Think Twice Before Recognizing:
Large Multimodal Models for General Fine-grained Traffic Sign Recognition
Abstract
We propose a new strategy called think twice before recognizing to improve fine-grained traffic sign recognition (TSR). Fine-grained TSR in the wild is difficult due to the complex road conditions, and existing approaches particularly struggle with cross-country TSR when data is lacking. Our strategy achieves effective fine-grained TSR by stimulating the multiple-thinking capability of large multimodal models (LMM). We introduce context, characteristic, and differential descriptions to design multiple thinking processes for the LMM. The context descriptions with center coordinate prompt optimization help the LMM to locate the target traffic sign in the original road images containing multiple traffic signs and filter irrelevant answers through the proposed prior traffic sign hypothesis. The characteristic description is based on few-shot in-context learning of template traffic signs, which decreases the cross-domain difference and enhances the fine-grained recognition capability of the LMM. The differential descriptions of similar traffic signs optimize the multimodal thinking capability of the LMM. The proposed method is independent of training data and requires only simple and uniform instructions. We conducted extensive experiments on three benchmark datasets and two real-world datasets from different countries, and the proposed method achieves state-of-the-art TSR results on all five datasets.
Index Terms:
Fine-grained, traffic sign recognition, large multimodal models.I Introduction
Ensuring traffic safety remains an important issue in the real world [1]. The latest statistics from the World Health Organization show that road traffic injuries are the leading cause of death among children and adolescents aged 5 to 29 years and that approximately 1.19 million people die each year due to road traffic accidents 111https://www.who.int/news-room/fact-sheets/detail/road-traffic-injuries. Furthermore, road traffic accidents result in substantial economic losses and impose a significant burden on society [2]. Consequently, there is an urgent need to decrease road traffic accidents.
Traffic sign recognition (TSR) is a technology that enables vehicles to identify traffic signs on dynamic road scenes. As an important part of the road, it is crucial to effectively recognize traffic signs for traffic safety. Advanced driver assistance systems help drivers make safer decisions by evaluating driving conditions based on traffic sign data and notifying drivers of inconsistencies on the road [3]. In addition, TSR helps global positioning systems and map service providers to update their databases. Therefore, TSR technology has attracted widespread attention.
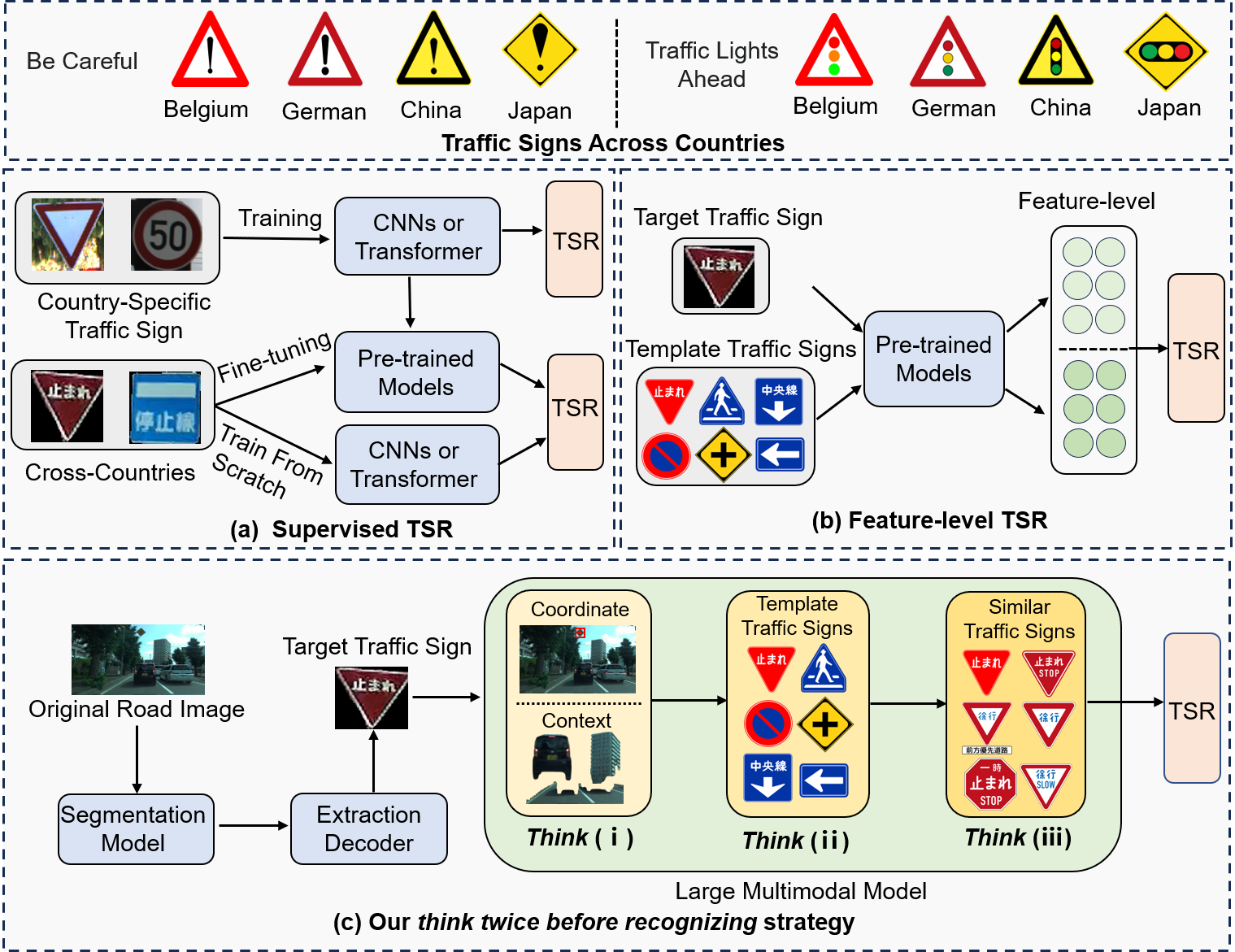
Early studies of TSR focus on using hand-crafted features such as histogram of oriented gradients (HOG) [4, 5, 6, 7] and scale-invariant feature transform (SIFT) [8, 9, 10]. Newer methods are based on convolutional neural networks (CNNs) [11, 12, 13, 14] and vision transformers [15, 16, 17, 18], which utilize the feature representation capabilities of convolutional layers or attention mechanisms to perform supervised recognition on country-specific traffic sign images as shown in Fig. 1-(a). These methods have two limitations: first, the supervised feature learning process requires a large amount of carefully crafted traffic sign data for training, which are usually clearly visible. In contrast, traffic signs on real-world roads can be blurred or broken due to the influence of dynamic road scenes, weather, and other factors. Second, to unify the traffic signs across countries, the Vienna Convention on Road Traffic [19] stipulates more than 300 different traffic sign categories and only 83 countries have signed the treaty. Even between countries that have signed the treaty, there are still some visual differences between traffic sign images. As shown at the top of Fig. 1, even for the same type of traffic signs “Be Careful” and “Traffic Lights Ahead”, there are differences between countries. Since trained on country-specific datasets, these methods require fine-tuning or training from scratch when recognizing traffic signs in other countries. These are costly due to data policy restrictions in various countries and the difficulty in obtaining data in underdeveloped regions. Some methods through unsupervised learning or feature matching have been proposed to solve the problems of this cross-country TSR problem [20, 21, 22, 23, 24]. These methods utilize strategies such as zero-shot learning or few-shot learning for TSR, thus reducing the dependence on training data and alleviating the applicability problem of cross-country traffic signs. However, cross-domain biases exist between the target and template traffic signs as shown in Fig. 1-(b), and performing pairwise matching at the feature level increases this important difference. Therefore, the recognition accuracy of these methods remains to be further improved.
Recent breakthroughs in large language models (LLMs) [25, 26, 27, 28] have brought general AI models that can solve a wide range of complex natural language tasks, many of which are approaching the level of human experts’ performance [27, 29]. In addition to text, people live in a multimodal world containing various images. Many studies have been devoted to constructing visual-text large multimodal models (LMMs) [30, 28, 31, 32, 33], to solve various visual problems existing in the real world [34, 35, 36, 37, 38]. In traffic safety, LMMs show great application value in constructing future intelligent transportation systems [39]. Besides, LMMs have great potential in autonomous driving and can revolutionize the traditional human-vehicle interaction model [40]. Users can communicate requests through languages, gestures, and even eyes, and LMM provides real-time in-vehicle feedback through integrated visual displays. However, despite the unprecedented recognition capabilities of LMM, its researches in TSR are limited to be explored. In general tasks, raw images are usually directly input into the LMM for recognition. On the one hand, it is difficult to recognize traffic signs directly as they are too small, e.g., in a road image with 1,280 960 pixels, the traffic sign may be only 30 30 pixels. On the other hand, unlike recognizing such as “cats” and “dogs”, different types of traffic signs are highly similar and TSR requires accurate recognition at a fine-grained level. Therefore, detailed studies are necessary to stimulate the potential of LMM to realize fine-grained TSR.
In this paper, we present a novel approach to improve fine-grained TSR. The core strategy of the proposed method is to make the LMM think in multiple dimensions, i.e., think twice before recognizing. As shown in Fig. 1-(c), we first design a traffic sign extraction module, which locates and extracts traffic signs in the original road image while excluding potential background interference. To stimulate the recognition ability of the LMM, we designed multiple thinking processes to inspire the LMM to enhance the fine-grained TSR.
Think (i): As previously mentioned, directly recognizing traffic signs from original road images remains a challenge. We propose the context description, which contains important contextual information about traffic signs, such as crosswalks, vehicles, pedestrians, etc. Referencing the real-world question answering and prompting process, we elaborated a prompting strategy that allows the LMM to generate context descriptions while giving potential candidate answer options, named the prior traffic sign hypothesis. The prior traffic sign hypothesis helps filter irrelevant answers and reduce the difficulty of subsequent thinking. In addition, multiple traffic signs often exist in an original road image, which brings difficulties for LMMs to generate accurate context descriptions for the target traffic sign. We simplify the complex and propose a center coordinates-based optimization method. The center coordinates help the LMM to quickly pinpoint the target traffic sign in the original road image and generate accurate context descriptions and the prior traffic sign hypothesis.
Think (ii): Due to the need for fine-grained recognition, although the LMM can recognize the features of the target traffic sign, it is difficult to accurately answer the traffic sign types based on the existing knowledge. We address this problem by introducing few-shot in-context learning based on template traffic signs. Specifically, considering the three important characteristics of traffic signs, namely shape, color, and composition, we generate the characteristic description of each type of template traffic sign by few-shot in-context learning. The characteristic descriptions stimulate the LMM’s fine-grained perceptual ability. Unlike the previous methods, the proposed method reduces the cross-domain differences between the real and template traffic signs by avoiding computation at the feature level. The template traffic signs can be easily obtained in the traffic sign databases of various countries.
Think (iii): Since the characteristics of certain types of traffic signs are highly similar. Our preliminary experiments demonstrate the limited ability of LMM to recognize similar traffic signs. We propose differential descriptions to emphasize the subtle dissimilarity between these traffic signs. Differential descriptions can further optimize our strategy and improve the fine-grained recognition performance of LMM.
When recognizing the traffic signs, the LMM performs multiple thinking based on the generated descriptions. Our thinking strategy can largely motivate the LMM for fine-grained TSR. The proposed method is independent of training data and applicable to cross-country TSR. In addition, the generation of each description is performed only once and requires only simple and uniform instructions. Our contributions can be concluded as follows.
-
•
We propose a new strategy of think twice before recognizing to stimulate the perceptual potential of fine-grained TSR by strengthening the multiple-thinking capability of LMMs.
-
•
We introduce the context descriptions of the original road images and propose the prior traffic sign hypothesis and center coordinate prompt optimization for localizing the target traffic sign in original road images containing multiple traffic signs and filtering irrelevant answers.
-
•
We introduce few-shot in-context learning based on template traffic signs, which enhances the fine-grained perceptual capability of LMMs. The characteristic descriptions reduce the cross-domain differences between the template and target traffic signs. We also generate differential description texts between similar traffic signs to optimize the multimodal thinking capability of the LMM.
-
•
We conducted extensive experiments on three benchmark datasets and two real-world datasets from different countries, and the proposed method achieves promising TSR results on all five datasets.
II Related Work
II-A Traffic Sign Recognition
TSR has become a widely researched field, deriving many approaches to challenge this task. TSR is generally divided into two key steps: traffic sign detection (TSD) and traffic sign classification (TSC). TSD performs localization and detection of traffic signs in road images, while TSC performs classification on the detected traffic signs. There have been many studies based on traditional and deep learning methods for TSR.
II-A1 Traditional TSR methods
The early TSR studies focus on performing recognition based on hand-crafted features and machine learning algorithms. For example, hand-crafted features were used to extract features from traffic signs and machine learning algorithms recognized the extracted features. Zaklouta et al. [5] introduce a real-time system for detecting and classifying circular and triangular traffic signs. Kus et al. [41] introduced a method for detecting and recognizing traffic signs by improving upon the SIFT [8] algorithm. They enhanced SIFT by integrating features associated with the color of local regions. Huang et al. [7] propose a streamlined method for TSR by using HOG features and a single classifier trained with the extreme learning machine algorithm. The HOG feature strikes a balance between redundancy and local details, improving the representation of distinctive shapes. In this way, the traditional methods rely heavily on hand-crafted features, which are sensitive to variations in lighting, occlusion, and complex backgrounds [42].
II-A2 Deep learning-based TSR methods
The emergence of deep learning has inspired TSR research. Compared with traditional hand-crafted feature-based methods, deep learning-based methods can better learn features of traffic sign images. Zhang et al. [43] introduced two lightweight networks for improving recognition accuracy with fewer parameters. Abudhagir et al. [44] utilized the LeNet model for traffic sign recognition. Their CNN architecture consisted of the first two layers adapted from LeNet, followed by two additional convolutional layers, a dropout layer, and a flattened layer. Zhu et al. [45] proposed a TSR method based on YOLOv5. Besides, transformer-based TSR methods have also been proposed. Zheng et al. [18] used a vision transformer (ViT) [46] to perform a detailed TSR evaluation. Luo et al. [16] proposed a TSR approach comprising a lightweight pre-locator network and a refined classification network based on Swin-Transformer [47]. The pre-locator network identifies sub-regions of traffic signs, while the refined classification network handles recognition within these regions. Guo et al. [17] proposed an end-to-end framework that integrates component detection, content reasoning, and semantic description generation for understanding traffic signs. Since trained on country-specific datasets, these supervised methods require fine-tuning or training from scratch when recognizing traffic signs in other countries. Several TSR approaches have been introduced to solve the problem. Cao et al. [48] proposed a zero-shot method, which synthesizes a hybrid feature representation by extracting both general and principal visual features from traffic sign images. Gan et al. [23] introduced a zero-shot approach utilizing midlevel features extracted from CNNs. However, due to the existence of cross-domain biases and the need for improving accuracy, more effective methods are expected to be explored.
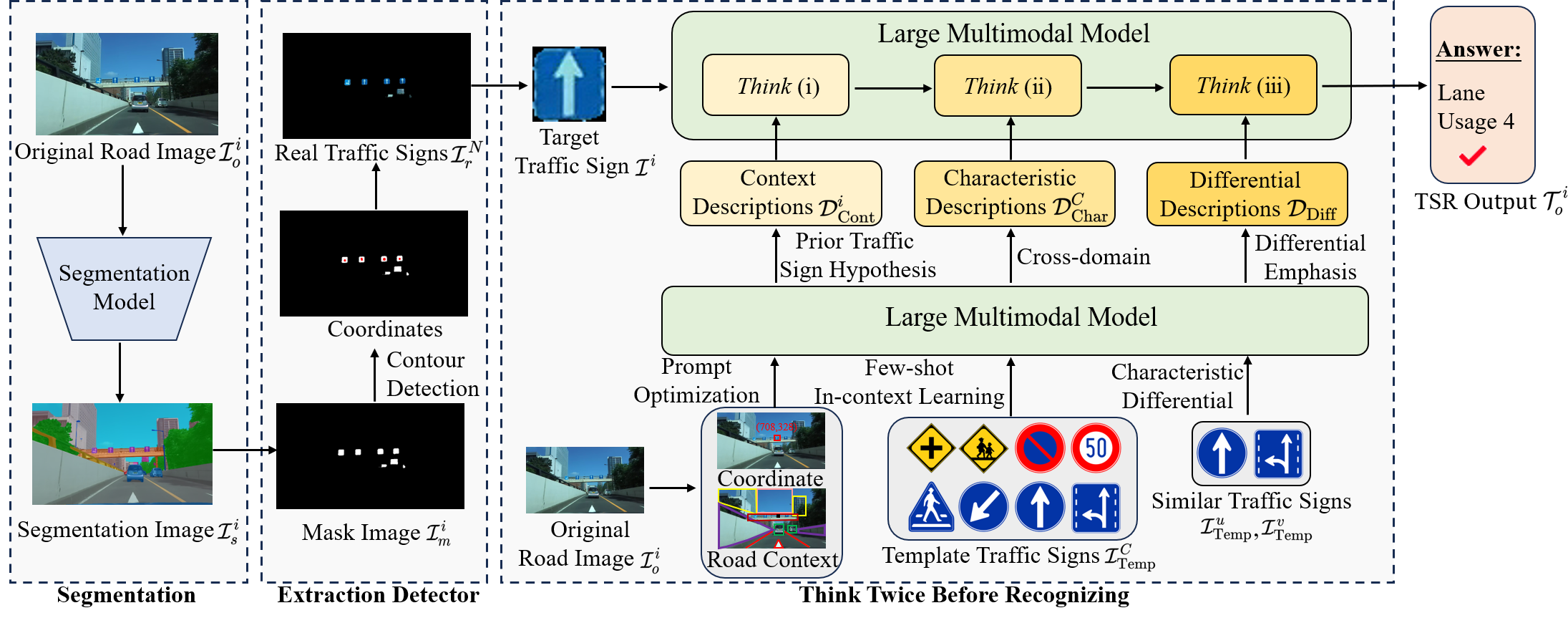
II-B Large Multimodal Models
The LLM has received a lot of attention recently [49]. As demonstrated by existing work [29], LLMs can solve a wide variety of tasks, in contrast to previous models that were limited to solving specific tasks. In addition, LMMs have been proposed [28, 31, 32, 50, 51, 52] to address a wide variety of visual problems that exist in the real world. LMM further extends the capabilities of language models by integrating visual information as part of the input. This integration of visual data enables LMM to efficiently understand and generate responses that contain both textual and visual prompts, thus enabling richer context conversations in multimodal environments. In recent months, LMMs have also drawn attention in intelligent transportation fields, such as autonomous driving and mapping systems [53]. LMMs have the potential to revolutionize the traditional human-vehicle interaction paradigm [40]. LMMs can process information from text and image inputs captured by in-vehicle cameras to understand complex traffic situations. In addition, they can significantly enhance personalized human-vehicle interactions through voice communication and user preference analysis. Drivers can use languages, gestures, and even eyes to communicate their requests while driving, and the LMM provides real-time in-vehicle feedback through integrated visual displays. However, despite the unprecedented capabilities of LMMs, TSR-related studies based on LMMs remain to be explored.
III Methodology
We explain the proposed fine-grained TSR method in this section. As shown in Fig. 2, our method first implements localization and detection on original road images and then the extraction of traffic signs is performed by the designed extraction detector. Next, we implement the proposed think twice before recognizing strategy for stimulating the fine-grained TSR capability of the LMM.
III-A Traffic Sign Extraction
III-A1 Segmentation
In the proposed method, we first perform segmentation of the original road image containing the traffic signs . represents the number of traffic signs contained in the original road image. The original road image is input to the segmentation model. The segmentation model generates segmentation images with various object category labels for the original image. As the recognition of traffic signs is performed, the traffic signs need to be distinguished from other objects. Specifically, in the segmentation image , each particular object category is coded as a different color for identification. We convert to a mask image , thereby separating the traffic sign from the other objects and background in . The segmentation model is not limited to a specific architecture.
III-A2 Extraction
The extraction of traffic signs is realized by a designed extraction detector. The extraction detector first obtains the coordinates of the traffic signs in the mask image using the contour detection algorithm [54]. Then, the extraction detector uses the original road image and the coordinates of the traffic signs to extract the image that contains only the real traffic signs. removes other objects and backgrounds in the original road image. The extraction detector finally retrieves the traffic sign image from using the corresponding coordinates of the traffic signs. represents the final extracted traffic sign image. Note that while can also be obtained directly from the original road image via the coordinates, the extracted traffic sign image contains unnecessary backgrounds. In contrast, the designed extraction detector can remove the backgrounds and avoid potential interference for subsequent recognition.
III-B Think Twice Before Recognizing
After obtaining the traffic sign image , we perform the think twice before recognizing strategy to stimulate the perceptual potential of fine-grained TSR with LMM. Our think twice before recognizing strategy consists of two steps: prior knowledge generation and multistep thinking.
III-B1 Prior Knowledge Generation
In the proposed method, prior knowledge includes context descriptions of original road images, characteristic descriptions of template traffic signs, and differential descriptions of similar traffic signs. The inputs of LMMs are typically an image and a text query with length , and LMMs generate a sequence of textual output with length as follows:
(1) |
Context Descriptions: Since original road images contain important contextual information about traffic signs, we transform original road images into context descriptions to fully utilize the scene information. Given an original road image , the context descriptions are generated as
(2) |
where represents the prompt for generating the context descriptions. As shown in Fig. 3, we carefully designed so that the generated contextual descriptions contain the context background information understood by LMM from the original road image. Furthermore, as in the real-world question-answering process, we find that narrowing the range of answers can reduce the recognition difficulty of LMM. To this end, we propose a prior traffic sign hypothesis, which allows LMM to filter irrelevant traffic sign types and provide potential candidates. Similar to human cognition, where irrelevant answers are swiftly filtered based on existing knowledge, the potential traffic sign candidates generated by the prior traffic sign hypothesis are obtained from LMM’s preliminary understanding of the original road image. This preliminary understanding stimulates subsequent detailed thinking. In addition, when multiple traffic signs exist in the original road image, it is difficult for the LMM to perform context description and the prior traffic sign hypotheses generation. Therefore, we simplify the complex and propose a prompt optimization method based on center coordinates. The prompt optimization method provides the center coordinates of traffic signs to inspire the LMM to locate the target traffic sign from the original road image. Since the center coordinates are obtained from the extraction detector, no additional calculations for center coordinates are required. The center coordinates help the LMM to locate the target traffic sign and generate corresponding background descriptions and prior traffic sign hypotheses.
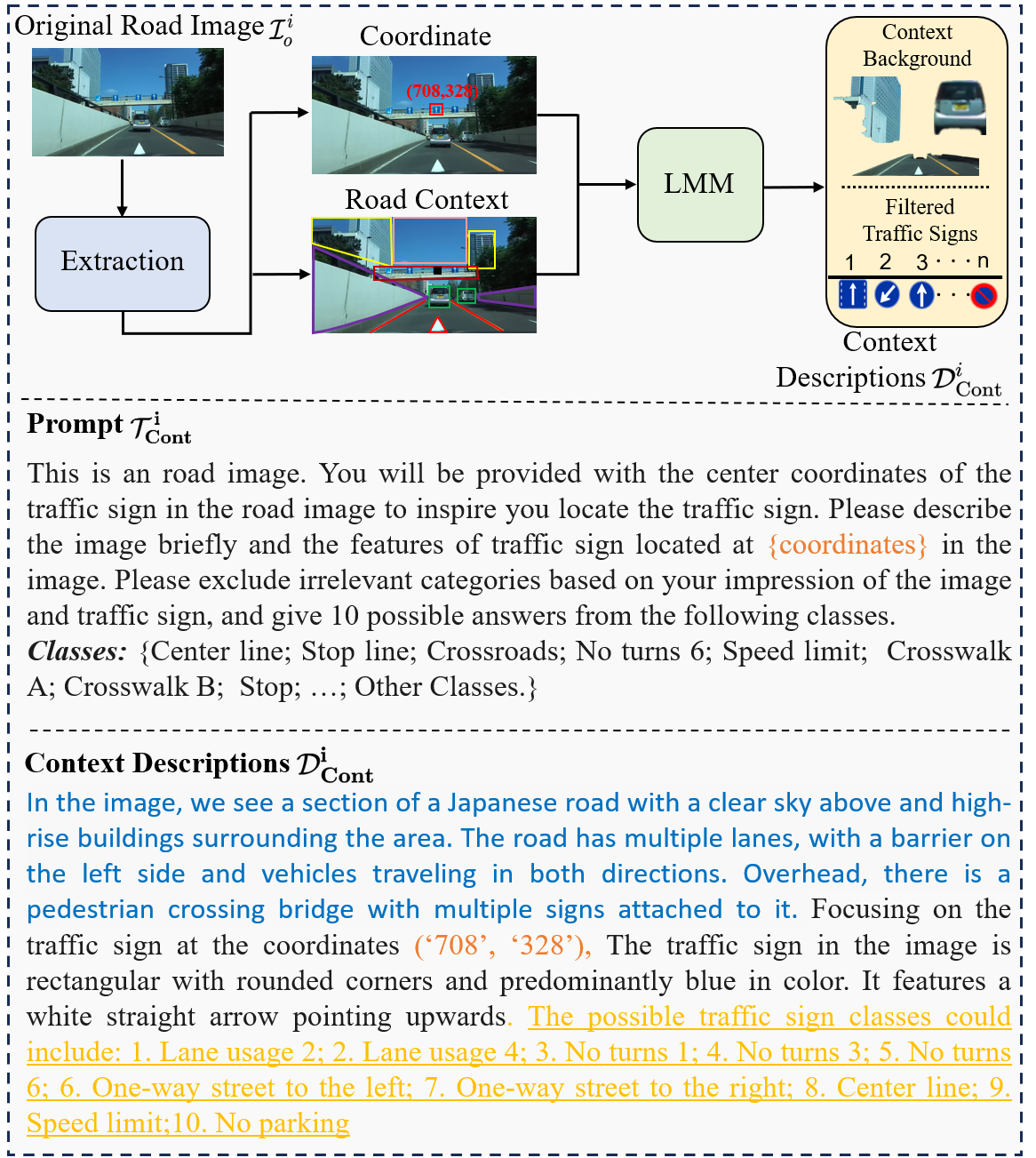
Characteristic Descriptions: Since TSR is a fine-grained task, it is difficult for LMMs to accurately answer the specific types of traffic signs based on the existing knowledge. We consider that template traffic signs are easily accessible in national traffic sign databases, which helps to reduce the dependence on training data. Although previous TSR methods have utilized template traffic signs at the feature level, actual traffic sign images are diverse due to lighting conditions, angles, occlusions, etc., and can be different from template traffic sign images. It increases the difficulty of cross-domain recognition at the feature level. We introduce the few-shot in-context learning to generate characteristic descriptions of each class of template traffic sign with the prompts as follows:
(3) |
where represents the corresponding prompt for the template traffic sign . As shown in Fig. 4, traffic signs in all countries have three key features: shape, color, and composition. When performing in-context learning, the designed prompt makes the LMM focus on these three features without introducing any other irrelevant information. The proposed in-context learning method only needs to generate the characteristic descriptions once for each template traffic sign. By avoiding computation at the feature level, the generated characteristic descriptions can reduce cross-domain differences between templates and real traffic signs. The prompts we design are uniform and simple for each traffic sign and require no special adjustments. We construct a memory bank to store the generated characteristic descriptions.
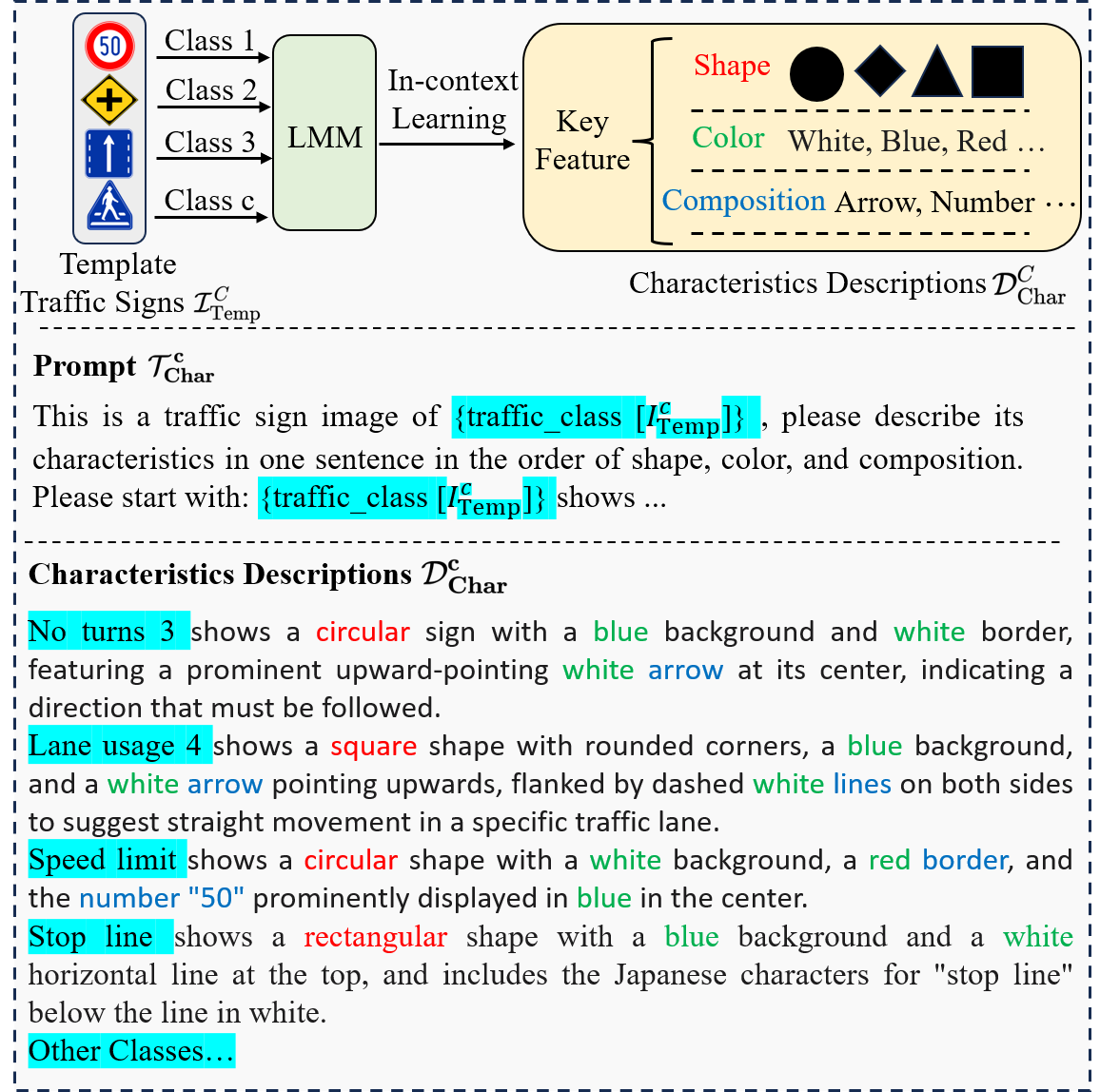
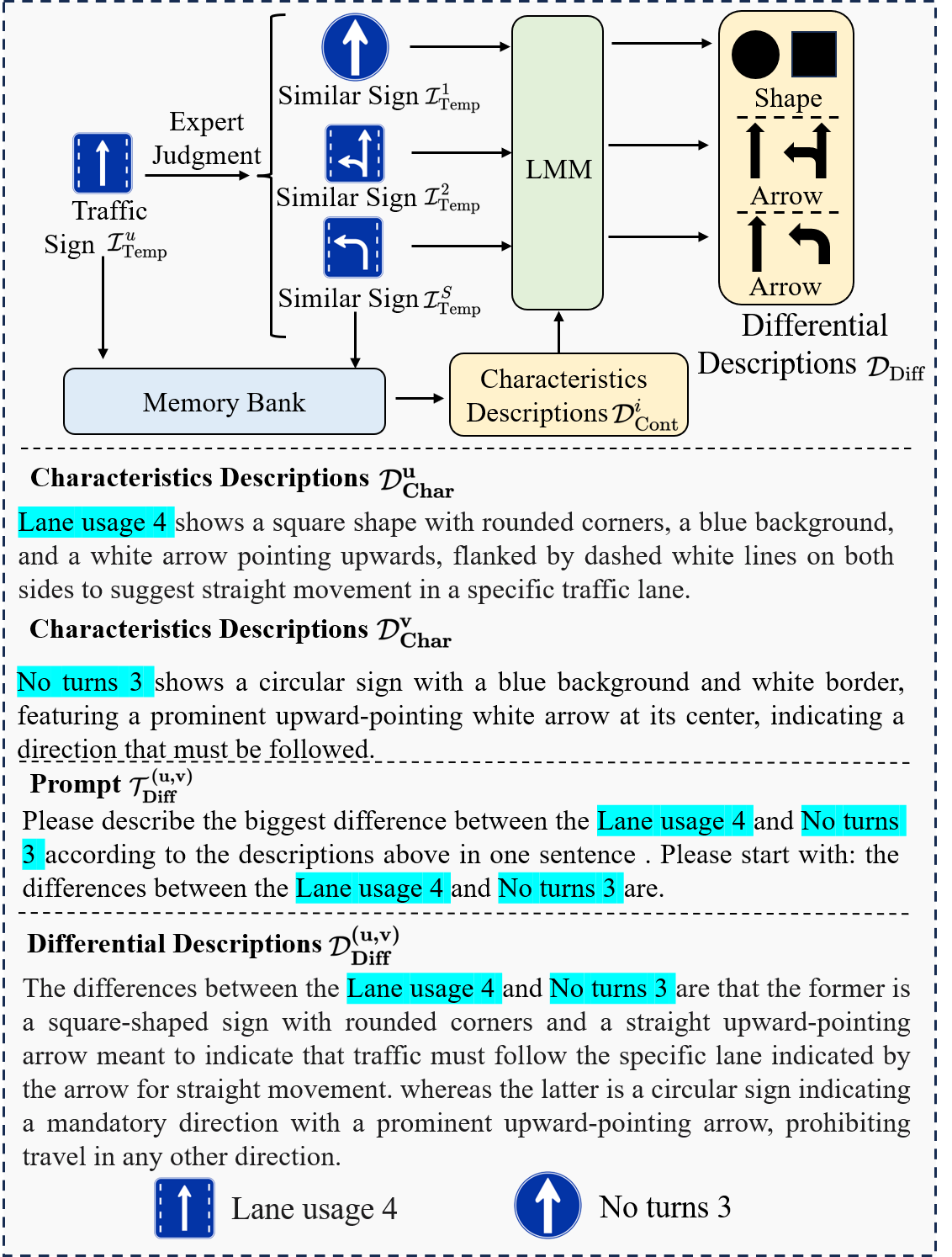
Differential Descriptions: Since the characteristics of certain types of traffic signs are similar, we generate differential descriptions to emphasize the differences between these traffic signs. Given a template traffic sign and the similar traffic sign , the characteristic descriptions of and can be obtained by Eq. (3), and are respectively expressed as
(4) |
(5) |
The differential descriptions are then obtained by inputting and into the LMM as follows:
(6) |
where represents the prompt for generating the differential descriptions of and . Thus, the final differential descriptions are expressed as
(7) |
As shown in Fig. 5, experts perform the judgments of the signs similar to the traffic sign. The characteristic descriptions in the memory bank are imported to help generate differential descriptions of the traffic sign and each similar sign. The differential descriptions emphasize the subtle differences between similar traffic signs and can further optimize the fine-grained recognition ability of LMMs.
III-B2 Multistep Thinking
After obtaining the context descriptions , characteristic descriptions , and differential descriptions , the LMM performs multistep reasoning for a target traffic sign. Step 1: LMM first conducts a preliminary understanding of the target traffic sign image based on existing knowledge. Step 2: LMM understands the scene information around the target traffic sign by referring to the context descriptions. Meanwhile, LMM further narrows the thinking scope by referring to the prior traffic sign hypotheses. Step 3: referring to the characteristic descriptions, LMM understands the basic features of various traffic signs of the shape, color, and composition, and compares the understanding of the target traffic sign image with the characteristic descriptions, thus stimulating the fine-grained TSR. Final: referring to the differential descriptions, LMM gains insight into understanding the differences between the target traffic sign and other similar traffic signs to optimize the recognition results as follows:
(8) |
where represents the designed multistep prompt, and is the final TSR results of the LMM. Through multistep thinking, the LMM performs feature inference step by step to finally find out the “real face” of the target traffic sign. Multistep thinking can largely stimulate the LMM’s ability to recognize traffic signs at a fine-grained level. Therefore, the fine-grained TSR performance in real-world scenarios of LMM is improved.
IV Experiments
IV-A Experimental Settings
We conducted comprehensive experiments on several datasets, including three benchmark datasets: the German traffic sign recognition benchmark (GTSRB) dataset [55], the Belgium traffic sign dataset [56] and the Tsinghua-Tencent 100K (TT-100K) dataset [57]. TT-100K focuses on complex scenarios in the real world and is therefore a difficult benchmark to recognize. Besides, to fully evaluate the performance of the proposed method in real-world scenes, we also conducted experiments on two real-world datasets in Japan, the Sapporo urban road dataset (Sapporo) and the Yokohama urban road dataset (Yokohama). We perform fine-grained TSR by Gpt-4v and Gpt-4o. The proposed method does not require training any model. However, due to the rate limits of LMM’s API 222https://platform.openai.com/account/limits, we followed the experimental setting strategy in [58] and randomly used the subset of GTSRB, BTSD, and TT-100K validation data for our study. Note that we do not reduce the number of categories in the subset, but rather keep it consistent with the categories in the full dataset to fully validate the fine-grained TSR performance of our think twice before recognizing strategy. In addition, since the traffic signs in the GTSRB and BTSD datasets have been extracted, multiple thinking is directly performed on them, and due to the lack of original road images, context descriptions were not generated. For TT-100K, Sapporo, and Yokohama datasets, we utilize the proposed traffic sign extraction framework to locate and extract traffic signs from the original road images. To evaluate the performance of the proposed fine-grained TSR method, we adopted the common evaluation metric of Top- accuracy, which performs comprehensive evaluations of the TSR performance. Top- is defined as follows:
(9) |
Here, represents the number of correctly recognized target traffic signs in the Top- results. Considering the challenges of fine-grained TSR when no training data is available, the Top- metric can effectively measure the TSR performance.
Method | GTSRB | BTSD | TT-100K | Sapporo | Yokohama | ||||||||||
---|---|---|---|---|---|---|---|---|---|---|---|---|---|---|---|
Top-1 | Top-3 | Top-5 | Top-1 | Top-3 | Top-5 | Top-1 | Top-3 | Top-5 | Top-1 | Top-3 | Top-5 | Top-1 | Top3 | Top-5 | |
Song et al. [4]* | 0.10 | 0.23 | 0.29 | 0.19 | 0.25 | 0.32 | 0.04 | 0.10 | 0.13 | 0.04 | 0.57 | 0.77 | 0.04 | 0.18 | 0.42 |
Ren et al. [20] | 0.41 | 0.64 | 0.77 | 0.11 | 0.36 | 0.50 | 0.26 | 0.42 | 0.50 | 0.34 | 0.47 | 0.50 | 0.21 | 0.42 | 0.48 |
Gan et al. [23] | 0.56 | 0.76 | 0.84 | 0.67 | 0.84 | 0.91 | 0.12 | 0.22 | 0.36 | 0.42 | 0.71 | 0.79 | 0.27 | 0.42 | 0.51 |
DenseNet-121 [59] | 0.31 | 0.46 | 0.59 | 0.21 | 0.32 | 0.49 | 0.08 | 0.14 | 0.24 | 0.73 | 0.82 | 0.84 | 0.23 | 0.47 | 0.70 |
EfficientNet-B0 [60] | 0.52 | 0.76 | 0.90 | 0.60 | 0.86 | 0.93 | 0.17 | 0.30 | 0.38 | 0.51 | 0.66 | 0.74 | 0.25 | 0.44 | 0.60 |
Li et al. [61]* | 0.75 | 0.83 | 0.89 | 0.82 | 0.91 | 0.94 | 0.27 | 0.46 | 0.60 | 0.70 | 0.80 | 0.83 | 0.29 | 0.45 | 0.69 |
Zheng et al. (ViT-L) [18]* | 0.44 | 0.58 | 0.70 | 0.39 | 0.57 | 0.64 | 0.09 | 0.16 | 0.21 | 0.54 | 0.63 | 0.75 | 0.19 | 0.36 | 0.44 |
Luo et al. [16]* | 0.15 | 0.35 | 0.48 | 0.22 | 0.27 | 0.34 | 0.14 | 0.28 | 0.41 | 0.39 | 0.57 | 0.70 | 0.18 | 0.35 | 0.56 |
MobileViT [62] | 0.05 | 0.11 | 0.22 | 0.02 | 0.07 | 0.10 | 0.05 | 0.11 | 0.15 | 0.08 | 0.10 | 0.29 | 0.06 | 0.35 | 0.42 |
Swin-Transformer V2 [63] | 0.14 | 0.26 | 0.37 | 0.06 | 0.17 | 0.32 | 0.09 | 0.17 | 0.23 | 0.06 | 0.10 | 0.18 | 0.09 | 0.27 | 0.58 |
MAE [64] | 0.20 | 0.32 | 0.47 | 0.13 | 0.36 | 0.49 | 0.06 | 0.10 | 0.13 | 0.14 | 0.27 | 0.41 | 0.17 | 0.32 | 0.51 |
DeiT [65] | 0.27 | 0.45 | 0.57 | 0.12 | 0.28 | 0.42 | 0.34 | 0.60 | 0.70 | 0.71 | 0.83 | 0.88 | 0.26 | 0.47 | 0.69 |
CLIP (ViT-B/32) [66] | 0.24 | 0.35 | 0.48 | 0.20 | 0.30 | 0.38 | 0.29 | 0.50 | 0.62 | 0.27 | 0.50 | 0.57 | 0.14 | 0.48 | 0.60 |
EVA-02 [67] | 0.41 | 0.67 | 0.75 | 0.30 | 0.51 | 0.66 | 0.32 | 0.61 | 0.76 | 0.48 | 0.53 | 0.62 | 0.29 | 0.46 | 0.70 |
Ours (Gpt-4v) | 0.91 | 0.96 | 0.97 | 0.89 | 0.91 | 0.92 | 0.90 | 0.97 | 0.99 | 0.77 | 0.86 | 0.89 | 0.83 | 0.91 | 0.95 |
Ours (Gpt-4o) | 0.93 | 0.97 | 0.98 | 0.88 | 0.91 | 0.91 | 0.97 | 0.99 | 0.99 | 0.89 | 0.95 | 1.00 | 0.85 | 0.96 | 0.97 |
IV-B Experimental Results
Table I shows the Top- fine-grained TSR performance compared with the state-of-the-art methods. We performed evaluations of the three benchmarks and two real-world datasets to validate the effectiveness of the proposed method. As shown in Table I, all comparison methods have limited accuracy without training data, reflecting the difficulty of fine-grained TSR in the wild. Meanwhile, the recognition performance of methods such as Li et al. [61] and Zheng et al. (ViT-L) [18] show significant performance differences on datasets from different countries, highlighting that these methods struggle with cross-country TSR when there is a lack of training data. The Top- and Top- accuracies of the proposed method outperform the comparison methods on all the five datasets with significant improvements compared to the hand-craft features (Song et al. [4], Ren et al. [20]), the CNN-based (Gan et al. [23], DenseNet-121 [59], EfficientNet-B0 [60], Li et al. [61]), and Transformer-based (Zheng et al. (ViT-L) [18], Luo et al. [16]) TSR methods, proving the effectiveness of our think twice before recognizing strategy. We also compare the fine-grained TSR performance with advanced transformer architectures (MobileViT [62], Swin-Transformer V2 [63], MAE [64], DeiT [65], CLIP (ViT-B/32) [66], EVA-02 [67]). Our approach similarly shows promising performance. Especially, our LMM-based method is substantially ahead in Top-1 recognition accuracy for all datasets compared to the compared methods, which shows the superior ability of our strategy for fine-grained TSR. Note that all the experimental results are based on the average of five trials to verify the recognition stability of the proposed method.
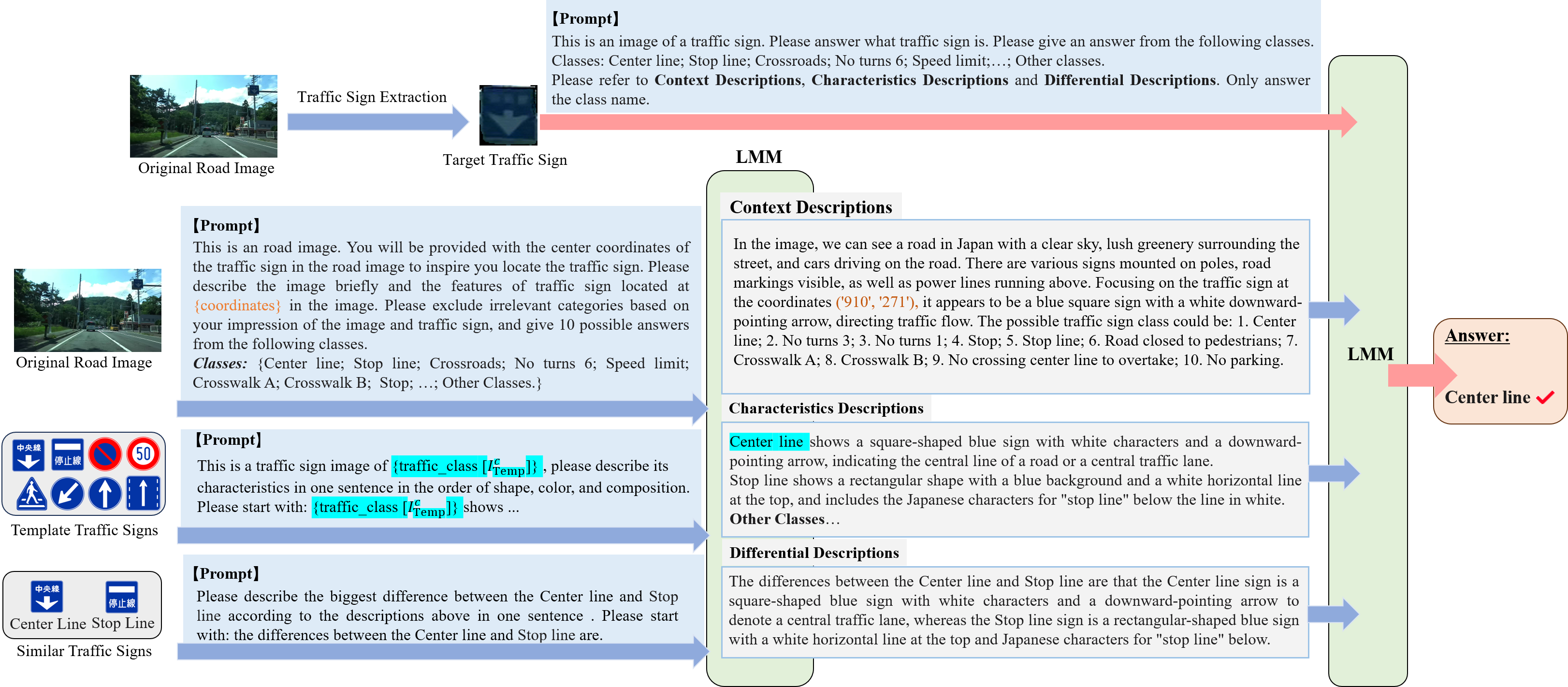
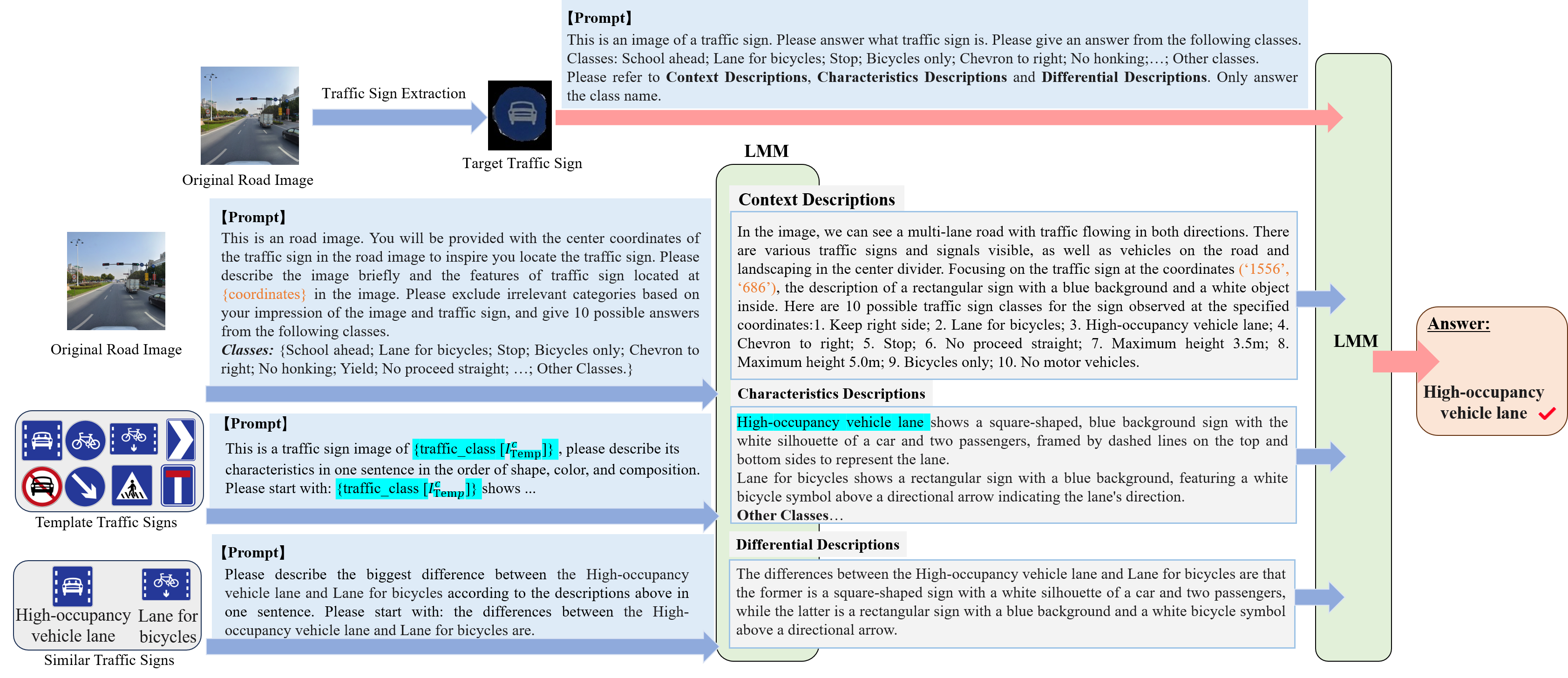
LMM | Cont* | Char* | Diff* | GTSRB | BTSD | TT-100K | Sapporo | Yokohama | ||||||||||
---|---|---|---|---|---|---|---|---|---|---|---|---|---|---|---|---|---|---|
Top-1 | Top-3 | Top-5 | Top-1 | Top-3 | Top-5 | Top-1 | Top-3 | Top-5 | Top1 | Top-3 | Top-5 | Top-1 | Top-3 | Top-5 | ||||
Baseline | 0.81 | 0.85 | 0.87 | 0.70 | 0.83 | 0.87 | 0.72 | 0.82 | 0.86 | 0.32 | 0.39 | 0.47 | 0.22 | 0.62 | 0.68 | |||
Gpt-4v | ✓ | - | - | - | - | - | - | 0.77 | 0.86 | 0.88 | 0.48 | 0.60 | 0.68 | 0.49 | 0.78 | 0.91 | ||
✓ | 0.87 | 0.95 | 0.96 | 0.87 | 0.90 | 0.91 | 0.84 | 0.90 | 0.91 | 0.55 | 0.65 | 0.74 | 0.66 | 0.74 | 0.79 | |||
✓ | 0.82 | 0.87 | 0.88 | 0.76 | 0.86 | 0.88 | 0.77 | 0.85 | 0.88 | 0.42 | 0.54 | 0.66 | 0.35 | 0.64 | 0.77 | |||
✓ | ✓ | - | - | - | - | - | - | 0.76 | 0.85 | 0.89 | 0.62 | 0.74 | 0.78 | 0.55 | 0.83 | 0.91 | ||
✓ | ✓ | - | - | - | - | - | - | 0.85 | 0.92 | 0.92 | 0.76 | 0.84 | 0.86 | 0.66 | 0.87 | 0.94 | ||
✓ | ✓ | 0.91 | 0.96 | 0.97 | 0.89 | 0.91 | 0.92 | 0.88 | 0.94 | 0.95 | 0.68 | 0.82 | 0.87 | 0.81 | 0.88 | 0.94 | ||
✓ | ✓ | ✓ | - | - | - | - | - | - | 0.90 | 0.97 | 0.99 | 0.77 | 0.86 | 0.89 | 0.83 | 0.91 | 0.95 | |
Baseline | 0.89 | 0.89 | 0.90 | 0.83 | 0.86 | 0.87 | 0.74 | 0.83 | 0.86 | 0.57 | 0.69 | 0.78 | 0.49 | 0.71 | 0.83 | |||
Gpt-4o | ✓ | - | - | - | - | - | - | 0.82 | 0.91 | 0.93 | 0.77 | 0.79 | 0.83 | 0.50 | 0.83 | 0.89 | ||
✓ | 0.92 | 0.96 | 0.98 | 0.86 | 0.88 | 0.88 | 0.93 | 0.98 | 0.98 | 0.86 | 0.91 | 0.95 | 0.82 | 0.93 | 0.97 | |||
✓ | 0.89 | 0.95 | 0.95 | 0.85 | 0.89 | 0.89 | 0.92 | 0.97 | 0.97 | 0.74 | 0.85 | 0.92 | 0.58 | 0.74 | 0.85 | |||
✓ | ✓ | - | - | - | - | - | - | 0.93 | 0.97 | 0.97 | 0.85 | 0.91 | 0.93 | 0.68 | 0.85 | 0.90 | ||
✓ | ✓ | - | - | - | - | - | - | 0.95 | 0.98 | 0.98 | 0.87 | 0.93 | 0.96 | 0.79 | 0.94 | 0.96 | ||
✓ | ✓ | 0.93 | 0.97 | 0.98 | 0.88 | 0.91 | 0.91 | 0.96 | 0.98 | 0.99 | 0.89 | 0.94 | 0.99 | 0.82 | 0.94 | 0.96 | ||
✓ | ✓ | ✓ | - | - | - | - | - | - | 0.97 | 0.99 | 0.99 | 0.89 | 0.95 | 1.00 | 0.85 | 0.96 | 0.97 |
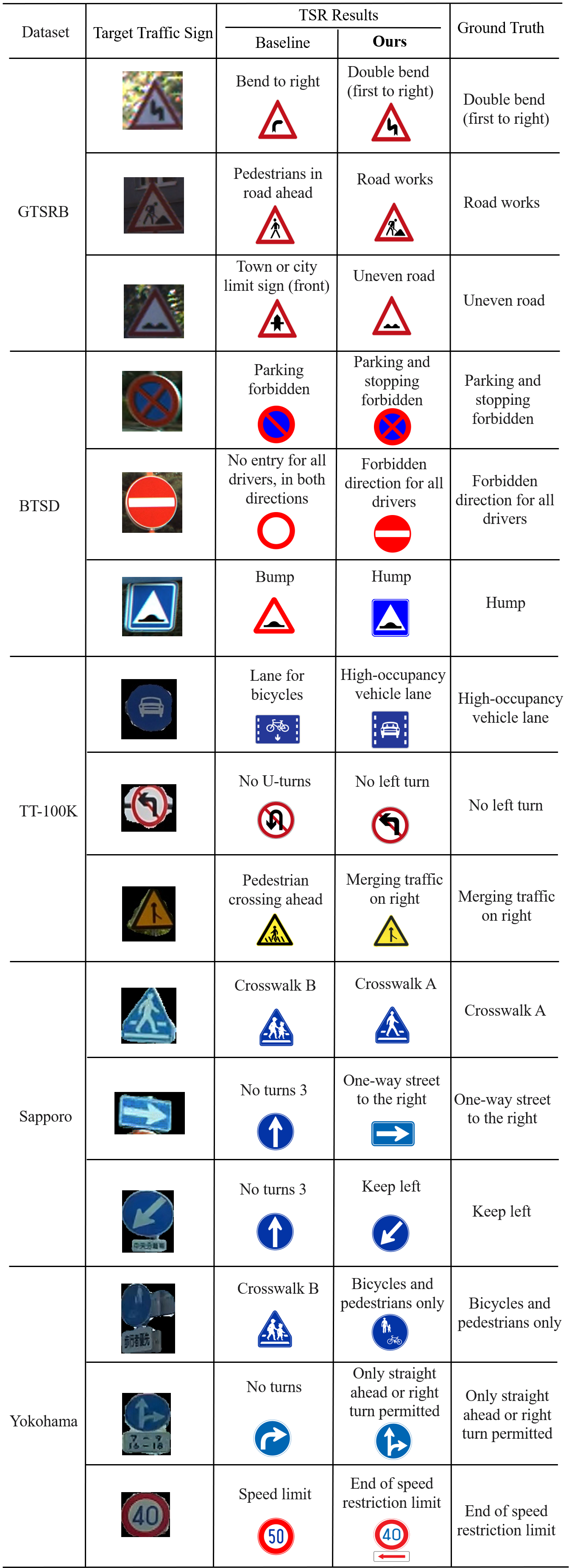
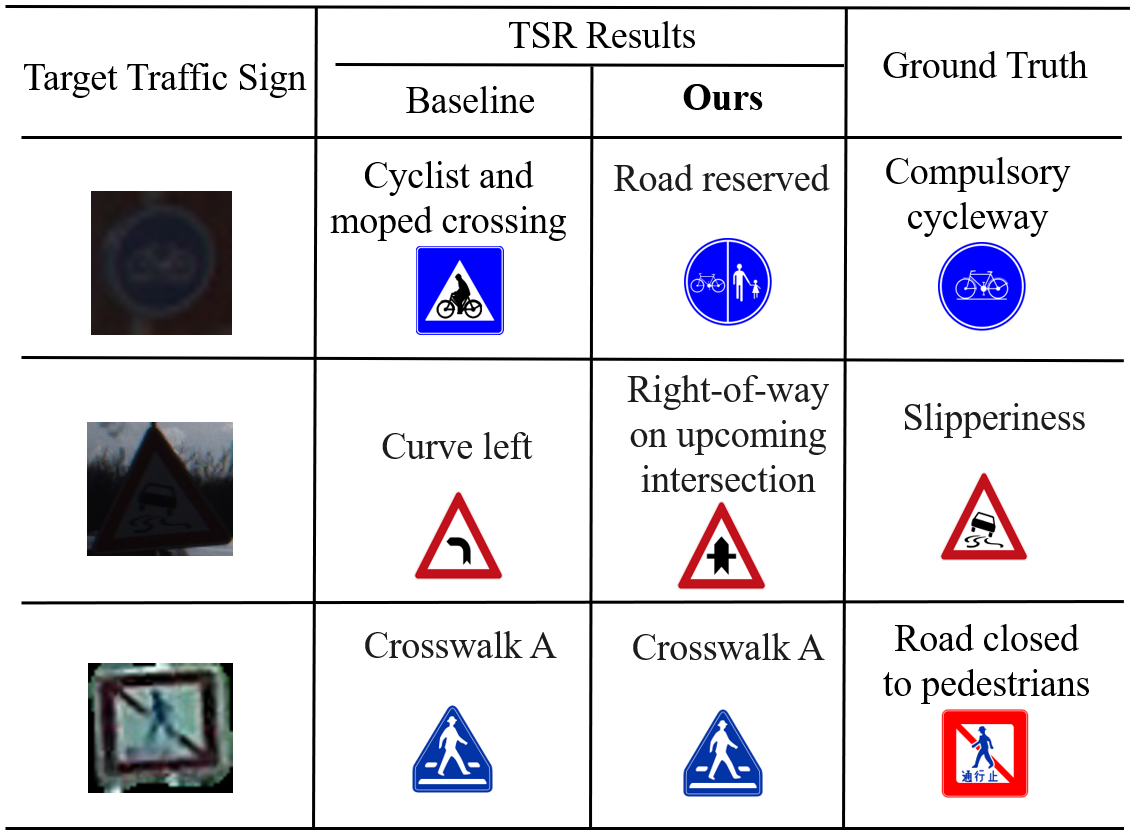
Figure 6 shows an example of the recognition results of the proposed method. We show the detailed prompts and generated descriptions for our think twice before recognizing strategy. As shown in Fig. 6, when generating the context descriptions, the center coordinates of the target traffic sign are provided in the prompts to help the LMM to accurately locate the target traffic sign among multiple traffic signs, such as Center Line (910, 271). In addition, the prior traffic sign hypothesis allows the LMM to filter irrelevant answers among all traffic sign candidates. When generating characteristic descriptions, we carefully design the prompts to fully allow LMM to identify key features such as the shape, color, and composition of traffic signs. The LMM performs few-shot in-context learning and generates a brief characteristic description for each traffic sign. By converting the generating descriptions using the LMM’s strong recognition of image features, our method reduces the cross-domain discrepancy between the template and the target traffic sign images. For generating the differential descriptions, we input similar traffic signs into the LMM to strengthen the fine-grained recognition capability of the LMM by emphasizing the differences between similar traffic signs. All input prompts in our method are simple and uniform and do not need to be specially adjusted for different target traffic signs. Figure 7 shows the recognition examples on the TT-100K dataset. For cross-country traffic signs, the multi-step thinking is general and requires no specific adjustments.
LMM | Prior hypothesis | Center coordinates | TT-100K | Sapporo | Yokohama | ||||||
---|---|---|---|---|---|---|---|---|---|---|---|
Top-1 | Top-3 | Top-5 | Top-1 | Top-3 | Top-5 | Top-1 | Top-3 | Top-5 | |||
Gpt-4v | 0.87 | 0.92 | 0.92 | 0.68 | 0.86 | 0.88 | 0.78 | 0.84 | 0.88 | ||
✓ | 0.86 | 0.92 | 0.93 | 0.60 | 0.76 | 0.76 | 0.73 | 0.88 | 0.91 | ||
✓ | 0.85 | 0.93 | 0.95 | 0.67 | 0.87 | 0.88 | 0.74 | 0.88 | 0.91 | ||
✓ | ✓ | 0.90 | 0.97 | 0.99 | 0.77 | 0.86 | 0.89 | 0.83 | 0.91 | 0.95 | |
Gpt-4o | 0.90 | 0.95 | 0.98 | 0.80 | 0.88 | 0.89 | 0.80 | 0.92 | 0.95 | ||
✓ | 0.86 | 0.90 | 0.93 | 0.75 | 0.82 | 0.84 | 0.77 | 0.90 | 0.92 | ||
✓ | 0.90 | 0.95 | 0.97 | 0.78 | 0.88 | 0.90 | 0.79 | 0.92 | 0.95 | ||
✓ | ✓ | 0.97 | 0.99 | 0.99 | 0.89 | 0.95 | 1.00 | 0.85 | 0.96 | 0.97 |
LMM | Change Thinking | GTSRB | BTSD | TT-100K | Sapporo | Yokohama | ||||||||||
---|---|---|---|---|---|---|---|---|---|---|---|---|---|---|---|---|
Top-1 | Top-3 | Top-5 | Top-1 | Top-3 | Top-5 | Top-1 | Top-3 | Top-5 | Top-1 | Top-3 | Top-5 | Top-1 | Top-3 | Top-5 | ||
Gpt-4v | w | 0.91 | 0.96 | 0.97 | 0.89 | 0.91 | 0.92 | 0.89 | 0.97 | 0.99 | 0.77 | 0.87 | 0.89 | 0.83 | 0.92 | 0.95 |
w/o | 0.91 | 0.96 | 0.97 | 0.89 | 0.91 | 0.92 | 0.90 | 0.97 | 0.99 | 0.77 | 0.86 | 0.89 | 0.83 | 0.91 | 0.95 | |
Gpt-4o | w | 0.93 | 0.97 | 0.98 | 0.88 | 0.91 | 0.91 | 0.96 | 0.98 | 0.99 | 0.89 | 0.95 | 1.00 | 0.87 | 0.97 | 0.98 |
w/o | 0.93 | 0.97 | 0.98 | 0.88 | 0.91 | 0.91 | 0.97 | 0.99 | 0.99 | 0.89 | 0.95 | 1.00 | 0.85 | 0.96 | 0.97 |
IV-C Ablation Studies
IV-C1 Different Thinking Strategies
To further verify the effectiveness of the proposed multi-step thinking strategy and explore the respective effectiveness of each proposed description. We calculated the Top- fine-grained TSR performance for different thinking strategies on five datasets as shown in Table II. When there are no context, characteristic, and differential descriptions, target traffic signs are directly input into LMM for recognition. Table II demonstrates that the results of baseline exhibit the lowest accuracy on all datasets compared with the performing of thinking strategy. As the thinking step increases, the Top- TSR recognition accuracy increases, which proves the effectiveness of the proposed method. Besides, the results also show that each proposed description has a positive impact on improving the fine-grained TSR capabilities of LMM. Comparing the results of using only one kind of description, the characteristic description contributes most to the TSR recognition accuracy. Through characteristic descriptions, LMM can consider the features of the target traffic sign and the descriptions of template traffic signs, thereby improving fine-grained recognition capabilities. In addition, context and differential descriptions show the ability to further optimize fine-grained TSR recognition on all five datasets, which is consistent with our hypothesis.
Figure 8 shows the recognition examples of the baseline and proposed method under five datasets (Gpt-4o). Compared to the baseline, our strategy shows stable recognition performance for traffic signs in real-world scenarios and can be generalized to recognize traffic signs in different countries. In particular, as shown in Fig. 8, most of the identified traffic signs of the baseline and our strategy show only minor differences. This illustrates that the proposed strategy helps the LMM to fully consider the diversity and similarity of traffic signs to perform accurate fine-grained level recognition. Figure 9 shows examples of recognition errors of the proposed method. When traffic signs are too blurred, it is difficult to understand the traffic sign images for accurate recognition.
IV-C2 Hypothesis and Coordinate
To validate the effectiveness of the proposed prior traffic sign hypothesis and center coordinate prompt optimization method, we conducted experiments on the effectiveness of different context description generation methods on three real-world datasets, TT-100K, Sapporo, and Yokohama. Note that all results in Table III use context descriptions, characteristic descriptions, and difference descriptions for multi-step thinking. As shown in Table III, without the prior traffic sign hypothesis and center coordinate prompt optimization, i.e., with only simple image background descriptions in the contextual description, the Top- fine-grained TSR performance is reasonably similar to the accuracy in Table II using only the characteristic and differential descriptions. Since the characteristic and differential descriptions are performed, only simple background descriptions of images in the context description have limited contribution to improving the fine-grained capability of the LMM. The situation is also similar when only the center coordinates optimization is performed. Although the LMM can locate the target traffic sign from multiple traffic signs in the original road image and simply describe the features. However, the simple descriptions in the contextual description contribute limited since characteristic descriptions are already provided. When only the prior traffic sign hypothesis is performed without center coordinate prompt optimization, it is difficult for the LMM to locate the target traffic sign from multiple traffic signs in the original road image, thus generating confusing descriptions. The confusing descriptions negatively affect the accuracy. When both the prior traffic sign hypothesis and the center ordinate prompt optimization are performed, the Top- fine-grained TSR performance is improved by locating the target traffic signs and filtering irrelevant answers.
IV-C3 Thinking Orders
In Table IV, we validate the comparison of TSR performance under different thinking steps. For the GTSRB and BTSD datasets, we change the order of thinking for characteristic and differential descriptions. For the TT-100K, Sapporo, and Yokohama datasets, we change the thinking order of context and characteristic descriptions. The experimental results are shown in Table IV. After the order of thinking is changed, the TSR performance remains almost the same as the original, which shows the robustness of our method. Since the cues obtained by LMM are the same even when the order of thinking is changed, there is no significant difference in recognition accuracy.
IV-C4 Extensibility
Previous experiments demonstrate that the proposed multi-step thinking can be easily extended to different LMMs and maintains robust performance. In addition, in our designed traffic sign extraction module, the segmentation model is not limited to a specific model and can easily be extended to advanced models. Figure 10 shows traffic sign extraction examples with segment anything model 2 (SAM 2) [68] and ViT-Adapter [69]. As shown in Fig. 10, under different segmentation models, target traffic signs are extracted through the designed extraction module. The most advanced segmentation model such as SAM 2 performs better extraction on traffic signs.
IV-C5 Inference Speed
Table V shows the inference speed of the proposed method. When the segmentation model is ViT-Adapter, the inference speed is 0.4s per road image, and the SAM 2-based extraction module can achieve real-time extraction. For LMM, the multistep thinking of Gpt-4o is faster than Gpt-4v by 0.4s and the fastest total inference speed is 1.2 seconds. Since our method can be extended to future LMMs, the inference speed has the potential to be faster.
V Disscussion
V-A Test Set Contamination
Since LMMs are trained on large amounts of internet data, there are concerns and speculation that they have memorized public benchmarks [70]. In this paper, we not only tested our method on three public benchmark datasets (GTSRB, BTSD, TT-100K) but also on two private datasets (Sapporo and Yokohama). Our method shows consistent and robust performance on all five datasets. It is impossible that these two private datasets had been used for training. Therefore, there is no test set contamination in our method.
V-B Importance and Application
Our method can achieve efficient TSR in natural dynamic road environments and maintain stable TSR performance in different countries without the need for training data. This highlights the significant application value of our approach. Collecting and preparing data for training and testing across various countries is costly, especially given the differing data and privacy policies, as well as the challenges in obtaining data from less developed regions. By reducing the need for extensive data collection, our approach not only reduces costs but also promotes equity. Current advanced driving assistance systems and autonomous driving technologies are often limited to certain regions, neglecting less developed areas. By achieving effective cross-country TSR, our method has the potential to extend the technologies to underserved regions, thereby promoting greater equity.
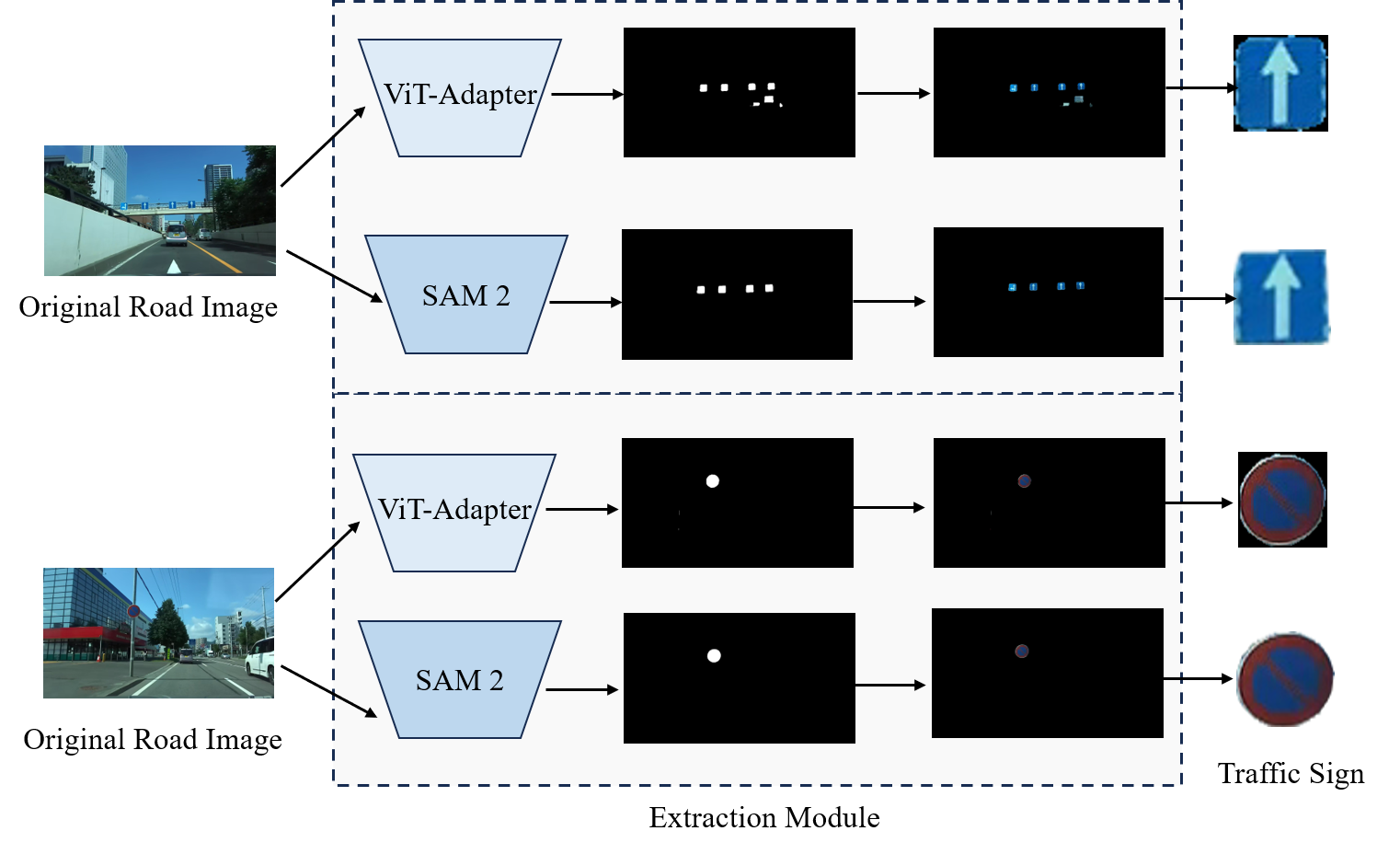
Extraction | LMM | Inference Speed |
---|---|---|
ViT-Adapter-base | Gpt-4v | 2.0s |
Gpt-4o | 1.6s | |
SAM 2-base | Gpt-4v | 1.6s |
Gpt-4o | 1.2s |
V-C Limitation
V-C1 Determination of Similar Traffic Signs
In this work, we designed the differential descriptions for the LMMs and demonstrated the effectiveness of the differential descriptions. However, similar traffic signs are determined based on expert knowledge to generate the differential descriptions. In future work, automatic methods for determining similar traffic signs need to be explored.
V-C2 Performance in Different Weather Conditions
In this paper, five datasets including three public datasets (GTSRB, BTSD, TT-100K), and two private datasets (Sapporo, Yokohama) are used to verify the performance of the proposed method. However, all five datasets were taken under sunny weather. As a result, the traffic sign images are relatively clear. Under the weather such as rain, fog, and snow), the traffic sign images can become blurred, which affects the TSR performance. Improving TSR performance in this condition is a direction we look forward to exploring in the future.
VI Conclusion
In this paper, we proposed the think twice before recognizing strategy for constructing a general fine-grained TSR method. The proposed framework is simple, effective, and easily extensible. The designed multi-thinking strategy stimulates the fine-grained recognition ability of LMM for traffic signs. Experimental results conducted on three benchmark datasets and two real-world datasets demonstrate the effectiveness of the proposed method.
References
- [1] Xinchen Liu, Wu Liu, Tao Mei, and Huadong Ma. Provid: Progressive and multimodal vehicle reidentification for large-scale urban surveillance. IEEE Transactions on Multimedia, 20(3):645–658, 2017.
- [2] Hong Tan, Fuquan Zhao, Han Hao, and Zongwei Liu. Cost analysis of road traffic crashes in china. International Journal of Injury Control and Safety Promotion, 27(3):385–391, 2020.
- [3] Nadra Ben Romdhane, Hazar Mliki, and Mohamed Hammami. An improved traffic signs recognition and tracking method for driver assistance system. In Proceedings of the IEEE International Conference on Computer and Information Science (ICIS), pages 1–6, 2016.
- [4] Song Yucong and Guo Shuqing. Traffic sign recognition based on hog feature extraction. Journal of Measurements in Engineering, 9(3):142–155, 2021.
- [5] Fatin Zaklouta and Bogdan Stanciulescu. Segmentation masks for real-time traffic sign recognition using weighted hog-based trees. In Proceedings of the International IEEE Conference on Intelligent Transportation Systems (ITSC), pages 1954–1959. IEEE, 2011.
- [6] Fatin Zaklouta and Bogdan Stanciulescu. Real-time traffic sign recognition using spatially weighted hog trees. In Proceedings of the International Conference on Advanced Robotics (ICAR), pages 61–66. IEEE, 2011.
- [7] Zhiyong Huang, Yuanlong Yu, Jason Gu, and Huaping Liu. An efficient method for traffic sign recognition based on extreme learning machine. IEEE Transactions on Cybernetics, 47(4):920–933, 2016.
- [8] David G Lowe. Distinctive image features from scale-invariant keypoints. International Journal of Computer Vision, 60:91–110, 2004.
- [9] Shouyi Yin, Peng Ouyang, Leibo Liu, Yike Guo, and Shaojun Wei. Fast traffic sign recognition with a rotation invariant binary pattern based feature. Sensors, 15(1):2161–2180, 2015.
- [10] Zumra Malik and Imran Siddiqi. Detection and recognition of traffic signs from road scene images. In Proceedings of the International Conference on Frontiers of Information Technology (FIT), pages 330–335, 2014.
- [11] Kehua Guo, Zheng Wu, Weizheng Wang, Sheng Ren, Xiaokang Zhou, Thippa Reddy Gadekallu, Entao Luo, and Chao Liu. Grtr: Gradient rebalanced traffic sign recognition for autonomous vehicles. IEEE Transactions on Automation Science and Engineering, 2023.
- [12] Zhongqin Bi, Ling Yu, Honghao Gao, Ping Zhou, and Hongyang Yao. Improved vgg model-based efficient traffic sign recognition for safe driving in 5g scenarios. International Journal of Machine Learning and Cybernetics, 12:3069–3080, 2021.
- [13] Arindam Baruah, Rakesh Kumar, and Meenu Gupta. Traffic sign recognition using deep learning neural network and spatial transformer. In Proceedings of the International Conference on Advances in Computing, Communication and Applied Informatics (ACCAI), pages 1–8, 2023.
- [14] Hengliang Luo, Yi Yang, Bei Tong, Fuchao Wu, and Bin Fan. Traffic sign recognition using a multi-task convolutional neural network. IEEE Transactions on Intelligent Transportation Systems, 19(4):1100–1111, 2017.
- [15] Omid Nejati Manzari, Amin Boudesh, and Shahriar B Shokouhi. Pyramid transformer for traffic sign detection. In Proceedings of the International Conference on Computer and Knowledge Engineering (ICCKE), pages 112–116. IEEE, 2022.
- [16] Qiang Luo and Wenbin Zheng. Pre-locator incorporating swin-transformer refined classifier for traffic sign recognition. Intelligent Automation & Soft Computing, 37(2), 2023.
- [17] Yunfei Guo, Wei Feng, Fei Yin, and Cheng-Lin Liu. Signparser: An end-to-end framework for traffic sign understanding. International Journal of Computer Vision, 132(3):805–821, 2024.
- [18] Yuping Zheng and Weiwei Jiang. Evaluation of vision transformers for traffic sign classification. Wireless Communications and Mobile Computing, 2022:1–14, 2022.
- [19] Economic Commission for Europe-Inland Tansport Committee et al. Convention on road signs and signals. United Nations Treaty Series, 1091:3, 1968.
- [20] FeiXiang Ren, Jinsheng Huang, Ruyi Jiang, and Reinhard Klette. General traffic sign recognition by feature matching. In Proceedings of the IEEE International Conference Image and Vision Computing New Zealand (IVCNZ), pages 409–414, 2009.
- [21] Catur Supriyanto, Ardytha Luthfiarta, and Junta Zeniarja. An unsupervised approach for traffic sign recognition based on bag-of-visual-words. In Proceedings of the International Conference on Information Technology and Electrical Engineering (ICITEE), pages 1–4, 2016.
- [22] Shichao Zhou, Chenwei Deng, Zhengquan Piao, and Baojun Zhao. Few-shot traffic sign recognition with clustering inductive bias and random neural network. Pattern Recognition, 100:107160, 2020.
- [23] Yaozong Gan, Guang Li, Ren Togo, Keisuke Maeda, Takahiro Ogawa, and Miki Haseyama. Zero-shot traffic sign recognition based on midlevel feature matching. Sensors, 23(23):9607, 2023.
- [24] Gan Yaozong, Li Guang, Togo Ren, Maeda Keisuke, Ogawa Takahiro, and Haseyama Miki. A note on traffic sign recognition based on vision transformer adapter using visual feature matching. ITE technical report, 47(6):1–4, 2023.
- [25] Tom Brown, Benjamin Mann, Nick Ryder, Melanie Subbiah, Jared D Kaplan, Prafulla Dhariwal, Arvind Neelakantan, Pranav Shyam, Girish Sastry, Amanda Askell, et al. Language models are few-shot learners. In Proceedings of the Annual Conference on Advances in Neural Information Processing Systems (NeurIPS), volume 33, pages 1877–1901, 2020.
- [26] Aakanksha Chowdhery, Sharan Narang, Jacob Devlin, Maarten Bosma, Gaurav Mishra, Adam Roberts, Paul Barham, Hyung Won Chung, Charles Sutton, Sebastian Gehrmann, et al. Palm: Scaling language modeling with pathways. Journal of Machine Learning Research, 24(240):1–113, 2023.
- [27] Gpt-4 technical report, 2024.
- [28] Hugo Touvron, Thibaut Lavril, Gautier Izacard, Xavier Martinet, Marie-Anne Lachaux, Timothée Lacroix, Baptiste Rozière, Naman Goyal, Eric Hambro, Faisal Azhar, et al. Llama: Open and efficient foundation language models. arXiv preprint arXiv:2302.13971, 2023.
- [29] Sébastien Bubeck, Varun Chandrasekaran, Ronen Eldan, Johannes Gehrke, Eric Horvitz, Ece Kamar, Peter Lee, Yin Tat Lee, Yuanzhi Li, Scott Lundberg, et al. Sparks of artificial general intelligence: Early experiments with gpt-4. arXiv, pages 1–155, 2023.
- [30] Maria Tsimpoukelli, Jacob L Menick, Serkan Cabi, SM Eslami, Oriol Vinyals, and Felix Hill. Multimodal few-shot learning with frozen language models. In Proceedings of the Annual Conference on Advances in Neural Information Processing Systems (NeurIPS), volume 34, pages 200–212, 2021.
- [31] Openai. gpt-4o system card. 2024.
- [32] Openai. gpt-4v(ision) system card. 2023.
- [33] Openai. gpt-4v(ision) technical work and authors. 2023.
- [34] Li Yunxin, Hu Baotian, Chen Xinyu, Ma Lin, Xu Yong, and Zhang Min. Lmeye: An interactive perception network for large language models. IEEE Transactions on Multimedia, pages 1–13, 2024.
- [35] Yinghui Xing, Qirui Wu, De Cheng, Shizhou Zhang, Guoqiang Liang, Peng Wang, and Yanning Zhang. Dual modality prompt tuning for vision-language pre-trained model. IEEE Transactions on Multimedia, 2023.
- [36] Han Fang, Pengfei Xiong, Luhui Xu, and Wenhan Luo. Transferring image-clip to video-text retrieval via temporal relations. IEEE Transactions on Multimedia, 25:7772–7785, 2022.
- [37] Fang Peng, Xiaoshan Yang, Linhui Xiao, Yaowei Wang, and Changsheng Xu. Sgva-clip: Semantic-guided visual adapting of vision-language models for few-shot image classification. IEEE Transactions on Multimedia, 2023.
- [38] Yaozong Gan, Guang Li, Ren Togo, Keisuke Maeda, Takahiro Ogawa, and Miki Haseyama. Cross-domain few-shot in-context learning for enhancing traffic sign recognition. arXiv preprint arXiv:2407.05814, 2024.
- [39] Ou Zheng, Dongdong Wang, Zijin Wang, and Shengxuan Ding. Chat-gpt is on the horizon: Could a large language model be suitable for intelligent traffic safety research and applications? ArXiv, 2023.
- [40] Can Cui, Yunsheng Ma, Xu Cao, Wenqian Ye, Yang Zhou, Kaizhao Liang, Jintai Chen, Juanwu Lu, Zichong Yang, Kuei-Da Liao, et al. A survey on multimodal large language models for autonomous driving. In Proceedings of the IEEE/CVF Winter Conference on Applications of Computer Vision (WACV), pages 958–979, 2024.
- [41] Merve Can Kus, Muhittin Gokmen, and Sima Etaner-Uyar. Traffic sign recognition using scale invariant feature transform and color classification. In Proceedings of the International Symposium on Computer and Information Sciences (ISCIS), pages 1–6, 2008.
- [42] Abdulrahman Kerim and Mehmet Önder Efe. Recognition of traffic signs with artificial neural networks: A novel dataset and algorithm. In Proceedings of the International Conference on Artificial Intelligence in Information and Communication (ICAIIC), pages 171–176, 2021.
- [43] Jianming Zhang, Wei Wang, Chaoquan Lu, Jin Wang, and Arun Kumar Sangaiah. Lightweight deep network for traffic sign classification. Annals of Telecommunications, 75:369–379, 2020.
- [44] U Syed Abudhagir and N Ashok. Highly sensitive deep learning model for road traffic sign identification. Mathematical Statistician and Engineering Applications, 71(4):3194–3205, 2022.
- [45] Yanzhao Zhu and Wei Qi Yan. Traffic sign recognition based on deep learning. Multimedia Tools and Applications, 81(13):17779–17791, 2022.
- [46] Alexey Dosovitskiy, Lucas Beyer, Alexander Kolesnikov, Dirk Weissenborn, Xiaohua Zhai, Thomas Unterthiner, Mostafa Dehghani, Matthias Minderer, Georg Heigold, Sylvain Gelly, Jakob Uszkoreit, and Neil Houlsby. An image is worth 16x16 words: Transformers for image recognition at scale. In Proceedings of the International Conference on Learning Representations (ICLR), pages 1–21, 2021.
- [47] Ze Liu, Yutong Lin, Yue Cao, Han Hu, Yixuan Wei, Zheng Zhang, Stephen Lin, and Baining Guo. Swin transformer: Hierarchical vision transformer using shifted windows. In Proceedings of the IEEE/CVF International Conference on Computer Vision (ICCV), pages 10012–10022, 2021.
- [48] Weipeng Cao, Yuhao Wu, Chinmay Chakraborty, Dachuan Li, Liang Zhao, and Soumya Kanti Ghosh. Sustainable and transferable traffic sign recognition for intelligent transportation systems. IEEE Transactions on Intelligent Transportation Systems, 2022.
- [49] Yupeng Chang, Xu Wang, Jindong Wang, Yuan Wu, Kaijie Zhu, Hao Chen, Linyi Yang, Xiaoyuan Yi, Cunxiang Wang, Yidong Wang, et al. A survey on evaluation of large language models. arXiv, pages 1–45, 2023.
- [50] Gemini Team, Rohan Anil, Sebastian Borgeaud, Yonghui Wu, Jean-Baptiste Alayrac, Jiahui Yu, Radu Soricut, Johan Schalkwyk, Andrew M Dai, Anja Hauth, et al. Gemini: a family of highly capable multimodal models. arXiv preprint arXiv:2312.11805, 2023.
- [51] Haoxuan You, Haotian Zhang, Zhe Gan, Xianzhi Du, Bowen Zhang, Zirui Wang, Liangliang Cao, Shih-Fu Chang, and Yinfei Yang. Ferret: Refer and ground anything anywhere at any granularity. arXiv preprint arXiv:2310.07704, 2023.
- [52] Hanoona Rasheed, Muhammad Maaz, Sahal Shaji, Abdelrahman Shaker, Salman Khan, Hisham Cholakkal, Rao M Anwer, Erix Xing, Ming-Hsuan Yang, and Fahad S Khan. Glamm: Pixel grounding large multimodal model. arXiv preprint arXiv:2311.03356, 2023.
- [53] Can Cui, Yunsheng Ma, Xu Cao, Wenqian Ye, and Ziran Wang. Drive as you speak: Enabling human-like interaction with large language models in autonomous vehicles. In Proceedings of the IEEE/CVF Winter Conference on Applications of Computer Vision (WACV), pages 902–909, 2024.
- [54] Satoshi Suzuki et al. Topological structural analysis of digitized binary images by border following. Computer Vision, Graphics, and Image Processing, 30(1):32–46, 1985.
- [55] Johannes Stallkamp, Marc Schlipsing, Jan Salmen, and Christian Igel. Man vs. computer: Benchmarking machine learning algorithms for traffic sign recognition. Neural Networks, 32:323–332, 2012.
- [56] Markus Mathias, Radu Timofte, Rodrigo Benenson, and Luc Van Gool. Traffic sign recognition—how far are we from the solution? In Proceedings of the International Joint Conference on Neural Networks (IJCNN), pages 1–8, 2013.
- [57] Zhe Zhu, Dun Liang, Songhai Zhang, Xiaolei Huang, Baoli Li, and Shimin Hu. Traffic-sign detection and classification in the wild. In Proceedings of the IEEE/CVF Conference on Computer Vision and Pattern Recognition (CVPR), pages 2110–2118, 2016.
- [58] Jianwei Yang, Hao Zhang, Feng Li, Xueyan Zou, Chunyuan Li, and Jianfeng Gao. Set-of-mark prompting unleashes extraordinary visual grounding in gpt-4v. arXiv, pages 1–23, 2023.
- [59] Gao Huang, Zhuang Liu, Laurens Van Der Maaten, and Kilian Q Weinberger. Densely connected convolutional networks. In Proceedings of the IEEE/CVF Conference on Computer Vision and Pattern Recognition (CVPR), pages 4700–4708, 2017.
- [60] Mingxing Tan and Quoc Le. Efficientnet: Rethinking model scaling for convolutional neural networks. In Proceedings of the International Conference on Machine Learning (ICML), pages 6105–6114, 2019.
- [61] Jia Li and Zengfu Wang. Real-time traffic sign recognition based on efficient cnns in the wild. IEEE Transactions on Intelligent Transportation Systems, 20(3):975–984, 2018.
- [62] Sachin Mehta and Mohammad Rastegari. Mobilevit: light-weight, general-purpose, and mobile-friendly vision transformer. arXiv preprint arXiv:2110.02178, 2021.
- [63] Ze Liu, Han Hu, Yutong Lin, Zhuliang Yao, Zhenda Xie, Yixuan Wei, Jia Ning, Yue Cao, Zheng Zhang, Li Dong, et al. Swin transformer v2: Scaling up capacity and resolution. In Proceedings of the IEEE/CVF Conference on Computer Vision and Pattern Recognition (CVPR), pages 12009–12019, 2022.
- [64] Kaiming He, Xinlei Chen, Saining Xie, Yanghao Li, Piotr Dollár, and Ross Girshick. Masked autoencoders are scalable vision learners. In Proceedings of the IEEE/CVF Conference on Computer Vision and Pattern Recognition (CVPR), pages 16000–16009, 2022.
- [65] Hugo Touvron, Matthieu Cord, Matthijs Douze, Francisco Massa, Alexandre Sablayrolles, and Hervé Jégou. Training data-efficient image transformers & distillation through attention. In Proceedings of the International Conference on Machine Learning (ICML), pages 10347–10357, 2021.
- [66] Alec Radford, Jong Wook Kim, Chris Hallacy, Aditya Ramesh, Gabriel Goh, Sandhini Agarwal, Girish Sastry, Amanda Askell, Pamela Mishkin, Jack Clark, et al. Learning transferable visual models from natural language supervision. In Proceedings of the International Conference on Machine Learning (ICML), pages 8748–8763, 2021.
- [67] Yuxin Fang, Quan Sun, Xinggang Wang, Tiejun Huang, Xinlong Wang, and Yue Cao. Eva-02: A visual representation for neon genesis. arXiv preprint arXiv:2303.11331, 2023.
- [68] Nikhila Ravi, Valentin Gabeur, Yuan-Ting Hu, Ronghang Hu, Chaitanya Ryali, Tengyu Ma, Haitham Khedr, Roman Rädle, Chloe Rolland, Laura Gustafson, Eric Mintun, Junting Pan, Kalyan Vasudev Alwala, Nicolas Carion, Chao-Yuan Wu, Ross Girshick, Piotr Dollár, and Christoph Feichtenhofer. Sam 2: Segment anything in images and videos. arXiv preprint arXiv:2408.00714, 2024.
- [69] Zhe Chen, Yuchen Duan, Wenhai Wang, Junjun He, Tong Lu, Jifeng Dai, and Yu Qiao. Vision transformer adapter for dense predictions. In Proceedings of the International Conference on Learning Representations (ICLR), pages 1–20, 2023.
- [70] Yonatan Oren, Nicole Meister, Niladri Chatterji, Faisal Ladhak, and Tatsunori B Hashimoto. Proving test set contamination in black box language models. In Proceedings of the International Conference on Learning Representations (ICLR), pages 1–19, 2024.
![]() |
Yaozong Gan received the B.S. degree from SiChuan University, China, in 2020 and the M.S. degree in Information Science from Hokkaido University, Japan, in 2023. He is currently pursuing the Ph.D. degree with the Graduate School of Information Science and Technology at Hokkaido University. His research interests include large multimodal models, autonomous driving, and multimodal understanding of sports videos (especially soccer videos). He has served as a reviewer for ICLR and ACM MM. He is a member of IEEE. |
![]() |
Guang Li received the B.S. degree in Software Engineering and the B.A. dual degree in Japanese from Dalian University of Technology, China, in 2019, and the M.S. and Ph.D. degrees in Information Science from Hokkaido University, Japan, in 2022 and 2023, respectively. He is currently a Specially Appointed Assistant Professor with the Education and Research Center for Mathematical and Data Science, Hokkaido University. His research interests include Dataset Distillation, Self-Supervised Learning, Data-Centric AI, and Medical Image Analysis. He is a member of IEEE. He has served as Area Chair for ACM MM and as a Program Committee Member for top-tier conferences such as NeurIPS, ICLR, CVPR, and ECCV. He also served as a Reviewer for prestigious journals such as TIP, TMI, TMM, and TCSVT. |
![]() |
Ren Togo received the B.S. degree in Health Sciences from Hokkaido University, Japan, in 2015, and the M.S. and Ph.D. degrees from the Graduate School of Information Science and Technology, Hokkaido University, in 2017 and 2019, respectively. He is also a Radiological Technologist. He is currently a Specially Appointed Assistant Professor with the Laboratory of Media Dynamics, Faculty of Information Science and Technology, Hokkaido University. His research interests include machine learning and its applications. He is a member of the IEEE, ACM, AAAI, and IEICE. |
![]() |
Keisuke Maeda received his B.S., M.S., and Ph.D. degrees in Electronics and Information Engineering from Hokkaido University, Japan in 2015, 2017, and 2019. At present, he is currently a Specially Appointed Associate Professor in the Data-Driven Interdisciplinary Research Emergence Department, Hokkaido University. His research interests include multimodal signal processing and machine learning and its applications. He is an IEEE and IEICE member. |
![]() |
Takahiro Ogawa received his B.S., M.S. and Ph.D. degrees in Electronics and Information Engineering from Hokkaido University, Japan in 2003, 2005 and 2007, respectively. He joined Graduate School of Information Science and Technology, Hokkaido University in 2008. He is currently a professor in the Faculty of Information Science and Technology, Hokkaido University. His research interests are AI, IoT and big data analysis for multimedia signal processing and its applications. He was a special session chair of IEEE ISCE2009, a Doctoral Symposium Chair of ACM ICMR2018, an organized session chair of IEEE GCCE2017-2019, a TPC Vice Chair of IEEE GCCE2018, a Conference Chair of IEEE GCCE2019, etc. He has been also an Associate Editor of ITE Transactions on Media Technology and Applications. He is a senior member of IEEE and a member of ACM, IEICE and ITE. |
![]() |
Miki Haseyama received her B.S., M.S. and Ph.D. degrees in Electronics from Hokkaido University, Japan in 1986, 1988 and 1993, respectively. She joined the Graduate School of Information Science and Technology, Hokkaido University as an associate professor in 1994. She was a visiting associate professor of Washington University, USA from 1995 to 1996. She is currently a professor in the Faculty of Information Science and Technology Division of Media and Network Technologies, Hokkaido University. Her research interests include image and video processing and its development into semantic analysis. She has been a Vice-President of the Institute of Image Information and Television Engineers, Japan (ITE), an Editor-in-Chief of ITE Transactions on Media Technology and Applications, a Director, International Coordination and Publicity of The Institute of Electronics, Information and Communication Engineers (IEICE). She is a member of the IEEE, IEICE, Institute of Image Information and Television Engineers (ITE) and Acoustical Society of Japan (ASJ). |