To BERT or Not to BERT: Comparing Task-specific and Task-agnostic Semi-Supervised Approaches for Sequence Tagging
Abstract
Leveraging large amounts of unlabeled data using Transformer-like architectures, like BERT, has gained popularity in recent times owing to their effectiveness in learning general representations that can then be further fine-tuned for downstream tasks to much success. However, training these models can be costly both from an economic and environmental standpoint. In this work, we investigate how to effectively use unlabeled data: by exploring the task-specific semi-supervised approach, Cross-View Training (CVT) and comparing it with task-agnostic BERT in multiple settings that include domain and task relevant English data. CVT uses a much lighter model architecture and we show that it achieves similar performance to BERT on a set of sequence tagging tasks, with lesser financial and environmental impact.
1 Introduction
Exploiting unlabeled data to improve performance has become the foundation for many natural language processing tasks. The question we investigate in this paper is how to effectively use unlabeled data: in a task-agnostic or a task-specific way. An example of the former is training models on language model (LM) like objectives on a large unlabeled corpus to learn general representations, as in ELMo (Embeddings from Language Models) Peters et al. (2018) and BERT (Bidirectional Encoder Representations from Transformers) Devlin et al. (2019). These are then reused in supervised training on a downstream task. These pre-trained models, particularly the ones based on the Transformer architecture Vaswani et al. (2017)111Not only BERT, but other models like RoBERTa Liu et al. (2019b) and BART Lewis et al. (2019) have achieved state-of-the-art results in a variety of NLP tasks, but come at a great cost financially and environmentally Strubell et al. (2019); Schwartz et al. (2019).
In contrast, Cross-View Training (CVT) Clark et al. (2018) is a semi-supervised approach that uses unlabeled data in a task-specific manner, rather than trying to learn general representations that can be used for many downstream tasks. Inspired by self-learning McClosky et al. (2006); Yarowsky (1995) and multi-view learning Blum and Mitchell (1998); Xu et al. (2013), the key idea is that the primary prediction module, which has an unrestricted view of the data, trains on the task using labeled examples, and makes task-specific predictions on unlabeled data. The auxiliary modules, with restricted views of the unlabeled data, attempt to replicate the primary module predictions. This helps to learn better representations for the task.
We present an experimental study that investigates different task-agnostic and task-specific approaches to use unsupervised data and evaluates them in terms of performance as well as financial and environmental impact. On the one hand, we use BERT in three different settings: 1) standard BERT setup in which BERT pretrained on a generic corpus is fine-tuned on a supervised task; 2) pre-training BERT on domain and/or task relevant unlabeled data and fine-tuning on a supervised task (Pretrained BERT); and 3) continued pretraining of BERT on domain and/or task relevant unlabeled data followed by fine-tuning on a supervised task (Adaptively Pretrained BERT) Gururangan et al. (2020). On the other hand, we use CVT based on a much lighter architecture (CNN-BiLSTM) which uses domain and/or task relevant unlabeled data in a task-specific manner. We experiment on several tasks framed as a sequence labeling problem: opinion target expression detection, named entity recognition and slot-labeling. We find that the CVT-based approach using less unlabeled data achieves similar performance with BERT-based models, while being superior in terms of financial and environmental cost as well.
2 Background, Tasks and Datasets
Task | Labeled Data | Unlabeled Data |
---|---|---|
OTE | SE16-R Train: 2000; Test: 676 | Yelp-R: 32.5M |
SE14-L Train: 3045; Test: 800 | Amazon-E: 95M | |
NER | CONLL-2003 Train: 14987; Test: 3684 | CNN-DM: 4M |
CONLL-2012 Train: 59924; Test: 8262 | ||
Slot-labeling | MIT-Movies Train: 9775; Test: 2443 | IMDb: 271K |
Before presenting the models and their training setups, we discuss the relevant literature and introduce the tasks and datasets used for our experiments. We focus on three tasks: opinion target expression (OTE) detection; named entity recognition (NER), and slot-labeling, each of which can be modeled as a sequence tagging problem Xu et al. (2018); Liu et al. (2019a); Louvan and Magnini (2018). The IOB sequence tagging scheme Ramshaw and Marcus (1999) is used for each of these tasks.
Related Work. The usefulness of continued training of large transformer-based models on domain/task-related unlabeled data has been shown recently Gururangan et al. (2020); Rietzler et al. (2019); Xu et al. (2019), with a varied use of terminology for the process. Xu et al. (2019) and Rietzler et al. (2019) show gains of further tuning BERT using in-domain unlabeled data and refer to this as Post-training, and LM finetuning, respectively. More recently, Gururangan et al. (2020) use the term Domain-Adaptive Pretraining and show benefits over RoBERTa Liu et al. (2019b). There have also been efforts to reduce model sizes for BERT, such as DistilBERT Sanh et al. (2019), although these come at significant losses in performance.
Opinion Target Expression (OTE) Detection:
An integral component of fine-grained sentiment analysis is the ability to identify segments of text towards which opinions are expressed. These segments are referred to as Opinion Target Expressions or OTEs. An example of this task is provided in Figure 1. The commonly used labeled datasets for Opinion Target Expression (OTE) detection are those released as part of SemEval Aspect-based Sentiment shared tasks: SemEval-2014 Laptops Pontiki et al. (2014) (SE14-L) and SemEval-2016 Restaurants Pontiki et al. (2016) (SE16-R). These consist of reviews from the laptop and restaurant domains, respectively, with OTEs annotated for each sentence of a review. We use the provided train-test splits but further split the training data randomly into 90% training and 10% validation sets. As unlabeled data that is similar to the domain and task, we extract restaurant reviews from the Yelp222https://www.yelp.com/dataset dataset (Yelp-R) and reviews of electronics products from Amazon Product Reviews dataset333http://jmcauley.ucsd.edu/data/amazon/ (Amazon-E) (see Table 1).



Named Entity Recognition (NER):
NER is the task of identifying and categorizing named entities from unstructured text into pre-defined categories such as Person (PER), Location (LOC), Organization (ORG) etc. Figure 1 contains an example of this task. CONLL-2003 Tjong Kim Sang and De Meulder (2003) and CONLL-2012 (OntoNotes v5.0) Pradhan et al. (2012) are the commonly used labeled datasets to build and evaluate performance for Named Entity Recognition models (Lample et al., 2016; Ma and Hovy, 2016; Akbik et al., 2018, inter-alia). We focus on the English parts of these datasets. CONLL-2003 contains annotations for Reuters news for 4 entity types (Person, Location, Organization, and Miscellaneous). CONLL-2012 dataset contains 18 entity types, consisting of various genres (weblogs, news, talk shows, etc.) with newswire being majority. We use the provided train, validation and test splits for these datasets. As newswire is the predominant genre in these datasets, as we use stories from the CNN and Daily Mail datasets444https://github.com/abisee/cnn-dailymail (CNN-DM) as an unlabeled dataset from the news genre (see Table 1).
Slot-labeling:
Slot-labeling is a key component of Natural Language Understanding (NLU) in dialogue systems, which involves labeling words of an utterance with pre-defined attributes - slots. For this task, we use the widely-used MIT-Movie dataset555https://groups.csail.mit.edu/sls/downloads/movie/ as labeled data which contains queries related to movie information, with 12 slot labels such as Plot, Actor, Director, etc.. An example from this dataset is demonstrated in Figure 1. We use the default train-test split, and create a validation set by randomly selecting 10% of the training samples. IMDb Movie review dataset (IMDb) is used as in-domain unlabeled data Maas et al. (2011) (see Table 1).
3 Models and Experimental Setup
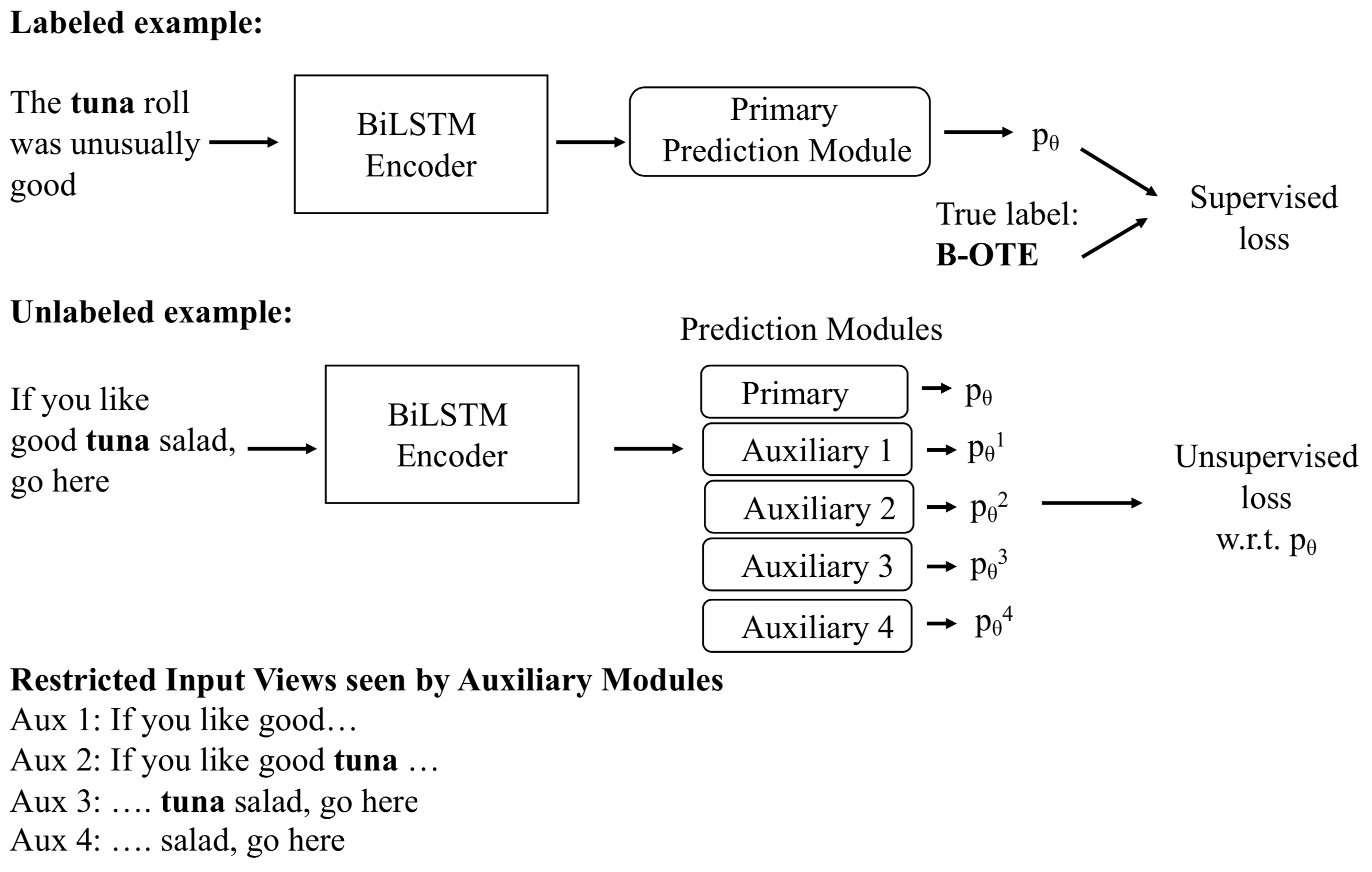
Results for SemEval2016 Restaurants Dataset
Model
Unlabeled Data
Mean F1
CVT
Yelp-R (25.6M)
80.080.18
BERTBase
Wiki+Books (192M)
75.041.00
Pre-BERTBase
Yelp-R (261M)
79.820.22
APBERTBase
Yelp-R (246M)
80.28 0.29
DE-CNN
Yelp-R (-)
74.37
Results for SemEval2014 Laptops Dataset
Model
Unlabeled Data
Mean F1
CVT
Amazon-E (25.6M)
81.770.24
BERTBase
Wiki+Books(192M)
80.690.51
Pre-BERTBase
Amazon-E (261M)
83.980.42
APBERTBase
Amazon-E (238M)
84.460.9
DE-CNN
Amazon-L (-)
81.59
We describe the various models we compare in this work and the experimental setup for each of them. Experiments are geared towards comparing the performance accuracy of the models, while also measuring impact on the environment and the resources required for training these models. Details on model architecture and training are provided in Appendix A.
Cross-View Training (CVT)
CVT is a semi-supervised approach proposed by Clark et al. (2018) that leverages unlabeled data in a task-specific manner. The underlying model is a two-layer CNN-BiLSTM sentence encoder followed by a linear layer and a softmax per prediction module. There are two kinds of prediction modules - primary and auxiliary. CVT alternates between learning from labeled and unlabeled data during training. The key idea is that the primary prediction module, which has an unrestricted view of the data, trains on the task using labeled examples, and makes task-specific predictions on unlabeled data. The auxiliary modules, with different restricted views of the unlabeled data, attempt to mimic the predictions of the primary module. Standard cross-entropy loss is minimized when learning from labeled examples, while for unlabeled examples, KL-Divergence Kullback and Leibler (1951) between the predicted primary and auxiliary probability distributions is minimized (see Clark et al. (2018) for more details). We demonstrate the training strategy in Figure 2. Thus, the model is trained to produce consistent results despite seeing partial views of the input - thereby improving underlying representations.
We use Glove 840B.300d embeddings Pennington et al. (2014) instead of Glove 6B.300d embeddings used by the authors for a larger vocabulary coverage. For each of the labeled datasets (Section 2), we use the corresponding domain/task-relevant unlabeled data to train a sequence tagging model for 400K steps, with early stopping enabled using validation set convergence.
BERTBase
BERT Devlin et al. (2019) has achieved state-of-the-art results on many NLP tasks. The key innovation lies in the use of bi-directional Transformers as well as the Masked Language Model (MLM) and Next Sentence Prediction (NSP) objectives used during training. Learning happens in two steps: 1) training the model on a very large generic dataset (using the two objectives above); 2) fine-tuning the learned representations on a downstream task in a supervised fashion. For our experiments we use BERTBase, which has 12 layers, 768 hidden dimensions per token and 12 attention heads and is pre-trained on the cased English Wikipedia and Books Corpus data (Wiki+Books). In order to fine-tune on the downstream sequence tagging task, the model we use consists of the BERTBase encoder, followed by a dropout layer and a classification layer that classifies each token into B-label, I-label, O, where . Cross-entropy loss is the loss function used.
Pretrained BERTBase (Pre-BERTBase)
In this setup, we use BERTBase architecture and pre-train it from scratch on the domain/task relevant unlabeled data. Each training step trains on a batch of size 256. A validation set is created from each unlabeled dataset by random sampling (details in Appendix A). The convergence criteria is set to be validation MLM accuracy improvement 0.05 when evaluated every 30K steps. We then perform the second step of fine-tuning on the downstream task data, as in the regular BERT setup.
Adaptively Pretrained BERTBase(APBERTBase)
Here, we start with BERTBase trained on the generic unlabeled dataset (English Wikipedia and Book Corpus) and continue pretraining on the corresponding domain/task-relevant unlabeled data (Section 2). Inspired by the nomenclature in Gururangan et al. (2020), we refer to this model as Adaptively Pretrained BERTBase. Further, we perform fine-tuning on the downstream task data, as with the previous BERT models.
Results on CONLL-2003 dataset
Model
Unlabeled Data
Mean F1
CVT
CNN-DM (17M)
92.260.11
BERTBase
Wiki+Books (192M)
91.220.21
Pre-BERTBase
CNN-DM (146M)
85.540.19
APBERTBase
CNN-DM (138M)
88.020.18
Cloze
Wiki+Books (192M)
93.5
Results on CONLL-2012 dataset
Model
Unlabeled Data
Mean F1
CVT
CNN-DM (18M)
89.260.1
BERTBase
Wiki+Books (192M)
89.00.23
Pre-BERTBase
CNN-DM (146M)
84.200.19
APBERTBase
CNN-DM (138M)
85.880.17
BERT-MRC+DSC
Wiki+Books (192M)
92.07
4 Results
We present here metrics-based and resource-based comparison of CVT and BERT models on all tasks. State-of-the-art (SOTA) baselines are included for reference.
Performance Metrics
We report mean F1 (with standard deviation) on the labeled test splits for each task over 5 randomized runs, and compare the models using statistical significance tests over these runs. Further, we report the approximate number of unlabeled sentences seen by each model. Table 2 shows the results for the OTE detection task. Here, out of the 3 BERT-based variations, the best result is achieved by the APBERTBase model across both SemEval datasets. For SemEval2016 Restaurants, we find the mean F1 from the APBERTBase model to be comparable to that of CVT (p-value 0.26). Both models outperform the SOTA baseline. For SemEval2014 Laptops, APBERTBase is found to have a statistically significant (p-value 0.04) higher F1 than CVT, and both models outperform SOTA.
In Tables 3 and 4, we present F1 results on NER and Slot-labeling task, respectively. For all 3 datasets, we find CVT to outperform all BERT models (statistically significant for CONLL-2003 and MIT Movies dataset, at p-values 0.0086 and 0.0085, respectively). For these tasks, BERTbase outperforms APBERTBase models. Furthermore, CVT outperforms SOTA for Slot-labeling task.
Model | Unlabeled Data | Mean F1 |
---|---|---|
CVT | IMDb (24.1M) | 88.160.12 |
BERTBase | Wiki+Books (192M) | 86.910.36 |
Pre-BERTBase | IMDb (30.7M) | 85.770.57 |
APBERTBase | IMDb (30M) | 86.780.1 |
HSCRF + softdict | - | 87.41 |
These results show that the CVT model, using unlabeled data in a task-specific manner, is more robust across different tasks and types of unlabeled data. For OTE detection, the unlabeled data is closely related to both domain and task, while for NER and Slot-labeling, the unlabeled data is related to genre (newswire) and domain (movies), but not necessarily to the specific tasks. In line with the findings of Gururangan et al. (2020), Adaptive Pre-training shows best results when using unlabeled data that is domain and task relevant (superior results for the OTE task). It is also worth noting that CVT requires significantly smaller amount of unlabeled data than the BERT models (Tables 2, 3 and 4).
Model | HW | Hours | Cost | Power | CO2 |
---|---|---|---|---|---|
CVT | 1/8 | 56 | 172 | 14.82 | 14.14 |
Pre-BERTBase | 8/64 | 85 | 2081 | 273.62 | 261.04 |
APBERTBase | 8/64 | 80 | 1958 | 260.63 | 248.64 |
Resource Cost
Table 5 shows computational cost and environmental impact by means of estimated CO2 emissions occurring during training. We use the procedure described by Strubell et al. (2019). Tesla V100 GPUs are used for training. For computational cost, we refer to the average cost per hour for the training instances used.666https://aws.amazon.com/ec2/instance-types/p3/ To compute energy consumed, we query the NVIDIA System Management Interface 777https://web.archive.org/web/20190504134329/https:/developer.nvidia.com/nvidia-system-management-interface multiple times during training, to note the average GPU power consumption. For CPU and DRAM power usage, we use Linux’s turbostat package.888http://manpages.ubuntu.com/manpages/xenial/man8/turbostat.8.html The models trained using Yelp Restaurants unlabeled data are used as an example in Table 5, but the same computations hold for other models. Note that we do not perform initial pretraining of BERTbase nor pretrain the Glove 840B.300d embeddings used in CVT, but these come at a one-time cost that we consider constant. Worth noting though, that BERTbase pretraining is more expensive than Glove pretraining. As is evident, training the CVT model incurs much less financial cost than the corresponding BERT models (11x lower than APBERTbase), while also emitting lesser CO2 emissions (18x lower than APBERTbase).999 If we consider just fine-tuning BERTBase on the supervised data of the downstream task (OTE detection on SemEval2016 Restaurants Data) the numbers corresponding to Table 5 are: HW: 1/8, Hours: 0.283, Cost: 0.87, Power: 0.094, CO2: 0.09. Although fine-tuning BERT on the downstream task (using supervised data) is relatively cheap, and one could amortize the cost of pre-training BERT over a large number of such tasks, this requires an understanding of what the number and type of such tasks are.
5 Conclusion & Future Work
We compare the task-specific semi-supervised method, CVT, with a task-agnostic semi-supervised approach, BERT (with and without adaptive pre-training), on a variety of problems that can be modeled as sequence tagging tasks. We find that the CVT-based approach is more robust than BERT-based models across tasks and types of unsupervised data available to them. Furthermore, the financial and environmental costs incurred are also significantly lower using CVT as compared to BERT.
As part of future work, we will explore CVT on other sequence-labeling tasks such as chunking, elementary discourse unit segmentation and argumentative discourse unit segmentation, thus moving beyond entity-level spans. Moreover, other supervised tasks such as classification could also be studied in this context. Furthermore, we intend to implement CVT as a training strategy over Transformers (BERT) and compare it with Adaptively-Pretrained BERT.
References
- Akbik et al. (2018) Alan Akbik, Duncan Blythe, and Roland Vollgraf. 2018. Contextual String Embeddings for Sequence Labeling. In Proceedings of the 27th International Conference on Computational Linguistics, pages 1638–1649, Santa Fe, New Mexico, USA. Association for Computational Linguistics.
- Baevski et al. (2019) Alexei Baevski, Sergey Edunov, Yinhan Liu, Luke Zettlemoyer, and Michael Auli. 2019. Cloze-driven Pretraining of Self-attention Networks. ArXiv, abs/1903.07785.
- Blum and Mitchell (1998) Avrim Blum and Tom Mitchell. 1998. Combining labeled and unlabeled data with co-training. In Proceedings of the eleventh annual conference on Computational learning theory, pages 92–100. ACM.
- Clark et al. (2018) Kevin Clark, Minh-Thang Luong, Christopher D Manning, and Quoc V Le. 2018. Semi-supervised sequence modeling with cross-view training. arXiv preprint arXiv:1809.08370.
- Devlin et al. (2019) Jacob Devlin, Ming-Wei Chang, Kenton Lee, and Kristina Toutanova. 2019. BERT: Pre-training of Deep Bidirectional Transformers for Language Understanding. pages 4171–4186.
- Gururangan et al. (2020) Suchin Gururangan, Ana Marasović, Swabha Swayamdipta, Kyle Lo, Iz Beltagy, Doug Downey, and Noah A. Smith. 2020. Don’t Stop Pretraining: Adapt Language Models to Domains and Tasks. ArXiv, abs/2004.10964.
- Kullback and Leibler (1951) S. Kullback and R. A. Leibler. 1951. On information and sufficiency. Ann. Math. Statist., 22(1):79–86.
- Lample et al. (2016) Guillaume Lample, Miguel Ballesteros, Sandeep Subramanian, Kazuya Kawakami, and Chris Dyer. 2016. Neural Architectures for Named Entity Recognition. In Proceedings of the 2016 Conference of the North American Chapter of the Association for Computational Linguistics: Human Language Technologies, pages 260–270, San Diego, California. Association for Computational Linguistics.
- Lewis et al. (2019) Mike Lewis, Yinhan Liu, Naman Goyal, Marjan Ghazvininejad, Abdelrahman Mohamed, Omer Levy, Ves Stoyanov, and Luke Zettlemoyer. 2019. BART: Denoising Sequence-to-Sequence pre-training for Natural Language Generation, translation, and comprehension.
- Li et al. (2019) Xiaoya Li, Xiaofei Sun, Yuxian Meng, Junjun Liang, Fei Wu, and Jiwei Li. 2019. Dice Loss for Data-imbalanced NLP Tasks. ArXiv, abs/1911.02855.
- Liu et al. (2019a) Tianyu Liu, Jin-Ge Yao, and Chin-Yew Lin. 2019a. Towards Improving Neural Named Entity Recognition with Gazetteers. In Proceedings of the 57th Annual Meeting of the Association for Computational Linguistics, pages 5301–5307, Florence, Italy. Association for Computational Linguistics.
- Liu et al. (2019b) Yinhan Liu, Myle Ott, Naman Goyal, Jingfei Du, Mandar Joshi, Danqi Chen, Omer Levy, Mike Lewis, Luke Zettlemoyer, and Veselin Stoyanov. 2019b. RoBERTa: A Robustly Optimized BERT Pretraining Approach. ArXiv, abs/1907.11692.
- Louvan and Magnini (2018) Samuel Louvan and Bernardo Magnini. 2018. Exploring Named Entity Recognition As an Auxiliary Task for Slot Filling in Conversational Language Understanding. In Proceedings of the 2018 EMNLP Workshop SCAI: The 2nd International Workshop on Search-Oriented Conversational AI, pages 74–80, Brussels, Belgium. Association for Computational Linguistics.
- Ma and Hovy (2016) Xuezhe Ma and Eduard H. Hovy. 2016. End-to-end sequence labeling via bi-directional LSTM-CNNs-CRF. ArXiv, abs/1603.01354.
- Maas et al. (2011) Andrew L. Maas, Raymond E. Daly, Peter T. Pham, Dan Huang, Andrew Y. Ng, and Christopher Potts. 2011. Learning Word Vectors for Sentiment Analysis. In Proceedings of the 49th Annual Meeting of the Association for Computational Linguistics: Human Language Technologies, pages 142–150, Portland, Oregon, USA. Association for Computational Linguistics.
- McClosky et al. (2006) David McClosky, Eugene Charniak, and Mark Johnson. 2006. Effective Self-Training for Parsing. In Proceedings of the main conference on human language technology conference of the North American Chapter of the Association of Computational Linguistics, pages 152–159. Association for Computational Linguistics.
- Pennington et al. (2014) Jeffrey Pennington, Richard Socher, and Christopher D. Manning. 2014. Glove: Global vectors for word representation. In Empirical Methods in Natural Language Processing (EMNLP), pages 1532–1543.
- Peters et al. (2018) Matthew E. Peters, Mark Neumann, Mohit Iyyer, Matt Gardner, Christopher Clark, Kenton Lee, and Luke Zettlemoyer. 2018. Deep Contextualized Word Representations. In Proc. of NAACL.
- Pontiki et al. (2016) Maria Pontiki, Dimitris Galanis, Haris Papageorgiou, Ion Androutsopoulos, Suresh Manandhar, AL-Smadi Mohammad, Mahmoud Al-Ayyoub, Yanyan Zhao, Bing Qin, Orphée De Clercq, et al. 2016. Semeval-2016 task 5: Aspect based sentiment analysis. In Proceedings of the 10th international workshop on semantic evaluation (SemEval-2016), pages 19–30.
- Pontiki et al. (2014) Maria Pontiki, Dimitris Galanis, John Pavlopoulos, Harris Papageorgiou, Ion Androutsopoulos, and Suresh Manandhar. 2014. SemEval-2014 Task 4: Aspect Based Sentiment Analysis. In Proceedings of the 8th International Workshop on Semantic Evaluation (SemEval 2014), pages 27–35, Dublin, Ireland. Association for Computational Linguistics.
- Pradhan et al. (2012) Sameer Pradhan, Alessandro Moschitti, Nianwen Xue, Olga Uryupina, and Yuchen Zhang. 2012. CoNLL-2012 Shared Task: Modeling Multilingual Unrestricted Coreference in OntoNotes. In Proceedings of the Sixteenth Conference on Computational Natural Language Learning (CoNLL 2012), Jeju, Korea.
- Ramshaw and Marcus (1999) Lance A Ramshaw and Mitchell P Marcus. 1999. Text Chunking using Transformation-based Learning. In Natural language processing using very large corpora, pages 157–176. Springer.
- Rietzler et al. (2019) Alexander Rietzler, Sebastian Stabinger, Paul Opitz, and Stefan Engl. 2019. Adapt or Get Left Behind: Domain Adaptation through BERT Language Model Finetuning for Aspect-Target Sentiment Classification.
- Sanh et al. (2019) Victor Sanh, Lysandre Debut, Julien Chaumond, and Thomas Wolf. 2019. DistilBERT, a distilled version of BERT: smaller, faster, cheaper and lighter. ArXiv, abs/1910.01108.
- Schwartz et al. (2019) Roy Schwartz, Jesse Dodge, Noah A. Smith, and Oren Etzioni. 2019. Green AI.
- Strubell et al. (2019) Emma Strubell, Ananya Ganesh, and Andrew McCallum. 2019. Energy and Policy Considerations for Deep Learning in NLP. In Proceedings of the 57th Annual Meeting of the Association for Computational Linguistics, pages 3645–3650, Florence, Italy. Association for Computational Linguistics.
- Tjong Kim Sang and De Meulder (2003) Erik F. Tjong Kim Sang and Fien De Meulder. 2003. Introduction to the CoNLL-2003 Shared Task: Language-Independent Named Entity Recognition. In Proceedings of the Seventh Conference on Natural Language Learning at HLT-NAACL 2003, pages 142–147.
- Vaswani et al. (2017) Ashish Vaswani, Noam Shazeer, Niki Parmar, Jakob Uszkoreit, Llion Jones, Aidan N Gomez, Łukasz Kaiser, and Illia Polosukhin. 2017. Attention is all you need. In Advances in neural information processing systems, pages 5998–6008.
- Xu et al. (2013) Chang Xu, Dacheng Tao, and Chao Xu. 2013. A survey on multi-view learning. arXiv preprint arXiv:1304.5634.
- Xu et al. (2019) Hu Xu, Bing Liu, Lei Shu, and Philip Yu. 2019. BERT post-training for review reading comprehension and aspect-based sentiment analysis. In Proceedings of the 2019 Conference of the North American Chapter of the Association for Computational Linguistics: Human Language Technologies, Volume 1 (Long and Short Papers), pages 2324–2335, Minneapolis, Minnesota. Association for Computational Linguistics.
- Xu et al. (2018) Hu Xu, Bing Liu, Lei Shu, and Philip S. Yu. 2018. Double Embeddings and CNN-based Sequence Labeling for Aspect Extraction. In Proceedings of the 56th Annual Meeting of the Association for Computational Linguistics (Volume 2: Short Papers), pages 592–598, Melbourne, Australia. Association for Computational Linguistics.
- Yarowsky (1995) David Yarowsky. 1995. Unsupervised Word Sense Disambiguation Rivaling Supervised Methods. In 33rd annual meeting of the association for computational linguistics.
Appendix A Appendices
A.1 Source Code and Data Preprocessing Steps
For CVT, we use the official author-provided codebase.101010https://github.com/tensorflow/models/tree/master/research/cvt_text The unlabeled datasets are preprocessed to have one sentence per line using NLTK’s sentence tokenizer 111111https://www.nltk.org/api/nltk.tokenize.html, as required by the model. For BERT pretraining, we use GluonNLP’s open-source code.121212https://github.com/dmlc/gluon-nlp/tree/master/scripts/bert For each unlabeled dataset, we create a randomly sampled validation set of about K samples during these experiments. Unlabeled data is processed to be in the required format.131313https://github.com/dmlc/gluon-nlp/blob/master/scripts/bert/sample_text.txt
A.2 Model Hyperparameters, Training Details and Validation F1
Here, we enlist the hyperparameters used for each model, and describe the training process. conlleval is used as the evaluation metric for each of the models.141414https://www.clips.uantwerpen.be/conll2003/ner/bin/conlleval
CVT: A batch-size of 64 is used for both labeled and unlabeled data. We use character embeddings of size 50, with char CNN filter widths of [2,3,4], and 300 char CNN filters. Encoder LSTMs have sizes 1024 and 512, respectively for the 1st and 2nd layer, with a projection size of 512. Dropout of 0.5 for labeled examples and 0.8 for unlabeled examples is used. Base learning rate of 0.5 is used, with an adaptive learning rate scheme, using SGD with Momentum as the optimizer.
Pretrained BERTBase (Pre-BERTBase) and Adaptive Pretraining BERTBase (APBERTBase): Batch-size of 256 is used during training. Number of steps for gradient accumulation is set to 4. BERTAdam is used as optimizer. Base learning rate used of 0.0001 is used, which is adaptive w.r.t. the number of steps. Maximum input sequence length is set to 512.
Steps at which Pre-BERTBase models converge are 1.02M for Yelp Restaurants and Amazon Electronics, 570K for CNN DailyMail, 119K for IMDb. Model convergence steps for APBERTBasewere 960K for Yelp-R, 930K for Amazon-E, 539K for CNN-DM, 117K for IMDb.
BERTBase Sequence Tagging model: Batch size for supervised fine-tuning on the downstream task is 10. We perform manual hyperparameter tuning over learning rate (0.00001, 0.0001 and 0.001) and dropout (0.0 to 0.5 in steps of 0.1). Validation F1 is used to select the best set of hyper-parameters which were learning rate of 0.00001, dropout of 0.0 for NER and Slot-labeling, and 0.1 for OTE detection.
We demonstrate mean validation set F1 numbers for each task and dataset in Table 6.
Task/Dataset | Model | Mean Val F1 |
---|---|---|
OTE/SE16-R | CVT | 75.120.49 |
BERTBase | 78.650.27 | |
Pre-BERTBase | 82.150.63 | |
APBERTBase | 82.880.74 | |
OTE/SE14-L | CVT | 81.581.56 |
BERTBase | 78.261.3 | |
Pre-BERTBase | 78.400.49 | |
APBERTBase | 79.751.13 | |
NER/CONLL2003 | CVT | 95.540.1 |
BERTBase | 95.710.04 | |
Pre-BERTBase | 91.150.10 | |
APBERTBase | 93.190.14 | |
NER/CONLL2012 | CVT | 87.140.11 |
BERTBase | 88.230.08 | |
Pre-BERTBase | 83.380.15 | |
APBERTBase | 84.900.09 | |
Slot-labeling/MIT-M | CVT | 88.310.27 |
BERTBase | 88.040.13 | |
Pre-BERTBase | 87.420.10 | |
APBERTBase | 93.10.06 |