the IEEE/CVF Conference on Computer Vision and Pattern Recognition, pp. 23242–23251, 2023b.
- Wang et al. (2022a) Junke Wang, Dongdong Chen, Zuxuan Wu, Chong Luo, Luowei Zhou, Yucheng Zhao, Yujia Xie, Ce Liu, Yu-Gang Jiang, and Lu Yuan. Omnivl: One foundation model for image-language and video-language tasks. In Advances in Neural Information Processing Systems, 2022a.
- Wang et al. (2022b) Peng Wang, An Yang, Rui Men, Junyang Lin, Shuai Bai, Zhikang Li, Jianxin Ma, Chang Zhou, Jingren Zhou, and Hongxia Yang. Ofa: Unifying architectures, tasks, and modalities through a simple sequence-to-sequence learning framework. In International Conference on Machine Learning, pp. 23318–23340. PMLR, 2022b.
- Wang et al. (2023c) Teng Wang, Yixiao Ge, Feng Zheng, Ran Cheng, Ying Shan, Xiaohu Qie, and Ping Luo. Accelerating vision-language pretraining with free language modeling. In Proceedings of the IEEE/CVF Conference on Computer Vision and Pattern Recognition, pp. 23161–23170, 2023c.
- Wang et al. (2021a) Wenhui Wang, Hangbo Bao, Li Dong, and Furu Wei. Vlmo: Unified vision-language pre-training with mixture-of-modality-experts. arXiv preprint arXiv:2111.02358, 2021a.
- Wang et al. (2022c) Wenhui Wang, Hangbo Bao, Li Dong, Johan Bjorck, Zhiliang Peng, Qiang Liu, Kriti Aggarwal, Owais Mohammed, Saksham Singhal, Subhojit Som, and Furu Wei. Image as a foreign language: Beit pretraining for all vision and vision-language tasks. arXiv, abs/2208.10442, 2022c.
- Wang et al. (2023d) Wenhui Wang, Hangbo Bao, Li Dong, Johan Bjorck, Zhiliang Peng, Qiang Liu, Kriti Aggarwal, Owais Khan Mohammed, Saksham Singhal, Subhojit Som, et al. Image as a foreign language: Beit pretraining for vision and vision-language tasks. In Proceedings of the IEEE/CVF Conference on Computer Vision and Pattern Recognition, pp. 19175–19186, 2023d.
- Wang et al. (2021b) Zirui Wang, Jiahui Yu, Adams Wei Yu, Zihang Dai, Yulia Tsvetkov, and Yuan Cao. Simvlm: Simple visual language model pretraining with weak supervision. In International Conference on Learning Representations, 2021b.
- Wei & Zou (2019) Jason Wei and Kai Zou. Eda: Easy data augmentation techniques for boosting performance on text classification tasks. In Proceedings of the 2019 Conference on Empirical Methods in Natural Language Processing and the 9th International Joint Conference on Natural Language Processing, pp. 6382–6388, 2019.
- Wu et al. (2019) Yuxin Wu, Alexander Kirillov, Francisco Massa, Wan-Yen Lo, and Ross Girshick. Detectron2. https://github.com/facebookresearch/detectron2, 2019.
- Xue et al. (2021) Hongwei Xue, Yupan Huang, Bei Liu, Houwen Peng, Jianlong Fu, Houqiang Li, and Jiebo Luo. Probing inter-modality: Visual parsing with self-attention for vision-and-language pre-training. Advances in Neural Information Processing Systems, 34:4514–4528, 2021.
- Yang et al. (2022a) Jianwei Yang, Chunyuan Li, Pengchuan Zhang, Bin Xiao, Ce Liu, Lu Yuan, and Jianfeng Gao. Unified contrastive learning in image-text-label space. In Proceedings of the IEEE/CVF Conference on Computer Vision and Pattern Recognition, pp. 19163–19173, 2022a.
- Yang et al. (2022b) Jinyu Yang, Jiali Duan, Son Tran, Yi Xu, Sampath Chanda, Liqun Chen, Belinda Zeng, Trishul Chilimbi, and Junzhou Huang. Vision-language pre-training with triple contrastive learning. In Proceedings of the IEEE/CVF Conference on Computer Vision and Pattern Recognition, pp. 15671–15680, 2022b.
- Yang et al. (2022c) Zhengyuan Yang, Zhe Gan, Jianfeng Wang, Xiaowei Hu, Faisal Ahmed, Zicheng Liu, Yumao Lu, and Lijuan Wang. Unitab: Unifying text and box outputs for grounded vision-language modeling. In European Conference on Computer Vision,, 2022c.
- Yao et al. (2022) Lewei Yao, Runhui Huang, Lu Hou, Guansong Lu, Minzhe Niu, Hang Xu, Xiaodan Liang, Zhenguo Li, Xin Jiang, and Chunjing Xu. Filip: Fine-grained interactive language-image pre-training. In International Conference on Learning Representations, 2022.
- You et al. (2022) Haoxuan You, Luowei Zhou, Bin Xiao, Noel Codella, Yu Cheng, Ruochen Xu, Shih-Fu Chang, and Lu Yuan. Learning visual representation from modality-shared contrastive language-image pre-training. In European Conference on Computer Vision, 2022.
- You et al. (2017) Yang You, Igor Gitman, and Boris Ginsburg. Large batch training of convolutional networks. arXiv preprint arXiv:1708.03888, 2017.
- Yu et al. (2016) Licheng Yu, Patrick Poirson, Shan Yang, Alexander C Berg, and Tamara L Berg. Modeling context in referring expressions. In European Conference on Computer Vision, pp. 69–85. Springer, 2016.
- Yuan et al. (2020) Siyang Yuan, Ke Bai, Liqun Chen, Yizhe Zhang, Chenyang Tao, Chunyuan Li, Guoyin Wang, Ricardo Henao, and Lawrence Carin. Advancing weakly supervised cross-domain alignment with optimal transport. In British Machine Vision Conference, 2020.
- Yuan et al. (2021) Xin Yuan, Zhe Lin, Jason Kuen, Jianming Zhang, Yilin Wang, Michael Maire, Ajinkya Kale, and Baldo Faieta. Multimodal contrastive training for visual representation learning. In Proceedings of the IEEE/CVF Conference on Computer Vision and Pattern Recognition, pp. 6995–7004, 2021.
- Zbontar et al. (2021) Jure Zbontar, Li Jing, Ishan Misra, Yann LeCun, and Stéphane Deny. Barlow twins: Self-supervised learning via redundancy reduction. In International Conference on Machine Learning, pp. 12310–12320. PMLR, 2021.
- Zeng et al. (2022) Yan Zeng, Xinsong Zhang, and Hang Li. Multi-grained vision language pre-training: Aligning texts with visual concepts. In International Conference on Machine Learning, 2022.
- Zhang et al. (2020) Chi Zhang, Yujun Cai, Guosheng Lin, and Chunhua Shen. Deepemd: Few-shot image classification with differentiable earth mover’s distance and structured classifiers. In Proceedings of the IEEE/CVF Conference on Computer Vision and Pattern Recognition, pp. 12203–12213, 2020.
- Zhang et al. (2022) Haotian Zhang, Pengchuan Zhang, Xiaowei Hu, Yen-Chun Chen, Liunian Harold Li, Xiyang Dai, Lijuan Wang, Lu Yuan, Jenq-Neng Hwang, and Jianfeng Gao. Glipv2: Unifying localization and vision-language understanding. Advances in Neural Information Processing Systems, 2022.
- Zhang et al. (2021) Pengchuan Zhang, Xiujun Li, Xiaowei Hu, Jianwei Yang, Lei Zhang, Lijuan Wang, Yejin Choi, and Jianfeng Gao. Vinvl: Revisiting visual representations in vision-language models. In Proceedings of the IEEE/CVF Conference on Computer Vision and Pattern Recognition, pp. 5579–5588, 2021.
- Zhou et al. (2020) Luowei Zhou, Hamid Palangi, Lei Zhang, Houdong Hu, Jason Corso, and Jianfeng Gao. Unified vision-language pre-training for image captioning and vqa. In Proceedings of the AAAI Conference on Artificial Intelligence, volume 34, pp. 13041–13049, 2020.
Appendix A Pesudo Code of VoLTA
The training pseudo code for VoLTA is as follows:
Appendix B Overview of Vision-Language Pre-training Models
Model | Venue | Vision Encoder | Text Enc. | Multimodality Fusion | Pre-train | Pre-training Objectives | |
I-T | I-T-B | ||||||
ViLBERT | NeurIPS’ | OD+Xformer | Xformer | Co-attn | ✓ | MLM+ITM+MIM | |
LXMERT | EMNLP’ | ✓ | MLM+ITM+MIM+VQA | ||||
\cdashline3-5 VisualBERT | ACL’ | OD | Emb. | Merged attn | ✓ | MLM+ITM | |
VL-BERT | ICLR’ | ✓ | MLM+MIM | ||||
UNITER | ECCV’ | ✓ | MLM+ITM+MIM+WRA | ||||
OSCAR | ECCV’ | ✓ | MLM+ITM | ||||
VinVL | CVPR’ | ✓ | MLM+ITM | ||||
VL-T5 | ICML’ | ✓ | MLM+ITM+VQA+Grnd+Cap | ||||
SOHO | CVPR’ | CNN | Emb. | Merged attn | ✓ | MLM+ITM+MIM | |
SimVLM | ICLR’ | ✓ | PrefixLM | ||||
\cdashline4-4 MDETR | ICCV’ | Xformer | ✓ | OD+TP+CA | |||
ViLT | ICML’ | Patch Emb. | Emb. | Merged attn | ✓ | MLM+ITM | |
\cdashline3-3 Visual Parsing | NeurIPS’ | Xformer | ✓ | MLM+ITM+MIM | |||
\cdashline4-5 ALBEF | NeurIPS’ | Xformer | Co-attn | ✓ | MLM+ITM+ITC | ||
METER | CVPR’ | ✓ | MLM+ITM | ||||
CLIP | ICML’ | CNN/Xformer | Xformer | None | ✓ | ITC | |
DeCLIP | ICLR’ | ✓ | ITC+MLM+SL+MVS+NNS | ||||
\cdashline3-3 ALIGN | ICML’ | CNN | ✓ | ITC | |||
\cdashline3-3 \cdashline5-5 GLIP | CVPR’ | OD+Xformer | Cross-modality MHA | ✓ | ✓ | OD+CE+WRA | |
GLIPv2 | NeurIPS’ | ✓ | ✓ | OD+CE+WRA+MLM | |||
\cdashline3-3 BLIP | ICML’ | Xformer | ✓ | ITC+ITM+LM | |||
OmniVL | NeurIPS’ | ✓ | UniVLC+VLM+LM | ||||
X-VLM | ICML’ | ✓ | ✓ | BBP+ITC+MP+MLM | |||
\cdashline5-5 CMAL | ACM MM’ | None | ✓ | AMC+MLM+MRM+ITM+ITC | |||
LOUPE | NeurIPS’ | ✓ | ITC+FSA+TSA | ||||
FILIP | ICLR’ | ✓ | ITC | ||||
\cdashline3-3 UniCL | CVPR’ | CNN/Xformer | ✓ | ITC | |||
\cdashline5-5 UniTAB | ECCV’ | CNN | Merged attn | ✓ | LM | ||
\cdashline3-3 TCL | CVPR’ | Xformer | ✓ | CMA+IMC+LMI+ITM+MLM | |||
\cdashline5-5 MS-CLIP | ECCV’ | Shared Attention | ✓ | ITC | |||
\cdashline5-5 FLM | CVPR’ | Cross-modality MHA | ✓ | FLM + ITM | |||
BLIP-2 | ICML’ | ✓ | ITC+ITM+ITG | ||||
\cdashline5-5 Fame-ViL | CVPR’ | Cross-modality Adaptive Attention | ✓ | ITC | |||
\cdashline5-5 \cdashline3-3 PTP | CVPR’ | OD+Xformer | Cross-modality MHA | ✓ | ITC+ITM+LM | ||
\cdashline5-5 \cdashline3-3 Softmask++ | CVPR’ | Xformer | Merged attn | ✓ | ITC+ITM+MLM | ||
\cdashline5-5 OneR | AAAI’ | Unified attn | ✓ | ITC+XMC+CIC+CMC | |||
\cdashline5-5 BEiT-3 | CVPR’ | Shared MHA | ✓ | MDM | |||
\cdashline5-5 FLIP | CVPR’ | None | ✓ | ITC | |||
\cdashline5-5 \cdashline4-4 GIT | TMLR’ | Emb. | Merged attn | ✓ | LM | ||
FIBER | NeurIPS’ | Xformer | Xformer | Merged Co-attn | ✓ | ✓ | MLM+ITM+ITC |
VoLTA | TMLR’ | CNN/Xformer | Xformer | Merged Co-attn | ✓ | BT+GOT+MLM+ITM |
Vision-Language Pre-trained (VLP) models have proven extremely beneficial for multi-modal tasks in recent years. Earlier works were predominantly focused on using pre-trained object detectors to extract patch (region) level information from corresponding images (Lu et al., 2019; Li et al., 2020a; Tan & Bansal, 2019; Chen et al., 2020d; Su et al., 2019). In some of these models, such as ViLBERT (Lu et al., 2019), and LXMERT (Tan & Bansal, 2019), multi-modality fusion has been achieved via co-attention using a third transformer which contains fused information independently obtained from respective vision and language encoders. On the contrary, VisualBERT (Li et al., 2020a), VL-BERT (Su et al., 2019), and UNITER (Chen et al., 2020d) employ a merged attention strategy to fuse both image patches and text features together into a unified transformer through corresponding image and text embedders. In addition to these, OSCAR (Li et al., 2020b) uses object tags as inputs. VinVL (Zhang et al., 2021) follows a similar strategy to that of OSCAR, the only difference being their novel 3-way contrastive loss which optimizes the training objectives used for VQA and text-image matching. VL-T5 (Cho et al., 2021) exploits bounding-box coordinate information, image IDs, and region IDs along with ROI features for visual embedding. Here, encoded visual and textual features are fed into a bi-directional multi-modal encoder and an auto-regressive text decoder framework, respectively, for pre-training.
In all the above methods, pre-trained object detectors are kept frozen during the training. Furthermore, extracting region-level features from images can be tedious. To address these shortcomings, end-to-end pre-training methods have been developed. PixelBERT (Huang et al., 2020) uses a CNN-based vision encoder and sentence encoder to obtain image and text representations, respectively. These representations are subsequently fed into a transformer via a cross-modality alignment. SOHO (Huang et al., 2021) uses grid features-based discretization via a learned vision dictionary which is then fed into a cross-modal module. SimVLM (Wang et al., 2021b) uses CNN and text token embedding for image and text feature representation extraction with a unified encoder-decoder transformer trained on a PrefixLM objective. Finally, MDETR (Kamath et al., 2021) uses CNN and RoBERTa (along with corresponding projection layers) for image and text feature extraction. These extracted features are concatenated before passing through a unified transformer trained on 1.3M Image-Text-Box (I-T-B) annotated data.
In recent years, the rise of Vision Transformers (ViT) (Dosovitskiy et al., 2021) has motivated the research community to have an all-transformer framework by incorporating ViTs (instead of CNN backbones) in VLP models. Image patch features and text token embeddings are fed directly into a ViT model for pre-training in ViLT (Kim et al., 2021). Visual Parsing (Xue et al., 2021), ALBEF (Li et al., 2021a), and METER (Dou et al., 2022b) use ViTs as vision encoders for image feature generation. ALBEF and METER use co-attention in their pre-training frameworks for multimodality fusion.
Another class of VLP models in the form of CLIP (Radford et al., 2021), DeCLIP (Li et al., 2021b), and ALIGN (Jia et al., 2021) has been introduced lately. Although known for their impressive zero-shot recognition ability and excellent transferability to downstream tasks, these models typically rely on huge amounts of image-text pairs for pre-training. Contrastive loss forms the core component of the pre-training objectives in these VLP models. In such models (e.g., CLIP (Radford et al., 2021), DeCLIP (Li et al., 2021b)), separate encoders have been used for each modality. On the contrary, modality-shared contrastive language-image pre-training (MS-CLIP) (You et al., 2022) leverages knowledge distribution across multiple modalities (image and text) through parameter sharing. In their unified framework, the parameters which are being shared between two modalities include the attention and feedforward modules and the layerNorm layers.
GLIP (Li et al., 2022c) and GLIPv2 (Zhang et al., 2022) use a localization loss along with a word-region alignment loss for pre-training corresponding encoders using image-text-box annotations. BLIP (Li et al., 2022b) employs image and text encoders connected through a cross-modality multi-head attention which are pre-trained on image-text pairs using contrastive and language modeling objectives. OmniVL (Wang et al., 2022a) utilizes a unified image (and video) encoder and a text encoder pre-trained on image-text, image-label, video-text, and video-label pairs using unified vision-language contrastive, vision-language matching and language modeling losses. Furthermore, a visual-grounded alignment decoder is also present for facilitating better learning and alignment between various modalities. X-VLM (Zeng et al., 2022) employs a vision transformer to extract features from the subset of patches representing images/regions/objects. These patch features are then paired with associated text features for contrastive learning, matching, and masked language modeling. Additionally, image and text pairings are also done for bounding-box prediction which is used to locate visual concepts in the image. CMAL (Ma et al., 2022) proposes interactions between features (obtained from respective image and text encoders) via cross-modal associative mappings which help in fine-grained semantic alignment between the learned representations. LOUPE (Li et al., 2022a) implements token-level and semantics-level Shapley interaction modeling with global image-text contrastive loss (in a dual-encoder setting) for explicit learning of fine-grained semantic alignment between visual regions and textual phrases without using expensive bounding-box annotations. FILIP (Yao et al., 2022) removes the need for cross-modality attention fusion by modeling the fine-grained semantic alignment between visual and textual tokens via a novel cross-modal late interaction mechanism in contrastive loss. TCL (Yang et al., 2022b) uses global cross-modal alignment, intra-modal alignment, and local mutual information maximization losses along with masked language modeling and image-text matching to learn robust image-text representations during pre-training. UniCL (Yang et al., 2022a) utilizes a unified learning method with a two-way contrastive loss (image-to-text and text-to-image) in the image-text-label space which can learn representations from either of the image-label and image-text data or both. UniTAB (Yang et al., 2022c) employs a transformer-based encoder-decoder framework that can jointly output open-ended text and box, encouraging alignment between words and boxes.
In order to accelerate the convergence of VL pretraining, Wang et al. (2023c) proposed free language modeling (FLM) which addresses the issues inherent to masked language modeling (MLM) and autoregressive modeling. Using FLM as a pre-training objective, the authors have achieved impressive performance on several downstream tasks. Fame-ViL (Han et al., 2023) introduces a parameter-efficient VLP approach employing a task-versatile architecture with cross-attention and task-specific adapters. Fame-ViL applies a single model for various heterogeneous fashion tasks achieving performance gains over previous SOTA benchmarks. Wang et al. (2023b) have introduced a simple yet effective position-guided text prompt (PTP) paradigm to improve the visual grounding capability of existing cross-modal VL architectures and help them better handle various downstream tasks. Park & Han (2023) have proposed a VL framework based on the explainable soft feature masking and regularization via diversification strategies for improving the performance of VL models in several downstream tasks. Li et al. (2023a) have devised BLIP-2, where a lightweight querying transformer is pre-trained using a two-stage strategy to bridge the modality gap. A frozen encoder is used in the first stage to bootstrap VL representation learning, and vision-to-language generative learning is bootstrapped in the second stage employing a frozen LLM, allowing zero-shot generation capabilities. Jang et al. (2023) have developed a simple and unified VL model (as a single tower) in a modality-agnostic manner. BEiT-3 (Wang et al., 2023d) introduces a general-purpose multimodal foundation model to pre-train a multiway transformer by performing masked data modeling on inputs irrespective of modalities (i.e., images, texts, and image-text pairs). FLIP (Li et al., 2023b) extends CLIP (Radford et al., 2021) by performing contrastive learning on pairs of masked image patches and corresponding texts without reconstructing the masked image content. GIT (Wang et al., 2023a) unifies the VL architecture (an image encoder and a text decoder) under a single language modeling task while also scaling up the pre-training data and the model size to gain superior performance on captioning and question-answering downstream tasks.
FIBER (Dou et al., 2022a) fuses vision and language encoder backbones through merged co-attention which are then pre-trained on 4M data with two-stage pre-training (coarse- and fine-grained). Image-text pairs are used in the coarse-grained pre-training stage which is then followed by a fine-grained pre-training stage with image-text-box annotations. However, these bounding box annotations come with extra overheads. Therefore, in our model, VoLTA, we propose an alternate solution for optimal-transport based local feature-level alignment using global image-caption annotations which performs well not only on coarse-grained tasks (such as VQA and Image Captioning), but also on fine-grained tasks (such as Referring Expression Comprehension and Object Detection). Table B.1 encapsulates an overview of the details of all these aforementioned methods.
Appendix C Downstream Datasets
Modality | Task | Dataset | Image Src | #Images | #Text | Metric |
---|---|---|---|---|---|---|
Uni-modal | Image Classfn. | IN1K | IN1K | 1.3M | - | Accuracy |
COCO | COCO | 123K | - | F | ||
VOC0712 | VOC0712 | 16K | - | mAP | ||
\cdashline2-7 | Object Det. | COCO | COCO | 123K | - | APbb |
VOC0712 | VOC0712 | 16K | - | |||
\cdashline2-7 | Instance Seg. | COCO | COCO | 123K | - | APmk |
Multi-modal coarse-grained | VQA | VQA | COCO | 204K | 1.1M | VQA-Score |
NLVR2 | NLVR2 | Web Crawled | 214K | 107K | Accuracy | |
IR-TR | Flickr30K | Flickr30K | 32K | 160K | Recall@1 | |
Captioning | COCO | COCO | 123K | 615K | B,M,C,S | |
\cdashline1-7 Multi-modal fine-grained | Ref. Exp. Comp. | RefCOCO | COCO | 20K | 142K | Accuracy |
RefCOCO | 20K | 142K | ||||
RefCOCOg | 26K | 95K | ||||
\cdashline2-7 | Mul. Obj. Det. | COCO | COCO | 123K | 615K | AP |
LVIS Mini | COCO | 123K | 615K |
Our downstream tasks can be categorized into three groups: uni-modal, multi-modal coarse-grained, and multi-modal fine-grained.
Uni-modal: For uni-modal tasks, we fine-tune (and validate) our pre-trained model on ImageNet-1k (Deng et al., 2009) for image classification, VOC07+12 (Everingham et al., 2010) for image classification and object detection, and COCO (Lin et al., 2014) for image classification, object detection, and instance segmentation.
Multi-modal Coarse-grained: Here, we fine-tune (and validate) our pre-trained model on VQAv2 (Antol et al., 2015) for visual question answering, NLVR2 (Suhr et al., 2019) for visual reasoning, Flickr30k (Plummer et al., 2015) for image and text retrieval, and COCO (Lin et al., 2014) for image captioning.
Multi-modal Fine-grained: For these tasks, we fine-tune (and validate) our pre-trained model on RefCOCO, RefCOCO+, and RefCOCOg (Kazemzadeh et al., 2014; Yu et al., 2016) for referring expression comprehension, and COCO (Lin et al., 2014) and LVIS Mini (Gupta et al., 2019) for language-conditioned object detection.
Several multi-modal downstream tasks are built based on the COCO dataset, where the validation and test splits of these downstream tasks are scattered across the raw COCO splits. Therefore, during pre-training, we carefully selected the portion of the COCO dataset which does not overlap with the validation/test splits of these multi-modal downstream tasks.
Appendix D Implementation Details Hyper-parameter Values
D.1 Data Augmentation
We use ResNet/Swin-T/Swin-B (He et al., 2016; Liu et al., 2021) as image encoder and RoBERTa (Liu et al., 2019) as text encoder. Each encoder is followed by a projector network which is a -layer MLP with the configuration [---]. Here, represents the embedding dimension of the encoder’s output.
Data Type | View # | Augmentation | Probability |
---|---|---|---|
Image | 1 | RandomResizedCrop | 1.0 |
1 | RandomHorizontalFlip | 0.5 | |
1 | ColorJitter | 0.8 | |
1 | RandomGrayscale | 0.2 | |
1 | GaussianBlur | 1.0 | |
1 | Solarization | 0.0 | |
\cdashline2-4 | 2 | RandomResizedCrop | 1.0 |
2 | RandomHorizontalFlip | 0.5 | |
2 | ColorJitter | 0.8 | |
2 | RandomGrayscale | 0.2 | |
2 | GaussianBlur | 0.1 | |
2 | Solarization | 0.2 | |
Text | 1 | Synonym Replacement | 0.1 |
1 | Random Insertion | 0.1 | |
1 | Random Swap | 0.1 | |
1 | Random Deletion | 0.1 | |
\cdashline2-4 | 2 | Synonym Replacement | 0.1 |
2 | Random Insertion | 0.2 | |
2 | Random Swap | 0.1 | |
2 | Random Deletion | 0.2 |
Image Augmentations: Two sets of random transformations sampled from an augmentation pool are applied on each input image to generate two disparate distorted views. The augmentation policy is composed of RandomResizedCrop, RandomHorizontalFlip, ColorJitter, RandomGrayscale, GaussianBlur, and Solarization augmentations. RandomResizedCrop is applied with a probability of 1.0, whereas the remaining ones are applied randomly with varying probabilities following Zbontar et al. (2021) (see Table D.1).
D.2 Pre-training Setup
Table D.2 shows the details of hyper-parameters used during training.
VoLTA comprises a vision encoder and a language encoder with a merged co-attention for cross-modality fusion. In our experiments, we have considered two types of vision encoder backbones - ResNet- (He et al., 2016) and Swin Transformer (Liu et al., 2021). For fair comparisons with related works (Dou et al., 2022b; a), the input image resolution for ResNet-50 encoder backbone is kept as 224 224, whereas for Swin-B, it is 384 384. The output embedding dimension of the image encoder in both cases is 1024. Similarly, to be consistent with Dou et al. (2022b; a), we have selected RoBERTa as the language encoder with a vocabulary size of 50265, a tokenizer as ‘roberta-base’, a maximum input text length of 30, and an output embedding dimension of 768 (please refer to Table D.2 for more details).
Separate projector heads follow vision and language encoders. A projector head consists of 3 linear layers, each with 2048 output units (except for the last one, which has 1024 output units), followed by a Batch Normalization layer and ReLU activation (for exact configuration, please refer to Table D.2). The final projected output denotes the input (image/text) feature representation used in downstream tasks. The embeddings (i.e., output from respective encoders) are fed into the loss function of VoLTA to learn these representations.
The loss function of VoLTA includes four different loss components, namely, multi-modal Barlow Twins for intra- and inter-modality redundancy reduction, GOT for alignment of local features, and MLM and ITM together for encouraging cross-modal attention fusion. For MLM, we randomly mask 222Following BERT, we decompose this into random words, unchanged, and with a special token [MASK]. (MLM probability in Table D.2) of the input tokens, and the model is trained to reconstruct the original tokens. For ITM, the model predicts whether a given image-text pair is matched.
For optimization, we follow the same protocol as described in Zbontar et al. (2021), where we use the LARS (You et al., 2017) optimizer to train our model for 20 epochs with a batch size of 256. A base LR of 0.1 is used for the weights and 0.0048 for the biases and batch normalization parameters which are then multiplied by a factor of 2. We employ a learning rate warm-up (linear) up to a period of 2 epochs followed by a cosine decay schedule to reduce the LR by a factor of 1000. A weight decay parameter 1e-6 is used, excluding the biases and batch normalization parameters. We conduct a grid search for the GOT loss hyperparameter (), and we empirically found the best value to be 100.
Hyper-parameters | Notation | Value |
---|---|---|
Model | ||
Img. proj. layer config. | - | |
Img. embed. dim | 1024 | |
Img. reso. (RN-50 & Swin-T) | - | 224 224 |
Img. reso. (Swin-B) | - | 384 384 |
Txt. proj. layer config. | - | |
Txt. embed. dim | 768 | |
Tokenizer | - | ’roberta-base’ |
Vocab size | - | 50265 |
MLM prob. | - | 0.15 |
Max. length of text | - | 30 |
# Heads of Xformer | - | 12 |
# Layers of Xformer | - | 12 |
# Fusion block | - | 6 |
Dropout rate | - | 0.1 |
Task names | - | ’BTGOT, MLM, ITM’ |
Training | ||
Batch size | - | 256 |
Epochs | - | 20 |
Lambda_BT | 0.005 | |
WD and GWD loss weight | 0.1 | |
GOT loss weight | 100.0 | |
Optimizer | - | LARS |
Base LR for weights | - | 0.1 |
Base LR for biases | - | 0.0048 |
Momentum | - | 0.9 |
LR scheduler | - | Cosine LR decay (with linear warm-up) |
Warm-up steps | - | 2 Epochs |
Weight decay | - | 1e-6 |
End LR factor | - | 0.001 |
Cosine LR amplitude factor | - | 0.5 |
Pre-training Cost: Our Swin-B backbone takes 6 hours per epoch to train on 64 V GPUs, with per GPU batch-size of .
D.3 Downstream Setup
D.3.1 Uni-modal downstream tasks
Linear Evaluation:
For ImageNet, the linear classifier has been trained for 100 epochs with a batch size of 256, an LR of 0.3, and a cosine LR schedule. Cross-entropy loss is minimized with SGDM optimizer (momentum of 0.9), and a weight decay of 1e-6. For both COCO and VOC, the linear classifier has been trained for 100 epochs with AdamW optimizer with batch size of 256, an LR of 5e-2, and a weight decay of 1e-6.
Object Detection: For training the detection model, the detectron2 library (Wu et al., 2019) has been used. The backbone networks for Faster R-CNN (Ren et al., 2015) and Mask R-CNN (He et al., 2017) has been initialized using our pre-trained model.
For VOC07+12, we used the trainval set comprising 16K images for training a Faster R-CNN (Ren et al., 2015) C-4 backbone for 24K iterations using a batch size of 16 across 8 GPUs (using SyncBatchNorm). The initial learning rate for the model is 0.15, which is reduced by a factor of 10 after 18K and 22K iterations. Linear warmup (Goyal et al., 2017) is used with a slope of 0.333 for 1000 iterations.
D.3.2 Coarse-grained multi-modal downstream tasks
Vision-Language Classification (VQAv2 and NLVR2): Vision-Language Classification task encompasses VQAv2 and NLVR2, whose hyper-parameter setup has been taken from METER (Dou et al., 2022b) and FIBER (Dou et al., 2022a). Model finetuning is done with peak learning rates of 2e-5 for the backbones, 1e-4 for the cross-modal parameters, and 1e-3 for the head layer for 10 epochs with a batch size of 512. The image resolutions are set to 576 for VQAv2 and 384 for NLVR2 and the models are evaluated with the VQA-Scores for VQAv2 and accuracy for NLVR2 (Table C.1).
Image-Text Retrieval (IRTR): We follow Dou et al. (2022a) for IR-TR setup for the Flickr30k dataset, where the cross-attention layers in the backbones are removed during IR-TR fine-tuning and evaluation. The peak learning rates are set to 2e-5 for the backbones, and 1e-4 for the head layer. Furthermore, a batch size of 1024 is considered, and each image resolution is set to 576. We evaluate the Recall@1 metric for both the text and image retrieval tasks as outlined in Table C.1.
Image Captioning: For image captioning, only the image-to-text attentions are kept for the cross-modality attention fusion, and the model is converted into a standard seq2seq model (Dou et al., 2022a). We used a causal mask on the decoding side, and the outputs are predicted auto-regressively (Dou et al., 2022a). Models are trained with the cross-entropy loss for 5 epochs with the peak learning rates of 5e-5 for the backbones, and 2.5e-4 for the rest of the parameters, followed by a two-stage finetuning. In the first stage, finetuning with GOLD (Pang & He, 2021) is done for 5 epochs with a peak learning rate of 1e-5 for the backbones, since it is efficient and has been proven to be effective when the model input can correspond to different outputs. The second stage of fine-tuning involves CIDEr optimization where the learning rate is further reduced to 1e-6, and the model is trained for 3 epochs. A batch size of 512 is considered in both these cases, and a beam size of 5 is used during inference. Evaluation metrics include BLEU (Papineni et al., 2002), METEOR (Banerjee & Lavie, 2005), CIDEr (Vedantam et al., 2015), and SPICE (Anderson et al., 2016) scores (shown in Table C.1).
D.3.3 Fine-grained multi-modal downstream tasks
Referring Expression Comprehension (REC): We follow Dou et al. (2022a) for training and evaluation on 3 different datasets (RefCOCO, RefCOCO+, and RefCOCO) where the models are finetuned with a batch size of 16 for 20 epochs. A warmup of 2000 steps with a peak LR of 1e-5 is used for the OD head as well as the rest of the model’s parameters. LR drops twice, once at 67% and the other at 89% of the total number of steps. Horizontal flip augmentation has been turned off during REC training because it was observed by Dou et al. (2022a) that horizontal flip adversely affected the performance, particularly on the RefCOCO dataset. Accuracy is used as the evaluation metric in this case (Table C.1).
Object Detection: We follow the training and evaluation setup of Dou et al. (2022a) for text-conditioned (multi-modal) object detection. For both COCO and LVIS datasets, the model has been finetuned for 24 epochs with a batch size of 32, an LR of 1e-5, and two learning rate drops, once at 67% and the other at 89% of the total number of steps. AP scores are used in this case for model evaluation (Table C.1).
Appendix E Error Analysis
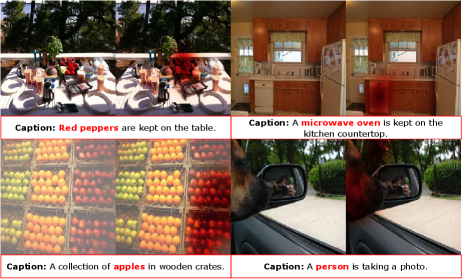
Although VoLTA learns impressive fine-grained region-level understanding during pre-training, there are still some cases where the model fails to identify tiny and hindered objects, especially in cluttered environments. We show four such examples in Figure E.1. In the first image, the object ‘red peppers’ is barely visible even in human eyes, and thus, VoLTA can not precisely identify these objects. However, it can identify the coarse region (the fruit basket) where ‘red peppers’ can be present. In the second image, VoLTA confuses a ‘dishwasher’ with a ‘microwave oven,’ probably because the ‘microwave oven’ is present in a cluttered environment, and both objects have similar appearances in low-resolution frames. In the third image, VoLTA can correctly identify ‘red apples’, but fails to spot ‘green apples’, probably because VoLTA has not seen enough such samples. In the last image, the face of the ‘person’ is hindered by the camera, and VoLTA fails to locate it. Since we pre-train VoLTA with images, such tiny objects are often hard to be distinguished. However, higher-resolution images will be more helpful in addressing such intricate scenarios, which we plan to explore in future works.
Appendix F Additional Quantitative Results on Coarse-grained Vision-Language Tasks: Comparison with Methods using More Pre-training Data
Table F.1 presents a comparison of VoLTA on the multi-modal coarse-grained tasks with state-of-the-art methods pre-trained using magnitude more data. On VQA, VoLTA beats ViLBERT, UNITER-B, VILLA-B, UNIMO-B, and ViLT-B, each pre-trained on M datasets. Please note that VoLTA is trained only on COCO and VG, whereas the other methods use a combination of COCO, VG, CC, and SBU datasets. Such strong performance proves the generalizability of VoLTA. On captioning, VoLTA beats Unified VLP, OSCAR, UFO-B, ViTCAP, VinVL-B, METER-CLIP-B, and XGPT. However, for IRTR and NLVR VoLTA can not yield better performance over these baselines. We assume that the large domain difference between pre-training and downstream datasets is the reason behind the limited performance on IRTR and NLVR.
Method | #Pre-train Data | VQAv | NLVR2 | Fk IRTR | Method | #Pre-train Data | COCO Captioning | ||||||
dev | std | dev | test-P | IR@ | TR@ | B | M | C | S | ||||
Models pre-trained on COCO (k) and/or VG (k) | Models fine-tuned without CIDEr optimization | ||||||||||||
SCAN | k | 48.6 | 67.4 | VL-T5 | k | 34.5 | 28.7 | 116.5 | 21.9 | ||||
SCG | k | 49.3 | 71.8 | VL-BART | k | 35.1 | 28.7 | 116.6 | 21.5 | ||||
PFAN | k | 50.4 | 70.0 | Unified VLP | M | 36.5 | 28.4 | 117.7 | 21.3 | ||||
MaxEnt | k | 54.1 | 54.8 | OSCAR | M | 36.5 | 30.3 | 123.7 | 23.1 | ||||
VisualBERT | k | 70.8 | 71.0 | 67.4 | 67.0 | UFO-B | M | 36.0 | 28.9 | 122.8 | 22.2 | ||
LXMERT | k | 72.4 | 72.5 | 74.9 | 74.5 | ViTCAP | M | 36.3 | 29.3 | 125.2 | 22.6 | ||
SOHO | k | 73.2 | 73.4 | 76.3 | 77.3 | 72.5 | 86.5 | METER-CLIP-B | M | 38.8 | 30.0 | 128.2 | 23.0 |
Models pre-trained on COCO, VG, SBU (M) and/or CC (M) | VinVL-B | M | 38.2 | 30.3 | 129.3 | 23.6 | |||||||
ViLBERT | M | 70.5 | 70.9 | 58.2 | XGPT | M | 37.2 | 28.6 | 120.1 | 21.8 | |||
UNITER-B | M | 72.7 | 72.9 | 77.2 | 77.9 | 72.5 | 85.9 | FIBER-B | M | 39.1 | 30.4 | 128.4 | 23.1 |
VILLA-B | M | 73.6 | 73.7 | 78.4 | 79.3 | 74.7 | 86.6 | FIBER-GOLD-B | M | 40.3 | 30.7 | 133.6 | 23.6 |
UNIMO-B | M | 73.8 | 74.0 | VoLTA-GOLD-B | k | 38.9 | 30.5 | 128.5 | 23.4 | ||||
ViLT-B | M | 71.3 | - | 75.7 | 76.1 | 64.4 | 83.5 | Models fine-tuned with CIDEr optimization | |||||
ALBEF-B | M | 74.5 | 74.7 | 80.2 | 80.5 | 82.8 | 94.3 | ViTCAP | M | 41.2 | 30.1 | 138.1 | 24.1 |
VLMo-B | M | 76.6 | 76.9 | 82.8 | 83.3 | 79.3 | 92.3 | VinVL-B | M | 40.9 | 30.9 | 140.4 | 25.1 |
METER-Swin-B | M | 76.4 | 76.4 | 82.2 | 83.5 | 79.0 | 92.4 | FIBER-B | M | 42.8 | 31.0 | 142.8 | 24.3 |
FIBER-B | M | 78.6 | 78.4 | 84.6 | 85.5 | 81.4 | 92.9 | FIBER-GOLD-B | M | 43.4 | 31.3 | 144.4 | 24.6 |
VoLTA-B | k | 74.6 | 74.6 | 76.7 | 78.1 | 72.7 | 83.6 | VoLTA-GOLD-B | k | 40.2 | 30.9 | 137.5 | 23.7 |
Appendix G Additional Qualitative Results
Visual Question Answering and Visual Reasoning: Visual question answering (VQA) is a widely recognized multi-modal task that infers an answer in response to a text-based question about an image. In Figure G.1, we demonstrated several examples image-question pairs and corresponding answers predicted by VoLTA on the VQAv2 validation set. The primary aim of the visual reasoning task is to ascertain the veracity of a natural language statement against an associated image pair. Figure G.2 displays examples of responses (True/False) predicted by VoLTA on the NLVR2 validation set.
Language-conditioned Object Detection: Object detection forms an indispensable constituent of several multi-modal understanding systems. However, the conventional object detection pipeline is employed as a black-box tool and predicts all possible objects in the image. On the other hand, for better apprehension of combinations of these objects in free-form texts, a language-conditioned object detection task is considered (Kamath et al., 2021; Dou et al., 2022a). We use pre-trained VoLTA and fine-tuned and evaluated COCO and LVIS datasets for the text-conditioned object detection task. As illustrated in Figure G.3, VoLTA predicts bounding boxes relevant to the text prompts (captions) and labels them with the corresponding spans from the text. For example, the top-middle image has 4 objects. However, based on the text prompt, our model predicts boxes only for person and cup.
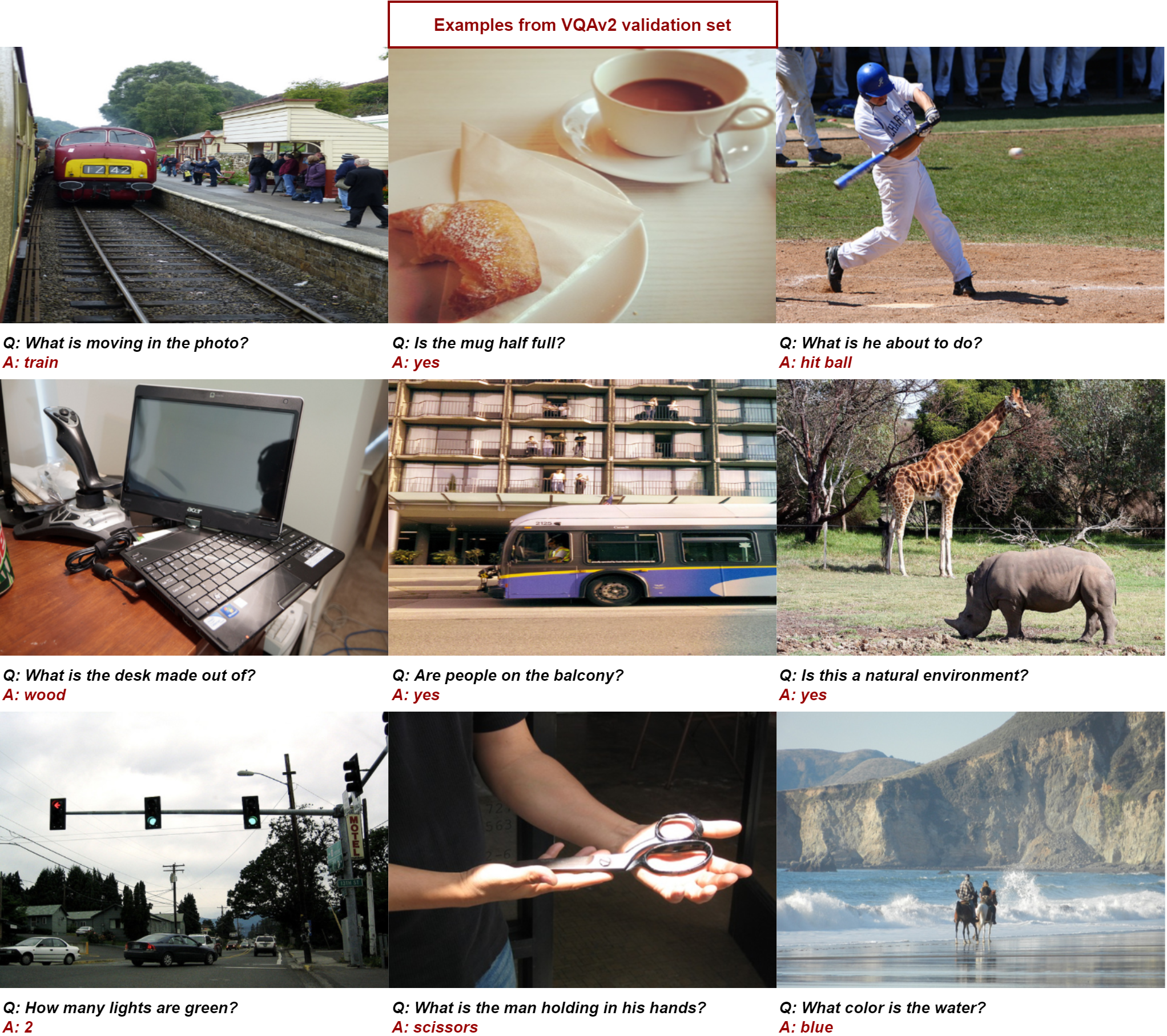
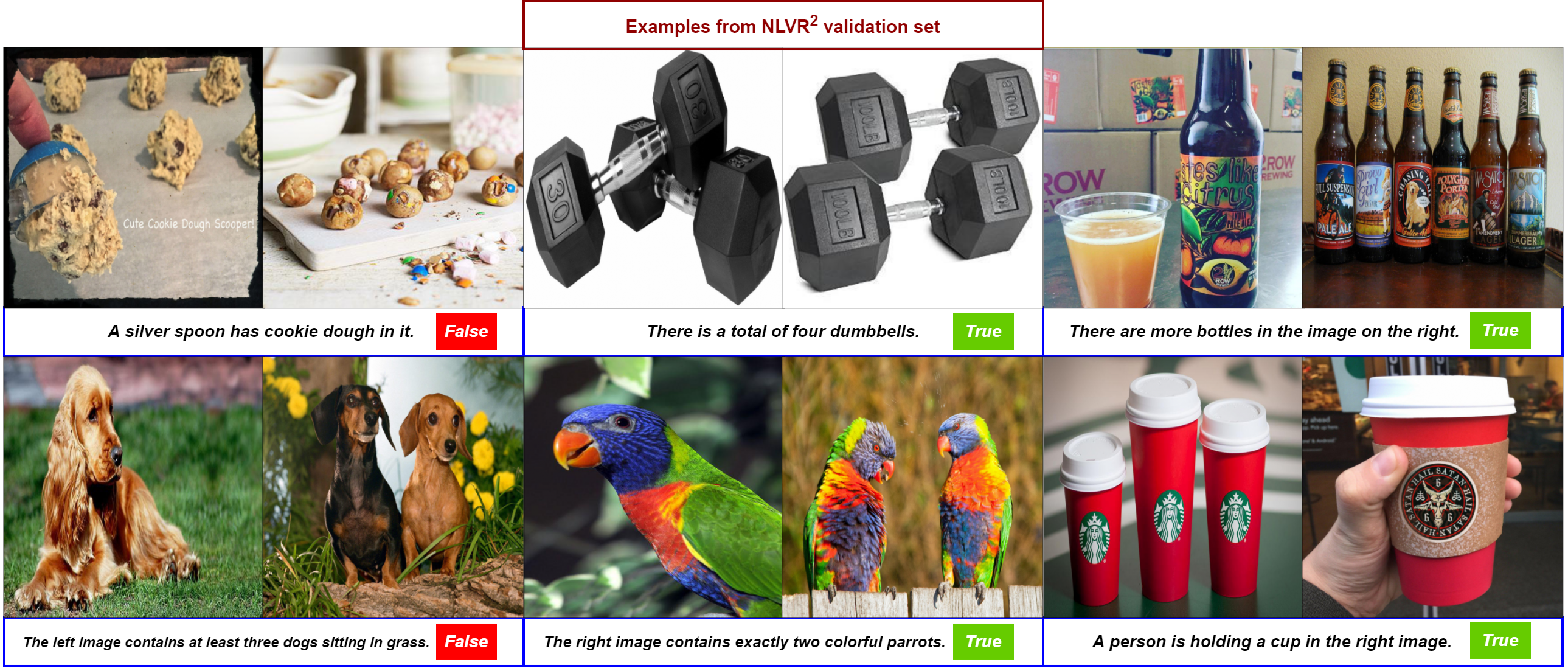
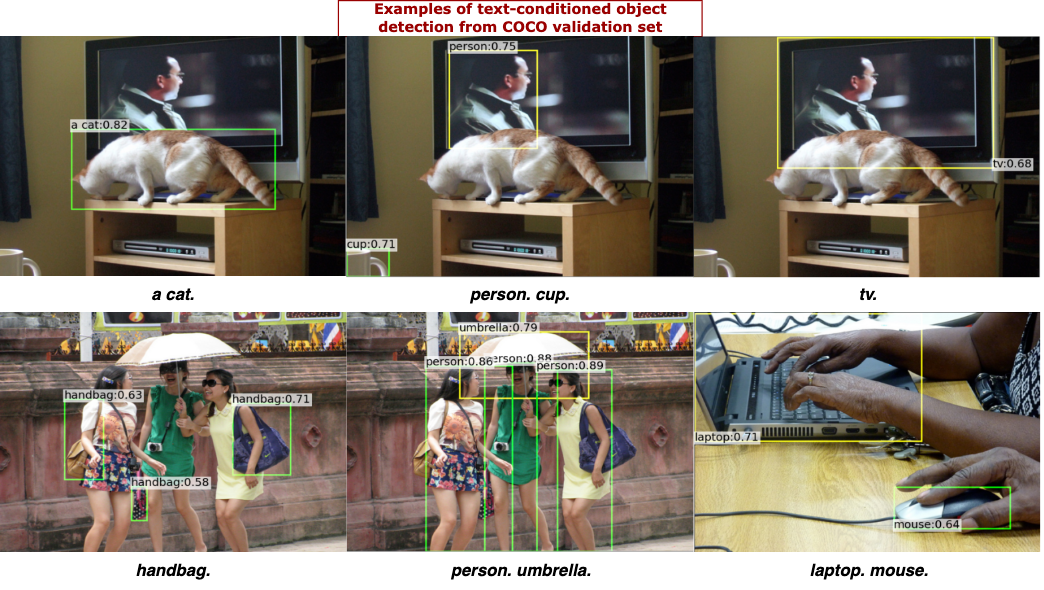
Referring Expression Comprehension (REC): The objective of REC is to align the entire referring expression (text) with the corresponding box by disambiguating among the several occurrences of an object belonging to the same category and therefore, one box per expression is to be predicted. For example, the bottom-left image in Figure G.4 depicts VoLTA’s box prediction for the corresponding referring expression: the slice of cake on the left.
Comparison of CLIP vs. VoLTA on Referring Expression Comprehension (REC): Figure G.5 shows a comparative qualitative evaluation between frozen CLIP + dynamic head (Dai et al., 2021), and VoLTA. We concatenate the vision and text features from the CLIP encoders and train a dynamic head on top of frozen features for the REC task. Since CLIP is a dual-encoder system pre-trained with image-level features, it can not learn superior fine-grained features. Hence, CLIP fails on harder REC samples. For example, if multiple similar-looking objects (benches or bowls) exist in an image, CLIP fails to distinguish between them. However, VoLTA succeeds on such complex samples, which can be attributed to the fine-grained alignment achieved by the GOT objective.
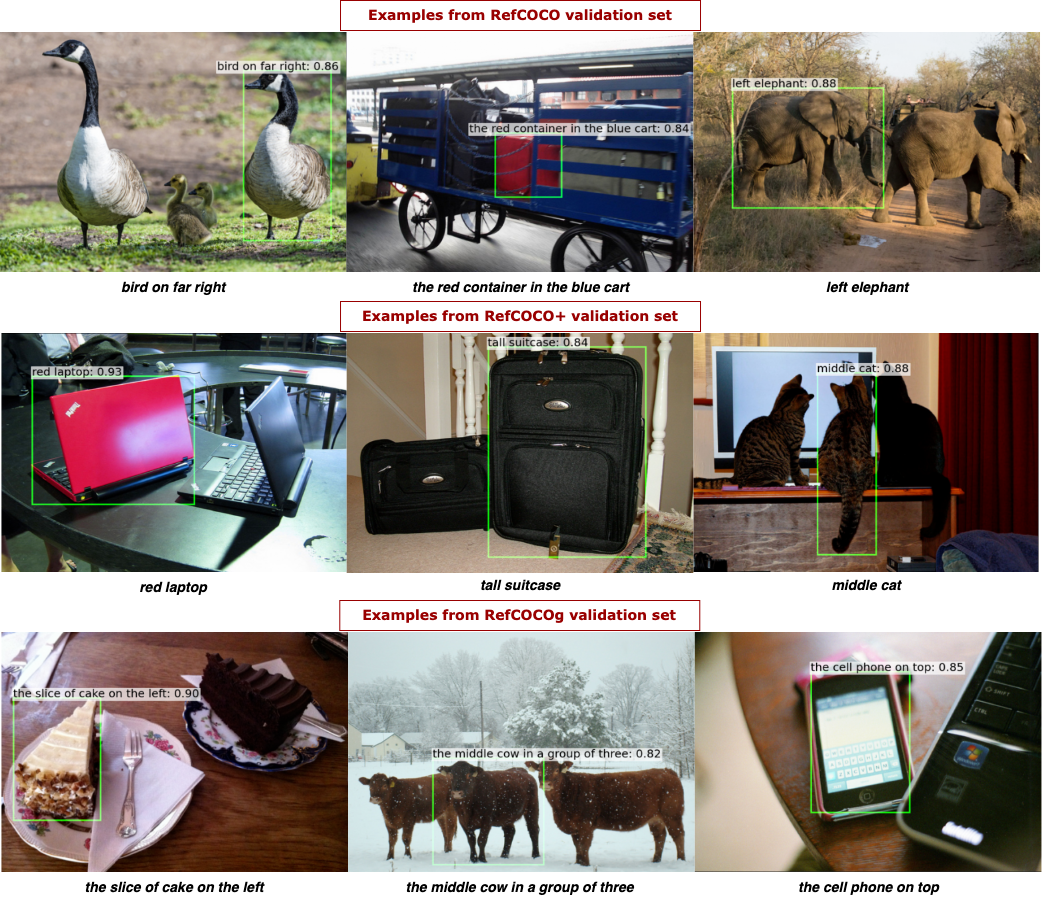
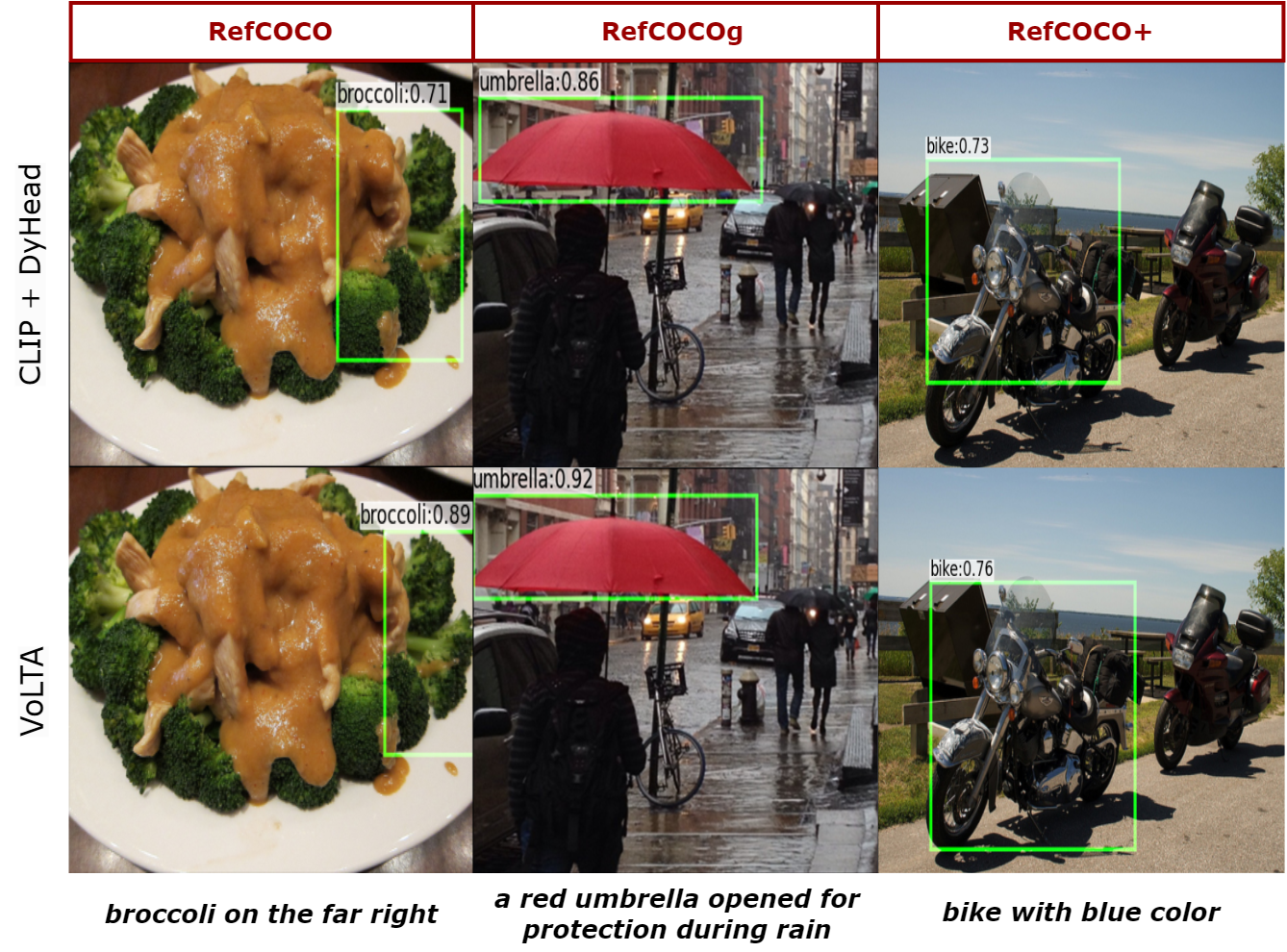
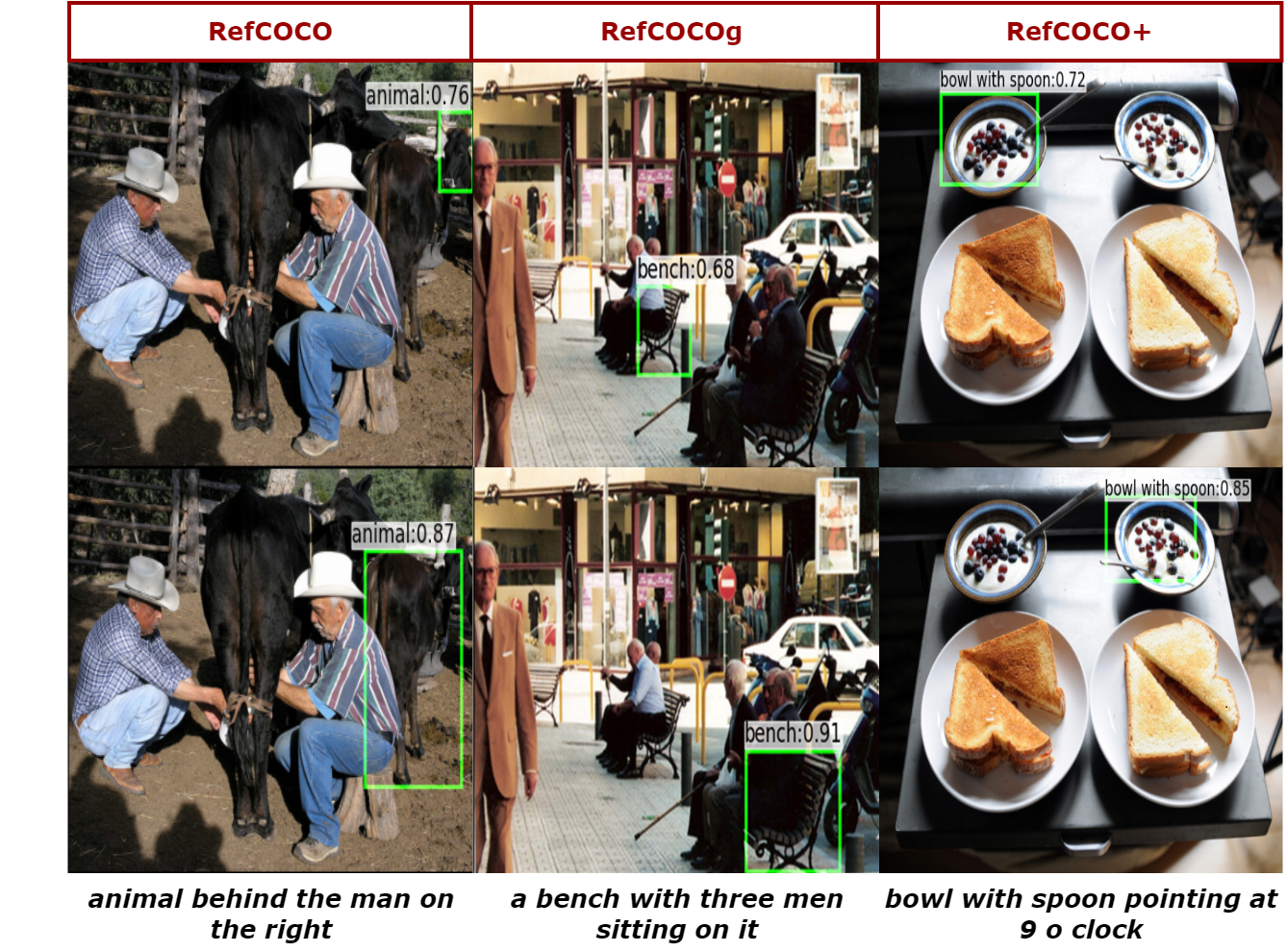
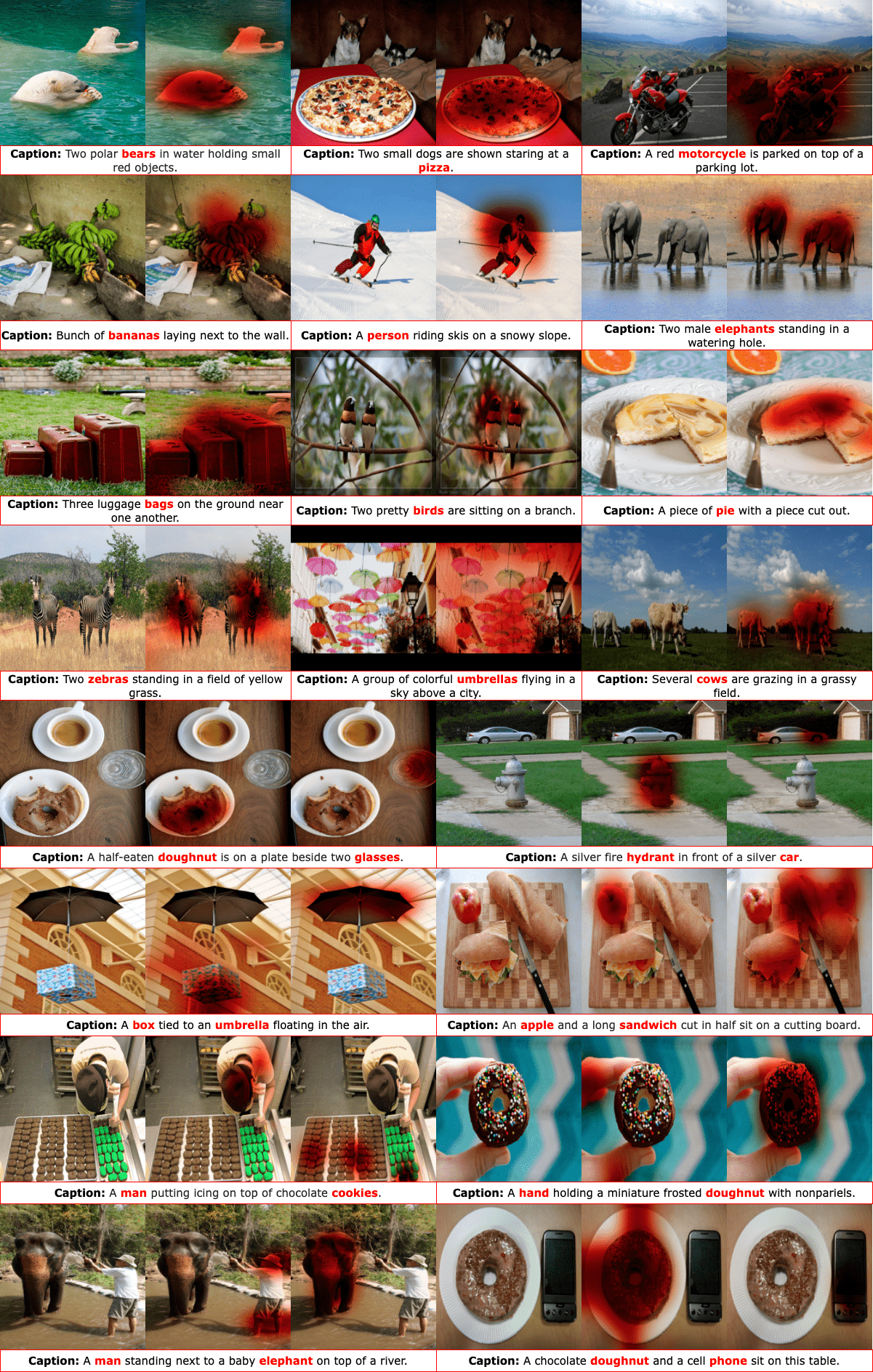