Towards Lightweight Transformer via Group-wise Transformation for Vision-and-Language Tasks
Abstract
Despite the exciting performance, Transformer is criticized for its excessive parameters and computation cost. However, compressing Transformer remains as an open problem due to its internal complexity of the layer designs, i.e., Multi-Head Attention (MHA) and Feed-Forward Network (FFN). To address this issue, we introduce Group-wise Transformation towards a universal yet lightweight Transformer for vision-and-language tasks, termed as LW-Transformer111Source codes are released https://github.com/luogen1996/LWTransformer.. LW-Transformer applies Group-wise Transformation to reduce both the parameters and computations of Transformer, while also preserving its two main properties, i.e., the efficient attention modeling on diverse subspaces of MHA, and the expanding-scaling feature transformation of FFN. We apply LW-Transformer to a set of Transformer-based networks, and quantitatively measure them on three vision-and-language tasks and six benchmark datasets. Experimental results show that while saving a large number of parameters and computations, LW-Transformer achieves very competitive performance against the original Transformer networks for vision-and-language tasks. To examine the generalization ability, we also apply our optimization strategy to a recently proposed image Transformer called Swin-Transformer for image classification, where the effectiveness can be also confirmed.
Index Terms:
Lightweight Transformer, Visual Question Answering, Image Captioning, Reference Expression Comprehension.I Introduction
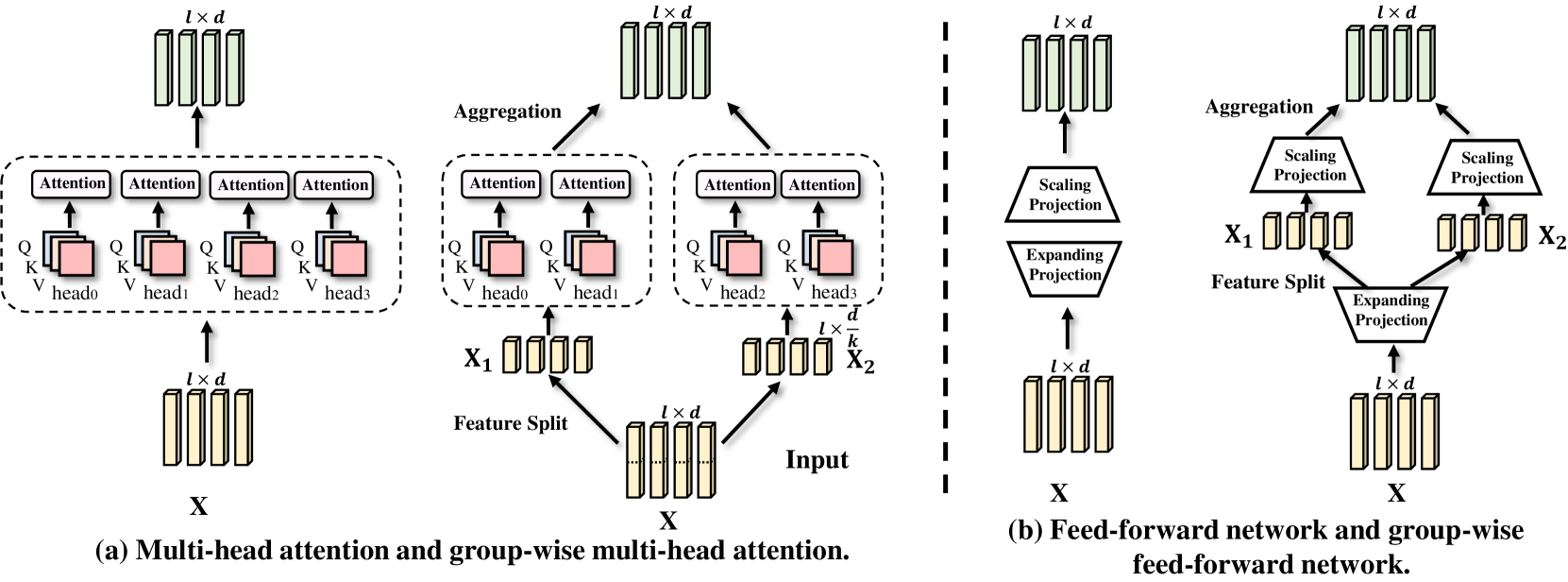
Transformer [1] has become the model of choice in the field of natural language processing due to its superior capability of modeling long-range dependencies and learning effective representations. Its great success has also attracted the attention of the computer vision community [2, 3, 4, 5]. At present, Transformer and its variants have been applied to a variety of vision-and-language tasks, such as visual question answering (VQA) [6, 7, 2], image captioning [8, 9, 3, 10], and referring expression comprehension [4, 11], achieving dominant performance in multiple benchmarks. Besides, Transformer also yields a new trend of large-scale multi-modal pretraining [12, 13, 14, 15, 16, 17, 18, 19, 20, 21], which greatly promotes the development of joint vision-and-language learning.
Coming with the outstanding ability of long-distance dependency modeling, Transformer-based models are also known for their extreme demand on computation power and memory space. Such an issue will become more prominent when applying Transformer to vision-and-language tasks, where the multi-modal network is typically built based on a large visual backbone, e.g., ResNet [22] or Faster R-CNN [23]. For instance, VL-BERT-large [18] needs to be trained on 8 GPUs in parallel for nearly 20 days, and its parameters are about 340 millions, which takes about 1.2 GB of memory to deploy. Thus, the large latency and memory footprint greatly hinder the application of Transformer on the mobile devices.
To this end, the network compression for Transformer-based models has attracted significant research attention [24, 25, 26, 27, 28]. Popular directions include distillation [25, 27], layer pruning [24] and quantization [26, 28]. Beyond these methodologies, one orthogonal direction is to directly optimize the Transformer layer itself [29, 30, 31], which has not been paid enough attention and is the focus of this paper. By designing a more efficient version of Transformer that still preserves the accuracy, one can directly train a model based on the improved structure to avoid the training-distilling process [32], which can be also combined with the popular compression methods to further improve their efficiency.
However, designing an efficient Transformer remains as a challenging problem, mainly due to the unique structures of the Multi-Head Attention (MHA) and the Feed-Forward Network (FFN). First, as a key component in Transformer, MHA is used to capture the dependency of input features in various subspaces, upon which efficient feature transformations can be obtained [1]. In this case, these transformations in MHA not only map features to new semantic spaces but also compute the attention scores using the softmax function. Second, as a layer-to-layer connection design, FFN achieves efficient transformations by expanding and scaling the feature dimensions, which inevitably involves a large number of parameters. Both designs pose a huge obstacle in reducing Transformer parameters.
In this paper, we introduce Group-wise Transformation to achieve a lightweight Transformer for vision-and-language tasks, termed LW-Transformer, which well addresses the aforementioned issues in a principled way. Group-wise Transformation is an efficient method used in many convolution neural networks (CNNs) [33, 34, 35, 36, 37, 38]. It splits the input features into groups by channels to perform parameterized transformations, and then concatenates the transformed features as the output. This group-wise operation can reduce the parameters and computations of the original transformation by . Based on this strategy, we propose the group-wise multi-head attention (G-MHA) and group-wise feed forward networks (G-FFN) for LW-Transformer.
Notably, in addition to improve model compactness, LW-Transformer can well maintain the desired principles of the original Transformer. The effectiveness of Transformer mainly lies in its MHA design [1], which captures the diverse feature dependencies through different feature subspaces. These subspaces are obtained by truncating the input features after linear projections, as shown in Fig. 1. The principle of Group-wise transformation is essentially consistent with that of MHA, which also aims to learn more efficient feature representations through more diverse feature spaces. In this case, the proposed G-MHA takes a step forward than MHA, which directly splits input features before projections. More importantly, this operation does not change neither the feature dimension nor the scaled-dot product for attention modeling. Meanwhile, G-FFN also maintains the expanding-scaling property of FFN while reducing the computation and parameters to a large extent, as shown in Fig. 1.
During experiments, we build LW-Transformer based on the structures of the default Transformer and its variants, including MCAN [2], LXMERT [19] and Swin-Transformer [39], and conduct extensive experiments on six benchmark datasets, i.e., GQA [40], VQA2.0 [41], CLEVR [42], COCO [43], RefCOCO [44], RefCOCO+ [44], of three language-and-vision tasks, i.e., VQA [41], image captioning [43] and referring expression comprehension (REC) [45]. We also test LW-Transformer on the large-scale and BERT-style pretraining [19] and the task of image classification. The experimental results show that with an overall reduction of up to 45% of parameters and 28% of computations222Under the setting of LWTransformer1×., LW-Transformer still achieves competitive performance against the default Transformer on six benchmark datasets of the three VL tasks and even obtains performance gains on some benchmarks, e.g., +1% on GQA, +0.1% on COCO Captioning and +3.2% on RefCOCO, which well confirms its effectiveness for vision-and-language tasks. In addition, its generalization ability is also well validated on ImageNet and CIFAR-100. Compared with Swin-Transformer, LW-Transformer can obtain the same performance on CIFAR-100 while saving 42.4% computations and 43.3% parameters. On ImageNet, LW-Transformer can even obtain better performance with similar experimental cost to Swin-Transformer.
As a summary, our contributions are three-fold:
-
•
We present the first application of Group-wise transformation to Transformer, and propose an efficient Transformer network called LW-Transformer.
-
•
On six benchmarks of three language-and-vision tasks, LW-Transoformer achieves competitive performance against the default Transformers with a reduction of up to 45% parameters and 28% computations. Its generalization ability is also validated on the recently proposed Swin-Transformer [39] for image classification.
-
•
We conduct extensive experiments to examine the optimizations of different designs in Transformer, which can provide useful insights for its future optimization.
II Related Work
Due to the superior capability of learning efficient representations, Transformer [1] is becoming popular in both natural language processing [29, 46, 47, 1] and computer vision [8, 6, 7, 9, 3, 10, 2]. A set of Transformer-based approaches have been proposed to achieve the state-of-the-art performance in VQA [6, 7, 2, 48] and image captioning [9, 3, 10, 49, 50, 51, 52, 53]. For example, the recent X-Linear Transformer [49] integrates bilinear pooling [54] and squeeze-excitation module [55] into self-attention to selectively capitalize on visual information and to perform multimodal reasoning. With these novel designs, X-Linear Transformer improves the SOTA performance of image captioning on the CIDEr [56] metric by +1.6. Meanwhile, these Transformer-based networks also lead to a trend of large-scale language-and-vision pre-training [14, 15, 16, 17, 18, 19, 21, 57], which break the best performance of various tasks, such as Visual Question Answering [58] and Image Captioning [43]. In addition, a lot of vision transformers [39, 59] have been proposed recently, which shows the superior performance on image classification. For example, XCiT [59] achieves 86.0 % top-1 accuracy on ImageNet, outperforming conventional CNNs by a large margin. Despite the great success, Transformer and its variants have been criticized for the excessive parameters and high computation overhead. For instance, the parameter sizes of BERT [13], ViLBERT [16] and LXMERT [19] are 85, 221 and 181 millions, respectively. Such a large amount of parameters greatly hinder the applications of Transformer and Transformer-based models on the mobile applications, where both the storage and computation resources are limited.
Several recent advances [29, 30, 31] are committed to design the efficient Transformer networks. Specifically, Linformer [31] reduces the computation cost of self-attention by compressing the sequence length of input features. Performer [30] applies the scalable kernel methods to approximate the scale-dot product attention to reduce computation overhead when the sequence length is very large. Tensorized Transformer [29] uses tensor decomposition to reduce the parameters of Q, K and V in self-attention. Compared with these efficient Transformers, the proposed LW-Transformer neither changes the length of input sequences nor the definition of attention modeling.
The principle of the introduced Group-wise Transformation is also related to the group convolution, which can be traced back to a set of classical convolutional neural networks [33, 60, 34, 35, 36, 37, 38] like AlexNet [34]. The depth-wise separable convolution in Xception [33] generalized the method into Inception Networks [36, 37]. ShuffleNets [38] and MobileNets [60, 35] use the group-wise and depth-wise separable convolution to achieve lightweight deep networks with competitive performance. However, due to the huge difference between the principles of multi-head self-attention (MHA) and convolution, its application to Transformer networks is still left unexploited. Except for improving model efficiency, the motivation of this paper is different from the mentioned CNNs. Existing methods like Xception [33] use group-wise transformation to realize the multi-branch structure, so as to increase the capacity of the model. In contrast, our application is to inherit the property of global dependency in self-attention, as discussed in the introduction.
III Approach
In this section, we first recap the main components of Transformer, introduce Group-wise Transformation, and then describe the proposed LW-Transformer in detail.
III-A Preliminary
In Transformer, each layer typically contains two main components, i.e., Multi-Head Attentions (MHA) for dependency modeling and Feed-Forward Network (FFN) for position-wise transformations.
Multi-Head Attention is used to capture the dependency between input features by the scaled dot-product attention [1], upon which efficient representations are learned. Specifically, given the transformed matrices of query , key and value , the scaled dot-product attention function is formulated as:
(1) |
Here, and denote the sequential length and the feature depth, respectively. To further improve the representational ability, Vaswani et al. [1] extends Eq. 1 to a multi-head version, denoted as multi-head attention (MHA), which consists paralleled “heads” to make the scale dot-product attention. Specifically, given the input features , MHA is formulated as:
(2) | ||||
Here, , and are the split features for the i-th head, and they are obtained by truncating the input features X after projection. Meanwhile, a linear-merge projection is used after MHA, defined as:
(3) |
where is the weight matrix and is the final output.
Feed-Forward Network makes a position-wise and dense transformation from the input features to the output ones, which keeps the non-linearity by expanding the hidden units in the ReLU layer, as shown in Fig. 1 (c). This design is beneficial to prevent manifold collapse caused by ReLU, as well as to extend the model capacity [35]. The formulation of FFN can be written as
(4) |
Here , , and are weights and bias, and is the ReLU activation. Typically, is a quadruple as large as .
III-B Lightweight Transformer
To achieve the lightweight Transformer, we optimize its two main designs, i.e., MHA and FFN, with Group-wise transformation. In this subsection, we begin with the introduction of Group-wise transformation, and then describe its deployment on MHA and FFN in detail.
Group-wise transformation. As shown in Fig. 1, given the input features , we first divide it into groups. Those features of different groups are transformed by the function and then concatenated again as one complete output. The formulation of Group-wise Transformation, denoted as , is defined by
(5) | ||||
Here, denotes the truncated features of the -th group, denotes concatenation and can be any parameterized function, e.g., linear/ non-linear projections or more complex modules like self-attention [1].
With the split-transform-concatenate design, the Group-wise Transformation can save of parameters and of the computations. To further reduce the number of parameters, we can also share the learnable weights for each group, consistently resulting in reductions of parameters.
Group-wise Multi-Head Attention. Specifically, we extend the original Multi-Head Attention (MHA) in Transformer to a group-wise version (G-MHA), as shown in Fig. 1 (a).
As described in Sec. III-A, MHA obtains the multiple attentions via truncating Q, K and V after the projection of X. In this case, G-MHA can be considered as its extension in principle, which directly splits the input features before projection. Concretely, the input features are first divided into splits , based on which the multi-head attentions are performed. Afterwards, those attention outputs are concatenated again. Then, G-MHA is defined as:
(6) | ||||
As shown in Fig. 1 (a), the group operation of G-MHA is conducted on the feature dimension instead of the sequence length. Therefore, each feature can still obtain its coupling coefficients to the others through the comparisons on the truncated features, similar to the default MHA. To this end, the attention patterns learned by G-MHA can be close to that of MHA in principle.
Notably, we do not replace the linear-merge layer in MHA, i.e., in Eq.2, which is founded to be important for expanding the learning capacity of Transformer and facilitating the cross-group information exchange. Overall, G-MHA reduces the parameter size from to about , and the computational complexity from O to about O.
Group-wise Feed-Forward Network. We further deploy Group-wise Transformation to FFN, of which implementation is illustrated in Fig. 1 (b). Specifically, given an input , the group-wise feed-forward network (G-FFN) performs non-linear projections to expand the feature size by
(7) |
where and . Then, the obtained hidden features are divided into k independent features , which is further linearly transformed with sharable weights. G-FFN can be formulated as:
(8) | ||||
where and . Compared to the original FFN, G-FFN reduces the parameter size from O() to O() and the complexity from O() to O().
As shown in Eq. 8 and Fig. 1 (b), G-FFN can still maintains the expanding-scaling property of the default FFN. Notably, the group-wise operation is only deployed on the last linear layer, while the first non-linear projection remains intact. To explain, this design is beneficial to protect the non-linearity of FFN and prevent the manifold collapse [35], therefore avoiding the performance degeneration.
Notations | Description |
---|---|
n (the suffix) | The expanding multiples of projection dimensions |
in Q and K. | |
mini (the suffix) | The mini version illustrated in Tab.III. |
k | The number of groups in the transformations. |
WS | Weight sharing. |
G-MHA | Group-wise multi-head attention. |
G-FFN | Group-wise feed-forward network. |
G-LML | Group-wise linear-merge layer in MHA. |
G-IL | Group-wise intermediate layer in FFN. |
IV Experiments
To validate the proposed LW-Transformer, we apply it to a set of Transformer and BERT-style models, and conduct extensive experiments on six benchmark datasets, i.e., VQA2.0 [41], GQA [40], CLEVR [42], MS-COCO [43], RefCOCO [44], RefCOCO+ [44], of three vision-and-language tasks, i.e., Visual Question Answering (VQA) [58], Image Captioning (IC) [43] and Referring Expression Comprehension (REC) [45]. To examine its generalization ability, we build LW-Transformer based on the newly proposed Swin-Transformer [39] for image classification.
IV-A Deployed Networks
Transformer: For VQA, IC and REC, we use the Transformer networks proposed in [1, 2] as our baseline model, which all follow a classical encoder-decoder structure [1]. In the baseline, we set the , , defined in Eq.1 - 2, as 512, 2,048, 64, respectively. The number of attention heads is 8 for each Transformer layer, and both the encoder and decoder branches are composed of six Transformer layers. For simplicity, we denote the baseline network as Transformer. We further replace Transformer with the proposed LW-Transformer layers, and keep the rest designs unchanged. We denote the compressed network as LW-Transformer. In LW-Transformer, the basic settings of , , are the same as Transformer. The number of groups is set to 2 by default, and each group has 4 attention heads, so the total number of attentions is kept as 8 as Transformer.
Bert-style Model: The deployed Bert-style model is the recently proposed LXMERT [19], of which structure is slightly different from the conventional Transformer network. It has 9 and 6 Transformer encoder layers for the language and vision modelings, respectively, and 5 cross-modal Transformer layers333Cross-modal Transformer layer has two MHA and FFN. for the multi-modal interactions. During experiments, we replace the encoder and decoder Transformer layers with the proposed LW-Transformer layer. The model settings are similar to the standard bert [13], i.e., 768, , , , . For simplicity, we denote the network with our optimization methods as LW-LXMERT. The detailed explanation of the notations is given in Tab. I.
During experiments, we also examine the sensitivity in mapping dimensions of query Q and key K towards the model performance. Hence, we add an suffix after LW-Transformer to indicate the change in attention dimensions of Q and K. For instance, of LW-Transformer1× is 64, while the one of LW-Transformer3× is 192.
Swin-Transformer: For image classification, we use Swin-Transformer-tiny [39] as our baseline structure. Swin-Transformer-tiny is a hierarchical Transformer, which contains 12 Transformer blocks in 4 stages. During experiments, we simply replace the MHA and FFN of the original Swin-Transformer-tiny with our G-MHA and G-FFN. The modified model is denoted as LW-Transformer. To compare with the original Swin-Transformer under the same parameters, we also build a large model containing 16 Transformer blocks, namely LW-Transformer-large. Meanwhile, we remove weight-sharing in our model, since we find that it degrades the performance of image classification.
Model | G-MHA | G-FFN | WS | k | #Params333The parameters of the language and vision encoders, i.e., CNN and LSTM, are not counted. | MAdds | VQA2.0 | COCO* | RefCOCO |
---|---|---|---|---|---|---|---|---|---|
Transformer | - | - | - | - | 44.1M | 2.58G | 67.17 | 117.0 | 80.8 |
LW-Transformer1× | ✓ | ✗ | ✗ | 2 | 37.0M | 2.21G | 67.11 | 116.7 | 80.9 |
LW-Transformer1× | ✗ | ✓ | ✗ | 2 | 37.7M | 2.22G | 67.15 | 117.1 | 81.0 |
LW-Transformer1× | ✓ | ✓ | ✗ | 2 | 32.4M | 1.85G | 67.13 | 117.1 | 80.9 |
LW-Transformer | ✓ | ✓ | ✓ | 2 | 24.0M | 1.85G | 67.10 | 116.9 | 80.7 |
LW-Transformer2× | ✓ | ✓ | ✓ | 2 | 26.3M | 2.16G | 67.14 | 117.1 | 80.8 |
LW-Transformer | ✓ | ✓ | ✓ | 2 | 28.7M | 2.46G | 67.19 | 117.2 | 80.9 |
LW-Transformer3× | ✓ | ✓ | ✓ | 4 | 21.9M | 1.83G | 66.68 | 115.9 | 80.0 |
LW-Transformer3× | ✓ | ✓ | ✓ | 8 | 19.8M | 1.51G | 66.29 | 114.6 | 79.3 |
* CIDEr is used as the metric. These results are before the CIDEr optimization stage. | |||||||||
denotes the model for the further experiments. |
IV-B Datasets
Visual Question Answering: We conduct experiments on three vqa benchmark datasets, i.e., VQA2.0 [41], GQA [40] and CLEVR [42]. VQA2.0 contains about 1.1M image-question pairs from real word, in which there are 440K examples for training, 210K for validation, and 450K for testing. CLEVR is a synthetically generated dataset that aims to test the visual reasoning ability of models. It includes 700K and 150K examples for training and test, respectively. GQA contains 22M questions over 140K images, which is designed to test the visual reasoning ability of models in real scenes. In terms of the evaluation metric, we use the VQA accuracy [58] for VQA2.0, and the classification accuracy for GQA and CLEVR.
Referring Expression Comprehension: RefCOCO [45] and RefCOCO+ [45] datasets are used in our experiments. Both datasets contain nearly 140K referring expressions for 50K bounding boxes of 20K images. The categories of TestA are about people and the ones of TestB are about objects. RefCOCO has more descriptions related to the spatial relations, while RefCOCO+ excludes these spatial-related expressions and adds more appearance-related ones. Following [61, 62, 63, 64, 65, 66, 67, 68], we use the top-1 accuracy as the metric on both datasets.
Image Captioning: COCO Captioning [43] contains more than 120K images from MS-COCO [43], each of which is annotated with 5 different captions. We train and test our models with the Karpathy splits [69]. BLEU [70], METEOR [71], ROUGE [72], CIDEr [56] and SPICE [73] are used as the metrics for evaluation.
Image Classification: ImageNet-1K [74] is the most widely-used benchmark for image classification, which contains 1.28M training images and 50K validation images from 1,000 classes. Cifar-100 [75] is a benchmark containing 60,000 low-resolution images from 100 classes. In Cifar-100, there are 50,000 images for training and 10,000 images for validation.
Model | G-LML | G-IL | #Params | MAdds | VQA2.0 | COCO* | RefCOCO |
---|---|---|---|---|---|---|---|
Transformer [1] | - | - | 44.1M | 2.58G | 67.17 | 117.0 | 80.8 |
LW-Transformer1× | ✗ | ✗ | 24.0M | 1.85G | 67.10 | 116.9 | 80.7 |
LW-Transformer1× | ✓ | ✗ | 20.4M | 1.69G | 66.93 | 116.5 | 80.2 |
LW-Transformer | ✗ | ✓ | 14.5M | 1.50G | 66.57 | 115.7 | 79.8 |
LW-Transformer1× | ✓ | ✓ | 11.0M | 1.33G | 66.36 | 114.8 | 79.3 |
* CIDEr is used as the metric. These results are before the CIDEr optimization stage. |
Model | #Params | All | Y/N | Num | Other |
---|---|---|---|---|---|
MCB [54] | 32M | 61.23 | 79.73 | 39.13 | 50.45 |
Tucker [76] | 14M | 64.21 | 81.81 | 42.28 | 54.17 |
MLB [77] | 16M | 64.88 | 81.34 | 43.75 | 53.48 |
MFB [78] | 24M | 65.56 | 82.35 | 41.54 | 56.74 |
MUTAN [76] | 14M | 65.19 | 82.22 | 42.10 | 55.94 |
MFH [79] | 48M | 65.72 | 82.82 | 40.39 | 56.94 |
BLOCK [80] | 18M | 66.41 | 82.86 | 44.76 | 57.30 |
LW-Transformer | 14.5M | 69.68 | 86.03 | 51.62 | 59.80 |
IV-C Experiment Setups
Transformer: For all datasets except CLEVR [42], we use the regional features from [81] as the visual inputs. On VQA2.0, GQA and IC, all networks are trained for 13 epochs, 3 of which are for warming-up. The basic learning rate is set to 1e-4, where denotes the number of groups, and decayed on 10 epochs and 12 epochs with a factor of 0.2. For CLEVR, we follow the previous works [42, 82, 83, 84, 85] to use grid features extracted by ResNet101 [22]. The model is trained for up to 16 epochs and warmed up for 3 epochs. The basic learning rate is set to 4e-4 and decayed by 0.2 on 13 epochs and 15 epochs. For IC, another 17 training epochs is further used for the CIDEr-D [56, 51] optimization with a learning rate of 5e-6. The Adam optimizer [86] is used to train all networks.
Bert-style Model: Following the settings of LXMERT [19], the visual features are extracted by Faster R-CNN [23] with ResNet101 [22] backbone pretrained on the Visual Genome [87]. The training procedures are divide into two steps, i.e., pretraining and finetune. We follow the default setting of LXMERT to pre-train the model. The pretraining takes 20 epochs overall, where the optimizer is Adam [86] with a initial learning rate of 1e-4. We then finetune the model on VQA and GQA with a learning rate of 1e-5 for 5 epochs.
Swin-Transformer: Following the default settings of Swin-Transformer [39], we use the AdamW optimizer with an initial learning rate of 0.001 and a weight decay of 0.05. We use a cosine decay learning rate scheduler with 20 epochs of linear warm-up. Models are trained for total 300 epochs with 1024 batchsize. For ImageNet and Cifar-100, we adopt the input image resolution of . Following the training strategy of Swin-Transformer, a set of data augmentations, i.e., RandAugment, Mixup, Cutmix, random erasing and stochastic depth, are applied to avoid overfitting.
Model | #Params33footnotemark: 3 | MAdds | VQA2.0 | COCO* | RefCOCO |
---|---|---|---|---|---|
Transformer [1] | 44.1M | 2.58G | 67.17 | 117.0 | 80.8 |
Tensorized Transformer [29] | 27.5M | 3.71G | 67.03 | 116.3 | 80.6 |
Performer [30] | 44.1M | 3.36G | 65.20 | 116.2 | 80.0 |
Linformer [31] | 44.2M | 2.53G | 64.20 | - | 80.3 |
LW-Transformer1× | 24.0M | 1.85G | 67.10 | 116.9 | 80.7 |
LW-Transformer3× | 28.7M | 2.46G | 67.19 | 117.2 | 80.9 |
* CIDEr is used as the metric. These results are before the CIDEr | |||||
optimization stage. |
VQA2.0 | CLEVR | GQA | |||
model | test-dev | model | test | model | test-dev |
Bottom-Up [81] | 65.3 | SAN [42] | 76.7 | Bottom-Up [81] | 49.7 |
MFH [79] | 68.8 | RN [84] | 95.5 | MAC [2] | 54.1 |
BAN [88] | 70.0 | FiLM [83] | 97.7 | LCGN [85] | 57.1 |
MCAN [2] | 70.6 | MAC [82] | 98.9 | BAN [88] | 57.1 |
Transformer [1] | 70.6 | Transformer [1] | 98.4 | Transformer [1] | 57.4 |
LW-Transformer1× | 70.4 | LW-Transformer1× | 98.6 | LW-Transformer1× | 58.4 |
LW-Transformer3× | 70.5 | LW-Transformer3× | 98.7 | LW-Transformer3× | 57.5 |
RefCOCO | RefCOCO+ | ||||
---|---|---|---|---|---|
model | testA | testB | model | testA | testB |
Spe+Lis+Rl [89] | 73.1 | 64.9 | Spe+Lis+Rl [89] | 60.0 | 49.6 |
DDPN [66] | 80.1 | 72.4 | DDPN [66] | 70.5 | 54.1 |
MattNet [65] | 81.1 | 70.0 | MattNet [65] | 71.6 | 56.2 |
NMTree [63] | 81.2 | 70.1 | NMTree [63] | 72.0 | 57.5 |
Transformer [1] | 84.0 | 73.4 | Transformer [1] | 75.9 | 61.1 |
LW-Transformer1× | 84.2 | 73.7 | LW-Transformer1× | 75.9 | 61.0 |
LW-Transformer3× | 83.9 | 74.3 | LW-Transformer3× | 76.5 | 60.8 |
COCO Captioning | ||||||
---|---|---|---|---|---|---|
model | Params | BLEU-4 | METEOR | ROUGE | CIDEr | SPICE |
ORT [9] | 45M | 38.6 | 28.7 | 58.4 | 128.3 | 22.6 |
AoANet [3] | 64M | 38.9 | 29.2 | 58.8 | 129.8 | 22.4 |
GCN-LSTM+HIP [52] | - | 39.1 | 28.9 | 59.2 | 130.6 | 22.3 |
Transformer [8] | 33M | 39.1 | 29.2 | 58.6 | 131.2 | 22.6 |
X-Transformer [49] | 138M | 39.7 | 29.5 | 59.1 | 132.8 | 23.4 |
Transformer [1] | 44M | 38.9 | 29.0 | 58.5 | 131.0 | 22.3 |
LW-Transformer1× | 24M | 38.7 | 29.2 | 58.3 | 130.9 | 22.7 |
LW-Transformer3× | 29M | 38.9 | 29.2 | 58.6 | 131.3 | 22.6 |
IV-D Experiment Results
IV-D1 Ablation Study
We first examine different designs of the proposed LW-Transformer including group-wise multi-head attention (G-MHA), group-wise feed-forward network (G-FFN) and weight sharing (WS), and also evaluate the sensitivity of hyper-parameters like the group number () and the dimensions of query and key in MHA (indicated by the subfix after LW-Transformer, i.e., defined in Eq. 2). The results are reported in Tab. II.
The effect of group-wise transformation. The second block of Tab. II illustrates the impact of G-MHA, G-FFN and WS on the parameter size, computation cost and overall performance of LW-Transformer. From these results we can see that while greatly reducing the model parameters and computations, each of these designs will hardly reduce model performance, and even help the model obtain slightly improvements on COCO and RefCOCO. In addition, after deploying all designs, i.e., LW-Transformer1×, the parameters and computations are reduced by 45.5% and 28.3%, respectively. However, the performance drop is still very marginal, e.g., -0.1% on VQA2.0, -0.08% on COCO and -0.12% on RefCOCO. Thus, we can conclude that group-wise designs in LW-Transformer can effectively reduce both parameter size and computation cost, while maintaining the performance.
The impact of expanding attention dimensions. We explore the effects of expanding the attention dimensions of query and key in MHA, of which results are given in the third block of Tab.II. Here, the suffixes, i.e., and , indicate the multipliers of dimension expansion. From these results, we observe that expanding the MHA dimension further improve the model performance, which helps LW-Transformer outperform the original Transformer on all datasets with much fewer parameters. For instance, compared with LW-Transformer1×, the efficiency of LW-Transformer2× is relatively less significant, but it still reduce 40% parameters and 16.3% computations, respectively. More importantly, it has almost the same performance to the default Transformer. We also notice that the increased parameter size is still small, e.g., +4.7M for . In contrast, expanding the projection dimensions significantly increases the model size in the original MHA, e.g., +18.8M for . To explain, the input features in G-MHA are first truncated into multiple groups, which will leads to less parameters than the direct projection. This observation also confirms another merit of Group-wise transformation.
The impact of group number. Next, we test LW-Transformer with different group numbers (), as reported in the last block of Tab. II . From this table, we notice that although increasing the group number further reduces the model size, the performance degradation becomes relatively obvious. For this case, our understanding is that both the attention modeling and feature transformation require a certain capacity to learn the large-scale language-and-vision data, e.g., VQA2.0, and setting too small feature dimensions will be counterproductive. In addition, since some layers are not included in our optimization scheme, e.g., the linear merge layer in MHA, the lower bound of LW-Transformer’s parameter size is about 20M. After experiments, we find that a trade-off between the efficiency and the performance is .
Model | groups | params | MAdds | Cifar-100 | ImageNet | ||
---|---|---|---|---|---|---|---|
top-1 | top-5 | top-1 | top-5 | ||||
Swin-Transformer [39] | - | 28.3M | 4.5G | 77.9 | 94.5 | 81.2 | 95.5 |
Performer [30] | - | 27.5M | 4.1G | - | - | 79.0 | 94.2 |
LW-Transformer1× | 2 | 20.0M | 3.3G | 77.8 | 94.7 | 79.9 | 94.9 |
LW-Transformer-large1× | 2 | 27.0M | 4.5G | 78.8 | 95.2 | 81.5 | 95.7 |
LW-Transformer1× | 4 | 16.9M | 2.6G | 77.7 | 94.5 | 78.9 | 94.4 |
Metrics | Visual Question Answering | Reffering Expression Comprehension | Image Captioning | V&L Pre-training | Image Classification | |||
---|---|---|---|---|---|---|---|---|
VQA2 | CLEVR | GQA | RefCOCO | RefCOCO+ | MS-COCO | GQA | Cifar100 | |
Performance Gains | -0.1 | +0.3 | +1.0 | +0.2 | +0.0 | -0.1 | +0.6 | -0.1 |
Parameter Reduction | -45% | -45% | -45% | -45% | -45% | -45% | -45% | -41% |
Computational Reduction | -28.3% | -28.3% | -28.3% | -28.3% | -28.3% | -28.3% | -28.3% | -42.2% |
IV-D2 Fully Group-wise LW-Transformer
In LW-Transformer, Group-wise transformation is not applied in two inner layers of Transformer, i.e., linear merge layer of MHA and the intermediate layer of FFN. In practice, we find that these two layers are important for keeping the model capacity and facilitating the cross-group information exchange. In Tab. III, we quantitatively validate the effects of deploying Group-wise transformation on these two layers, denoted as Group-wise Linear-Merge Layer (G-LML) and Group-wise Intermediate Layer (GIL), respectively. From Tab. III, we can see that when G-LML and G-IL are deployed, the parameters and computations are further reduced by 29.5% and 19.7%, respectively, but the performance declines are relatively significant, e.g., from 67.10 to 66.36 on VQA and from 116.9 to 114.8 on COCO. To explain, the completely group-wise structure divides the network into two independent sub-networks without any cross-group information exchange, which ultimately results in the performance degeneration. Based on these observations, we therefore keep the linear-merge layer of MHA and the intermediate layer of FFN intact in LW-Transformer.
In addition, we also notice that retaining G-IL is the best choice after the design introduced in the paper, where both compactness and performance are satisfactory. Therefore, we further extend LW-Transformer to a mini version, i.e., deploying G-IL and keeping the linear-merge layer intact for cross-group information exchange, termed as LW-Transformermini and compare it with the existing multi-modal fusion networks [76, 80, 54, 77, 78, 79], of which results are shown in Tab. IV. From Tab. IV, we find that even compared to state-of-the-art methods, i.e., BLOCK [80], LW-Transformer outperforms it by a large margin, while the number of parameters is much smaller, i.e., 14.5M vs 18M. Such results greatly support the effectiveness of LW-Transformer under the extremely lightweight settings. Meanwhile, it also suggests that the liner merge layer (LML) is an important design in MHA, which needs to be carefully considered during optimization.
IV-D3 Comparisons with other Efficient Transformers
In Tab. V, we also compare LW-Transformer with existing efficient transformers including Tensorized Transformer [29], Performer [30] and Linformer [31]. In Tab. V, Tensorized Transformer applies Tucker Decomposition, a popular method in network compression [29], to decompose the projection weights in MHA. Performer [30] approximates the scaled-dot product attention by using scalable kernel methods. Linformer [31] uses additional projection layers to reduce the length of the input sequence, which is hard to apply to apply to the sequence-to-sequence generation tasks like Image Captioning. So, we only report the results of Linformer on VQA2.0 and RefCOCO.
From Tab. V, we can see that LW-Transformer obtains better performance than the other compressing methods, and its performance gains are very distinct on VQA2.0. For these results, our understanding is that changing the original definition of self-attention will affect the effectiveness of Transformer to some degree. For instance, Linformer reduces the length of the features for attention, which inevitably leads to the loss of information for fine-grained tasks like VQA. The performance of Performer also suggests that there is still a gap between the approximated attention and the default one.
Secondly, compared with the original structure, Performer and Tensorized Transformer greatly increases the computation cost (+30% and +43.8%), while our methods can reduce it by up to 28.3%. The merit of Linformer in computation is also very limited. To explain, Linformer and Performer are proposed for the tasks with very large input sequences, e.g., language modeling [90] and unidirectional/causal modeling [91]. In unidirectional/causal modeling, the sequence length of input features can be up to 12,288, so the computation reductions by Linformer and Performer can be up to 68.8% and 41.3%, respectively. In contrast, the sequence length for VL tasks typically ranges from 30 to 100 [41, 45, 43], which are much smaller. Therefore, these efficient transformer will increase the amount of computations for VL tasks in contrast.
From these observations, we can conclude that the efficiency of LW-Transformer is indeed obvious, especially considering its performance gains to other optimization methods. More importantly, it can improve the efficiency of Transformer while maintaining the original definition of attention modeling.
IV-D4 Comparisons with the State-of-the-art Methods
We further compare LW-Transformer with SOTA methods on six benchmark datasets of three multi-modal tasks, i.e., VQA, IC and REC. The results are given in Tab. VI - VIII.
Tab. VI shows the comparison on three widely-used benchmark datasets of VQA, i.e., VQA2.0 [41], CLEVR [42] and GQA [40]. The first observation from this table is that the performance of the proposed LW-Transformer is very competitive on all three datasets. And it achieves new SOTA performance on GQA, which is the largest VQA dataset for visual reasoning. Besides, compared to these SOTA methods, LW-Transformer has an obvious advantage in terms of the parameter size. For instance, BAN-8 and MCAN have 93.4 and 44.1 millions of parameters33footnotemark: 3, respectively, while LW-Transformer1× and LW-Transformer3× only have 24.0 and 28.7 millions of parameters, respectively. In addition, we observe that LW-Transformer achieves better performance than Transformer except for VQA2.0, which has a strong language bias and requires a larger parameter size to accommodate the data distribution [41]. These observations greatly confirm the effectiveness of LW-Transformer and the introduced Group-wise Transformation on the VQA task.
On REC, which is a task of exploring language-vision alignment, the advantages of LW-Transformer are more significant, as shown in Tab. VII . Compare to SOTA methods like NMTree [63], the performance gains of LW-Transformer are up to 6.1% (on RefCOCO+), greatly showing the generalization ability of LW-Transformer. We also observe that its performance is very close to that of Transformer, and even slightly better on RefCOCO, which once again confirms our argument that Group-wise transformation can compress Transformer while still keeping its performance.
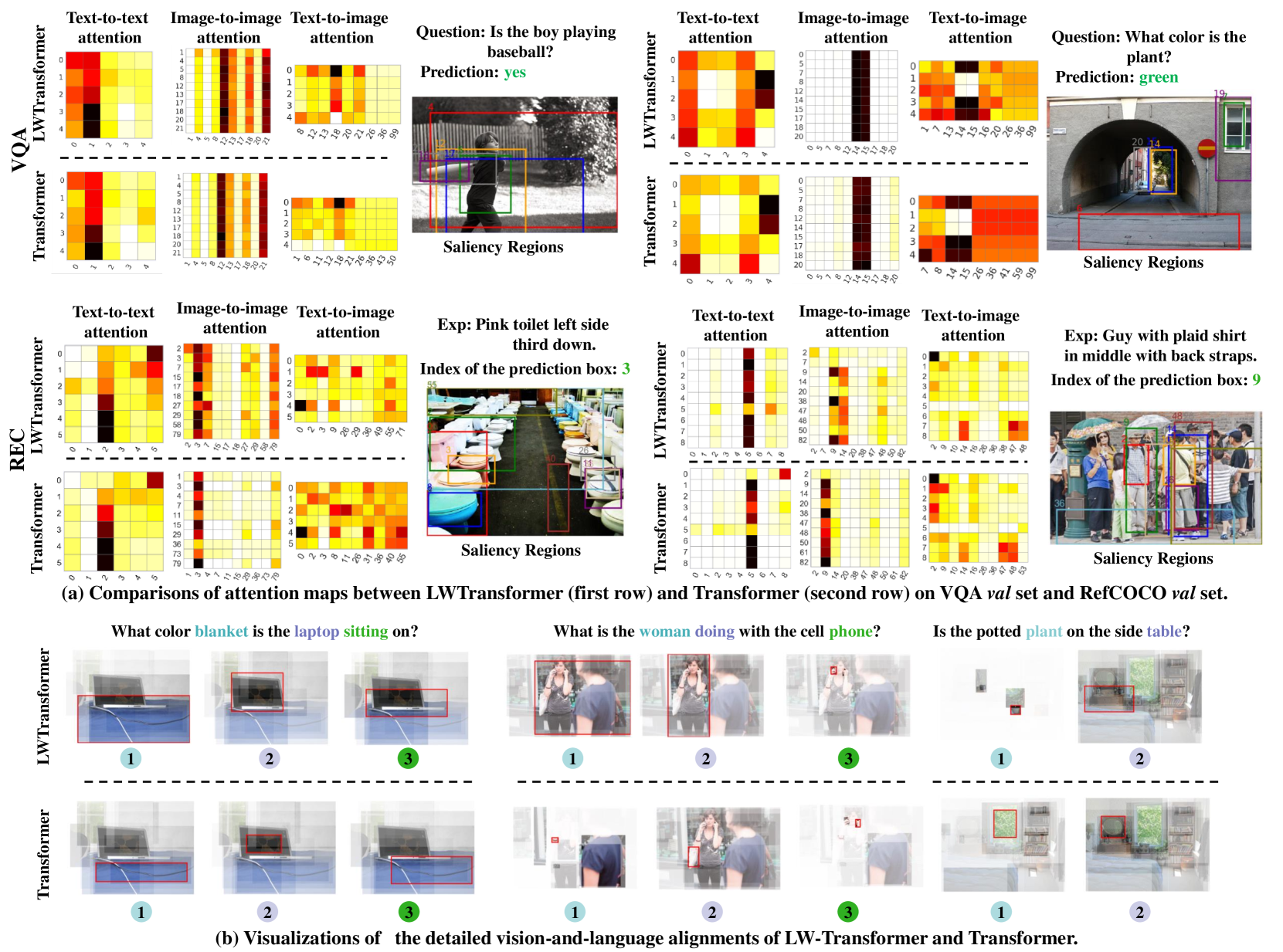
The comparison on the language-and-vision generative task, i.e., IC, is given in Tab. VIII . As shown in this table, LW-Transformer is an excellent generative model. Its performance is very close to Transformer, and even better on the metrics of CIDEr and SPICE. In addition, the parameter size of LW-Transformer is smaller than that of Transformer, i.e., 24 millions vs 33 millions. Considering M2 Transformer is already an efficient model, these improvements greatly suggest the effectiveness and efficiency of LW-Transformer. When compared to the SOTA method, i.e., X-Transformer, the advantage of LW-Transformer in efficiency becomes more prominent. Compared with X-Transformer, LW-Transformer has up to 79.0% fewer parameters, while the performance is only reduced by 1.12% on CIDEr.
IV-D5 Quantitative Results with the BERT-style Pre-training
We further apply our optimization strategy to a BERT-style model, i.e., LXMERT [19], and compare it with a set of methods pre-trained on the large-scale language-and-vision data. The result is given in Tab. XI. For simplicity, we denote the compressed LXMERT as LW-LXMERT. From this table, we first observe that after deploying G-MHA and G-FFN, the parameter size of LXMERT is reduced by up to 44.8%, while the performance is marginally reduced on VQA2.0 and even better on GQA. We notice that compared to some SOTA methods like 12in1 [17], the performance of LW-LXMERT on VQA2.0 is slightly worse. As mentioned about, VQA2.0 requires a larger model capacity due to the issue of language bias [41]. On GQA, examples of which are more balanced and more challenging, LW-LXMERT achieves new SOTA performance. Such results confirm again our argument that LW-Transformer can be applied to most Transformer-based networks, while maintaining their high performance.
IV-D6 Results of Image Classification
To further examine the generalization ability of LW-Transformer, we build it on the recently proposed Swin-Transformer [39] and conduct additional experiments on the task of image classification, of which results are given in Tab. IX. In this table, we report three LW-Transformer with different settings. The first two are LW-Transformer1× with different group numbers, and the last one is LW-Transformer1×-large, which has similar parameter size as Swin-Transformer by adding more Transformer layers.
From these results, we have some observations. Firstly, on Cifar-100, LW-Transformer1× hardly degrades the performance of Swin-Transformer. On top-5 accuracy, LW-Transformer1× even performs slightly better. These results are consistent with those in vision-and-language benchmarks. Secondly, on the large-scale ImageNet benchmark, LW-Transformer1× is slightly worse than Swin-Transformer. In this regard, our assumption is that ImageNet has a higher requirement for model capacity. Considering the saved experimental expenditure, e.g., saving 40.3% parameters and 42.2% MAdds when group number is 4, the performance drop (-2.8%) is still acceptable. This assumption is validated in the results of LW-Transformer-large. It improves Swin-Transformer on Cifar-100 and ImageNet with similar parameters and computations. Considering Swin-Transformer is carefully designed for image classification, these slightly improvements well validate the effectiveness of our method. Lastly, compared with Performer, LW-Transformer1× has all-round advantages. Retaining better performance, LW-Transformer1× merits in the parameter size and the computation cost, which greatly validates our motivation about the application of group-wise transformation. Overall, these results well support the generalization ability and efficiency of LW-Transformer for the traditional computer vision tasks.
IV-E Qualitative Experiment.
To gain deep insights into LW-Transformer, we visualize its attention maps and compare them with the ones of the default Transformer in Fig.2.
In Fig. 2 (a), we present the overall patterns of single- and multi-modal attentions learned by Transformer and the proposed LW-Transformer. From these visualizations, we can see that the global dependency patterns learned by two networks are roughly the same, which subsequently confirms the argument we made in this paper, i.e., group-wise transformation essentially inherits the principle of self-attention.
In addition to this observation, we capture some subtle differences between two networks and two multi-modal tasks. For example, the attention patterns of VQA and REC are slightly different. As shown in Fig. 2 (a), the words indicating question type will be more important in VQA, e.g., “is the” and “what color”. In contrast, REC requires the model to focus more on spatial information like “ left” or “ middle”. This result further reflects the difference of model reasoning between two tasks. That is, VQA relies more on the language prior of question types to answer the question, and REC locates the target instance based more on spatial information. Nevertheless, we find that these different attention patterns can be mastered by both LW-Transformer and Transformer.
In terms of vision-and-language alignment, the attention focus of LW-Transformer is more concentrated, while the one of Transformer is more divergent. For instance, in the second example of VQA, Transformer’s attention is flat, mainly focusing on the incorrect text-image relationships (4-7). In contrast, the attention of LW-Transformer is more accurate. These properties is also reflected in the visualizations of cross-modal attentions in Fig.2.b. From the examples of Fig. 2.b, we can see that LW-Transformer can obtain more precise “attention” than Transformer. Specifically, in cross-modal attention, Transformer and LW-Transformer focus on similar image regions. However, LW-Transformer will be more accurate in terms of the most attended regions (with red boxes), for example, the “phone” in the second example. This observation confirms the merits of LW-Transformer in vision-and-language alignment.
V Conclusion
In this paper, we introduce the Group-wise Transformation to achieve a lightweight yet general Transformer network, termed LW-Transformer. Compressing Transformer still retains challenging mainly due to its complex layer designs, i.e., MHA and FFN. The proposed LW-Transformer can well maintain their main principles, i.e., the efficient attention modelings on diverse subspaces and the expanding and scaling feature transformation, and reduce the parameter sizes and computation costs to a large extend. More importantly, the intention of group-wise transformation is consistent with MHA, which can ensure LW-Transformer to learn similar or even better attention patterns as the default Transformer. To validate our optimization strategy, we build LW-Transformer based on Transformer and a BERT-style model called LXMERT, and conduct extensive experiments on six benchmark datasets of three multi-modal tasks. The experimental results show that while saving up to 45% of parameters and 28% of computation costs, LW-Transformer can achieve almost or even better performance than Transformer on these datasets. Meanwhile, the generalization ability of LW-Transformer is also well validated on a newly proposed image Transformer called Swin-Transformer [39] for the task of image classification. These results greatly validate the effectiveness of LW-Transformer as well as our motivation.
Acknowledgment
This work is supported by the National Science Fund for Distinguished Young Scholars (No.62025603), the National Natural Science Foundation of China (No.U1705262, No. 62072386, No. 62072387, No. 62072389, No.62002305, No.61772443, No.61802324 and No.61702136), China Postdoctoral Science Foundation (2021T40397), Guangdong Basic and Applied Basic Research Foundation (No.2019B1515120049) and the Fundamental Research Funds for the central universities (No. 20720200077, No. 20720200090 and No. 20720200091).
References
- [1] A. Vaswani, N. Shazeer, N. Parmar, J. Uszkoreit, L. Jones, A. N. Gomez, Ł. Kaiser, and I. Polosukhin, “Attention is all you need,” in NeurIPS, 2017, pp. 5998–6008.
- [2] Z. Yu, J. Yu, Y. Cui, D. Tao, and Q. Tian, “Deep modular co-attention networks for visual question answering,” in CVPR, 2019, pp. 6281–6290.
- [3] L. Huang, W. Wang, J. Chen, and X.-Y. Wei, “Attention on attention for image captioning,” in Proceedings of the IEEE International Conference on Computer Vision, 2019, pp. 4634–4643.
- [4] Z. Yu, Y. Cui, J. Yu, D. Tao, and Q. Tian, “Multimodal unified attention networks for vision-and-language interactions,” arXiv preprint arXiv:1908.04107, 2019.
- [5] N. Carion, F. Massa, G. Synnaeve, N. Usunier, A. Kirillov, and S. Zagoruyko, “End-to-end object detection with transformers,” arXiv preprint arXiv:2005.12872, 2020.
- [6] P. Gao, Z. Jiang, H. You, P. Lu, S. C. Hoi, X. Wang, and H. Li, “Dynamic fusion with intra-and inter-modality attention flow for visual question answering,” in CVPR, 2019, pp. 6639–6648.
- [7] P. Gao, H. You, Z. Zhang, X. Wang, and H. Li, “Multi-modality latent interaction network for visual question answering,” in Proceedings of the IEEE International Conference on Computer Vision, 2019, pp. 5825–5835.
- [8] M. Cornia, M. Stefanini, L. Baraldi, and R. Cucchiara, “Meshed-Memory Transformer for Image Captioning,” in Proceedings of the IEEE/CVF Conference on Computer Vision and Pattern Recognition, 2020.
- [9] S. Herdade, A. Kappeler, K. Boakye, and J. Soares, “Image captioning: Transforming objects into words,” in Advances in Neural Information Processing Systems, 2019, pp. 11 135–11 145.
- [10] G. Li, L. Zhu, P. Liu, and Y. Yang, “Entangled transformer for image captioning,” in Proceedings of the IEEE International Conference on Computer Vision, 2019, pp. 8928–8937.
- [11] Z. Yu, Y. Cui, J. Yu, M. Wang, D. Tao, and Q. Tian, “Deep multimodal neural architecture search,” arXiv preprint arXiv:2004.12070, 2020.
- [12] C. Alberti, J. Ling, M. Collins, and D. Reitter, “Fusion of detected objects in text for visual question answering,” arXiv preprint arXiv:1908.05054, 2019.
- [13] J. Devlin, M.-W. Chang, K. Lee, and K. Toutanova, “Bert: Pre-training of deep bidirectional transformers for language understanding,” arXiv preprint arXiv:1810.04805, 2018.
- [14] G. Li, N. Duan, Y. Fang, D. Jiang, and M. Zhou, “Unicoder-vl: A universal encoder for vision and language by cross-modal pre-training,” arXiv preprint arXiv:1908.06066, 2019.
- [15] L. H. Li, M. Yatskar, D. Yin, C.-J. Hsieh, and K.-W. Chang, “Visualbert: A simple and performant baseline for vision and language,” arXiv preprint arXiv:1908.03557, 2019.
- [16] J. Lu, D. Batra, D. Parikh, and S. Lee, “Vilbert: Pretraining task-agnostic visiolinguistic representations for vision-and-language tasks,” in Advances in Neural Information Processing Systems, 2019, pp. 13–23.
- [17] J. Lu, V. Goswami, M. Rohrbach, D. Parikh, and S. Lee, “12-in-1: Multi-task vision and language representation learning,” 2019.
- [18] W. Su, X. Zhu, Y. Cao, B. Li, L. Lu, F. Wei, and J. Dai, “Vl-bert: Pre-training of generic visual-linguistic representations,” arXiv preprint arXiv:1908.08530, 2019.
- [19] H. Tan and M. Bansal, “Lxmert: Learning cross-modality encoder representations from transformers,” arXiv preprint arXiv:1908.07490, 2019.
- [20] Z. Yang, Z. Dai, Y. Yang, J. Carbonell, R. R. Salakhutdinov, and Q. V. Le, “Xlnet: Generalized autoregressive pretraining for language understanding,” in Advances in neural information processing systems, 2019, pp. 5754–5764.
- [21] L. Zhou, H. Palangi, L. Zhang, H. Hu, J. J. Corso, and J. Gao, “Unified vision-language pre-training for image captioning and vqa,” arXiv preprint arXiv:1909.11059, 2019.
- [22] K. He, X. Zhang, S. Ren, and J. Sun, “Deep residual learning for image recognition,” in Proceedings of the IEEE conference on computer vision and pattern recognition, 2016, pp. 770–778.
- [23] S. Ren, K. He, R. Girshick, and J. Sun, “Faster r-cnn: Towards real-time object detection with region proposal networks,” in Advances in neural information processing systems, 2015, pp. 91–99.
- [24] A. Fan, E. Grave, and A. Joulin, “Reducing transformer depth on demand with structured dropout,” 2019.
- [25] V. Sanh, L. Debut, J. Chaumond, and T. Wolf, “Distilbert, a distilled version of bert: smaller, faster, cheaper and lighter,” arXiv preprint arXiv:1910.01108, 2019.
- [26] S. Shen, Z. Dong, J. Ye, L. Ma, Z. Yao, A. Gholami, M. W. Mahoney, and K. Keutzer, “Q-bert: Hessian based ultra low precision quantization of bert,” arXiv preprint arXiv:1909.05840, 2019.
- [27] J. Y. Tian, A. P. Kreuzer, P.-H. Chen, and H.-M. Will, “Waldorf: Wasteless language-model distillation on reading-comprehension,” arXiv preprint arXiv:1912.06638, 2019.
- [28] O. Zafrir, G. Boudoukh, P. Izsak, and M. Wasserblat, “Q8bert: Quantized 8bit bert,” arXiv preprint arXiv:1910.06188, 2019.
- [29] X. Ma, P. Zhang, S. Zhang, N. Duan, Y. Hou, M. Zhou, and D. Song, “A tensorized transformer for language modeling,” in NeurIPS, 2019, pp. 2229–2239.
- [30] K. M. Choromanski, V. Likhosherstov, D. Dohan, X. Song, A. Gane, T. Sarlos, P. Hawkins, J. Q. Davis, A. Mohiuddin, L. Kaiser et al., “Rethinking attention with performers,” in International Conference on Learning Representations, 2020.
- [31] S. Wang, B. Z. Li, M. Khabsa, H. Fang, and H. Ma, “Linformer: Self-attention with linear complexity,” arXiv preprint arXiv:2006.04768, 2020.
- [32] N. Kitaev, L. Kaiser, and A. Levskaya, “Reformer: The efficient transformer,” in International Conference on Learning Representations, 2019.
- [33] F. Chollet, “Xception: Deep learning with depthwise separable convolutions,” in CVPR, 2017, pp. 1251–1258.
- [34] A. Krizhevsky, I. Sutskever, and G. E. Hinton, “Imagenet classification with deep convolutional neural networks,” in Advances in neural information processing systems, 2012, pp. 1097–1105.
- [35] M. Sandler, A. Howard, M. Zhu, A. Zhmoginov, and L.-C. Chen, “Mobilenetv2: Inverted residuals and linear bottlenecks,” in CVPR, 2018, pp. 4510–4520.
- [36] C. Szegedy, S. Ioffe, V. Vanhoucke, and A. A. Alemi, “Inception-v4, inception-resnet and the impact of residual connections on learning,” in Thirty-first AAAI conference on artificial intelligence, 2017.
- [37] C. Szegedy, V. Vanhoucke, S. Ioffe, J. Shlens, and Z. Wojna, “Rethinking the inception architecture for computer vision,” in Proceedings of the IEEE conference on computer vision and pattern recognition, 2016, pp. 2818–2826.
- [38] X. Zhang, X. Zhou, M. Lin, and J. Sun, “Shufflenet: An extremely efficient convolutional neural network for mobile devices,” in CVPR, 2018, pp. 6848–6856.
- [39] Z. Liu, Y. Lin, Y. Cao, H. Hu, Y. Wei, Z. Zhang, S. Lin, and B. Guo, “Swin transformer: Hierarchical vision transformer using shifted windows,” arXiv preprint arXiv:2103.14030, 2021.
- [40] D. A. Hudson and C. D. Manning, “Gqa: A new dataset for real-world visual reasoning and compositional question answering,” in CVPR, 2019, pp. 6700–6709.
- [41] Y. Goyal, T. Khot, D. Summers-Stay, D. Batra, and D. Parikh, “Making the v in vqa matter: Elevating the role of image understanding in visual question answering,” in Proceedings of the IEEE Conference on Computer Vision and Pattern Recognition, 2017, pp. 6904–6913.
- [42] J. Johnson, B. Hariharan, L. van der Maaten, L. Fei-Fei, C. Lawrence Zitnick, and R. Girshick, “Clevr: A diagnostic dataset for compositional language and elementary visual reasoning,” in CVPR, 2017, pp. 2901–2910.
- [43] X. Chen, H. Fang, T.-Y. Lin, R. Vedantam, S. Gupta, P. Dollár, and C. L. Zitnick, “Microsoft coco captions: Data collection and evaluation server,” arXiv preprint arXiv:1504.00325, 2015.
- [44] L. Yu, P. Poirson, S. Yang, A. C. Berg, and T. L. Berg, “Modeling context in referring expressions,” in European Conference on Computer Vision. Springer, 2016, pp. 69–85.
- [45] S. Kazemzadeh, V. Ordonez, M. Matten, and T. Berg, “Referitgame: Referring to objects in photographs of natural scenes,” in EMNLP, 2014, pp. 787–798.
- [46] D. R. So, C. Liang, and Q. V. Le, “The evolved transformer,” arXiv preprint arXiv:1901.11117, 2019.
- [47] S. Sukhbaatar, E. Grave, P. Bojanowski, and A. Joulin, “Adaptive attention span in transformers,” arXiv preprint arXiv:1905.07799, 2019.
- [48] Y. Zhou, R. Ji, X. Sun, G. Luo, X. Hong, J. Su, X. Ding, and L. Shao, “K-armed bandit based multi-modal network architecture search for visual question answering,” in Proceedings of the 28th ACM International Conference on Multimedia, 2020, pp. 1245–1254.
- [49] Y. Pan, T. Yao, Y. Li, and T. Mei, “X-linear attention networks for image captioning,” in Proceedings of the IEEE/CVF Conference on Computer Vision and Pattern Recognition, 2020, pp. 10 971–10 980.
- [50] X. Liu, H. Li, J. Shao, D. Chen, and X. Wang, “Show, tell and discriminate: Image captioning by self-retrieval with partially labeled data,” in Proceedings of the European Conference on Computer Vision (ECCV), 2018, pp. 338–354.
- [51] S. Liu, Z. Zhu, N. Ye, S. Guadarrama, and K. Murphy, “Improved image captioning via policy gradient optimization of spider,” in Proceedings of the IEEE international conference on computer vision, 2017, pp. 873–881.
- [52] T. Yao, Y. Pan, Y. Li, and T. Mei, “Hierarchy parsing for image captioning,” in Proceedings of the IEEE/CVF International Conference on Computer Vision, 2019, pp. 2621–2629.
- [53] J. Ji, Y. Luo, X. Sun, F. Chen, G. Luo, Y. Wu, Y. Gao, and R. Ji, “Improving image captioning by leveraging intra-and inter-layer global representation in transformer network,” 2021.
- [54] A. Fukui, D. H. Park, D. Yang, A. Rohrbach, T. Darrell, and M. Rohrbach, “Multimodal compact bilinear pooling for visual question answering and visual grounding,” in Proceedings of the 2016 Conference on Empirical Methods in Natural Language Processing, 2016, pp. 457–468.
- [55] J. Hu, L. Shen, and G. Sun, “Squeeze-and-excitation networks,” in Proceedings of the IEEE conference on computer vision and pattern recognition, 2018, pp. 7132–7141.
- [56] R. Vedantam, C. Lawrence Zitnick, and D. Parikh, “Cider: Consensus-based image description evaluation,” in Proceedings of the IEEE conference on computer vision and pattern recognition, 2015, pp. 4566–4575.
- [57] Y. Li, Y. Pan, T. Yao, J. Chen, and T. Mei, “Scheduled sampling in vision-language pretraining with decoupled encoder-decoder network,” arXiv preprint arXiv:2101.11562, 2021.
- [58] S. Antol, A. Agrawal, J. Lu, M. Mitchell, D. Batra, C. Lawrence Zitnick, and D. Parikh, “Vqa: Visual question answering,” in Proceedings of the IEEE international conference on computer vision, 2015, pp. 2425–2433.
- [59] A. El-Nouby, H. Touvron, M. Caron, P. Bojanowski, M. Douze, A. Joulin, I. Laptev, N. Neverova, G. Synnaeve, J. Verbeek et al., “Xcit: Cross-covariance image transformers,” arXiv preprint arXiv:2106.09681, 2021.
- [60] A. G. Howard, M. Zhu, B. Chen, D. Kalenichenko, W. Wang, T. Weyand, M. Andreetto, and H. Adam, “Mobilenets: Efficient convolutional neural networks for mobile vision applications,” arXiv preprint arXiv:1704.04861, 2017.
- [61] R. Hu, M. Rohrbach, J. Andreas, T. Darrell, and K. Saenko, “Modeling relationships in referential expressions with compositional modular networks,” in Proceedings of the IEEE Conference on Computer Vision and Pattern Recognition, 2017, pp. 1115–1124.
- [62] R. Hu, H. Xu, M. Rohrbach, J. Feng, K. Saenko, and T. Darrell, “Natural language object retrieval,” in Proceedings of the IEEE Conference on Computer Vision and Pattern Recognition, 2016, pp. 4555–4564.
- [63] D. Liu, H. Zhang, F. Wu, and Z.-J. Zha, “Learning to assemble neural module tree networks for visual grounding,” in Proceedings of the IEEE International Conference on Computer Vision, 2019, pp. 4673–4682.
- [64] J. Liu, L. Wang, and M.-H. Yang, “Referring expression generation and comprehension via attributes,” in Proceedings of the IEEE International Conference on Computer Vision, 2017, pp. 4856–4864.
- [65] L. Yu, Z. Lin, X. Shen, J. Yang, X. Lu, M. Bansal, and T. L. Berg, “Mattnet: Modular attention network for referring expression comprehension,” in Proceedings of the IEEE Conference on Computer Vision and Pattern Recognition, 2018, pp. 1307–1315.
- [66] Z. Yu, J. Yu, C. Xiang, Z. Zhao, Q. Tian, and D. Tao, “Rethinking diversified and discriminative proposal generation for visual grounding,” in Proceedings of the 27th International Joint Conference on Artificial Intelligence, 2018, pp. 1114–1120.
- [67] G. Luo, Y. Zhou, X. Sun, L. Cao, C. Wu, C. Deng, and R. Ji, “Multi-task collaborative network for joint referring expression comprehension and segmentation,” in Proceedings of the IEEE/CVF Conference on Computer Vision and Pattern Recognition, 2020, pp. 10 034–10 043.
- [68] Y. Zhou, R. Ji, G. Luo, X. Sun, J. Su, X. Ding, C.-W. Lin, and Q. Tian, “A real-time global inference network for one-stage referring expression comprehension,” IEEE Transactions on Neural Networks and Learning Systems, 2021.
- [69] A. Karpathy and L. Fei-Fei, “Deep visual-semantic alignments for generating image descriptions,” in Proceedings of the IEEE conference on computer vision and pattern recognition, 2015, pp. 3128–3137.
- [70] K. Papineni, S. Roukos, T. Ward, and W.-J. Zhu, “Bleu: a method for automatic evaluation of machine translation,” in Proceedings of the 40th annual meeting on association for computational linguistics. Association for Computational Linguistics, 2002, pp. 311–318.
- [71] S. Banerjee and A. Lavie, “Meteor: An automatic metric for mt evaluation with improved correlation with human judgments,” in Proceedings of the acl workshop on intrinsic and extrinsic evaluation measures for machine translation and/or summarization, 2005, pp. 65–72.
- [72] C.-Y. Lin, “Rouge: A package for automatic evaluation of summaries,” in ACL Workshop. Springer, 2004, pp. 382–398.
- [73] P. Anderson, B. Fernando, M. Johnson, and S. Gould, “Spice: Semantic propositional image caption evaluation,” in European Conference on Computer Vision. Springer, 2016, pp. 382–398.
- [74] J. Deng, W. Dong, R. Socher, L.-J. Li, K. Li, and L. Fei-Fei, “Imagenet: A large-scale hierarchical image database,” in 2009 IEEE conference on computer vision and pattern recognition. Ieee, 2009, pp. 248–255.
- [75] A. Krizhevsky, G. Hinton et al., “Learning multiple layers of features from tiny images,” 2009.
- [76] H. Ben-Younes, R. Cadene, M. Cord, and N. Thome, “Mutan: Multimodal tucker fusion for visual question answering,” in Proceedings of the IEEE international conference on computer vision, 2017, pp. 2612–2620.
- [77] J.-H. Kim, K.-W. On, W. Lim, J. Kim, J.-W. Ha, and B.-T. Zhang, “Hadamard product for low-rank bilinear pooling,” arXiv preprint arXiv:1610.04325, 2016.
- [78] Z. Yu, J. Yu, J. Fan, and D. Tao, “Multi-modal factorized bilinear pooling with co-attention learning for visual question answering,” in Proceedings of the IEEE international conference on computer vision, 2017, pp. 1821–1830.
- [79] Z. Yu, J. Yu, C. Xiang, J. Fan, and D. Tao, “Beyond bilinear: Generalized multimodal factorized high-order pooling for visual question answering,” in NeurIPS, 2018, pp. 5947–5959.
- [80] H. Ben-Younes, R. Cadene, N. Thome, and M. Cord, “Block: Bilinear superdiagonal fusion for visual question answering and visual relationship detection,” in Proceedings of the AAAI Conference on Artificial Intelligence, vol. 33, 2019, pp. 8102–8109.
- [81] P. Anderson, X. He, C. Buehler, D. Teney, M. Johnson, S. Gould, and L. Zhang, “Bottom-up and top-down attention for image captioning and visual question answering,” in CVPR, 2018, pp. 6077–6086.
- [82] D. A. Hudson and C. D. Manning, “Compositional attention networks for machine reasoning,” in ICLR, 2018.
- [83] E. Perez, F. Strub, H. De Vries, V. Dumoulin, and A. Courville, “Film: Visual reasoning with a general conditioning layer,” in Thirty-Second AAAI Conference on Artificial Intelligence, 2018.
- [84] A. Santoro, D. Raposo, D. G. Barrett, M. Malinowski, R. Pascanu, P. Battaglia, and T. Lillicrap, “A simple neural network module for relational reasoning,” in Advances in neural information processing systems, 2017, pp. 4967–4976.
- [85] R. Hu, A. Rohrbach, T. Darrell, and K. Saenko, “Language-conditioned graph networks for relational reasoning,” in Proceedings of the IEEE International Conference on Computer Vision, 2019, pp. 10 294–10 303.
- [86] D. P. Kingma and J. Ba, “Adam: A method for stochastic optimization,” arXiv preprint arXiv:1412.6980, 2014.
- [87] R. Krishna, Y. Zhu, O. Groth, J. Johnson, K. Hata, J. Kravitz, S. Chen, Y. Kalantidis, L.-J. Li, D. A. Shamma et al., “Visual genome: Connecting language and vision using crowdsourced dense image annotations,” International Journal of Computer Vision, vol. 123, no. 1, pp. 32–73, 2017.
- [88] J.-H. Kim, J. Jun, and B.-T. Zhang, “Bilinear attention networks,” in Advances in Neural Information Processing Systems, 2018, pp. 1564–1574.
- [89] L. Yu, H. Tan, M. Bansal, and T. L. Berg, “A joint speaker-listener-reinforcer model for referring expressions,” in Proceedings of the IEEE Conference on Computer Vision and Pattern Recognition, 2017, pp. 7282–7290.
- [90] C. Chelba, T. Mikolov, M. Schuster, Q. Ge, T. Brants, P. Koehn, and T. Robinson, “One billion word benchmark for measuring progress in statistical language modeling,” arXiv preprint arXiv:1312.3005, 2013.
- [91] N. Parmar, A. Vaswani, J. Uszkoreit, Ł. Kaiser, N. Shazeer, A. Ku, and D. Tran, “Image transformer,” arXiv preprint arXiv:1802.05751, 2018.
![]() |
Gen Luo is currently pursuing the phd’s degree in Xiamen University. His research interests include vision-and-language interactions. |
![]() |
Yiyi Zhou received his Ph.D. degree supervised by Prof. Rongrong Ji from Xiamen University, Chian, in 2019. He is a Post-doctoral Research Fellow of the School of Informatics and a member of Media Analytics and Computing (MAC) lab of Xiamen University, China. |
![]() |
Xiaoshuai Sun (Senior Member, IEEE) received the B.S. degree in computer science from Harbin Engineering University, Harbin, China, in 2007, and the M.S. and Ph.D. degrees in computer science and technology from the Harbin Institute of Technology, Harbin, in 2009 and 2015, respectively. He was a Postdoctoral Research Fellow with the University of Queensland from 2015 to 2016. He served as a Lecturer with the Harbin Institute of Technology from 2016 to 2018. He is currently an Associate Professor with Xiamen University, China. He was a recipient of the Microsoft Research Asia Fellowship in 2011. |
![]() |
Yan Wang got his PhD degree in Oct. 2015, under supervision of Prof. Shih-Fu Chang. He had rich R&D experience in Adobe Research, Microsoft Research, and Facebook, with his algorithms integrated in Facebook Graph Search and Adobe Photoshop, and granted patents. He held the Olympic Torch of Beijing Olympic Games as a torchbearer in 2008, won the Tech Draft (a nation-wide programming challenge) in 2014, and is a certified airplane pilot. His current research interests include computer vision and machine learning. |
![]() |
Liujuan Cao received her Bachelor’s, Master’s, and Ph.D. degrees from the School of Computer Science and Technology, Harbin Engineering University, Harbin, China. She was a Visiting Researcher with Columbia University from 2012 to 2013. She joined Xiamen University in 2014. She is currently an Associate Professor with the School of Informatics, Xiamen University, Xiamen, China. She has published more than 30 papers in the top and major tiered journals and conferences, including IEEE CVPR, Information Sciences, Neurocomputing, Signal Processing, Digital Signal Processing, etc. Her research interests include covers pattern recognition, data mining, and computer vision. She is the Finance Chair of IEEE MMSP 2015. She has been project PI for various projects including NSFC, military projects, with over 1M RMB fundings. |
![]() |
Yongjian Wu received his master’s degree in computer science from Wuhan University, China, in 2008. He is currently the expert researcher and the director of the Youtu Lab, Tencent Co., Ltd. His research interests include face recognition, image understanding, and large scale data processing. |
![]() |
Feiyue Huang received his B.S. and Ph.D. degrees in computer science from Tsinghua University, China, in 2001 and 2008, respectively. He is the expert researcher and the director of the Tencent Youtu Lab. His research interests include image understanding and face recognition. |
![]() |
Rongrong Ji (Senior Member, IEEE) is currently a Professor and the Director of the Intelligent Multimedia Technology Laboratory, School of Informatics, Xiamen University, Xiamen, China. His work mainly focuses on innovative technologies for multimedia signal processing, computer vision, and pattern recognition, with over 100 papers published in international journals and conferences. He is a member of the ACM. He also serves as a program committee member for several Tier-1 international conferences. He was a recipient of the ACM Multimedia Best Paper Award and the Best Thesis Award of Harbin Institute of Technology. He serves as an Associate/Guest Editor for international journals and magazines, such as Neurocomputing, Signal Processing, Multimedia Tools and Applications, the IEEE Multimedia Magazine, and Multimedia Systems. |