Variational Theory and Algorithms for a Class of Asymptotically Approachable Nonconvex Problems
Abstract
We investigate a class of composite nonconvex functions, where the outer function is the sum of univariate extended-real-valued convex functions and the inner function is the limit of difference-of-convex functions. A notable feature of this class is that the inner function may fail to be locally Lipschitz continuous. It covers a range of important yet challenging applications, including inverse optimal value optimization and problems under value-at-risk constraints. We propose an asymptotic decomposition of the composite function that guarantees epi-convergence to the original function, leading to necessary optimality conditions for the corresponding minimization problem. The proposed decomposition also enables us to design a numerical algorithm such that any accumulation point of the generated sequence, if exists, satisfies the newly introduced optimality conditions. These results expand on the study of so-called amenable functions introduced by Poliquin and Rockafellar in 1992, which are compositions of convex functions with smooth maps, and the prox-linear methods for their minimization. To demonstrate that our algorithmic framework is practically implementable, we further present verifiable termination criteria and preliminary numerical results.
Keywords:
epi-convergence; optimality conditions; nonsmooth analysis; difference-of-convex functions
1 Introduction.
We consider a class of composite optimization problems of the form:
(CP0) |
where for each , the outer function is proper, convex, lower semicontinuous (lsc), and the inner function is not necessarily locally Lipschitz continuous.
If each inner function is continuously differentiable, then the objective in (CP0) belongs to the family of amenable functions under a constraint qualification [25, 26]. For a thorough exploration of the variational theory of amenable functions, readers are referred to [30, Chapter 10(F)]. The properties of amenable functions have also led to the development of prox-linear algorithms, where convex subproblems are constructed through the linearization of the inner smooth mapping [16, 4, 5, 19, 14].
However, there are various applications of composite optimization problem in the form of (CP0) where the inner function is nondifferentiable. In the following, we provide two such examples.
Example 1.1 (The inverse optimal value optimization). For , consider the optimal value function
(1) |
with appropriate dimensional vectors and , and matrices and . The function is not smooth in general. The inverse (multi) optimal value problem [2, 24] finds a vector that minimizes the discrepancy between observed optimal values and true optimal values based on a prescribed metric, such as the -error:
(2) |
If is real-valued for , one can express problem (2) in the form of (CP0) by defining the outer function .
Example 1.2 (The portfolio optimization under a value-at-risk constraint). Given a random variable , the Value-at-risk (VaR) of at a confidence level is defined as . Let be the random return of investments and be a lsc function representing the profit of parameterized by . An agent’s goal is to maximize the expected profit, denoted by , while also controlling the risk via a constraint on under a prescribed level . The model can be written as
(3) |
Define as the indicator function of a set , where for and for . Problem (3) can then be put into the framework (CP0) by setting , , , and . We note that the function can be nondifferentiable even if the function is differentiable for every .
Due to the nondifferentiablity of the inner function in (CP0), the overall objective is not amenable and the prox-linear algorithm [16] is not applicable to solve this composite optimization problem. In this paper, we develop an algorithmic framework for a subclass of (CP0), where each inner function , although nondifferentiable, can be derived from DC functions through a limiting process. We refer to this class of functions as approachable difference-of-convex (ADC) functions (see section 2.1 for the formal definition). It is important to note that ADC functions are ubiquitous. In particular, we will show that the optimal value function in (1) and in (3) are instances of ADC functions under mild conditions. In fact, based on the result recently shown in [31], any lsc function is the epi-limit of piecewise affine DC functions.
With this new class of functions in hand, we have made a first step to understand the variational properties of the composite ADC minimization problem (CP0), including an in-depth analysis of its necessary optimality conditions. The novel optimality conditions are defined through a handy approximation of the subdifferential mapping that explores the ADC structure of . Using the notion of epi-convergence, we further show that these optimality conditions are necessary conditions for any local solution of (CP0). Additionally, we propose a double-loop algorithm to solve (CP0), where the outer loop dynamically updates the DC functions approximating each , and the inner loop finds an approximate stationary point of the resulting composite DC problem through successive convex approximations. It can be shown that any accumulation point of the sequence generated by our algorithm satisfies the newly introduced optimality conditions.
Our strategy to handle the nondifferentiable and possibly discontinuous inner function through a sequence of DC functions shares certain similarities with the approximation frameworks in the existing literature. For instance, Ermoliev et al. [15] have designed smoothing approximations for lsc functions utilizing convolutions with bounded mollifier sequences, a technique akin to local “averaging”. Research has sought to identify conditions that ensure gradient consistency for the smoothing approximation of composite nonconvex functions [10, 8, 6, 7]. Notably, Burke and Hoheisel [6] have emphasized the importance of epi-convergence for the approximating sequence, a less stringent requirement than the continuous convergence assumed in earlier works [10, 3]. In recent work, Royset [32] has studied the consistent approximation of the composite optimization in terms of the global minimizers and stationary solutions, where the inner function is assumed to be locally Lipschitz continuous. Our notion of subdifferentials and optimality conditions for (CP0) takes inspiration from these works but adapts to accommodate nonsmooth approximating sequences that exhibit the advantageous property of being DC.
The rest of the paper is organized as follows. Section 2 presents a class of ADC functions and introduces a new associated notion of subdifferential. In section 3, we investigate the necessary optimality conditions for problem (CP0). Section 4 is devoted to an algorithmic framework for solving (CP0) and its convergence analysis to the newly introduced optimality conditions. We also discuss termination criteria for practical implementation in section 4.3. Preliminary numerical experiments on the inverse optimal value problems are presented in the last section.
Notation and Terminology. Let denote the Euclidean norm in . We use the symbol to denote the Euclidean ball . The set of nonpositive and nonnegative are denoted by and , respectively, and the set of nonnegative integers is denoted by . We write and . Notation is used to simplify the expression of any sequence , where the elements can be points, sets, or functions. By and , we mean that the sequence and the subsequence indexed by converge to , respectively.
Given two sets and in and a scalar , the Minkowski sum and the scalar multiple are defined as and . We also define and whenever . When and are nonempty and closed, we define the one-sided deviation of from as , where . The Hausdorff distance between and is given by . The boundary and interior of are denoted by and . The topological closure and the convex hull of are indicated by and .
For a sequence of sets , we define its outer limit as
and the horizon outer limit as
The outer limit of a set-valued mapping is defined as
We say is outer semicontinuous (osc) at if . Consider some index set . A sequence of sets is equi-bounded if there exists a bounded set such that for all . Otherwise, the sequence is unbounded. If there is an integer such that is equi-bounded, then the sequence is said to be eventually bounded. Interested readers are referred to [30, Chapter 4] for a comprehensive study of set convergence.
The regular normal cone and the limiting normal cone of a set at are given by
The proximal normal cone of a set at is defined as , where is the projection onto that maps any to the set of points in that are closest to .
For an extended-real-valued function , we write its effective domain as , and the epigraph as . We say is proper if is nonempty and for all . We adopt the common rules for extended arithmetic operations, and the lower and upper limits of a sequence of scalars in (cf. [30, Chapter 1(E)]).
Let be a proper function. We write , if . The regular subdifferential and the limiting subdifferential of at are respectively defined as
For any , we set . When is locally Lipschitz continuous at , equals to the Clarke subdifferential . We further say is subdifferentially regular at if is lsc at and . When is proper and convex, , , and coincide with the concept of the subdifferential in convex analysis.
Finally, we introduce the notion of function convergence. A sequence of functions is said to converge pointwise to , written , if for any . The sequence is said to epi-converge to , written , if for any , it holds
The sequence is said to converge continuously to , written , if for any and any sequence .
2 Approachable difference-of-convex functions.
In this section, we formally introduce a class of functions that can be asymptotically approximated by DC functions. A new concept of subdifferential that is defined through the approximating functions is proposed. At the end of this section, we provide several examples that demonstrate the introduced concepts.
2.1 Definitions and properties.
An extended-real-valued function can be approximated by a sequence of functions in various notions of convergence, as comprehensively investigated in [30, Chapter 7(A-C)]. Among these approaches, epi-convergence has a notable advantage in its ability to preserve the global minimizers [30, Theorem 7.31]. Our focus lies on a particular class of approximating functions, wherein each function exhibits a DC structure.
Definition 1.
A function is said to be DC on its domain if there exist proper, lsc and convex functions such that and for any .
With this definition, we introduce the concept of ADC functions.
Definition 2 (ADC functions).
Let be a proper function.
(a) is said to be pointwise approachable DC (p-ADC) if there exist proper functions , DC on their respective domains, such that .
(b) is said to be epigraphically approachable DC (e-ADC) if there exist proper functions , DC on their respective domains, such that .
(c) is said to be continuously approachable DC (c-ADC) if there exist proper functions , DC on their respective domains, such that .
A function is said to be ADC associated with if confirms one of these convergence properties. By a slight abuse of notation, we denote the DC decomposition of each as , although the equality may only hold for .
A p-ADC function may not be lsc. An example is given by , where for a set , we write if and if . In this case, is not lsc at . However, is p-ADC associated with . In contrast, any e-ADC function must be lsc [30, Proposition 7.4(a)], and any c-ADC function is continuous [30, Theorem 7.14].
The relationships among different notions of function convergence, including the unaddressed uniform convergence, have been thoroughly examined in [30]. Generally, pointwise convergence and epi-convergence do not imply one another, but they coincide when the sequence is asymptotically equi-lsc everywhere [30, Theorem 7.10]. In addition, converges continuously to if and only if both and are satisfied [30, Theorem 7.11]. While verifying epi-convergence is often challenging, it becomes simpler for a monotonic sequence that converges pointwise to [30, Proposition 7.4(c-d)].
2.2 Subdifferentials of ADC functions.
Characterizing the limiting and Clarke subdifferentials can be challenging when dealing with functions that exhibit complex composite structures. Our focus in this subsection is on numerically computable approximations of the limiting subdifferentials. We begin with the definitions.
Definition 3 (approximate subdifferentials).
Consider an ADC function associated with . The approximate subdifferential of (associated with ) at is defined as
The approximate horizon subdifferential of (associated with ) at is defined as
Unlike the limiting subdifferential which requires , is defined using all the sequences without necessitating the convergence of function values. It follows directly from the definitions that the mappings and are osc. The following proposition presents a sufficient condition for at any .
Proposition 1.
Let . Then if for any sequence , we have for all sufficiently large . The latter condition is particularly satisfied whenever is closed and for all sufficiently large .
Proof.
Note that for any , we have for all sufficiently large due to . Thus, for any . ∎
In the subsequent analysis, we restrict our attention to . Admittedly, the set depends on the approximating sequence and the DC decomposition of each , which may contain irrelevant information concerning the local geometry of . In fact, for a given ADC function , we can make the set arbitrarily large by adding the same nonsmooth functions to both and . By Attouch’s theorem (see for example [30, Theorem 12.35]), for proper, lsc, convex functions and , if , we immediately have when taking and . In what follows, we further explore the relationships among and other commonly employed subdifferentials in the literature beyond the convex setting. As it turns out, with respect to an arbitrary DC function that is lsc, contains the limiting subdifferential of at any whenever .
Theorem 1 (subdifferentials relationships).
Consider an ADC function . The following statements hold for any .
(a) If is e-ADC associated with and is lsc, then and .
(b) If is locally Lipschitz continuous and bounded from below, then there exists a sequence of DC functions such that , , and . Consequently, , the set is nonempty and bounded, and when is subdifferentially regular at .
Proof.
(a) Let be a DC decomposition of . Since is e-ADC, it must be lsc [30, Proposition 7.4(a)]. Using epi-convergence of to , we know from [30, corollary 8.47(b)] and [30, Proposition 8.46(e)] that any element of can be generated as a limit of regular subgradients at with and for some . Indeed, we can further restrict since and . Then, we have
where the second inclusion can be verified as follows: Firstly, due to the lower semicontinuity of and , and , it follows from the sum rule of regular subdifferentials [30, corollary 10.9] that . Consequently, since and are proper and convex [30, Proposition 8.12]. Similarly, by [30, corollary 8.47(b)], we have
(b) For a locally Lipschitz continuous function , consider its Moreau envelope and the set-valued mapping . For any sequence , we demonstrate in the following that is the desired sequence of approximating functions. Firstly, since is bounded from below, it must be prox-bounded and, thus, each is continuous and for all (cf. [30, Theorem 1.25]). By the continuity of and , we have from [30, Proposition 7.4(c-d)]. It then follows from part (a) that . Consider the following DC decomposition of each :
It is clear that is level-bounded in locally uniformly in , since for any and any bounded set , the set
is bounded. Due to the level-boundedness condition, we can apply the subdifferential formula of the parametric minimization [30, Theorem 10.13] to get
where the last inclusion is due to the calculus rules [30, Proposition 10.5 and exercise 8.8(c)]. Since is convex, we have by [30, Theorem 9.61], which further yields that
(4) |
For any and any , we have
Then, due to the assumption that is bounded from below and therefore . By the local Lipschitz continuity of , it follows from [30, Theorem 9.13] that the mapping is locally bounded at . Thus, there is a bounded set such that for all sufficiently large . It follows directly from [30, Example 4.22] and the definition of the approximate horizon subdifferential that .
Next, we will prove . For any , from (4), there exist sequences of vectors and with each taken from the convex hull of a bounded set . By Carathéodory’s Theorem (see, e.g. [27, Theorem 17.1]), for each , we have for some nonnegative scalars with and a sequence with . It is easy to see that the sequences and are bounded for each . We can then obtain convergent subsequences with and for each . Since , we have by using the outer semicontinuity of . Thus, . This implies that . The rest of the statements in (b) follows from the fact that is nonempty and bounded whenever is locally Lipschitz continuous [30, Theorem 9.61]. ∎
Under suitable assumptions, Theorem 1(b) guarantees the existence of an ADC decomposition that has its approximate subdifferential contained in the Clarke subdifferential of the original function. Notably, this decomposition may not always be practically useful due to the necessity of computing the Moreau envelope for a generally nonconvex function. Another noteworthy remark is that the assumptions and results of Theorem 1 can be localized to any specific point . This can be accomplished by defining a notion of “local epi-convergence” at and extending the result of [30, corollary 8.47] accordingly.
2.3 Examples of ADC functions.
In this subsection, we provide examples of ADC functions, including functions that are discontinuous relative to their domains, with explicit and computationally tractable approximating sequences. Moreover, we undertake an investigation into the approximate subdifferentials of these ADC functions.
Example 2.1 (implicitly convex-concave functions). The concept of implicitly convex-concave (icc) functions is introduced in the monograph [13], and is further generalized to extended-real-valued functions in [20]. A proper function is icc if there exists a lifted function such that the following three conditions hold:
(i) if , and if ;
(ii) is convex for any fixed , and is concave for any fixed ;
(iii) for any .
A notable example of icc functions is the optimal value function in (1), which is associated with the lifted function defined by (the subscripts/superscripts are omitted for brevity):
(5) |
Let and denote the subdifferentials of the convex functions and , respectively, for any . For any , the partial Moreau envelope of an icc function associated with is given by
(6) |
This decomposition, established in [20], offers computational advantages compared to the standard Moreau envelope, as the maximization problem defining is concave in for any fixed . In what follows, we present new results on the conditions under which the icc function is e-ADC and c-ADC based on the partial Moreau envelope. Additionally, we explore a relationship between and , where the latter is known to be an outer estimate of [13, Proposition 4.4.26]. The proof is deferred to Appendix A.
Proposition 2.
Let be a proper, lsc, icc function associated with , where is closed and is lsc on , bounded below on , and continuous relative to . Given a sequence of scalars , we have:
(a) is e-ADC associated with , where each . In addition, if , then is c-ADC associated with .
(b) and for any .
Example 2.2 (VaR for continuous random variables). Given a continuous random variable , its conditional value-at-risk (CVaR) at a confidence level is defined as , where is the value-at-risk given in Example 1.2 (see, e.g., [29]). For any and , we define
(7) |
The following properties of VaR for continuous random variables hold, with proofs provided in Appendix A.
Proposition 3.
Let be a lsc function and be a random vector. Suppose that is convex for any fixed , and is a random variable having a continuous distribution induced by that of for any fixed . Additionally, assume that for any . For any given constant , the following properties hold.
(a) is lsc and e-ADC associated with (with the definitions of and in (7)). Additionally, if is continuous, then is continuous and c-ADC associated with .
(b) If there exists a measurable function such that and for all and , then for any ,
where for a random set-valued mapping and an event is defined as the set of conditional expectations for all measurable selections .
3 The convex composite ADC functions and minimization.
This section aims to derive necessary optimality conditions for (CP0), particularly focusing on the inner function that lacks local Lipschitz continuity. Throughout the rest of this paper, we assume that is proper, convex, lsc and is real-valued for all . Depending on whether is nondecreasing or not, we partition into two categories:
(8) |
We do not specifically address the case where is nonincreasing, as one can always redefine and , enabling the treatment of these indices in the same manner as those in . Therefore, the set should be viewed as the collection of indices where is not monotone. We further make the following assumptions on the functions and .
Assumption 1 For each , we have (a) is e-ADC associated with , and ; (b) for all ; (c) .
From Assumption 1(a), each is locally Lipschitz continuous since any real-valued convex function is locally Lipschitz continuous. Obviously, is sufficient for Assumption 1(b) to hold. Since , we have for each at any . However, does not hold trivially. For example, consider a continuous function and
which results in but . Additionally, Assumption 1(b) ensures that at each point and for any sequence , the sequence must be bounded.
It follows from [30, Exercise 7.8(c)] and [32, Theorem 2.4] that there are several sufficient conditions for Assumption 1(c) to hold, which differ based on the monotonicity of each : (i) For , either is real-valued or ; (ii) For , is c-ADC and for all with , there exists a sequence with . In addition, according to [30, Proposition 7.4(a)], Assumption 1(c) implies that is lsc. We also note that Assumption 1(c) doesn’t necessarily imply . To maintain epi-convergence under addition of functions, one may refer to the sufficient conditions in [30, Theorem 7.46].
3.1 Asymptotic stationarity under epi-convergence.
In this subsection, we introduce a novel stationarity concept for problem (CP0), grounded in a monotonic decomposition of univariate convex functions. We demonstrate that under certain constraint qualifications, epi-convergence of approximating functions ensures this stationarity concept as a necessary optimality condition. Alongside the known fact that epi-convergence also ensures the consistency of global optimal solutions [30, Theorem 7.31(b)], this highlights the usefulness of epi-convergence as a tool for studying the approximation of problem (CP0).
The following lemma is an extension of [13, Lemma 6.1.1] from real-valued univariate convex functions to extended-real-valued univariate convex functions.
Lemma 1 (a monotonic decomposition of univariate convex functions).
Let be a proper, lsc and convex function. Then there exist a proper, lsc, convex and nondecreasing function , as well as a proper, lsc, convex and nonincreasing function , such that . In addition, if , then for any .
Proof.
From the convexity of , is an interval on , possibly unbounded. In fact, we can explicitly construct and in following two cases.
Case 1. If has no direction of recession, i.e., there does not exist such that for any , is a nonincreasing function of , it follows from [27, Theorem 27.2] that attains its minimum at some . Define
Observe that . Consequently, from [27, Theorem 23.8], we have for any .
Case 2. Otherwise, there exists such that for any , is a nonincreasing function of . Consequently, must be an unbounded interval on . Let (or ) be such a recession direction, then is nonincreasing (or nondecreasing) on . We can set and (or and ). In this case, it is obvious that for any . The proof is thus completed. ∎
In the subsequent analysis, we use and to denote the monotonic decomposition of any univariate, proper, lsc, and convex function constructed in the proof of Lemma 1 and, in particular, we take whenever is nondecreasing. We are now ready to present the definition of asymptotically stationary points.
Definition 4 (asymptotically stationary points).
Let each be an ADC function associated with . For each , define
(9) |
We say that is an asymptotically stationary (A-stationary) point of problem (CP0) if for each , there exists such that
(10) |
We say that is a weakly asymptotically stationary (weakly A-stationary) point of problem (CP0) if for each , there exist , and such that
Remark 1.
(i) Given that the approximate subdifferential is determined by the approximating sequence and their corresponding DC decompositions, the notion of (weak) A-stationarity also depends on these sequences and decompositions. (ii) It follows directly from Lemma 1 that an A-stationary point must be a weakly A-stationary point if for each . (iii) When each is nondecreasing or nonincreasing, the concepts of weak A-stationarity and A-stationarity coincide. (iv) Given a point , we can rewrite (10) as
for some index set that is potentially empty. For each , although the scalar does not explicitly appear in this inclusion, its existence implies that , which plays a role in ensuring . For instance, if for some , then , and the existence of yields .
In the following, we take a detour to compare the A-stationarity with the stationarity defined in [32], where the author has focused on a more general composite problem
where is proper, lsc, convex and is a locally Lipschitz continuous mapping. Consider the special case where with . Under this setting, a vector is called a stationary point in [32] if there exist and such that
(11) |
which can be equivalently written as
(12) |
For any fixed , a surrogate set-valued mapping can be defined similarly as in (11) by substituting and with and for each . The cited paper provides sufficient conditions to ensure , which asserts that any accumulation point of a sequence with yields a stationary point . Our study on the asymptotic stationarity differs from [32] in the following aspects:
- 1.
-
2.
We do not require the inner function to be locally Lipschitz continuous.
If each is locally Lipschitz continuous and bounded from below, it then follows from Theorem 1 that is c-ADC associated with such that and for any . Moreover, by , one has . Thus, for any A-stationary point induced by these ADC decompositions, there exists for each such that(13) Hence, is also a stationary point defined in (12). Indeed, A-stationarity here can be sharper than the latter one as the last inclusion in (13) may not hold with equality.
When fails to be locally Lipschitz continuous for some , it is not known if (11) is still a necessary condition for a local solution of (CP0). This situation further complicates the fulfillment of conditions outlined in [32, Theorem 2.4], especially the requirement of , due to the potential discontinuity of . As will be shown in Theorem 2 below, despite these challenges, weak A-stationarity continues to be a necessary optimality condition under Assumption 1.
To proceed, for each and any , we define to be a collection of sequences:
(14) |
Theorem 2 (necessary conditions for optimality).
Let be a local minimizer of problem (CP0). Suppose that Assumption 1 and the following two conditions hold:
(i) For each and any sequence , there is a positive integer such that
(15) |
and
(16) |
(ii) One has
(17) |
Then is an A-stationary point of (CP0). Additionally, is a weakly A-stationary point of (CP0) if for each .
Proof.
By using Fermat’s rule [30, Theorem 10.1] and the sum rule of the limiting subdifferentials [30, Corrollary 10.9] due to the condition (17), we have
(18) |
The inclusion is due to in Assumption 1(c) and approximation of subgradients under epi-convergence [30, corollary 8.47] and [30, Proposition 8.46(e)]; follows from the nonsmooth Lagrange multiplier rule [30, Exercise 10.52] due to the local Lipschitz continuity of [30, Example 9.14] and the condition (15); and use the calculus rules of the Clarke subdifferential [12, Chapter 2.3]. For each , any sequence and any element
there is a subsequence with for some . Next, we show the existence of for each such that
(19) |
By Assumption 1(b), the subsequence is bounded. Taking a subsequence if necessary, we can suppose that . If is unbounded, then has a subsequence converging to and, thus, . Additionally, there exists such that
(20) |
The equation follows from [30, Proposition 8.12] by the convexity of . From and , we must have for sufficiently large . Since is lsc, it holds that and, thus, . Also, notice that is continuous relative to its domain as it is univariate convex and lsc [27, Theorem 10.2]. This continuity implies . The inclusion follows directly from the definition of the horizon subdifferential. Lastly, is due to the lower semicontinuity of the proper convex function and [30, Proposition 8.12]. Therefore, we have with due to , contradicting (16). So far, we conclude that is a bounded sequence. Suppose that and, thus, by the outer semicontinuity of [30, Proposition 8.7].
Case 1. If , inclusion (19) holds trivially for , and for we can find a subsequence such that converges to or with for all . Therefore, (19) follows from
Case 2. Otherwise, . This means that is bounded. Suppose . Then, , and (19) is evident from .
3.2 An example of A-stationarity.
We present an example to illustrate the concept of A-stationarity and to study its relationship with other known optimality conditions.
Example 3.1 (bi-parametrized two-stage stochastic programs). Consider the following bi-parametrized two-stage stochastic program with fixed scenarios described in [21]:
(21) |
where are convex, continuously differentiable for , and , as defined in (1), is real-valued for . At , let and represent the optimal solutions and multipliers for each second-stage problem (1). Suppose that and are bounded. Note that and are ADC functions since they are convex. Example 2.1 shows that is an ADC function, and therefore, problem (21) is a specific case of the composite model (CP0). Given an A-stationary point of (21), under the assumptions of Example 2.1, we have
(22) | ||||
where for and is defined in (5) for . By assumptions, both and are nonempty, bounded, and
It then follows from Danskin’s Theorem [11, Theorem 2.1] that
Combining these expressions with (22), we obtain
which are the Karush-Kuhn-Tucker (KKT) conditions for the deterministic equivalent of (21).
4 A computational algorithm.
In this section, we consider a double-loop algorithm for solving problem (CP0). The inner loop finds an approximate stationary point of the perturbed composite optimization problem
(23) |
by solving a sequence of convex subproblems, while the outer loop drives . It is important to note the potential infeasibility in (23) because in Assumption 1(c), together with , does not guarantee for all . This can be seen from the example of , and . Obviously and by [32, Theorem 2.4(d)], but we have for . Even though for all and each , this does not imply the feasibility of convex subproblems used in the inner loop to approximate (23).
For simplicity of the analysis, we assume that in problem (CP0), is real-valued for , and for . Namely, the problem takes the following form:
(CP1) |
For , the convexity of each real-valued function implies its continuity by [27, corollary 10.1.1]. Consequently, the composite function is also continuous for and due to the continuity of each approximating function . It is important to note that model (CP1) still covers discontinuous objective functions since each can be discontinuous, even though the approximating sequence only consists of locally Lipschitz continuous functions.
4.1 Assumptions and examples.
Firstly, we make an assumption to address the feasibility issue outlined at the start of this section. For all and , define
Based on these auxiliary sequences, we need an initial point that is strictly feasible to the constraints for each .
Assumption 2 (strict feasibility) There exist and nonnegative sequences for , such that for all and
To streamline our notation and analysis, we extend the definitions of and introduce for by setting for all and . Since the quantity depends on the sequence , Assumption 2 poses a condition for this approximating sequence. Consider a fixed index . One can use the following way to construct . Suppose that there exist a function and a nonnegative sequence such that
Additionally, assume that for any fixed , the function is continuous on and differentiable on , and there exists a constant such that for any and . For any fixed , by the mean value theorem, there exists a point such that . Thus,
Two more assumptions on the approximating sequences are needed.
Assumption 3 (smoothness of or ) For each , there exists such that Assumption 4 (level-boundedness) For each , the function is level-bounded, i.e., for any , the set is bounded.
Assumption 3 imposes conditions on the Lipschitz continuity of the subdifferential mapping or , which will be used to determine the termination rule of the inner loop. A straightforward sufficient condition for this assumption is that, for each and , at least one of the functions and is -smooth, i.e., or for any . We also remark that Assumption 3 can hold even though both and are nondifferentiable. This can be seen from the following univariate example: and for any . It is not difficult to verify that Assumption 3 holds for . Assumption 4 is a standard condition to ensure the boundedness of the generated sequences for each .
In addition, we need a technical assumption to ensure the boundedness of the multiplier sequences in our algorithm.
Assumption 5 (an asymptotic constraint qualification) For any , if there exists satisfying where for each (with the definition of in (9)), (24) then we must have .
The normal cone in (24) reduces to for and for . According to the definitions of and , Assumption 5 depends on the approximating sequences for . It holds trivially if each is real-valued and . By Theorem 1(b), the condition holds when the ADC decompositions are constructed using the Moreau envelope, provided that is locally Lipschitz continuous and bounded from below. However, in general, Assumption 5 is not easy to verify. For Example 3.1, the assumption translates into
This is equivalent to the Mangasarian-Fromovitz constraint qualification (MFCQ) for problem (21) by [30, Example 6.40]; see also [28].
Furthermore, if each is c-ADC associated with such that , and , Assumption 5 states that
This condition aligns with the constraint qualification for the composite optimization problem in [32, Proposition 2.1], and is stronger than the condition in the nonsmooth Lagrange multiplier rule [30, Exercise 10.52]. Finally, Assumption 5 implies the constraint qualifications (15)-(17) in Theorem 2. We formally present this conclusion in the following proposition. The proof of Proposition 4 is given in Appendix B.
Proposition 4 (consequences of Assumption 5).
In the following, we use two examples to further illustrate Assumption 3 and the computation of in Assumption 2.
Example 4.1 (icc constraints). Let be real-valued and icc associated with , where is Lipschitz continuous with modulus for any . For the sequence in Example 2.1, it follows from that Assumption 3 holds for . To construct the quantities in Assumption 2, we notice that
(25) |
where the second inequality is due to for any , and the last one uses the bound between the partial Moreau envelope and the original function [20, Lemma 3]. Thus, the sequence satisfies if is summable.
Alternatively, we can construct the quantities as follows. Let the partial Moreau envelope in (6) be the function jointly defined for , and for any . We claim that is continuous on and differentiable on for any fixed . Continuity in can be simply checked by a standard argument [30, Theorem 1.17(c)], noting that the optimal value is achieved at a unique point as the function is strongly convex for any fixed . Differentiability follows from the Danskin’s Theorem [11, Theorem 2.1] that with satisfying for any . It then follows from the Lipschitz continuity of that for any . Therefore, and for any sequence defined by the partial Moreau envelope with .
Example 4.2 (VaR constraints for log-normal distributions). Consider with for some random vector , where is a positive definite covariance matrix. We have . The variable is restricted to a compact set . Denote the -quantile of the standard normal distribution by and the cumulative distribution function of the standard normal distribution by . By direct calculation (cf. [23, Section 3.2]), we have
Hence, is neither convex nor concave if . For the sequence in Example 2.2, we can derive that
Since is positive definite, it is easy to see that is twice continuously differentiable. Consequently, is -smooth relative to the compact set for some , and Assumption 3 holds (relative to ). Next, we define for any and for any . Obviously, is continuous on and differentiable on for any fixed . By using the Leibniz rule for differentiating the parametric integral, for , we have
for some by the mean-value theorem. By using the fact , the monotonicity , and the compactness of , we further have
Therefore, and for any sequence with .
4.2 The algorithmic framework and convergence analysis.
We now formalize the algorithm for solving (CP1). For , recall the nonnegative sequences introduced in Assumption 2, and observe that as . For consistency of our notation, we also set for all and . At the -th outer iteration and for , consider the upper and lower approximation of at a point by taking some , and incorporating sequences :
(26) |
Observe that, for fixed , the upper approximation is convex while the lower approximation is concave. For , consider the following function
(27) |
which is a convex majorization of at a point by the fact that is nondecreasing and is nonincreasing. For , consider the convex constraint as an approximation for .
We summarize the properties of all the surrogate functions as follows. Note that (28a) and (28b) hold for , while (28c) holds only for .
(28a) | ||||
(28b) | ||||
(28c) |
The proposed method for solving problem (CP1) is outlined in Algorithm 1. The inner loop of the algorithm (indexed by ) is terminated when the following conditions are satisfied:
(29) |
Input: Given and satisfying Assumption 2. Let be a sequence satisfying Assumption 3. Choose , a positive sequence such that . Set .
(30) |
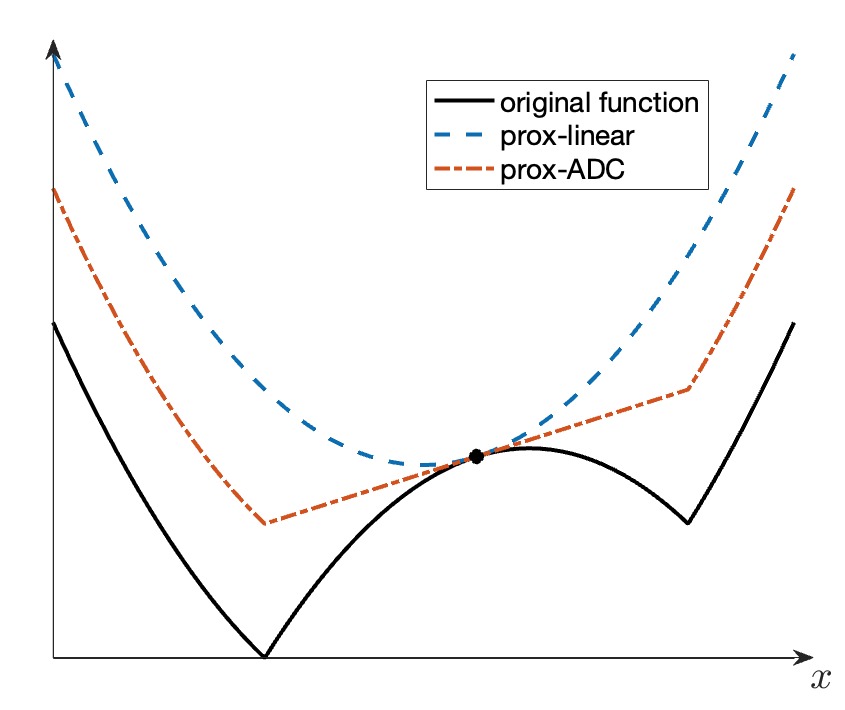
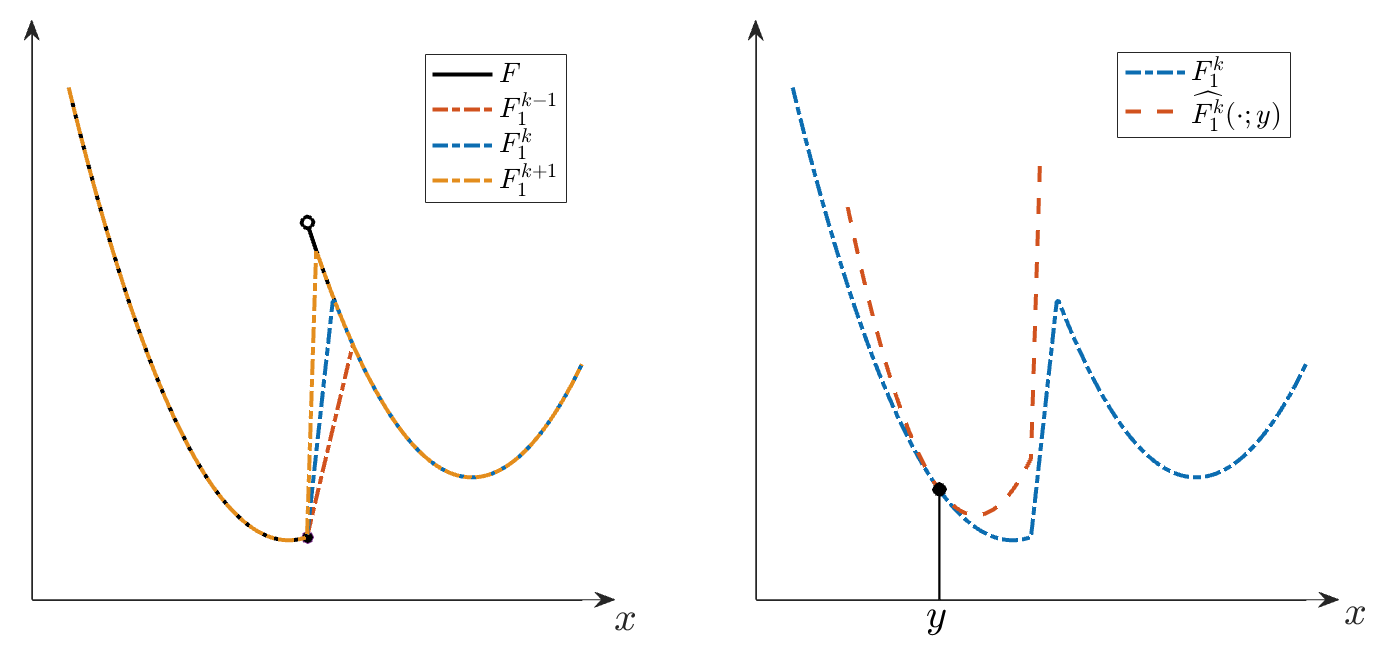
In contrast to the prox-linear algorithm that is designed to minimize amenable functions and adopts complete linearization of the inner maps, the prox-ADC method retains more curvature information inherent in these maps (see Figure 1). We emphasize that the prox-ADC method differs from [13, Algorithm 7.1.2] that is designed for solving a problem with a convex composite DC objective and DC constraints. Central to the prox-ADC method is the double-loop structure, where, in contrast to [13, Algorithm 7.1.2], the DC sequence is dynamically updated in the outer loop rather than remaining the same. This adaptation necessitates specialized termination criteria (29) and the incorporation of to maintain feasibility with each update of . In the following, we demonstrate the well-definedness of the prox-ADC method. Specifically, we establish that for each iteration , the criteria detailed in (29) are attainable in finitely many steps.
Theorem 3 (convergence of the inner loop).
Proof.
We prove (a) and (b) by induction. For , notice from Assumption 2 and (28a) that for . Thus, problem (30) is feasible for . Assume that (30) is feasible for and some . Consequently, is well-defined and for ,
which yields the feasibility of (30) for , . Hence, by induction, problem (30) is feasible for and any . To proceed, recall the function defined in Assumption 4. From the update of , we have
(31) |
The last inequality follows from the definition of and the second relation in (28c) that for . Observe that is bounded from below by the continuity of for (see the discussion following model (CP1)) and the level-boundedness of . Suppose for contradiction that the stopping rule of the inner loop is not achievable in finitely many steps. Then from (31), converges and . The latter further yields and thus the last condition in (29) is achievable in finitely many iterations. Next, to derive a contradiction, it suffices to prove that the first two conditions in (29) can also be achieved in finitely many steps. We only show the first one since the other can be done with similar arguments. By the level-boundedness of , the set is compact. Notice that for all due to (31). For , we then have
because is uniformly continuous on the compact set and is bounded by [27, Theorem 24.7]. Therefore, for a fixed , there exists some such that holds for . Thus, (a)-(b) hold for .
Now assume that (a)-(b) hold for some and, hence is finite. It then follows from and that for each ,
Thus, problem (30) is feasible for and any . Building upon this, we can now clearly see the validity of (b) for , as we have shown similar results earlier in the case of . By induction, we complete the proof of (a)-(b). ∎
For any , define the set of multipliers for problem (30) as
Here is uniquely determined by as the minimizer of a strongly convex problem (30). Notice that for since is nondecreasing and for . Let be a subsequence that converges to some point . As we will see in the following lemma, the asymptotic constraint qualification in Assumption 5 implies the non-emptiness and the compactness of for all sufficiently large and the eventual boundedness of . These technical results play an important role in the convergence analysis of the prox-ADC method. However, a stronger property of equi-boundedness appears necessary for designing practical termination criteria for the algorithm. We will establish this strengthened property in section 4.3 under non-asymptotic constraint qualifications.
Lemma 2 (non-emptiness and eventual boundedness of multipliers).
Let be a feasible point of problem (CP1). Suppose that Assumptions 1-5 hold. Consider any sequence generated by the prox-ADC method, with a subsequence converging to . The following statements hold.
(a) The set of multipliers is non-empty and compact for all sufficiently large .
(b) Additionally, if for (with the definition of in (8)), then the sequence is eventually bounded.
Proof.
(a) Observe that because and by conditions (29). The non-emptiness and compactness of for all sufficiently large is a direct consequence of the nonsmooth Lagrange multiplier rule [30, Exercise 10.52] for problem (30) if we can show that, for all sufficiently large , is the unique solution of the following system
(32) |
Suppose that the above claim does not hold. Then, there exists a subsequence such that is not the unique solution of (32) for all . Without loss of generality, suppose and take for satisfying (32) and . For each and , define
Then, for all , we have
where uses the inequalities and ; the first term in is because of the construction of upper convex majorization (26); the second term in is due to so that
Inequality is due to (32) and ; is by Assumption 3; and is implied by conditions (29) and . Equivalently, for all and , there exist with and
such that . For , since the subsequence is bounded by Assumption 1(b), we can assume without loss of generality that converges to some as . Furthermore, it can be easily seen from (28a) and (29) that converges to the same limit point for . Notice that for all and from Theorem 3(a) and, thus, each must satisfy . Suppose that for each . Then, by the outer semicontinuity of the normal cone [30, Proposition 6.6],
Obviously, , and the sequence has at least one nonzero element. Consider two cases.
Case 1. If is bounded for , then there are vectors with such that and , contradicting Assumption 5 since are not all zeros.
Case 2. Otherwise, there exists some such that is unbounded. Define the index sets
Notice that is bounded. Without loss of generality, assume that this sequence converges to some and, thus, .
Step 1: Next we prove by contradiction that, for each , the sequence is bounded. Suppose that the boundedness fails and by passing to a subsequence. Consider for . Then . Since for all , we can assume that there exist and such that . It then follows from the construction of that has a subsequence converging to some element of for each and, in particular, . From , we obtain
a contradiction to Assumption 5 since the coefficient of the term is nonzero. So far, we have shown the boundedness of for each .
Step 2: Now suppose that for each with . Thus and for each . Since , there exists such that . Then implies
which leads to a contradiction to Assumption 5. Thus, is non-empty and compact for all sufficiently large .
(b) By part (a), assume from now on that for all without loss of generality. We also assume that converges to some point for . Then, by (28a), (28b) and (29), for and for . For any and any , we have and for satisfying
(33) |
Due to Assumption 3 and similar arguments in the proof of part (a), the optimality condition (33) implies that
(34) |
Note that, for , is nondecreasing, i.e., . Then for all and , and the first inequality of (34) is equivalent to
(35) |
Observe that the sequences and must be bounded for . Otherwise, we could assume . Then every accumulation point of the unit vectors would be in the set , contradicting our assumption that for each .
For , given that is convex, real-valued, and , we can invoke [27, Theorem 24.7] to deduce the boundedness of . A parallel reasoning applies to demonstrate the boundedness of .
For , we proceed by contradiction to establish the boundedness of based on Assumption 5. Suppose that is unbounded and by passing to a subsequence. Consider the normalized subsequences and for each . Consequently, and for . By the triangle inequality and (35), we have
which further implies by the boundedness of and for . Now suppose that for . Then from a similar reasoning in (20), for ,
and obviously . The remaining argument to derive a contradiction to Assumption 5 follows the same steps as the proof of part (a) for the two cases, with the exception that the index set is replaced by . Thus, the sequences for and for are bounded. We can conclude that is bounded for sufficiently large integer , because otherwise we could extract a subsequence of multipliers from whose norms diverge to as , in contradiction to the result of boundedness that we have shown. Hence, the subsequence is eventually bounded. ∎
We make a remark on Lemma 2(b) about the additional assumption. According to the proof of part (b), the assumption for ensures the boundedness of the set for . There are some sufficient conditions for to hold: (i) If is locally Lipschitz continuous and bounded from below, by Theorem 1(b), we have at any for the approximating sequence generated by the Moreau envelope. (ii) If is icc associated with satisfying all assumptions in Proposition 2, it then follows from Proposition 2(b) that at any for the approximating sequence based on the partial Moreau envelope. It is worth mentioning that the icc function under condition (ii) is not necessarily locally Lipschitz continuous.
The main convergence result of the prox-ADC method follows.
Theorem 4.
Suppose that Assumptions 1-5 hold. Let be the sequence generated by the prox-ADC method. Suppose that has an accumulation point and, in addition, for . Then is a weakly A-stationary point of (CP1). Moreover, if for each , the functions and are -smooth for all , i.e., there exists a sequence such that for all ,
(36) |
then is also an A-stationary point of (CP1).
Proof.
Let be a subsequence converging to . By the stopping conditions (29) and , we also have . First, we prove . From Theorem 3(a), we have for and all . Due to epi-convergence in Assumption 1(c), it holds that
Thus for and . By Assumption 1(a), for all . This implies , and we can conclude that .
By Lemma 2(a), for all sufficiently large , we have
(37) |
where and for . It follows from Lemma 2(b) that the subsequences and are bounded for . Suppose that and for . Recall that the subsequence is bounded by Assumption 1(b) for . Without loss of generality, assume that converges to some point for . Then, by (28a), (28b) and (29), for and for . From the outer semicontinuity of and , we have for and for .
To proceed, we prove by contradiction that the sequence is bounded for . Suppose that . For each , the boundedness of and follows from the assumption ; otherwise, any accumulation point of the unit vectors would be in , leading to a contradiction. Since and for are also bounded, we conclude that the subsequence is bounded. Thus, we can assume that
By (35), it follows that . Consider for , and then . Given for all , there must exist such that . For each , it then follows from that has a subsequence converging to some element in . In particular, . Since , this implies that
which contradicts Assumption 5. Hence, is bounded for .
We are now ready to prove that is a weakly A-stationary point. Suppose that for with . It remains to show that for each , there exists such that
which can be derived similarly as the proof of (19) in Theorem 2. Summarizing these arguments, we conclude that is a weakly A-stationary point of (CP1).
Under the additional assumption of the theorem, there exist , for , and
such that
where is implied by the optimality condition (37), employs (36) and Assumption 3, and follows from conditions (29). This inequality is a tighter version of (35) in the sense that, for each and , and are elements taken from the single-valued mapping evaluated at the same point . A straightforward adaptation of the preceding argument confirms that is an A-stationary point of (CP1). ∎
4.3 Termination criteria.
The previous subsection demonstrates the asymptotic convergence of the algorithm, showing that any accumulation point of the sequence generated by the prox-ADC method is weakly A-stationary. This subsection is dedicated to the non-asymptotic analysis of verifiable termination criteria for practical implementation.
Assumption 6 (non-asymptotic constraint qualifications) Let be the parameter in Algorithm 1. For all and any pair satisfying if there exist for such that then we must have .
A direct consequence of Assumption 6 and the nonsmooth Lagrange multiplier rule [30, Exercise 10.52] is that the set of multipliers is non-empty and compact for any fixed . This is in contrast with Lemma 2(a), where the results only hold for sufficiently large . We will show below that the result on the eventual boundedness of the subsequence can be strengthened to the equi-boundedness under this assumption.
Proposition 5 (equi-boundedness of multipliers).
Suppose that Assumptions 1-6 hold. Consider any sequence generated by the prox-ADC method. The following statements hold.
(a) If there is a subsequence converging to some and for , then the subsequence is equi-bounded.
(b) If is bounded and for any and , then the sequence is equi-bounded, i,e,
(38) |
Proof.
(a) We know from Lemma 2(b) that the subsequence is eventually bounded. This implies the existence of an index such that is bounded. On the other hand, it follows from Assumption 6 that is non-empty and compact for any fixed . Thus, is bounded, and is equi-bounded.
(b) Suppose for contradiction that is not equi-bounded. Then for any nonnegative integer , there is an index such that for some multiplier . Observe that the nonnegative sequence of indices is either bounded or unbounded. It suffices to consider these two cases separately.
Suppose first that is bounded. There must be an index that appears infinitely many times in . Consequently, the set is unbounded, a contradiction to Assumption 6.
Suppose next that is unbounded. For some index set , we have as . Notice that the subsequence is bounded since is bounded. By passing to a subsequence if necessary, we assume that converges to some . Using epi-convergence in Assumption 1(c) and following the same procedure as in the proof of Theorem 4, we can obtain that . Then, by the assumption in (b), for . Henceforth, there is a subsequence converging to some with a corresponding subsequence of multipliers such that as , which is a contradiction to the result of part (a).
We have obtained contradictions for the two cases where is bounded or unbounded. Then we can conclude that is equi-bounded and the quantity defined in (38) is finite. ∎
After obtaining the equi-boundedness of the multipliers, we next introduce a relaxation of the weakly A-stationary point for preparation of the termination criteria. For a proper and convex function and any , we denote , which is related to the Goldstein’s -subdifferential [18].
Definition 5.
Given any , and , we say a point is a -weakly A-stationary point of problem (CP0) if there exists a nonnegative integer such that
We remark that, if each outer function is an identity function, i.e., for any , and each inner function is DC rather than ADC, the above definition in the context of a DC program is independent of and says about nearness to a -critical point [35, definition 2]. For nonsmooth optimization problem, similar definitions based on the idea of small nearby subgradients, together with the termination criteria, have appeared in the literature [18, 9].
The following proposition reveals the relationship between a -weakly A-stationary point and a weakly A-stationary point.
Proposition 6.
Proof.
By Assumption 1(b), the subsequence is bounded for each . Then, there is an index set such that converges to some for each . Using the outer semicontinuity of the subdifferential mapping of a convex function, we have
and
Thus, by taking an outer limit of the subdifferentials involved in the condition that is -weakly A-stationary for all , we know that is a weakly A-stationary point of (CP0). ∎
We conclude this section with our main result on the termination criteria.
Proposition 7 (termination criteria).
Proof.
The existence of satisfying (39) is a direct consequence of , , and . Recalling (34) in the proof of Lemma 2, at the -th outer iteration, we have
where , for . Thus, at the point , we have
where the parameters and are given by
Henceforth, for satisfying (39), is a -weakly A-stationary point of problem (CP1). ∎
5 Numerical examples.
We present some preliminary experiments to illustrate the performance of our algorithm on the inverse optimal value optimization with or without constraints. The first experiment aims to demonstrate the practical performance of the prox-ADC method under the termination criteria in section 4.3, by varying different approximating sequences and initial points. To demonstrate the computation of ADC constrained problems, especially the choice of the quantity and a feasible initial point in Assumption 2, we further consider the constrained inverse optimal value optimization. These experiments were tested on a MacBook Air laptop with an Apple M1 chip and 16GB of memory using Julia 1.10.2.
5.1 Inverse optimal value optimization with simple constraints.
Based on the setting in (2), we aim to find a vector to minimize the errors between the observed optimal values and true optimal values :
(40) |
where each is the optimal value function as defined in (1). We fix , , , and the number of inequality constraints in the minimization problem (1). Vectors and , and matrices are randomly generated with each entry independent and normally distributed with mean and variance . For numerical stability, we then normalize matrices and by a factor of . We also generate a positive definite matrix and a random solution with . We set for each and, therefore, .
We adopt the ADC decomposition in (6), denoted by with a sequence for some exponent . Consequently, . We apply the prox-ADC algorithm to solve this example with and . In this example, the strongly convex subproblem (30) can be easily reformulated to a problem with linear objective and convex quadratic constraints, which is solved by Gurobi in our experiments.
We first investigate the performance of our algorithm under the termination criteria with different values of parameters. Figure 2 displays the logarithm of the objective values against the number of outer iterations and the total number of inner iterations. We mark three different points on the curve where the termination criteria (39) with and are satisfied.
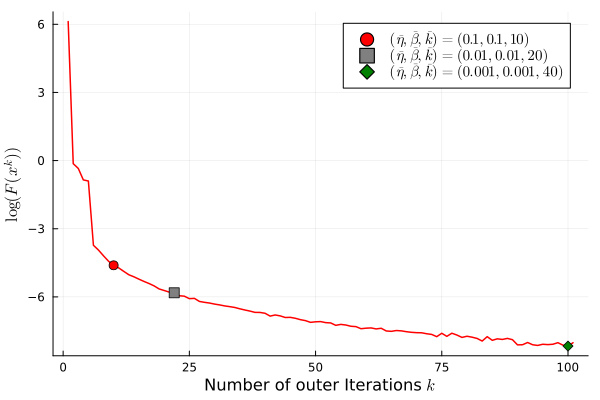
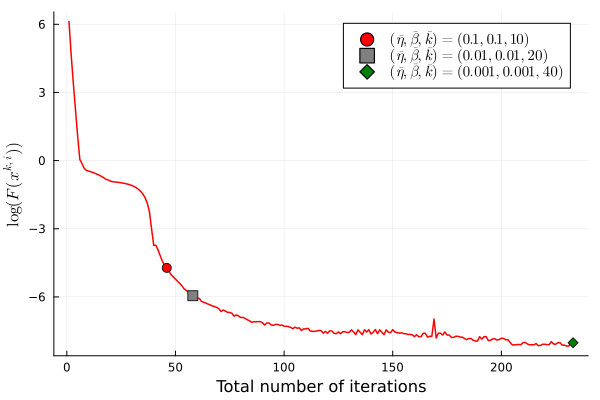
We have also experimented with various values of exponent that determine the convergence rate of the approximating sequence and various initial points. In both cases, we terminate the algorithm under the conditions (39) with and . In Figure 3, we observe that setting different values of under the same termination criteria leads to candidate solutions with similar objective values, and there are roughly two phases of convergence in terms of the total number of iterations. Initially, the objective value decreases faster for smaller , corresponding to poorer approximation. When the objective value is sufficiently small ( on this particular instance), larger results in faster convergence to high accuracy. We remark that for , the algorithm reaches the maximum number of outer iterations and does not output a -weakly A-stationary point. Figure 4 demonstrates the influence of using various initial points that are uniformly distributed on . On this instance, two of the initial points find -weakly A-stationary points with large objective values. For these two initial points, we rerun the algorithm with , and the algorithm still terminates with large objective values.
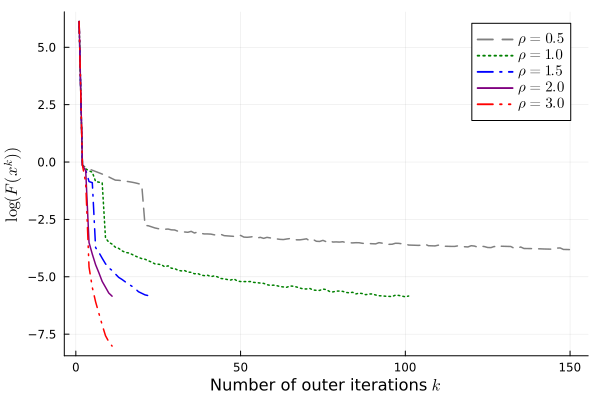
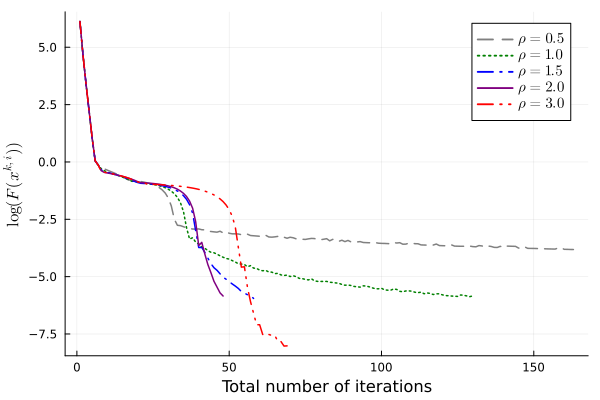
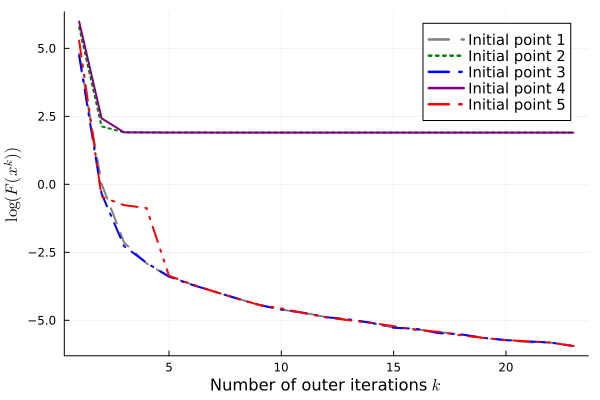
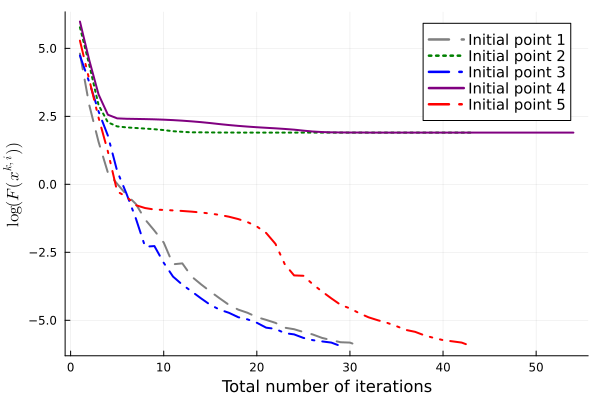
5.2 Inverse optimal value optimization with ADC constraints.
We consider a variant of the inverse optimal value optimization that is defined as follows:
(41) |
In this formulation, the observations of the optimal values are divided into two groups indexed by and . We aim to minimize the errors for the first group while ensuring the relative errors for the second group do not exceed a specified feasibility tolerance, denoted by . In our experiment, we fix , , , , , and the number of inequality constraints in the minimization problem (1). The solution and the data, including , are randomly generated in the same way as in section 5.1. We can see that is feasible to (41) and attains the minimal objective value .
Similar to the first example, we adopt the ADC decomposition in (6), denoted by with a sequence for some positive integer and . Due to the feasibility problem in Assumption 2, we introduce the additional parameter to control the approximating sequences, which will be explained in details later. We also note that treating and as two separate constraints for leads to the failure of the asymptotic constraint qualification in Assumption 5 because the approximate subdifferentials of the ADC functions and are linearly dependent. This issue can be resolved by rewriting the constraints in a composite ADC form and assuming a corresponding version of Assumption 5. We omit this technical detail since the main focus of this section is to illustrate the practical implementation of our algorithm.
To verify Assumption 2 that states the existence of a strictly feasible point, we first follow the discussion after Assumption 2 to construct the quantity where is the Lipschitz constant of for all . We can derive the Lipschitz constant by characterizing the subdifferential for a fixed based on Danskin’s Theorem [11, Theorem 2.1] and then upper bounding the norm of this subdifferential over . The extra denominator in the expression of is due to the scaling of the constraints in (41). Then, consider the following problem:
(Feas) |
where the objective is the sum of the compositions of univariate convex functions and DC functions . Notice that problem (Feas) takes the same form as (23). Thus, we can apply the inner loop of the prox-ADC method to solve it approximately. If solving this problem gives a solution with , then
(42) |
and is a strictly feasible point satisfying Assumption 2. We emphasize that using the inner loop of the prox-ADC method for solving problem (Feas) to obtain a strictly feasible point is merely a heuristic. Although this approach works well in our experiments, it is generally not easy to verify Assumption 2. We make a final remark on the role of . For small values of and , it is possible that , and, from (42), there is no strictly feasible point satisfying Assumption 2 for this fixed approximating sequence. Henceforth, the flexibility of the parameter is necessary to ensure the validity of Assumption 2.
We implement the above procedure to find an initial point and then apply the prox-ADC method with and . On most of the randomly generated instances, we observe that the point given by solving (Feas) is also feasible to the original problem (41) along the iterations, although this result cannot be implied by Assumption 2. In Figure 5, we again plot the logarithm of the objective values against the total number of iterations, using various combination of and and various initial points. It is worth mentioning that for this constrained problem, the random initial point is not directly utilized in the prox-ADC method. Instead, it is first used in problem (Feas) to generate a strictly feasible point satisfying Assumption 2, and the candidate solution for (Feas) then becomes the initial point of the prox-ADC method for solving (41).
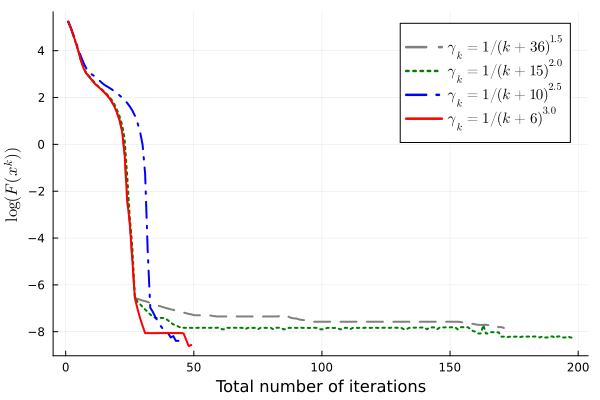
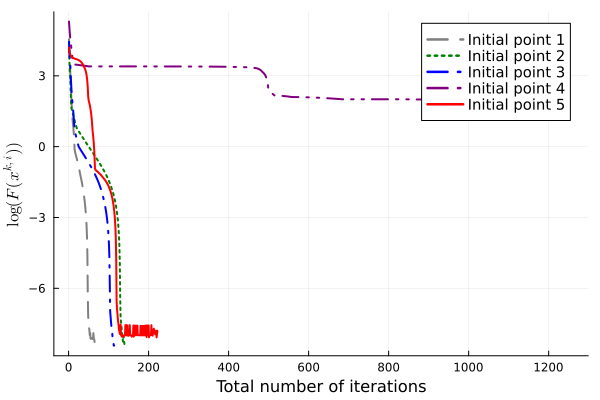
Acknowledgments.
The authors are partially supported by the National Science Foundation under grants CCF-2416172 and DMS-2416250, and the National Institutes of Health under grant 1R01CA287413-01. The authors are grateful to the associate editor and two reviewers for their careful reading and constructive comments that have substantially improved the paper.
References
- [1] Carlo Acerbi. Spectral measures of risk: A coherent representation of subjective risk aversion. Journal of Banking and Finance, 26(7):1505–1518, 2002.
- [2] Shabbir Ahmed and Yongpei Guan. The inverse optimal value problem. Mathematical Programming, 102(1):91–110, 2005.
- [3] Amir Beck and Marc Teboulle. Smoothing and first order methods: A unified framework. SIAM Journal on Optimization, 22(2):557–580, 2012.
- [4] James V Burke. Descent methods for composite nondifferentiable optimization problems. Mathematical Programming, 33(3):260–279, 1985.
- [5] James V Burke and Michael C Ferris. A Gauss-Newton method for convex composite optimization. Mathematical Programming, 71(2):179–194, 1995.
- [6] James V Burke and Tim Hoheisel. Epi-convergent smoothing with applications to convex composite functions. SIAM Journal on Optimization, 23(3):1457–1479, 2013.
- [7] James V Burke and Tim Hoheisel. Epi-convergence properties of smoothing by infimal convolution. Set-Valued and Variational Analysis, 25(1):1–23, 2017.
- [8] James V Burke, Tim Hoheisel, and Christian Kanzow. Gradient consistency for integral-convolution smoothing functions. Set-Valued and Variational Analysis, 21(2):359–376, 2013.
- [9] James V Burke, Adrian S Lewis, and Michael L Overton. A robust gradient sampling algorithm for nonsmooth, nonconvex optimization. SIAM Journal on Optimization, 15(3):751–779, 2005.
- [10] Xiaojun Chen. Smoothing methods for nonsmooth, nonconvex minimization. Mathematical Programming, 134(1):71–99, 2012.
- [11] Frank H Clarke. Generalized gradients and applications. Transactions of the American Mathematical Society, 205:247–262, 1975.
- [12] Frank H Clarke. Optimization and Nonsmooth Analysis. Society for Industrial and Applied Mathematics, Philadelphia, 1990.
- [13] Ying Cui and Jong-Shi Pang. Modern Nonconvex Nondifferentiable Optimization. Society for Industrial and Applied Mathematics, Philadelphia, 2021.
- [14] Dmitriy Drusvyatskiy and Courtney Paquette. Efficiency of minimizing compositions of convex functions and smooth maps. Mathematical Programming, 178(1–2):503–558, 2019.
- [15] Yuri M Ermoliev, Vladimir I Norkin, and Roger JB Wets. The minimization of semicontinuous functions: Mollifier subgradients. SIAM Journal on Control and Optimization, 33(1):149–167, 1995.
- [16] Roger Fletcher. A model algorithm for composite nondifferentiable optimization problems. Mathematical Programming Studies, 17:67–76, 1982.
- [17] Gerald B Folland. Real Analysis: Modern Techniques and Their Applications, volume 40. John Wiley & Sons, New York, 1999.
- [18] Allen A Goldstein. Optimization of Lipschitz continuous functions. Mathematical Programming, 13(1):14–22, 1977.
- [19] Adrian S Lewis and Stephen J Wright. A proximal method for composite minimization. Mathematical Programming, 158(1-2):501–546, 2016.
- [20] Hanyang Li and Ying Cui. A decomposition algorithm for two-stage stochastic programs with nonconvex recourse. SIAM Journal on Optimization, 34(1):306–335, 2024.
- [21] Junyi Liu, Ying Cui, Jong-Shi Pang, and Suvrajeet Sen. Two-stage stochastic programming with linearly bi-parameterized quadratic recourse. SIAM Journal on Optimization, 30(3):2530–2558, 2020.
- [22] Boris S Mordukhovich. Generalized differential calculus for nonsmooth and set-valued mappings. Journal of Mathematical Analysis and Applications, 183(1):250–288, 1994.
- [23] Matthew Norton, Valentyn Khokhlov, and Stan Uryasev. Calculating CVaR and bPOE for common probability distributions with application to portfolio optimization and density estimation. Annals of Operations Research, 299(1):1281–1315, 2021.
- [24] Giuseppe Paleologo and Samer Takriti. Bandwidth trading: A new market looking for help from the OR community. AIRO News, 6(3):1–4, 2001.
- [25] RA Poliquin and R Tyrrell Rockafellar. Amenable functions in optimization. Nonsmooth Optimization: Methods and Applications (Erice, 1991), pages 338–353, 1992.
- [26] RA Poliquin and R Tyrrell Rockafellar. A calculus of epi-derivatives applicable to optimization. Canadian Journal of Mathematics, 45(4):879–896, 1993.
- [27] R Tyrrell Rockafellar. Convex Analysis, volume 18. Princeton University Press, Princeton, NJ, 1970.
- [28] R Tyrrell Rockafellar. Lagrange multipliers and optimality. SIAM Review, 35(2):183–238, 1993.
- [29] R Tyrrell Rockafellar and Stanislav Uryasev. Optimization of conditional value-at-risk. Journal of Risk, 2(3):21–42, 2000.
- [30] R Tyrrell Rockafellar and Roger JB Wets. Variational Analysis, volume 317. Springer Science & Business Media, New York, 2009.
- [31] Johannes O Royset. Approximations of semicontinuous functions with applications to stochastic optimization and statistical estimation. Mathematical Programming, 184(1):289–318, 2020.
- [32] Johannes O Royset. Consistent approximations in composite optimization. Mathematical Programming, 201(1–2):339–372, 2022.
- [33] Alexander Shapiro, Darinka Dentcheva, and Andrzej Ruszczyński. Lectures on Stochastic Programming: Modeling and Theory (Third Edition). Society for Industrial and Applied Mathematics, Philadelphia, 2021.
- [34] Wim van Ackooij. A discussion of probability functions and constraints from a variational perspective. Set-Valued and Variational Analysis, 28(4):585–609, 2020.
- [35] Yao Yao, Qihang Lin, and Tianbao Yang. Large-scale optimization of partial AUC in a range of false positive rates. Advances in Neural Information Processing Systems, 35:31239–31253, 2022.
Appendix A. Proofs of Proposition 2 and Proposition 3.
Proof of Proposition 2..
(a) We first generalize the convergence result of the classical Moreau envelopes when (see, e.g., [30, Theorem 1.25]) to the partial Moreau envelopes. Fixing any , we consider the function with
Notice that . It is easy to verify that is proper and lsc based on our assumptions. Under the assumption that is bounded from below on , we can also show by contradiction that is level-bounded in locally uniformly in . Consequently, it follows from [30, Theorem 1.17] that for any fixed and each is lsc.
Hence, is a direct consequence of [30, Proposition 7.4(d)] by for all and the lower semicontinuity of . If , then is continuous, and thus by [30, Proposition 7.4(c-d)]. We then complete the proof of (a).
(b) For any ,
where follows from the convexity of for any and Danskin’s Theorem [11, Theorem 2.1]; is due to the optimality condition for , and is obtained by similar arguments in the proof of Theorem 1(b) due to our assumption that is bounded from below on ; and uses the outer semicontinuity of and at [20, Lemma 5]. Therefore, for any , . Moreover, due to the local boundedness of the mappings and at [20, Lemma 5], it follows from [30, Example 4.22] that . ∎
Proof of Proposition 3..
(a) Note that for any , is well-defined and takes finite value due to . Since follows a continuous distribution for any , we know from [29, Theorem 1] and [1] that CVaR has the following equivalent representations:
Moreover, is convex by the convexity of for any fixed (cf. [29, Theorem 2]). Therefore, both and defined in (7) are convex. By the definitions of and , we have
Note that is nondecreasing as a function of for any fixed . Namely,
Thus, for any and . Since as a function of on is left-continuous, it follows that for all . Observe that
Based on our assumptions and [34, Proposition 2.2], for any , the probability function is lsc, which implies the closedness of the level set for any . Hence, is lsc for any given and is continuous if is further assumed to be continuous. Then (a) is a direct consequence of [30, Proposition 7.4(c-d)] by the monotonicity and the continuity of .
(b) We use to denote the space of all random variables with . According to [33, Example 6.19], the function is subdifferentiable (see [33, (9.281)] for the definition). Consider any fixed . Given that is a continuous random variable in , it follows from [33, (6.81)] that the subdifferential of at is:
(43) |
We would like to mention that the event has zero probability and, thus, for every random variable . Let denote the probability measure associated with . By using [33, Theorem 6.14], we obtain the subdifferential of the convex function at :
(44) |
To see , it suffices to show that, for arbitrary two elements and in the set , we have
(45) |
To this end, we take any measurable selection . By the assumption that for all and , it holds that since subgradients of a convex function are uniformly bounded in norm by the Lipschitz constant. Consequently, both and are integrable as and for any by (43) and by our assumption. Observing that almost surely, we can conclude from [17, Proposition 2.23] that . This completes the proof of (45).
Next, we will explain why the closure can be removed in (44). By the convexity of for any fixed and the existence of a measurable function , it follows from [12, Theorem 2.7.2] that
where the right-hand-side is the subdifferential of a convex function and, thus, is a closed set. Then, we can omit the closure to obtain the equation in (44).
Now we use the expression of to characterize . For any , taking any and , we have
with
Since the event has zero probability, we have
By the definition of the approximate subdifferential, the proof is then completed. ∎
Appendix B. Proof of Proposition 4.
We start with the chain rules for and , where the inner function is merely lsc. These results are extensions of the nonlinear rescaling [30, Proposition 10.19(b)] to the case where may lack the strictly increasing property at a given point. One can also derive the same results through a general chain rule of the coderivative for composite set-valued mappings [22, Theorem 5.1]. However, to avoid the complicated computations accompanied by the introduction of the coderivative, we give an alternative proof below that is more straightforward. To prepare for the chain rules, we need a technical lemma about the proximal normal cone.
Lemma 3.
Let be a lsc function. For , it holds that
Proof of Lemma 3..
For . By [30, Example 6.16], we have
where is the projection operator. For any with , we have
which can be equivalently written as
Then restrict the feasible region of the above problem to a subset . Since is still a feasible point in this subset, we have . Hence, implies . Using this result and the expression of , we conclude that for . ∎
We present the chain rules with a self-contained proof in the following lemma.
Lemma 4 (chain rules for the limiting subdifferential).
Let be proper, lsc, convex, and nondecreasing with , and be lsc. Consider . If the only scalar with is , then
Proof of Lemma 4..
The basic idea is to rewrite as a parametric minimization problem and apply [30, Theorem 10.13]. Note that for . Define the corresponding set of optimal solutions as for any . Then, we have and for any . By our assumptions, it is obvious that for some . Based on our assumption that and is lsc, it is easy to verify that is proper, lsc, and level-bounded in locally uniformly in . Then we apply [30, Theorem 10.13] to obtain
(46) |
Case 1. If is a singleton , we can characterize by using the result in [30, Theorem 8.9]. Since and , it follows from our assumption that either or . Hence, based on the characterization of , (47) is satisfied.
Case 2. Otherwise, there exists such that since is lsc, nondecreasing and . Thus, from (46),
(48) |
Let and . In the following, we characterize and verify (47) separately for and .
Case 2.1. For any , we first prove the inclusion:
(49) |
Observe that for any , it holds that
(50) |
where the first inclusion is because any normal vector is a limit of proximal normals at nearby points [30, Exercise 6.18]; the second one uses Lemma 3; the last inclusion follows from the fact that the proximal normal cone is a subset of the limiting normal cone [30, Example 6.16]. Based on the the result of [30, Theorem 8.9] that
we conclude that for any . For any with , there exist , with . Then .
To prove (49), it remains to show that whenever . It follows from (50) that is a limit of proximal normals of at for some sequence . (i) First consider the case with . Following the argument in the proof of [30, Theorem 8.9], we can derive . Therefore,
where the first inclusion is due to the definition of the horizon subdifferential, and the last inclusion follows from a standard diagonal extraction procedure. (ii) In the other case, we have with and for all . It is easy to see . So far, we obtain inclusion (49). Since , we have , and our assumption implies that is the unique solution satisfying with . Combining this with (49), we immediately obtain (47).
Case 2.2. For any , consider any sequence converging to . Then for all sufficiently large since . It is easy to see that , which gives us due to [30, Exercise 6.18]. By following a similar pattern as the final part of Case 2.1, it is not difficult to obtain, for any ,
(51) |
In this case, (47) holds trivially. Hence, we have verified (47) for cases 1 and 2.
Step 2: Based on (47) in step 1, we can now apply the sum rule [30, corollary 10.9] for to obtain
(52) |
Equipped with the chain rules, we are now ready to prove Proposition 4.
Proof of Proposition 4..
Let be any feasible point, i.e., . Suppose for contradiction that (15) does not hold at . Thus, there exist , and an index set such that and for all . Take an arbitrary nonzero scalar for all . Let be any accumulation point of the unit scalars . Then, we have and , contradicting Assumption 5. This proves condition (15).
For any fixed , let for any in Assumption 5. Then the only scalar with is , which completes the proof of (16).
To derive the constraint qualification (17), we consider two cases.
Case 1. For , we have due to and for any by Theorem 1(a). Together with Assumption 5, we deduce that the only scalar with is . From this condition and the local Lipschitz continuity of for , we can apply the chain rule [30, Theorem 10.49] to get
(53) |
Case 2. For , to utilize the chain rules (Lemma 4) for , we must first confirm the validity of the condition:
(54) |
Indeed, it suffices to consider the case of or for some , because the statement holds trivially when is real-valued. For any element , there exist with and . Since , we must have for all sufficiently large k, i.e., , and is bounded from above due to or . The sequence is also bounded from below since is lsc as a consequence of . Then, we can assume that the bounded sequence converges to some . Note that due to . Thus, by the outer semicontinuity, . By , each can be expressed as the limit of a sequence with for any fixed . Using a standard diagonal extraction procedure, one can extract a subsequence with . Hence, and
(55) |
Using the subdifferentials relationships in Theorem 1 and the outer semicontinuity of , we have
(56) |
By (55), (56) and Assumption 5, we immediately get (54). Thus, we can apply the chain rule in Lemma 4, and use (55), (56) again to obtain
(57) |
For the last inclusion, we use by Theorem 1(a) and using a standard diagonal extraction procedure. Combining inclusions (53), (57) for two cases with Assumption 5, we derive (17) and complete the proof. ∎