Unsupervised Lexical Substitution with Decontextualised Embeddings
Abstract
We propose a new unsupervised method for lexical substitution using pre-trained language models. Compared to previous approaches that use the generative capability of language models to predict substitutes, our method retrieves substitutes based on the similarity of contextualised and decontextualised word embeddings, i.e. the average contextual representation of a word in multiple contexts. We conduct experiments in English and Italian, and show that our method substantially outperforms strong baselines and establishes a new state-of-the-art without any explicit supervision or fine-tuning. We further show that our method performs particularly well at predicting low-frequency substitutes, and also generates a diverse list of substitute candidates, reducing morphophonetic or morphosyntactic biases induced by article–noun agreement.111Code is available at: https://github.com/twadada/lexsub_decontextualised.
1 Introduction
There has been growing interest in developing automatic writing support systems to assist humans to write documents. One relevant task to this research goal is lexical substitution, where given a target word and its surrounding context, a system suggests a list of word substitutions that can replace the target word without changing its core meaning. For instance, given the target word great and the context He is a great artist, the model might suggest alternative words such as outstanding, terrific, or distinguished. Writers can use such suggestions to improve the fluency of their writing, reduce lexical repetition, or search for better expressions that represent their ideas more creatively.
As with other NLP tasks, recent studies have shown that masked language models such as BERT Devlin et al. (2019) perform very well on lexical substitution, even without any task-specific supervision. A common approach is to employ language models as generative models and predict substitutes based on their generative capability. However, this approach has some limitations. First, it is extremely difficult for language models to predict rare words — especially those that are segmented into multiple subword tokens — since the models inevitably assign them very low probabilities. Second, word prediction is highly affected by morphosyntactic constraints from the surrounding context, which overshadows the (arguably more important) question of semantic fit. For instance, if the target word is increase in the context … with an increase in …, language models tend to suggest words that also start with a vowel sound due to the presence of an before the target word, missing other possible substitutes such as hike or boost. In fact, this problem is even more pronounced in languages where words have grammatical gender (e.g. Italian nouns) or a high degree of inflection (e.g. Japanese verbs).
In this paper, we propose a new approach that explicitly deals with these limitations. Instead of generating words based on language model prediction, we propose to find synonymous words based on the similarity of contextualised and decontextualised word embeddings, where the latter refers to the “average” contextual representation of a word in multiple contexts. Experiments on English and Italian lexical substitution show that our fully unsupervised method outperforms previous models by a large margin. Furthermore, we show that our model performs particularly well at predicting low-frequency words, and also generates more diverse substitutes with less morphophonetic or morphosyntactic bias, e.g. as a result of article–noun agreement in English and Italian.
2 Method
2.1 Our Approach
Given a sentence that contains a target word and its surrounding context , we first feed the sentence into a pre-trained transformer model Vaswani et al. (2017) such as BERT and generate the contextualised representations of : , where denotes the layer of the model. We propose to predict substitutes of by retrieving words that have similar representations to . To this end, we calculate : the score of being a substitute of in context , as follows:
(1) | ||||
where denotes the decontextualised word embedding of ; is a set of selected layers; and denotes the cosine similarity between and . To obtain , we randomly sample sentences () that contain from a monolingual corpus, and take the average of the contextualised representations of given : . We pre-compute for each word in our pre-defined vocabulary , which consists of lexical items (i.e. no subwords) and contains different words from the pretrained model’s original vocabulary . If is segmented into multiple subwords (using the pretrained model’s tokeniser), we average its subword representations — this way we can include low-frequency words in and generate diverse substitutes. We obtain and by summing representations across different layers to capture various lexical information.222We also tried taking the weighted sum of the different-layer embeddings, but we did not see noticeable improvement.
2.2 Multi-Sense Embeddings
Representing in Eqn. (1) as a simple average of the contextualised representations of is clearly limited when has multiple meanings, since the representations will likely vary depending on its usage. For instance, Wiedemann et al. (2019) show that BERT representations of polysemous words such as bank create distinguishable clusters based on their usages. To address this issue, we first group the sentences into clusters based on the usages of , and for each cluster , we obtain the decontextualised embedding by averaging the contextualised representations, i.e.,
where denotes the set of the sentences that belong to the cluster . To obtain clusters, we apply -means Lloyd (1982); Arthur and Vassilvitskii (2007) to the L2-normalised representations of in sentences.333We concatenate across multiple layers . We expect that if has multiple senses, will to some degree capture the different meanings.444Note that the number of clusters is fixed across all words, forcing the model to “split” and “lump” senses Hanks (2012) to varying degrees. This methodology has been shown to be effective by Chronis and Erk (2020) on context-independent word similarity tasks. With , we can refine the similarity score in Eqn. (1) as follows:
In this way, we can compare with based on the sense that is most relevant to . Furthermore, we capture global similarity between and as:
(2) | |||
(3) |
where the second term in Eqn. (2) corresponds to the global similarity, which compares and outside of context .555To obtain , we compute the decontextualised embedding of and apply -means, as we do to compute . When is not included in our pre-defined vocabulary , we set to 1 and ignore the second term in Eqn. (2). However, it still considers in Eqn. (3) to retrieve the cluster that best represents the meaning of given .
While Eqn. (2) generally generates high-quality substitutes, we found that it sometimes retrieves words that share the same root word as and yet do not make good substitutes (e.g. pay and payer). This is mainly due to the fact that the vocabulary contains a large number of derivationally-related words, some of which are out-of-vocabulary (OOV) in the original vocabulary (e.g. pay ##er). To address this problem, we add a simple heuristic where is discarded if the normalised edit distance666The distance normalised by the maximum string length. between and is less than a threshold (0.5 for our English and Italian experiments).777We tuned this threshold based on English development data (i.e. the development split of SWORDS).
2.3 Reranking
In Eqn. (2), the context affects the representation of but not . Ideally, however, we want to consider the context on both sides to find the words that best fit the context. Therefore, we first generate top- candidates based on Eqn. (2), and rerank them using the following score:
(4) |
where denotes the contextualised representation of given , which can be obtained by replacing in with and feeding it into the model. In Eqn. (4), we calculate the similarity at each layer and take the average, which yields small yet consistent improvements over averaging the embeddings first and then calculating the similarity.888In Eqn. (1), we obtained similar results by averaging the embeddings or cosine similarities across layers. We limit the use of this scoring method to the candidates only, since it is computationally expensive to calculate for every single word in . Previously, a similar method was employed by Lee et al. (2021) but they used the last layer only (i.e. ). We show that using multiple layers substantially improves the performance. Following Lee et al. (2021), we set to 50.
2.4 Comparison to Previous Approaches
Our approach contrasts with previous approaches Zhou et al. (2019); Lee et al. (2021); Yang et al. (2022) that employ BERT as a generative model and predict lexical substitutes based on the generation probability :
(5) |
where denotes the output embedding of , which is usually tied with the input word embedding; is the representation at the very last layer of the model;999Note that this does not always correspond to the last layer of transformer: . E.g., BERT calculates by applying a feed forward network and layer normalisation to , whereas for XLNET, = .101010When consists of multiple subwords, the representation of the first or longest token is usually used. and is a scalar bias. While this approach is straightforward and well motivated, its predictions are highly influenced by morphosyntax, as discussed in Section 1. Moreover, Eqn. (5) shows three additional limitations compared to our approach: (1) the prediction is conditioned on the last layer only, despite previous studies showing that different layers capture different information, with the last layer usually containing less semantic information than the lower or middle layers Bommasani et al. (2020); Tenney et al. (2019); (2) is represented by the single vector , which may not work well when has multiple meanings — we alleviate this by clustering the embeddings (Section 2.2); and (3) the model is not capable of generating OOV words, unless we force the model to decode multiple subwords, e.g. by using multiple mask tokens or duplicating . Our approach, in contrast, can include rare words in the pre-defined vocabulary and generate diverse substitutes (Section 4.4).
3 Experiments
3.1 Data and Evaluation
We conduct experiments in two evaluation settings: generation and ranking. In the generation setting, systems produce lexical substitutes given target words and sentences, while in the ranking setting, they are also given substitute candidates and rank them based on their appropriateness.
For the generation task, we base our experiments on SWORDS Lee et al. (2021), the largest English lexical substitution dataset, which extends and improves CoInCo Kremer et al. (2014) by introducing a new annotation scheme: in CoInCo, the annotators were asked to come up with substitutes by themselves, whereas in SWORDS, the annotators were given substitute candidates pre-retrieved from a thesaurus, and only had to made binary judgements (“good” or “bad”).111111The annotators were asked if they would consider using the substitute candidate to replace the target word as the author of the context. A word is regarded as acceptable if it is judged to be good by more than five out of ten annotators, and conceivable if selected by at least one annotator. In this way, SWORDS provides more comprehensive lists of substitutes, including many low-frequency words that are good substitutes and yet difficult for humans to suggest — these words are of particular interest to us. For the evaluation metrics, the authors use the harmonic mean of the precision and recall given the gold and top-10 system-generated substitutes.121212More precisely, their evaluation script lemmatises the top-50 substitutes first and then extracts the top-10 distinct words. As gold substitutes, they use either the acceptable or conceivable words, and calculate the corresponding scores and , respectively. They also propose to measure those scores in both strict and lenient settings, which differ in that in the lenient setting, candidate words that are not scored under SWORDS are filtered out and discarded.
In the ranking task, we evaluate models on the traditional SemEval-2007 Task 10 (“SemEval-07”) data set (trial+test) McCarthy and Navigli (2007), as well as SWORDS. For the evaluation metric, we follow previous work in using Generalized Average Precision (GAP; Kishida (2005)):
(6) |
where and denote the gold weight of the -th item in the predicted and gold ranked lists respectively, with and indicating their sizes; is a binary function that returns if , and otherwise; and is the average weight of the gold ranked list from the 1st to the -th items. In our task, the weight corresponds to the aptness of the substitute, which is set to zero if it is not in the gold substitutes. Following previous work Melamud et al. (2015); Arefyev et al. (2020), we ignore multiword expressions in SemEval-07.131313We run the evaluation code at https://github.com/orenmel/lexsub with the no-mwe option.
3.2 Models
As shown in Eqn. (2), our approach requires only the vector representations of words and hence is applicable to any text encoder model. Therefore, we test our method with various pre-trained models, including five masked language models: BERT Devlin et al. (2019), mBERT, SpanBERT Joshi et al. (2020), XLNET Yang et al. (2019), and MPNet Song et al. (2020); one encoder-decoder model: BART Lewis et al. (2020); and two discriminative models: ELECTRA Clark et al. (2020) and DeBERTa-V3 He et al. (2021).141414See Appendix A for the details of all models. We also evaluate two sentence-embedding models: MPNet-based sentence transformer Reimers and Gurevych (2019) and SimCSE Gao et al. (2021), both of which are fine-tuned on semantic downstream tasks such as MNLI and achieve good performance on sentence-level tasks. Finally, we also evaluate the encoder of the fine-tuned mBART on English-to-Many translation Tang et al. (2021). Note that the discriminative models and embedding models (e.g. NMT-encoder, SimCSE) cannot generate words and hence are incompatible with the previous approach described in Section 2.4.
Models Lenient Strict HUMANS 48.8 77.9 – – CoInCo 34.1 63.6 – – GPT-3 34.6 49.0 22.7 36.3 BERT-Kfootnote 15 32.4 55.4 19.2 30.4 (w/o rerank)151515These results differ slightly from the reported scores in Lee et al. (2021), due to a bug in their code. 31.8 54.9 15.7 24.4 BERT-M 30.9 48.3 16.2 25.4 (w/o rerank) 30.9 48.1 10.7 16.5 Zhou et al. (2019)footnote 15 32.0 55.4 17.4 27.5 Yang et al. (2022)161616Updated from the original scores by the authors after they fixed some critical issues in their evaluation setup. 31.9 54.9 16.7 28.4 OURS BERT 33.2 64.1 21.1 34.9 (w/o rerank) 33.0 63.8 20.7 34.4 (w/o rerank, heuristic) 33.6 64.0 20.2 32.4 mBERT 27.0 52.7 12.4 22.6 SpanBERT 32.6 61.4 20.9 34.0 MPNet 33.8 63.8 22.0 34.1 XLNet 34.4 65.3 23.3 37.4 ELECTRA 33.5 64.2 23.2 36.7 DeBERTa-V3 33.6 65.8 24.5 39.9 BART (Enc) 33.6 62.8 21.9 34.8 BART (Dec) 33.5 60.5 21.4 34.0 BART (Enc-Dec) 33.7 64.9 23.5 37.2 SBERT (MPNet) 34.6 64.0 21.8 33.5 SimCSE (BERT) 33.4 64.3 21.6 35.7 NMT (mBART) 28.7 55.6 13.4 22.2 OURS (Rank Candidates) XLNet 35.2 72.9 – – BART 34.8 72.4 – – DeBERTa-V3 35.1 72.2 – –
We use the same vocabulary for all models, which consists of the 30,000 most common words171717We discard tokens that contain numerals, punctuation, or capital letters. As such, includes more lexical items (with less noise and no subwords) than the original vocabulary . in the OSCAR corpus Ortiz Suárez et al. (2020). We set the number of sentences we sample from OSCAR to calculate the decontextualised embeddings, i.e. , to 300; the clustering size to 4; and in Eqn. (2) to 0.7. For the set of transformer layers, , we employ all layers except for the first and last two, i.e. {3, 4, …, }. We tune all hyper-parameters on the development split of SWORDS.
3.3 Results
Table 1 shows the results on SWORDS, along with baseline scores from previous work (with some bug fixes, as noted). The first row, HUMANS, indicates the agreement of two independent sets of annotators on (a subset of) SWORDS, and approximates the upper bound for this task. The second row, CoInCo, shows the accuracy of the gold standard substitutes in CoInCo, which are suggested by human annotators without access to substitution candidates181818Since all of these words are in the substitute candidates of SWORDS, it cannot be evaluated under the strict setting. — this approximates how well humans perform when asked to elicit candidates themselves. The remainder of the rows above OURS denote baseline systems, all of which employ generative approaches. The first baseline uses GPT-3 Brown et al. (2020), and achieves the state-of-the-art in the strict setting. It generates substitutes based on “in-context learning”, where the model first reads several triplets of target sentences, queries, and gold-standard substitutes retrieved from the development set, and then performs on-the-fly inference on the test set. As such, it is not exactly comparable to the other fully unsupervised models. BERT-K generates substitutes based on Eqn. (5) by feeding the target sentence into BERT, and BERT-M works the same except that the target word is replaced by [MASK]. Both models further rerank the candidates based on Eqn. (4), using the last layer only; we show the performance without reranking as “w/o rerank” in Table 1. Yang et al. (2022) and Zhou et al. (2019) also use BERT to generate substitutes, and rerank them using their own method.
The rows below OURS indicate the performance of our approach using various off-the-shelf models. Our method with BERT substantially outperforms all the BERT-based baselines, even without the edit-distance heuristic (Section 2.2) or reranking method (Eqn. (4)). The best performing models are DeBERTa-V3, XLNet, and BART (Enc-Dec), all of which outperform the weakly-supervised GPT-3 model by a large margin in the strict setting; and XLNet even outperforms CoInCo in the lenient setting. The last three rows show the performance of the top-3 models when they are given the candidate words and rank them based on Eqn. (4), which emulates how the SWORDS annotators judged the words. The result shows that all the models still lag behind HUMANS, suggesting there is still substantial room for improvement. Interestingly, BART performs best when we average the scores obtained by its encoder and decoder, suggesting each layer captures complementary information. It is also intriguing to see that the discriminative models (DeBERTa-V3 and ELECTRA) perform much better than BERT, albeit they are not trained to generate words and not compatible with the previous generative approach. The sentence embedding models (SBERT, SimCSE) perform no better than the original models, which contrasts with their strong performance in sentence-level tasks. The multilingual models (mBERT, NMT) perform very poorly, even though the NMT model was fine-tuned on large English-X parallel corpora.
Models S-07 SW HUMANS — 66.2 Arefyev et al. (2020) (XLNet) 61.3191919The original score reported by Arefyev et al. (2020) is 59.6, but we found we could improve this result by appending unscored OOV words to the ranked list in random order. — Michalopoulos et al. (2022) (LMs+WN) 60.3 — Lacerra et al. (2021a) (BERT) 58.2 — Lacerra et al. (2021a) (BERT, sup) 60.5 — Zhou et al. (2019) (BERT) 60.5202020Similar to Lacerra et al. (2021a) and Arefyev et al. (2020), we were unable to reproduce this score. 53.5footnote 15 Lee et al. (2021) (BERT) 56.6 56.9 OURS (Eqn. (4)) BERT 58.6 60.7 mBERT 45.4 52.0 SpanBERT 59.3 60.8 MPNet 61.5 59.5 XLNet 63.8 62.9 ELECTRA 64.4 62.3 DeBERTa-V3 65.0 62.9 BART (Enc) 62.9 61.9 BART (Dec) 62.6 60.8 BART (Enc-Dec) 64.1 62.7 SBERT (MPNet) 61.0 62.5 SimCSE (BERT) 58.4 60.9 NMT (mBART, Enc) 46.0 51.5
Table 2 shows the results for the ranking task on SemEval-07 and SWORDS. Michalopoulos et al. (2022) harness WordNet Fellbaum (1998) to obtain synsets of the target word and also their glosses, and employ BERT and RoBERTa Liu et al. (2019) to rank candidates. The models proposed by Lacerra et al. (2021a) are different from the others in that they fine-tune BERT on lexical substitution data sets. They propose unsupervised (BERT) and supervised (BERT, sup) models, which are fine-tuned on automatically-generated or manually-annotated data. Table 2 shows that our method with BERT performs comparably with the unsupervised model of Lacerra et al. (2021a) without any fine-tuning, and outperforms Zhou et al. (2019) and Lee et al. (2021) (except for the score of Zhou et al. (2019) on SemEval-07, which couldn’t be reproduced in previous work). Just like the generation task, DeBERTa-V3 achieves the best performance on both data sets and establishes a new state-of-the-art. Other models also follow a similar trend to the generation results, e.g. BART performs best by combining its encoder and decoder, and the multilingual models perform very poorly. We hypothesise that their poor performance is mainly caused by suboptimal segmentation of English words. This hypothesis is also supported by the fact that DeBERTa-V3 has by far the largest vocabulary of all models.212121Note that differs from , the pre-defined vocabulary we used for all models. Appendix A compares the size of the model’s original vocabulary across different models.
3.4 Results on Italian Lexical Substitution
We further conduct an additional experiment on Italian, based on the data set from the EVALITA 2009 workshop Toral (2009). We report scores given top-10 predictions as in the English generation task, plus two traditional metrics used in the workshop, namely oot and best, which compare the top-10 and top-1 predictions against the gold substitutes.222222We report precision only, as it is the same as recall under those metrics when predictions are made for every sentence. We lemmatise all the generated words to make them match the gold substitutes, following the SWORDS evaluation script.232323We used the Italian lemmatiser (it_core_news_sm 3.2.0) in spaCy (ver. 3.2.2) Honnibal et al. (2020). We use the same hyper-parameters as for the English experiments, and Table 3 shows the results. Hintz and Biemann (2016) is a strong baseline that retrieves substitute candidates from MultiWordNet Pianta et al. (2002) and ranks them using a supervised ranker model. We also implement BERT-K using an Italian BERT model Schweter (2020), with and without the reranking method. The results show that our approach substantially outperforms the baselines, confirming its effectiveness. However, our reranking method is not as effective as in English, which we attribute to the influence of grammatical gender in Italian (which we return to in Section 4.2). The heuristic improves best-P but harms and oot-P, meaning it removes good candidates as well as bad ones, possibly because we used the threshold tuned on English.
best-P oot-P Hintz and Biemann (2016) — 16.2 41.3 BERT-K 14.3 14.4 39.1 (w/o rerank) 15.6 17.4 43.3 OURS (BERT) 17.3 19.9 47.5 (w/o rerank) 17.5 19.1 48.4 (w/o rerank, heuristic) 17.5 17.4 48.7 OURS (ELECTRA) 19.0 21.0 51.2 (w/o rerank) 18.9 21.3 51.0 (w/o rerank, heuristic) 19.2 20.2 52.1
BERT BART XLNet DeBERTa 34.1 26.2 32.9 35.8 32.8 34.1 34.1 35.6 32.9 32.0 33.7 35.7 is random 30.6 29.2 30.3 32.0 w/o heuristic 32.4 32.0 33.4 35.7 34.4 34.0 35.0 36.9 + rerank 34.9 37.2 37.4 39.9
4 Analysis
4.1 Ablation Studies
We perform ablation studies on SWORDS to see the effect of and the -clustered embeddings, and also the heuristic based on edit distance. Table 4 shows the results. Overall, our method with performs better than or , confirming the benefit of considering both in-context and out-of-context similarities. One interesting observation is that while BERT and DeBERTa perform better with than with , the opposite trend is observed for XLNet and especially BART (and hence the optimal value for is smaller than 0.7). This suggests that BART representations are highly influenced by context, containing much information that is not relevant to the semantics of the target word; we further confirm this in the next section. When we set the cluster size to 1, the performance of all the models drops sharply, indicating the effectiveness of the clustered embeddings. When we retrieve the cluster of at random instead of the closest one to in Eqn. (2), the performance decreases substantially, suggesting each cluster captures different semantics. The heuristic consistently improves the performance, filtering out derivationally-related yet semantically-dissimilar words to the target word. Lastly, our reranking method substantially improves the performance of all the models, demonstrating that it is important to incorporate the target context into both the target and candidate word representations.
4.2 Effects of Morphosyntactic Agreement
a an un una la/le il/i BERT-K 94.2 56.0 93.3 92.5 93.8 92.0 (w/o r) 91.1 54.0 90.0 87.5 90.3 87.4 OURS (BERT) 88.4 24.0 81.9 89.2 92.6 85.2 (w/o r) 86.8 18.0 70.5 66.7 69.7 64.6 (w/o r, h) 86.8 44.0 62.4 65.0 69.4 60.7 Gold 86.8 26.9 55.6 63.3 69.0 67.0
Compared to previous generative approaches, our method does not depend on the generation probabilities of language models, and hence we expect it to be less sensitive to morphosyntactic agreement effects. To investigate this, we analyse the performance on noun target words which immediately follow one of the following articles: a or an in English, and una, la, le, un, il or i in Italian. The first three Italian articles are used with feminine nouns, and the rest with masculine ones. Our hypothesis is that generative methods will be highly biased by these articles, despite the gold standard being semantically annotated, and thus largely oblivious to local morphosyntactic agreement effects.
Table 5 shows the percentage of top-10 predicted candidates that agree with the article.242424We retrieve Italian gender information using a dictionary API (https://github.com/sphoneix22/italian_dictionary), and English phonetic information using CMUdict (https://github.com/cmusphinx/cmudict) accessed via NLTK Steven et al. (2009). It demonstrates that the prediction of BERT-K is highly affected by the proceeding article as expected, resulting in substitutes which don’t satisfy this constraint being assigned low probabilities. In contrast, the results of our method are more balanced and close to the gold standard.252525Note that the big jump in results for an is based on a small number of instances (5 sentences). Conversely, our reranking method actually increases the bias greatly, suggesting that the contextualised embeddings and in Eqn. (4) become similar when and collocate similarly with the words in the context — overall, this leads to better results, but actually hurts in cases of local agreement effects biasing the results. This is one reason why reranking was not as effective in Italian as in English, as agreement effects are stronger in Italian.
Models a an BERT-K 94.2 56.0 BERT-M 99.5 88.0 BERT 88.4 24.0 SpanBERT 97.9 46.0 MPNet 91.6 52.0 XLNet 83.2 38.0 BART (Enc) 89.5 62.0 BART (Dec) 91.1 46.0 BART (Enc-Dec) 89.5 58.0 DeBERTa-V3 86.8 32.0 ELECTRA 88.4 36.0
Context The loan may be extended by the McAlpine group for an additional year with an increase in the conversion price to $2.50 a share. Gold (Conceivable) boost, gain, raise, hike, rise, swell, surge, upsurge, enlargement, growth, addition, escalation, expansion, upgrade, cumulation, swelling, exaggeration, step-up BERT-K increased, rise, enhancement, increasing, addition OURS (BERT) rise, raise, change, reduce, reduction OURS (BART) rise, uptick, hike, improvement, upping OURS (DeBERTa-V3) boost, rise, raise, hike, reduction Context Under an accord signed yesterday, the government and Union Bank of Finland would become major shareholders in the new company, each injecting 100 million Finnish markkaa ($23.5 million). Gold (Conceivable) arrangement, agreement, pact, contract, deal, treaty BERT-K understanding, agreement, pact, arrangement, agreeing OURS (BERT) agreement, pact, treaty, understanding, deal OURS (BART) agreement, deal, pact, arrangement, treaty OURS (DeBERTa-V3) pact, agreement, deal, arrangement, treaty
Table 6 shows the result when we use different pre-trained models in English. First, it shows that BERT-M is more sensitive to the articles than BERT-K, indicating the strong morphophonetic agreement effect on the masked word prediction. Among the pre-trained language models used by our method, SpanBERT and BART are the most sensitive to the article a and an, respectively. This suggests that the embeddings obtained from these models are highly sensitive to the context , partly explaining why BART performs very poorly with = 1, as shown in Section 4.1. Lastly, Table 7 shows examples of predicted substitutes when the article an comes before the target word. It shows that BERT-K and OURS with BART tend to retrieve words that start with a vowel sound, as quantitatively described in Table 6.
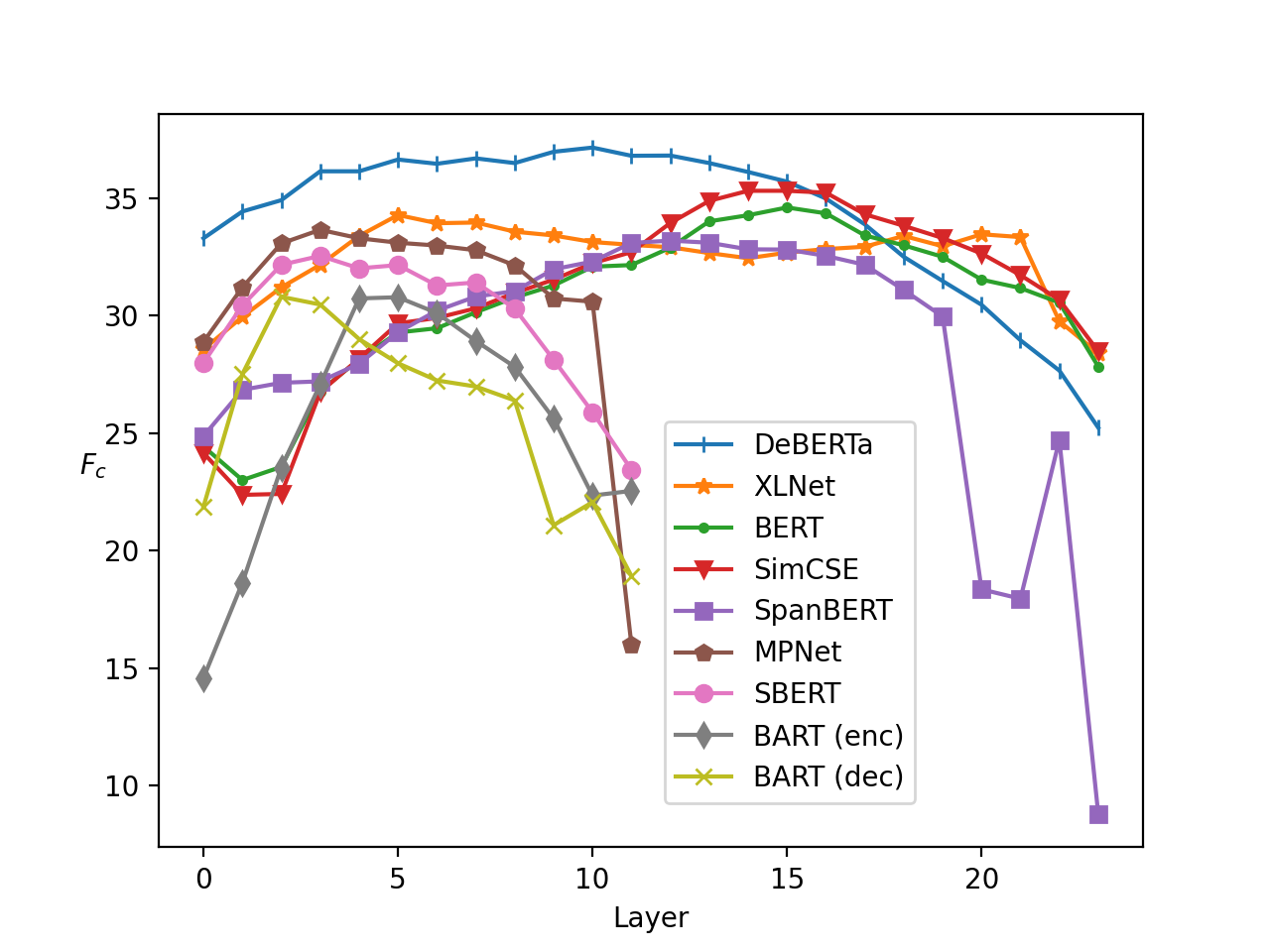
4.3 Layer-Wise Performance
We analyse the performance on SWORDS using different layers in Figure 1 (w/o rerank).262626We perform qualitative analysis in Appendix D. First, we clearly see that middle layers perform better than the first or last ones, for all models.272727We see a similar trend in the ranking task (Appendix B). The performance of BERT peaks at layer 16, in contrast with previous findings that the first quarter of layers perform best on context-independent word similarity tasks Bommasani et al. (2020), likely because lexical substitution critically relies on context.282828In fact, Tenney et al. (2019) show that high-level semantic information is encoded in higher layers. Our method using multiple layers performs mostly as well as using the best layer without the need to perform model-wise layer selection (see Table 11 in Appendix C). The last layer performs very poorly for all models, highlighting the limitation of the previous approach which uses the last layer only (Eqn. (5)). The downward trend is particularly evident for MPNet, BART (dec), and SpanBERT; for BART and SpanBERT, we attribute this to the fact that their last-layer representations of the word at position are used to predict the next word , or neighbouring words {..,} or {..,}.292929SpanBERT does this for Span Boundary Objective. This training objective may also lead to their sensitivity to articles before the target word, as shown in Section 4.2. Interestingly, the sentence-embedding models (SBERT and SimCSE) are no exception to the downward trend, which is somewhat counter-intuitive given that their last layer representations are fine-tuned (and used during inference) to perform semantic downstream tasks. Importantly, they do not perform better than the original models (MPNet and BERT), although in the ranking task, both models benefit moderately from fine-tuning (see Appendix B).
# Matched Words low med high BERT-K 121 144 2002 30.4 BERT (5k) 31 52 2017 28.1 BERT (10k) 72 111 2249 32.6 BERT (20k) 164 220 2194 34.5 BERT (30k) 241 267 2095 34.9 BART (30k) 380 274 2123 37.2 XLNet (30k) 395 292 2106 37.4 DeBERTa (30k) 429 287 2262 39.9
1 2 4 8 16 22.8 23.1 23.2 23.3 23.4 36.0 36.7 36.7 36.9 36.7
4.4 Analysis of Word Frequency
One of the strengths of our approach is that it can generate low-frequency substitutes that are OOV words in the original vocabulary. To confirm this, we analyse how well our method can generate low-frequency words from different vocabulary sizes . Table 8 shows the results, in which we experiment with our BERT-based model with the vocabulary sizes of 5k, 10k, 20k, and 30k. The columns under “# Matched Words” show the numbers of correctly-predicted words, grouped by frequency range: low, med, and high denote words with frequency 50k, 50k–100k, and 100k in a large web corpus. The table shows that our method with 30k words generates nearly twice as many low-frequency substitutes as the baseline. Our method with 10k words still outperforms BERT-K in , demonstrating its effectiveness. The last three rows show the performance of our method using other models, further demonstrating its ability to predict low-frequency words.
4.5 Effects of Cluster Size
Finally, we analyse the effect of the cluster size for ELECTRA, as shown in Table 9. While a larger cluster size yields better performance, the improvement is marginal. Rather than using a fixed , in future work we are interested in dynamically selecting the number of clusters per word.
5 Related Work
In the pre-BERT era, most lexical substitution methods employed linguistic resources such as WordNet Fellbaum (1998) to obtain substitute candidates Szarvas et al. (2013); Hintz and Biemann (2016). However, recent studies have shown that pre-trained language models such as BERT outperform these models without any external lexical resources. For instance, Zhou et al. (2019) feed a target sentence into BERT while partially masking the target word using dropout Srivastava et al. (2014), and generate substitutes based on the probability distribution at the target word position. The masking strategy was shown to be effective on SemEval-07 but not on SWORDS. Similarly, Yang et al. (2022) feed two sentences into BERT, concatenating the target sentence with itself but with the target word replaced by [MASK], and predict words based on the mask-filling probability. Michalopoulos et al. (2022) augment pre-trained language models with WordNet and outperform Zhou et al. (2019). Lacerra et al. (2021a) fine-tune BERT on lexical substitution data sets that are automatically generated using BERT. They show that this approach is effective at ranking, and that adding manually-annotated data further boosts performance. Lacerra et al. (2021b) fine-tune BART on human-annotated data, and make it generate a list of substitutes given a target sentence in an end-to-end manner. They show that this generative approach rivals Zhou et al. (2019). Note that all of these recent models are evaluated on English only.
6 Conclusion
We present a new unsupervised approach to lexical substitution using pre-trained language models. We showed that our method substantially outperforms previous methods on English and Italian data sets, establishing a new state-of-the-art. By comparing performance on lexical substitution using different layers, we found that middle layers perform better than first or last layers. We also compared the substitutes predicted by the previous generative approach and our method, and showed that our approach works better at predicting low-frequency substitutes and reduces morphophonetic or morphosyntactic biases induced by article–noun agreement in English and Italian.
References
- Arefyev et al. (2020) Nikolay Arefyev, Boris Sheludko, Alexander Podolskiy, and Alexander Panchenko. 2020. Always keep your target in mind: Studying semantics and improving performance of neural lexical substitution. In Proceedings of the 28th International Conference on Computational Linguistics, pages 1242–1255, Barcelona, Spain (Online). International Committee on Computational Linguistics.
- Arthur and Vassilvitskii (2007) David Arthur and Sergei Vassilvitskii. 2007. K-means++: The advantages of careful seeding. In Proceedings of the Eighteenth Annual ACM-SIAM Symposium on Discrete Algorithms, SODA ’07, page 1027–1035, USA. Society for Industrial and Applied Mathematics.
- Bommasani et al. (2020) Rishi Bommasani, Kelly Davis, and Claire Cardie. 2020. Interpreting pretrained contextualized representations via reductions to static embeddings. In Proceedings of the 58th Annual Meeting of the Association for Computational Linguistics, pages 4758–4781, Online. Association for Computational Linguistics.
- Brown et al. (2020) Tom Brown, Benjamin Mann, Nick Ryder, Melanie Subbiah, Jared D Kaplan, Prafulla Dhariwal, Arvind Neelakantan, Pranav Shyam, Girish Sastry, Amanda Askell, Sandhini Agarwal, Ariel Herbert-Voss, Gretchen Krueger, Tom Henighan, Rewon Child, Aditya Ramesh, Daniel Ziegler, Jeffrey Wu, Clemens Winter, Chris Hesse, Mark Chen, Eric Sigler, Mateusz Litwin, Scott Gray, Benjamin Chess, Jack Clark, Christopher Berner, Sam McCandlish, Alec Radford, Ilya Sutskever, and Dario Amodei. 2020. Language models are few-shot learners. In Advances in Neural Information Processing Systems, volume 33, pages 1877–1901. Curran Associates, Inc.
- Chronis and Erk (2020) Gabriella Chronis and Katrin Erk. 2020. When is a bishop not like a rook? When it’s like a rabbi! Multi-prototype BERT embeddings for estimating semantic relationships. In Proceedings of the 24th Conference on Computational Natural Language Learning, pages 227–244, Online. Association for Computational Linguistics.
- Clark et al. (2020) Kevin Clark, Minh-Thang Luong, Quoc V. Le, and Christopher D. Manning. 2020. ELECTRA: Pre-training text encoders as discriminators rather than generators. In International Conference on Learning Representations.
- Devlin et al. (2019) Jacob Devlin, Ming-Wei Chang, Kenton Lee, and Kristina Toutanova. 2019. BERT: Pre-training of deep bidirectional transformers for language understanding. In Proceedings of the 2019 Conference of the North American Chapter of the Association for Computational Linguistics: Human Language Technologies, Volume 1 (Long and Short Papers), pages 4171–4186, Minneapolis, Minnesota. Association for Computational Linguistics.
- Fellbaum (1998) Christiane Fellbaum. 1998. WordNet: An Electronic Lexical Database. Bradford Books.
- Gao et al. (2021) Tianyu Gao, Xingcheng Yao, and Danqi Chen. 2021. SimCSE: Simple contrastive learning of sentence embeddings. In Proceedings of the 2021 Conference on Empirical Methods in Natural Language Processing, pages 6894–6910, Online and Punta Cana, Dominican Republic. Association for Computational Linguistics.
- Hanks (2012) Patrick Hanks. 2012. The Corpus Revolution in Lexicography. International Journal of Lexicography, 25(4):398–436.
- He et al. (2021) Pengcheng He, Jianfeng Gao, and Weizhu Chen. 2021. DeBERTaV3: Improving DeBERTa using ELECTRA-style pre-training with gradient-disentangled embedding sharing. CoRR, abs/2111.09543.
- Hintz and Biemann (2016) Gerold Hintz and Chris Biemann. 2016. Language transfer learning for supervised lexical substitution. In Proceedings of the 54th Annual Meeting of the Association for Computational Linguistics (Volume 1: Long Papers), pages 118–129, Berlin, Germany. Association for Computational Linguistics.
- Honnibal et al. (2020) Matthew Honnibal, Ines Montani, Sofie Van Landeghem, and Adriane Boyd. 2020. spaCy: Industrial-strength natural language processing in Python.
- Joshi et al. (2020) Mandar Joshi, Danqi Chen, Yinhan Liu, Daniel S. Weld, Luke Zettlemoyer, and Omer Levy. 2020. SpanBERT: Improving pre-training by representing and predicting spans. Transactions of the Association for Computational Linguistics, 8:64–77.
- Kishida (2005) Kazuaki Kishida. 2005. Property of average precision and its generalization: An examination of evaluation indicator for information retrieval experiments. NII Technical Report.
- Kremer et al. (2014) Gerhard Kremer, Katrin Erk, Sebastian Padó, and Stefan Thater. 2014. What substitutes tell us — analysis of an “all-words” lexical substitution corpus. In Proceedings of the 14th Conference of the European Chapter of the Association for Computational Linguistics, pages 540–549, Gothenburg, Sweden. Association for Computational Linguistics.
- Lacerra et al. (2021a) Caterina Lacerra, Tommaso Pasini, Rocco Tripodi, and Roberto Navigli. 2021a. ALaSca: an automated approach for large-scale lexical substitution. In Proceedings of the 30th International Joint Conference on Artificial Intelligence. International Joint Conferences on Artificial Intelligence.
- Lacerra et al. (2021b) Caterina Lacerra, Rocco Tripodi, and Roberto Navigli. 2021b. GeneSis: A generative approach to substitutes in context. In Proceedings of the 2021 Conference on Empirical Methods in Natural Language Processing, pages 10810–10823, Online and Punta Cana, Dominican Republic. Association for Computational Linguistics.
- Lee et al. (2021) Mina Lee, Chris Donahue, Robin Jia, Alexander Iyabor, and Percy Liang. 2021. Swords: A benchmark for lexical substitution with improved data coverage and quality. In Proceedings of the 2021 Conference of the North American Chapter of the Association for Computational Linguistics: Human Language Technologies, pages 4362–4379, Online. Association for Computational Linguistics.
- Lewis et al. (2020) Mike Lewis, Yinhan Liu, Naman Goyal, Marjan Ghazvininejad, Abdelrahman Mohamed, Omer Levy, Veselin Stoyanov, and Luke Zettlemoyer. 2020. BART: Denoising sequence-to-sequence pre-training for natural language generation, translation, and comprehension. In Proceedings of the 58th Annual Meeting of the Association for Computational Linguistics, pages 7871–7880, Online. Association for Computational Linguistics.
- Liu et al. (2019) Yinhan Liu, Myle Ott, Naman Goyal, Jingfei Du, Mandar Joshi, Danqi Chen, Omer Levy, Mike Lewis, Luke Zettlemoyer, and Veselin Stoyanov. 2019. RoBERTa: A robustly optimized BERT pretraining approach. CoRR, abs/1907.11692.
- Lloyd (1982) S. Lloyd. 1982. Least squares quantization in pcm. IEEE Transactions on Information Theory, 28(2):129–137.
- McCarthy and Navigli (2007) Diana McCarthy and Roberto Navigli. 2007. SemEval-2007 task 10: English lexical substitution task. In Proceedings of the 4th International Workshop on Semantic Evaluations, SemEval ’07, page 48–53, USA. Association for Computational Linguistics.
- Melamud et al. (2015) Oren Melamud, Omer Levy, and Ido Dagan. 2015. A simple word embedding model for lexical substitution. In Proceedings of the 1st Workshop on Vector Space Modeling for Natural Language Processing, pages 1–7, Denver, Colorado. Association for Computational Linguistics.
- Michalopoulos et al. (2022) George Michalopoulos, Ian McKillop, Alexander Wong, and Helen Chen. 2022. LexSubCon: Integrating knowledge from lexical resources into contextual embeddings for lexical substitution. In Proceedings of the 60th Annual Meeting of the Association for Computational Linguistics (Volume 1: Long Papers), pages 1226–1236, Dublin, Ireland. Association for Computational Linguistics.
- Ortiz Suárez et al. (2020) Pedro Javier Ortiz Suárez, Laurent Romary, and Benoît Sagot. 2020. A monolingual approach to contextualized word embeddings for mid-resource languages. In Proceedings of the 58th Annual Meeting of the Association for Computational Linguistics, pages 1703–1714, Online. Association for Computational Linguistics.
- Pianta et al. (2002) Emanuele Pianta, Luisa Bentivogli, and Christian Girardi. 2002. MultiWordNet: Developing an aligned multilingual database. In Proceedings of the First International Conference on Global WordNet, pages 293–302, Mysore, India.
- Reimers and Gurevych (2019) Nils Reimers and Iryna Gurevych. 2019. Sentence-BERT: Sentence embeddings using Siamese BERT-Networks. In Proceedings of the 2019 Conference on Empirical Methods in Natural Language Processing. Association for Computational Linguistics.
- Schweter (2020) Stefan Schweter. 2020. Italian BERT and ELECTRA models. https://doi.org/10.5281/zenodo.4263142.
- Song et al. (2020) Kaitao Song, Xu Tan, Tao Qin, Jianfeng Lu, and Tie-Yan Liu. 2020. MPNet: Masked and permuted pre-training for language understanding. In Advances in Neural Information Processing Systems, volume 33, pages 16857–16867.
- Srivastava et al. (2014) Nitish Srivastava, Geoffrey Hinton, Alex Krizhevsky, Ilya Sutskever, and Ruslan Salakhutdinov. 2014. Dropout: A simple way to prevent neural networks from overfitting. Journal of Machine Learning Research, 15:1929–1958.
- Steven et al. (2009) Bird Steven, Edward Loper, and Ewan Klein. 2009. Natural Language Processing with Python. O’Reilly Media Inc.
- Szarvas et al. (2013) György Szarvas, Chris Biemann, and Iryna Gurevych. 2013. Supervised all-words lexical substitution using delexicalized features. In Proceedings of the 2013 Conference of the North American Chapter of the Association for Computational Linguistics: Human Language Technologies, pages 1131–1141, Atlanta, Georgia. Association for Computational Linguistics.
- Tang et al. (2021) Yuqing Tang, Chau Tran, Xian Li, Peng-Jen Chen, Naman Goyal, Vishrav Chaudhary, Jiatao Gu, and Angela Fan. 2021. Multilingual translation from denoising pre-training. In Findings of the Association for Computational Linguistics: ACL-IJCNLP 2021, pages 3450–3466, Online. Association for Computational Linguistics.
- Tenney et al. (2019) Ian Tenney, Dipanjan Das, and Ellie Pavlick. 2019. BERT rediscovers the classical NLP pipeline. In Proceedings of the 57th Annual Meeting of the Association for Computational Linguistics, pages 4593–4601, Florence, Italy. Association for Computational Linguistics.
- Toral (2009) Antonio Toral. 2009. The lexical substitution task at EVALITA 2009. In EVALITA workshop, 11th Congress of Italian Association for Artificial Intelligence, Reggio Emilia, Italy.
- Vaswani et al. (2017) Ashish Vaswani, Noam Shazeer, Niki Parmar, Jakob Uszkoreit, Llion Jones, Aidan N Gomez, Ł ukasz Kaiser, and Illia Polosukhin. 2017. Attention is all you need. In I. Guyon, U. V. Luxburg, S. Bengio, H. Wallach, R. Fergus, S. Vishwanathan, and R. Garnett, editors, Advances in Neural Information Processing Systems 30, pages 5998–6008. Curran Associates, Inc.
- Wiedemann et al. (2019) Gregor Wiedemann, Steffen Remus, Avi Chawla, and Chris Biemann. 2019. Does BERT make any sense? interpretable word sense disambiguation with contextualized embeddings. In Proceedings of the 15th Conference on Natural Language Processing (KONVENS 2019): Long Papers, pages 161–170, Erlangen, Germany. German Society for Computational Linguistics & Language Technology.
- Wolf et al. (2020) Thomas Wolf, Lysandre Debut, Victor Sanh, Julien Chaumond, Clement Delangue, Anthony Moi, Pierric Cistac, Tim Rault, Rémi Louf, Morgan Funtowicz, Joe Davison, Sam Shleifer, Patrick von Platen, Clara Ma, Yacine Jernite, Julien Plu, Canwen Xu, Teven Le Scao, Sylvain Gugger, Mariama Drame, Quentin Lhoest, and Alexander M. Rush. 2020. Transformers: State-of-the-art natural language processing. In Proceedings of the 2020 Conference on Empirical Methods in Natural Language Processing: System Demonstrations, pages 38–45, Online. Association for Computational Linguistics.
- Yang et al. (2022) Xi Yang, Jie Zhang, Kejiang Chen, Weiming Zhang, Zehua Ma, Feng Wang, and Nenghai Yu. 2022. Tracing text provenance via context-aware lexical substitution. In Proceedings of the 36th AAAI Conference on Artificial Intelligence, Virtual.
- Yang et al. (2019) Zhilin Yang, Zihang Dai, Yiming Yang, Jaime Carbonell, Russ R Salakhutdinov, and Quoc V Le. 2019. XLNet: Generalized autoregressive pretraining for language understanding. In Advances in Neural Information Processing Systems, volume 32.
- Zhou et al. (2019) Wangchunshu Zhou, Tao Ge, Ke Xu, Furu Wei, and Ming Zhou. 2019. BERT-based lexical substitution. In Proceedings of the 57th Annual Meeting of the Association for Computational Linguistics, pages 3368–3373, Florence, Italy. Association for Computational Linguistics.
Appendix A Details of Pre-trained Models
Table 10 describe the details of the pre-trained models used in our experiments. We sourced these models from the Transformers library Wolf et al. (2020) except for SpanBERT, which we obtained from the original GitHub repository (https://github.com/facebookresearch/SpanBERT).
Appendix B Layer-Wise Ranking Performance
Figure 2 shows the layer-wise performance in the ranking task. Similar to the generation results (Figure 1), middle layers perform better than the first or last layers. It also shows that sentence-embedding models (SimCSE/SBERT) outperform their original models (BERT/MPNet) for several layers, different from the generation results where they perform similarly. This suggests that fine-tuning on semantic downstream tasks improves the capacity of the model to differentiate subtle semantic differences between synonymous words, but not their ability to retrieve relevant words from a large pool of words; it also suggests that optimal representations for these objectives might differ.
Appendix C Effectiveness of Using Multiple Layers
Table 11 shows the generation and ranking performance of our model on SWORDS using different layers. It shows that our method using multiple layers performs comparably or even better than selecting the best layer tuned on the test set for each model. It also shows that the best-performing layer differs across models, suggesting they capture lexical information in a different manner.
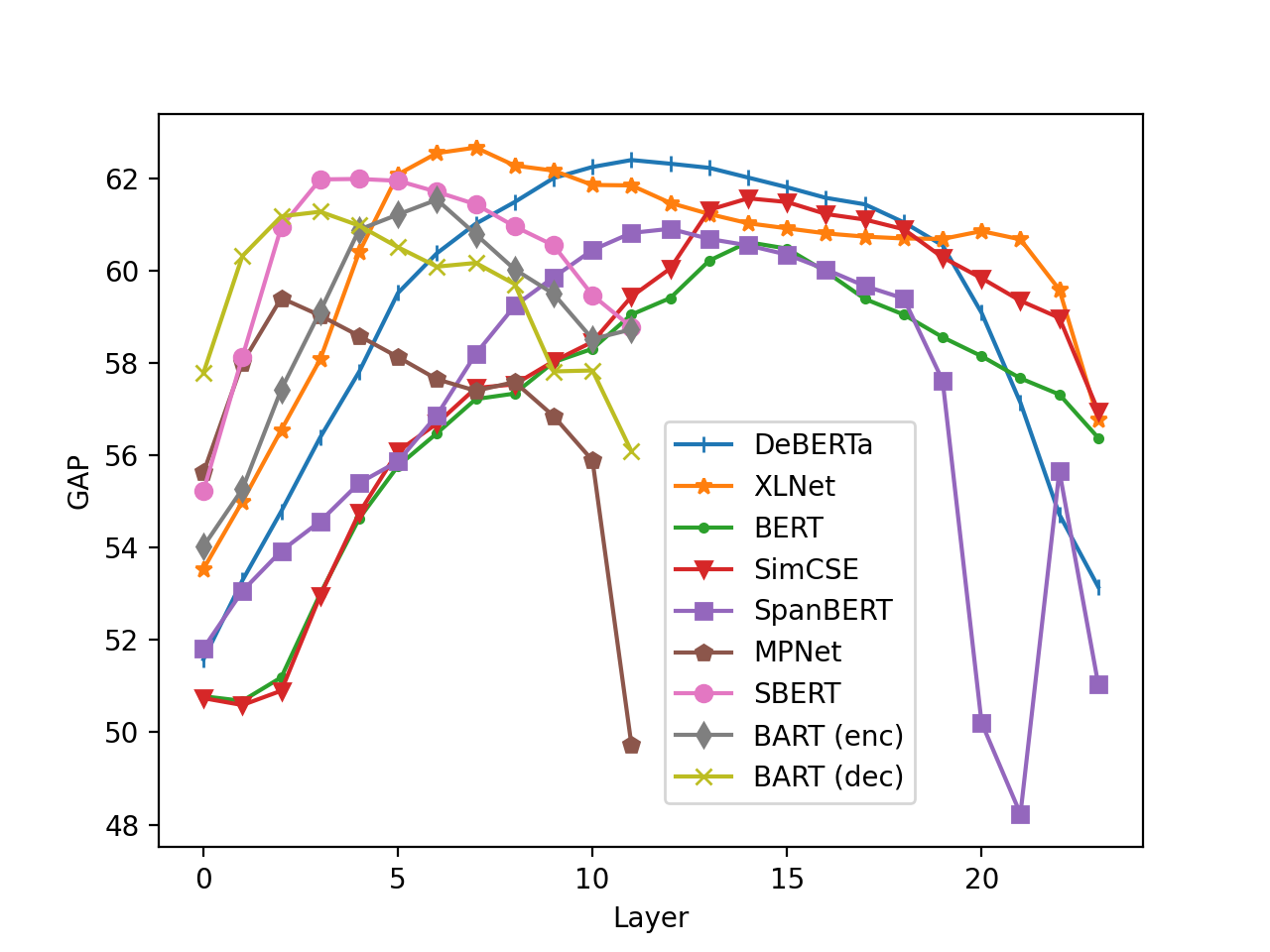
Models # Layer Emb Size Model Path BERT 24 1024 30522 bert-large-uncased mBERT 12 768 105879* bert-base-multilingual-uncased SpanBERT 24 1024 30522 spanbert-large-cased MPNet 12 768 30527 microsoft/mpnet-base XLNet 24 1024 32000 xlnet-large-cased ELECTRA 24 1024 30522 google/electra-large-discriminator DeBERTa-V3 24 1024 128000 microsoft/deberta-v3-large BART (Enc/Dec) 12 1024 50265 facebook/bart-large SBERT (MPNet) 12 768 30527 sentence-transformers/all-mpnet-base-v2 SimCSE (BERT) 24 1024 30522 princeton-nlp/sup-simcse-bert-large-uncased NMT (mBART, Enc) 12 1024 250054* facebook/mbart-large-50-one-to-many-mmt BERT (Italian) 12 768 31102 dbmdz/bert-base-italian-xxl-uncased ELECTRA (Italian) 12 768 31102 dbmdz/electra-base-italian-xxl-cased-discriminator
Generation Performance () Ranking Performance (GAP) Layer First Middle Last Best First Middle Last Best BERT 24.4 32.2 27.8 34.6 (16) 34.4 50.8 59.1 56.4 60.6 (15) 60.7 SpanBERT 24.9 33.1 8.8 33.2 (13) 31.1 51.8 60.8 51.0 60.9 (13) 60.8 MPNet 28.9 33.1 16.0 33.7 (4) 33.8 55.6 58.1 49.7 59.4 (3) 59.5 XLNet 28.6 33.0 28.4 34.3 (6) 35.0 53.5 61.9 56.8 62.7 (8) 62.9 DeBERTa-V3 33.3 36.8 25.2 37.2 (11) 36.9 51.6 62.4 53.2 62.4 (12) 62.9 BART (Enc) 14.6 30.8 22.6 30.8 (6) 30.0 54.0 61.2 58.7 61.5 (7) 61.9 BART (Dec) 21.9 28.0 18.9 30.8 (3) 29.0 57.8 60.5 56.1 61.3 (4) 60.8
Context I say I do not care about law, I care about service and she should care about money. Gold (Conceivable) worry, think, mind, desire, love, tend, cherish, consider, stress, concern, bother, watch BERT (L3-22) matter, caregiving, carefree, worry, concern, know, pay, caregivers, love, like BART (L3-10) concern, matter, carelessness, caretaker, carelessly, carefree, caretakers, worry, bother, mind BERT (L1) caregiving, carefree, caregiver, caregivers, aftercare, childcare, healthcare, skincare, custody, affections BERT (L12) matter, caregiving, worry, carefree, fret, love, despise, loathe, resent, pay BERT (L24) matter, worry, pay, concern, know, look, give, take, bother, think BART-Enc (L1) aftercare, caregiving, caretaker, carefree, carelessly, carelessness, caretakers, healthcare, caregivers, medicare BART-Enc (L6) concern, todo, caretaker, interest, carelessness, careless, disinterested, disdain, pay, carelessly BART-Enc (L12) todo, aswell, beleive, inbetween, zealand, pay, concern, usefull, noone, ofcourse BART-Dec (L1) caregiving, carelessness, caretaker, carelessly, carefree, aftercare, caretakers, concern, healthcare, worry BART-Dec (L6) concern, bother, worry, commit, reckon, dispose, shit, grieve, pay, strive BART-Dec (L12) worry, damn, concern, inquire, complain, passionate, shit, think, talk, whine Context “I’m starting to see more business transactions,” says Andrea West of American Telephone & Telegraph Co., noting growing interest in use of 900 service for stock sales, software tutorials and even service contracts. Gold (Conceivable) interestedness, enthusiasm, demand, attraction, popularity, excitement, curiosity, activity, importance, notice, significance, involvement, relevance, note, gain, passion, influence, accrual, concernment BERT (L3-22) curiosity, enthusiasm, intrigued, desire, concern, fascination, passion, attention, excitement, fondness BART (L3-10) fascination, enthusiasm, appetite, curiosity, excitement, concern, inclination, eagerness, desire, involvement BERT (L1) concern, importance, curiosity, investment, involvement, attention, focussed, fascination, focus, significance BERT (L12) curiosity, enthusiasm, concern, fascination, confidence, unease, belief, excitement, desire, passion BERT (L24) appetite, attracting, attractiveness, actively, demand, attention, intrigued, popularity, enthusiasm, flocking BART-Enc (L1) fascination, concern, intrigue, involvement, relevance, investment, stake, curiosity, enthusiasm, trustworthiness BART-Enc (L6) enthusiasm, fascination, appetite, curiosity, intrigued, excitement, intrigue, inclination, eagerness, enjoyment BART-Enc (L12) todo, aswell, inbetween, enthusiasm, fascination, eagerness, appetite, intrigued, attention, inclination BART-Dec (L1) fascination, involvement, intrigue, concern, participation, curiosity, investment, excitement, intrigued, importance BART-Dec (L6) fascination, appetite, involvement, engagement, affinity, uptake, demand, inclination, participation, appreciation BART-Dec (L12) participation, uptick, delight, decline, increase, spike, faith, decrease, grounding, surge
Appendix D Examples of Generated Substitutes
Table 12 shows examples of substitutes generated by our method using different layers (without reranking). It shows that the words retrieved by each layer are very different, indicating that each layer encodes very different information about the input word. For instance, given the target word care, the first layer of BERT and BART-Enc/Dec retrieves a large number of words that contain the target word as a sub-morpheme (e.g. aftercare, carefree).303030Since the edit distances between these words and the target word care are not greater than the threshold (0.5), they weren’t filtered out by our heuristic. This is presumably because the first-layer representations are highly affected by the input word embedding, and hence result in retrieving words that share the same subword token (e.g. care ##free) regardless of the semantic similarity. The last layer also performs poorly (as previously shown in Figure 1), e.g. BART-DEC (L12) retrieves participation as the closest word to the target word interest. This is because the last-layer representations of BART-decoder are used to directly predict the next word in after interest in the target sentence, and in fact, most of the retrieved words (e.g. uptick, faith, surge) are those that often collocate with in. Oddly, BART-Enc predicts a large number of substitutes that consist of multiple words (segmented by the tokeniser), none of which are relevant to the target word, e.g. aswell, todo, and inbetween as substitutes for interest. In fact, the number of such words increases (and the performance decreases) as the hyper-parameter gets bigger (which increases the influence of on the predictions). One possible interpretation is that the last layer representations of the BART encoder may contain vague contextual information rather than the lexical information of the input word, since they are used by the decoder to predict various words (esp. masked words) during pre-training. Lastly, another interesting observation is that, for the target word interest, the last layer representations of BERT and BART-enc retrieve a lot of words that start from a vowel sound, despite the absence of the article an before interest, suggesting that the embeddings contain some morphophonetic information.