The Threat of Offensive AI to Organizations
Abstract.
AI has provided us with the ability to automate tasks, extract information from vast amounts of data, and synthesize media that is nearly indistinguishable from the real thing. However, positive tools can also be used for negative purposes. In particular, cyber adversaries can use AI to enhance their attacks and expand their campaigns.
Although offensive AI has been discussed in the past, there is a need to analyze and understand the threat in the context of organizations. For example, how does an AI-capable adversary impact the cyber kill chain? Does AI benefit the attacker more than the defender? What are the most significant AI threats facing organizations today and what will be their impact on the future?
In this survey, we explore the threat of offensive AI on organizations. First, we present the background and discuss how AI changes the adversary’s methods, strategies, goals, and overall attack model. Then, through a literature review, we identify 33 offensive AI capabilities which adversaries can use to enhance their attacks. Finally, through a user study spanning industry and academia, we rank the AI threats and provide insights on the adversaries.
1. Introduction
For decades, organizations, including government agencies, hospitals, and financial institutions, have been the target of cyber attacks (Knake, 2017; Mattei, 2017; Tariq, 2018). These cyber attacks have been carried out by experienced hackers that has involved manual effort. In recent years there has been a boom in the development of artificial intelligence (AI), which has enabled the creation of software tools that have helped to automate tasks such as prediction, information retrieval, and media synthesis. Throughout this period, members of academia and industry have utilized AI111In this paper, we consider machine learning to be a subset of AI technologies. in the context of improving the state of cyber defense (Mirsky et al., 2018; Liu and Lang, 2019; Mahadi et al., 2018) and threat analysis (272, 2021; Ucci et al., 2019; Cohen et al., 2020). However, AI is a double edged sword, and attackers can utilize it to improve their malicious campaigns.
Recently, there has been a lot of work done to identify and mitigate attacks on AI-based systems (adversarial machine learning) (Barreno et al., 2010; Huang et al., 2011; Joseph et al., 2018; Biggio and Roli, 2018; Chakraborty et al., 2018; Papernot et al., 2018). However, an AI-capable adversary can do much more than poison or fool a machine learning model. Adversaries can improve their tactics to launch attacks that were not possible before. For example, with deep learning one can perform highly effective spear phishing attacks by impersonating a superior’s face and voice (Mirsky and Lee, 2021; Stupp, [n.d.]). It is also possible to improve stealth capabilities by using automation to perform lateral movement through a network, limiting command and control (C&C) communication (Zelinka et al., 2018; Truong et al., 2019). Other capabilities include the use of AI to find zero-day vulnerabilities in software, automate reverse engineering, exploit side channels efficiently, build realistic fake personas, and to perform many more malicious activities with improved efficacy (more examples are presented later in section 4).
1.1. Goal
In this work, we provide a survey of knowledge on offensive AI in the context of enterprise security. The goal of this paper is to help the community (1) better understand the current impact of offensive AI on organizations, (2) prioritize research and development of defensive solutions, and (3) identify trends that may emerge in the near future. This work isn’t the first to raise awareness of offensive AI. In (Brundage et al., 2018) the authors warned the community that AI can be used for unethical and criminal purposes with examples taken from various domains. In (Caldwell et al., 2020) a workshop was held that attempted to identify the potential top threats of AI in criminology. However, these works relate to the threat of AI on society overall and are not specific to organizations and their networks.
1.2. Methodology
Our survey was performed in the following way. First, we reviewed literature to identify and organize the potential threats of AI to organizations. Then, we surveyed experts from academia, industry, and government to understand which of these threats are actual concerns and why. Finally, using our survey responses, we ranked these threats to gain insights and to help identify the areas which require further attention. The survey participants were from a wide profile of organizations such as MITRE, IBM, Microsoft, Airbus, Bosch, Fujitsu, Hitachi, and Huawei.
To perform our literature review, we used the MITRE ATT&CK222https://attack.mitre.org/matrices/enterprise/ matrix as a guide. This matrix lists the common tactics (or attack steps) which an adversary performs when attacking an organization, from planning and reconnaissance leading to the final goal of exploitation. We divided the tactics among five different academic workgroups from different international institutions based on expertise. For each tactic in the MITRE ATT&CK matrix, a workgroup surveyed related works to see how AI has and can be used by an attacker to improve their tactics and techniques. Finally, each workgroup cross inspected each other’s content to ensure correctness and completeness.
1.3. Main Findings
From the Literature Survey.
-
•
There are three primary motivations for an adversary to use AI: coverage, speed, and success.
-
•
AI introduces new threats to organizations. A few examples include the poisoning of machine learning models, theft of credentials through side channel analysis, and the targeting of proprietary training datasets.
-
•
Adversaries can employ 33 offensive AI capabilities against organizations. These are categorized into seven groups: (1) automation, (2) campaign resilience, (3) credential theft, (4) exploit development, (5) information gathering, (6) social engineering, and (7) stealth.
- •
From the User Study.
-
•
The top three most threatening categories of offensive AI capabilities against organizations are (1) exploit development, (2) social engineering, and (3) information gathering.
-
•
24 of the 33 offensive AI capabilities pose significant threats to organizations.
-
•
For the most part, industry and academia are not aligned on the top threats of offensive AI against organizations. Industry is most concerned with AI being used for reverse engineering, with a focus on the loss of intellectual property. Academics, on the other hand, are most concerned about AI being used to perform biometric spoofing (e.g., evading fingerprint and facial recognition).
-
•
Both industry and academia ranked the threat of using AI for impersonation (e.g., real-time deepfakes to perpetrate phishing and other social engineering attacks) as their second highest threat. Jointly, industry and academia feel that impersonation is the biggest threat of all.
-
•
Evasion of intrusion detection systems (e.g., with adversarial machine learning) is considered to be the least threatening capability of the 24 significant threats, likely due to the adversary’s inaccessibility to training data.
-
•
AI impacts the cyber kill chain the most during the initial attack steps. This is because the adversary has access to the environment for training and testing of their AI models.
-
•
Because of an AI’s ability to automate processes, adversaries may shift from having a few slow covert campaigns to having numerous fast-paced campaigns to overwhelm defenders and increase their chances of success.
1.4. Contributions
In this survey, we make the following contributions:
-
•
An overview of how AI can be used to attack organizations and its influence on the cyber kill chain (section 3).
-
•
An enumeration and description of the 33 offensive AI capabilities which threaten organizations, based on literature and current events (section 4).
-
•
A threat ranking and insights on how offensive AI impacts organizations, based on a user study with members from academia, industry, and government (section 5).
-
•
A forecast of the AI threat horizon and the resulting shifts in attack strategies (section 6).
2. Background on Offensive AI
AI is intelligence demonstrated by a machine. It is often associated as a tool for automating some task which requires some level of intelligence. Early AI models were rule based systems designed using an expert’s knowledge (Yager, 1984), followed by search algorithms for selecting optimal decisions (e.g., finding paths or playing games (Zeng and Church, 2009)). Today, the most popular type of AI is machine learning (ML) where the machine can gain its intelligence by learning from examples. Deep learning (DL) is a type of ML where an extensive artificial neural network is used as the predictive model. Breakthroughs in DL have led to its ubiquity in applications such as automation, forecasting, and planning due to its ability to reason upon and generate complex data.
2.1. Training and Execution
In general, a machine learning model can be trained on data with an explicit ground-truth (supervised), with no ground-truth (unsupervised), or with a mix of both (semi-supervised). The trade-off between supervised and non-supervised approaches is that supervised methods often have much better performance at a given task, but require labeled data which can be expensive or impractical to collect. Moreover, unsupervised techniques are open-world, meaning that they can identify novel patterns that may have been overlooked. Another training method is reinforcement learning where a model is trained based on reward for good performance. Lastly, for generating content, a popular framework is adversarial learning. This was first popularised in (Goodfellow et al., 2014) where the generative adversarial network (GAN) was proposed. A GAN uses a discriminator model to ‘help’ a generator model produce realistic content by giving feedback on how the content fits a target distribution.
Where a model is trained or executed depends on the attacker’s task and strategy. For example, the training and execution of models for reconnaissance tasks will likely take place offsite from the organization. However, the training and execution of models for attacks may take place onsite, offsite, or both. Another possibility is where the adversary uses few-shot learning (Wang et al., 2020) by training on general data offsite and then fine tuning on the target data onsite. In all cases, the adversary will first design and evaluate their model offsite prior to its usage on the organization to ensure its success and to avoid detection.
For onsite execution, an attacker runs the risk of detection if the model is complex (e.g. a DL model). For example when the model is transferred over to the organization’s network or when the attacker’s model begins to utilize resources, it may trigger the organization’s anomaly detection system. To mitigate this issue, the adversary must consider a trade-off between stealth and effectiveness. For example the adversary may (1) execute the model during off hours or on non-essential devices, (2) leverage an insider to transfer the model, or (3) transfer the observations off-site for execution.
Training | Execution | |||
Offsite | Onsite | Offsite | Onsite | Example |
Vulnerability detection | ||||
Side channel keylogging | ||||
Channel compression for exfiltration | ||||
Traffic shaping for evasion | ||||
Few-shot learning for record tampering |
There are two forms of offensive AI: Attacks using AI and attacks against AI. For example, an adversary can (1) use AI to improve the efficiency of an attack (e.g., information gathering, attack automation, and vulnerability discovery) or (2) use knowledge of AI to exploit the defender’s AI products and solutions (e.g., to evade a defense or to plant a trojan in a product). The latter form of offensive AI is commonly referred to as adversarial machine learning.
2.2. Attacks Using AI
Although there are a wide variety of AI tasks which can be used in attacks, we found the following to be the most common:
- Prediction:
-
This is the task of making a prediction based on previously observed data. Common examples are classification, anomaly detection, and regression. Examples of prediction for an offensive purpose includes the identification of keystrokes on a smartphone based on motion (Hussain et al., 2016; Javed et al., 2020; Marquardt et al., 2011), the selection of the weakest link in the chain to attack (Abid et al., 2018), and the localization of software vulnerabilities for exploitation (Lin et al., 2020; Jiang et al., 2019; Mokhov et al., 2014).
- Generation:
-
This is the task of creating content that fits a target distribution which, in some cases, requires realism in the eyes of a human. Examples of generation for offensive uses include the tampering of media evidence (Mirsky et al., 2019; Schreyer et al., 2019), intelligent password guessing (Hitaj et al., 2019; Garg and Ahuja, 2019), and traffic shaping to avoid detection (Novo and Morla, 2020; Han et al., 2020). Deepfakes are another instance of offensive AI in this category. A deepfake is a believable media created by a DL model. The technology can be used to impersonate a victim by puppeting their voice or face to perpetrate a phishing attack (Mirsky and Lee, 2021).
- Analysis:
-
This is the task of mining or extracting useful insights from data or a model. Some examples of analysis for offense are the use of explainable AI techniques (Ribeiro et al., 2016) to identify how to better hide artifacts (e.g., in malware) and the clustering or embedding of information on an organization to identify assets or targets for social engineering.
- Retrieval:
-
This is the task of finding content that matches or that is semantically similar to to a given query. For example, in offense, retrieval algorithms can be used to track an object or an individual in a compromised surveillance system (Rahman et al., 2019; Zhu et al., 2018), to find a disgruntled employee (as a potential insider) using semantic analysis on social media posts, and to summarize lengthy documents (Zhang et al., 2016) during open source intelligence (OSINT) gathering in the reconnaissance phase.
- Decision Making:
2.3. Attacks Against AI - Adversarial Machine Learning
An attacker can use its AI knowledge to exploit ML model vulnerabilities violating its confidentiality, integrity, or availability. Attacks can be staged at either training (development) or test time (deployment) through one of the following attack vectors:
- Modify the Training Data.:
-
Here the attacker modifies the training data to harm the integrity or availability of the model. Denial of service (DoS) poisoning attacks (Biggio et al., 2012; Muñoz-González et al., 2017; Koh and Liang, 2017) are when the attacker decreases the model’s performance until it is unusable. A backdoor poisoning attack (Gu et al., 2017; Chen et al., 2017) or trojaning attack (Liu et al., 2017), is where the attacker teaches the model to recognize an unusual pattern that triggers a behavior (e.g., classify a sample as safe). A triggerless version of this attack causes the model to misclassify a test sample without adding a trigger pattern to the sample itself (Shafahi et al., 2018; Aghakhani et al., 2020)
- Modify the Test Data.:
-
In this case, the attacker modifies test samples to have them misclassified (Biggio et al., 2013; Szegedy et al., 2014; Goodfellow et al., 2015). For example, altering the letters of a malicious email to have it misclassified as legitimate, or changing a few pixels in an image to evade facial recognition (Sharif et al., 2016). Therefore, these types of attacks are often referred to as evasion attacks. By modifying test samples ad-hoc to increase the model’s resource consumption, the attacker can also slow down the model performances. (Shumailov et al., 2020).
- Analyze the Model’s Responses.:
-
Here, the attacker sends a number of crafted queries to the model and observes the responses to infer information about the model’s parameters or training data. To learn about the training data, there are membership inference (Shokri et al., 2017), deanonymization (Narayanan and Shmatikov, 2008), and model inversion (Hidano et al., 2017) attacks. For learning about the model’s parameters there are model stealing/extraction (Juuti et al., 2019; Jia et al., 2021), and blind-spot detection (Zhang et al., 2019), state prediction (Woh and Lee, 2018).
- Modify the Training Code.:
-
This is where the attacker performs a supply chain attack by modifying a library used to train ML models (e.g., via an open source project). For example, a compromised loss (training) function that inserts a backdoor (Bagdasaryan and Shmatikov, 2020).
- Modify the Model’s Parameters.:
-
In this attack vector, the attacker accesses a trained model (e.g., via a model zoo or security breach) and tamper its parameters to insert a latent behavior. These attacks can be performed at the software (Yao et al., 2019; Wang et al., 2020, 2020) or hardware (Breier et al., 2018b) levels (a.k.a. fault attacks).
Depending on the scenario, an attacker may not have full knowledge or access to the target model:
-
•
White-Box (Perfect-Knowledge) Attacks: The attacker knows everything about the target system. This is the worst case for the system defender. Although it is not very likely to happen in practice, this setting is interesting as it provides an empirical upper bound on the attacker’s performance.
-
•
Gray-Box (Limited-Knowledge) Attacks: The attacker has partial knowledge of the target system (e.g., the learning algorithm, architecture, etc.) but no knowledge of training data or the model’s parameters.
-
•
Black-Box (Zero-Knowledge) Attacks: The attacker knows only the task the model is designed to perform and which kind of features are used by the system in general (e.g., if a malware detector has been trained to perform static or dynamic analysis). The attacker may also be able to analyse the model’s responses in a black-box manner to get feedback on certain inputs.
In a black or gray box scenario, the attacker can build a surrogate ML model and try to devise the attacks against it as the attacks often transfer between different models. (Biggio et al., 2013; Demontis et al., 2019a).
3. Offensive AI vs Organizations
In this section, we provide an overview of offensive AI in the context of organizations. First we review a popular attack model for enterprise. Then we will identify how an AI-capable adversary impacts this model by discussing the adversary’s new motivations, goals, capabilities, and requirements. Later in section 4, we will detail the adversary’s techniques based on our literature review.
3.1. The Attack Model
There are a variety of threat agents which target organizations. These agents are cyber terrorists, cyber criminals, employees, hacktivists, nation states, online social hackers, script kiddies, and other organizations like competitors. There are also some non-target specific agents, such as certain botnets and worms, which threaten the security of an organization. A threat agent may be motivated for various reasons. For example, to (1) make money through theft or ransom, (2) gain information through espionage, (3) cause physical or psychological damage for sabotage, terrorism, fame, or revenge, (4) reach another organization, and (5) obtain foothold on the organization as an asset for later use (Krebs, 2014). These agents not only pose a threat to the organization, but also its employees, customers, and the general public as well (e.g., attacks on critical infrastructure).
In an attack, there may be number of attack steps which the threat agent must accomplish. These steps depend on the adversary’s goal and strategy. For example, in an advanced persistent threat (APT) (Messaoud et al., 2016; Chen et al., 2018b; Alshamrani et al., 2019), the adversary may need to reach an asset deep within the defender’s network. This would require multiple steps involving reconnaissance, intrusion, lateral movement through the network, and so on. However, some attacks can involve just a single step. For example, a spear phishing attack in which the victim unwittingly provides confidential information or even transfers money. In this paper, we describe the adversary’s attack steps using the MITRE ATT&CK Matrix for Enterprise333https://attack.mitre.org/ which captures common adversarial tactics based on real-world observations.
Attacks which involve multiple steps can be thwarted if the defender identifies or blocks the attack early on. The more progress which an adversary makes, the harder it is for the defender to mitigate it. For example, it is better to stop a campaign during the initial intrusion phase than during the lateral movement phase where an unknown number of devices in the network have been compromised. This concept is referred to as the cyber kill chain. From an offensive perspective, the adversary will want shorten and obscure the kill chain by being as to be as efficient and covert as possible. In particular, operation within a defender’s network usually requires the attacker to operate through a remote connection or send commands to compromised devices (bots) from a command and control (C2). This generates presence in the defenders network which can be detected over time.
3.2. The Impact of Offensive AI
Conventional adversaries use manual effort, common tools, and expert knowledge to reach their goals. In contrast, an AI-capable adversary can use AI to automate its tasks, enhance its tools, and evade detection. These new abilities affect the cyber kill chain.
First, let’s discuss why an adversary would consider using AI in its offensive on an organization.
3.2.1. The Three Motivators of Offensive AI
In our survey, we found that there are three core motivations for an adversary to use AI in an offensive against an organization: coverage, speed, and success.
- Coverage.:
-
By using AI, an adversary can scale up its operations through automation to decrease human labor and increase the chances of success. For example, AI can be used to automatically craft and launch spear phishing attacks, distil and reason upon data collected from OSINT, maintain attacks on multiple organizations in parallel, and reach more assets within a network to gain a stronger foothold. In other words, AI enables adversaries to target more organizations with higher precision attacks with a smaller workforce.
- Speed.:
-
With AI, an adversary can reach its goals faster. For example, machine learning can be used to help extract credentials, intelligently select the next best target during lateral movement, spy on users to obtain information (e.g., perform speech to text on eavesdropped audio), or find zero-days in software. By reaching a goal faster, the adversary not only saves time for other ventures but can also minimize its presence (duration) within the defender’s network.
- Success.:
-
By enhancing its operations with AI, an adversary increases its likelihood of success. Namely, ML can be used to (1) make the operation more covert by minimizing or camouflaging network traffic (such as C2 traffic) and by exploiting weaknesses in the defender’s AI models such as an ML-based intrusion detection system (IDS), (2) identify opportunities such as good targets for social engineering attacks and novel vulnerabilities, (3) enable better attack vectors such as using deepfakes in spear phishing attacks, (4) plan optimal attack strategies, and (5) strengthen persistence in the network through automated bot coordination and malware obfuscation.
We note that these motivations are not mutually exclusive. For example, the use of AI to automate a phishing campaign increases coverage, speed, and success.
3.2.2. AI-Capable Threat Agents
It is clear that some AI-capable threat agents will be able to perform more sophisticated AI attacks than others. For example, state actors can potentially launch intelligent automated botnets where hacktivists will likely struggle in accomplishing the same. However, we have observed over the years that AI has become increasingly accessible, even to novice users. For example, there are a wide variety of open source deepfakes technologies online which are plug and play444https://github.com/datamllab/awesome-deepfakes-materials. Therefore, the sophistication gap between certain threat agents may close over time as the availability to AI technology increases.
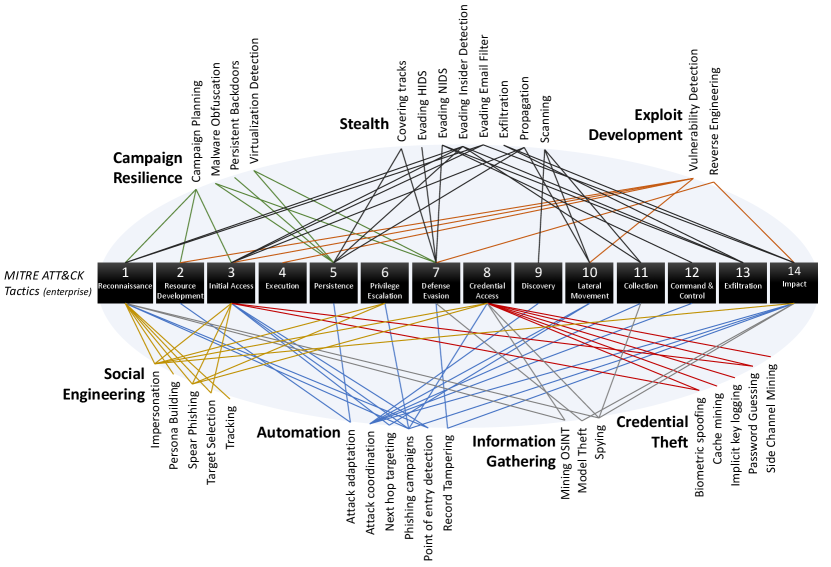
3.2.3. New Attack Goals
In addition to the conventional attack goals, AI-capable adversaries have new attack goals as well:
- Sabotage.:
-
The adversary may want to use its knowledge of AI to cause damage to the organization. For example, it may want to alter ML models in the organization’s products and solutions by poisoning their dataset to alter performance or by planting a trojan in the model for later exploitation. Moreover, the adversary may want to perform an adversarial machine learning attack on an AI system. For example, to evade detection in surveillance (Sharif et al., 2016) or to tip financial or energy forecasts models in the adversary’s favor. Finally, the adversary may also use generative AI to add or modify evidence in a realistic manner. For example, to modify or plant evidence in surveillance footage (Leetaru, 2019), medical scans (Mirsky et al., 2019), or financial records (Schreyer et al., 2019).
- Espionage.:
-
With AI, an adversary can improve its ability to spy on organizations and extract/infer meaningful information. For example, they can use speech to text algorithms and sentiment analysis to mine useful audio recordings (Abd El-Jawad et al., 2018) or steal credentials through acoustic or motion side channels (Liu et al., 2015a; Shumailov et al., 2019). AI can also be used to extract latent information from encrypted web traffic (Monaco, 2019), and track users through the organization’s social media (Malhotra et al., 2012). Finally, the attacker may want to achieve an autonomous persistent foothold using swarm intelligence (Zelinka et al., 2018).
- Information Theft.:
-
An AI-capable adversary may want to steal models trained by the organization to use in future white box adversarial machine learning attacks. Therefore, some data records and proprietary datasets may be targeted for the sake of training models. In particular, audio or video records of customers and employees may be stolen to create convincing deepfake impersonations. Finally, intellectual property may be targeted through AI powered reverse engineering tools (Hajipour et al., 2020).
3.2.4. New Attack Capabilities
Through our survey, we have identified 33 offensive AI capabilities (OAC) which directly improve the adversary’s ability to achieve attack steps. These OACs can be grouped into seven OAC categories: (1) automation, (2) campaign resilience, (3) credential theft, (4) exploit development, (5) information gathering, (6) social engineering, and (7) stealth. Each of these capabilities can be tied to the three motivators introduced in section 3.2.1.
In Fig. 1, we present the OACs and map their influence on the cyber kill chain (the MITRE enterprise ATT&CK model). An edge in the figure means that the indicated OAC improves attacker’s ability to achieve the given attack step. From the figure, we can see that offensive AI impacts every aspect of the attack model. Later in section 4 we will discuss each of these 33 OACs in greater detail.
These capabilities are materialized in one of two ways:
- AI-based tools:
-
are programs which performs a specific task in adversary’s arsenal. For example, a tool for intelligently predicting passwords (Hitaj et al., 2019; Garg and Ahuja, 2019), obfuscating malware code (Datta, 2020), traffic shaping for evasion (Li et al., 2019b; Novo and Morla, 2020; Han et al., 2020), puppeting a persona (Mirsky and Lee, 2021), and so on. These tools are typically in the form of a machine learning model.
- AI-driven bots:
-
are autonomous bots which can perform one or more attack steps without human intervention, or coordinate with other bots to efficiently reach their goal. These bots may use a combination of swarm intelligence (Castiglione et al., 2014) and machine learning to operate.
4. Survey of Offensive AI Capabilities
In section 3.2.4 we presented the 33 offensive AI capabilities. We will now describe each of the OACs in order of their 7 categories: automation, campaign resilience, credential theft, exploit development, information gathering, social engineering, and stealth.
4.1. Automation
The process of automation gives adversaries a hands-off approach to accomplishing attack steps. This not only reduces effort, but also increases the adversary’s flexibility and enables larger campaigns which are less dependent on C2 signals.
4.1.1. Attack Adaptation
Adversaries can use AI to help adapt their malware and attack efforts to unknown environments and find their intended targets. For example, identifying a system (cro, 2020) before attempting an exploit to increase the chances of success and avoid detection. In Black Hat’18, IBM researchers showed how a malware can trigger itself using DL by identifying a target’s machine by analysing the victim’s face, voice, and other attributes. With models such as decision trees, malware can locate and identify assets via complex rules like (Lunghi et al., 2017; Leong et al., 2019). Instead of transferring screenshots (Brumaghin et al., 2018; Arsene, 2020; Zhang, 2018; Mueller, 2018) DL can be used onsite to extract critical information.
4.1.2. Attack Coordination
Cooperative bots can use AI to find the best times and targets to attack. For example, swarm intelligence (Beni, 2020) is the study of autonomous coordination among bots in a decentralized manner. Researchers have proposed that botnets can use swarm intelligence as well. In (Zelinka et al., 2018) the authors discuss a hypothetical swarm malware and in (Truong et al., 2019) the authors propose another which uses DL to trigger attacks. AI bots can also communicate information on asset locations to fulfill attacks (e.g., send a stolen credential or relevant exploit to a compromised machine).
4.1.3. Next hop targeting
During lateral movement, the adversary must select the next asset to scan or attack. Choosing poorly may prolong the attack and risk detection by the defenders. For example, consider a browser like Firefox which has 4325 key-value pairs denoting the individual configurations. Only some inter-plays of these configurations are vulnerable (Otsuka et al., 2015; Chen et al., 2014). Reinforcement learning can be used to train a detection model which can identify the best browser to target. As for planning multiple steps, a strategy can be formed by using reinforcement learning on Petri nets (Bland et al., 2020) where attackers and defenders are modeled as competing players. Another approach is to use DL (Yousefi et al., 2018; Wu et al., 2021) to explore “attack graphs” (Ou et al., 2005) that contain the target’s network structure and the vulnerabilities. Notably, the Q-learning algorithms have enabled the approach to work on large-scale enterprise networks (Matta et al., 2019).
4.1.4. Phishing Campaigns
Phishing campaigns involve sending the same emails or robo-phone calls in mass. When someone falls prey and responds, the adversary takes over the conversation. These campaigns can be fully automated through AI like Google’s assistant which can make phone calls on your behalf (Leviathan and Matias, 2018; Singh and Thakur, 2020; Rebryk and Beliaev, 2020). Furthermore, adversaries can increase their success through mass spear phishing campaigns powered with deepfakes, where (1) a bot calls a colleague of the victim (found via social media), (2) clones his/her voice with 5 seconds of audio (Jia et al., 2018), and then (3) calls the victim in the colleague’s voice to exploit their trust.
4.1.5. Point of Entry Detection
The adversary can use AI to identify and select the best attack vector for an initial infection. For example, in (Leslie et al., 2019) statistical models on an organization’s attributes were used to predict the number of intrusions it receives. The adversary can train a model on similar information to select the weakest organizations (low hanging fruits) and the strongest attack vectors.
4.1.6. Record Tampering
An adversary may use AI to tamper records as part of their end-goal. For example, ML can be used to impact business decisions with synthetic data (Kumar et al., 2018), to obstruct justice by tampering evidence (Leetaru, 2019), to perform fraud (Schreyer et al., 2019) or to modify medical or satellite imagery (Mirsky et al., 2019). As shown in (Mirsky et al., 2019), DL-tampered records can fool human observers and can be accomplished autonomously onsite.
4.2. Campaign Resilience
In a campaign, adversaries try to ensure that their infrastructure and tools have a long life. Doing so helps maintain a foothold in the organization and enables reuse of tools and exploits for future and parallel campaigns. AI can be used to improve campaign resilience through planning, persistence, and obfuscation.
4.2.1. Campaign Planning
Some attacks require careful planning long before the attack campaign to ensure that all of the attacker’s tools and resources are obtainable. ML-based cost benefit analysis tools, such as in (Manning et al., 2018), may be used to identify which tools should be developed and how the attack infrastructure should be laid out (e.g., C2 servers, staging areas, etc). It could also be used to help identify other organizations that can be used as beach heads (Krebs, 2014). Moreover, ML can be used to plan a digital twin (Fuller et al., 2020; Bitton et al., 2018) of the victim’s network (based on information from reconnaissance) to be created offsite for tuning AI models and developing malware.
4.2.2. Malware Obfuscation
ML models such as GANs can be used to obscure a malware’s intent from an analyst. Doing so can enable reuse of the malware, hide the attacker’s intents and infrastructure, and prolong an attack campaign. The concept is to take an existing piece of software and emit another piece that is functionally equivalent (similar to translation in NLP). For example, DeepObfusCode (Datta, 2020) uses recurrent neural networks (RNN) to generate ciphered code. Alternatively, backdoors can be planted in open source projects and hidden using similar manners (Pasandi et al., 2019).
4.2.3. Persistent Access
An adversary can have bots establish multiple back doors per host and coordinate reinfection efforts among a swarm (Zelinka et al., 2018). Doing so achieves a foothold on an organization by slowing down the effort to purge the campaign. To avoid detection in payloads deployed during boot, the adversary can use a two-step payload which uses ML to identify when to deploy the malware and avoid detection (Anderson, 2017; Fang et al., 2019). Moreover, a USB sized neural compute stick555https://software.intel.com/content/www/us/en/develop/articles/intel-movidius-neural-compute-stick.html can be planted by an insider to enable covert and autonomous onsite DL operations.
4.2.4. Virtualization Detection
To avoid dynamic analysis and detection in sandboxes, an adversary may try to have the malware detect the sandbox before triggering. The malware could use ML to detect a virtual environment by measuring system timing (e.g., like in (Perianin et al., 2020)) and other system properties.
4.3. Credential Theft
Although a system may be secure in terms of access control, side channels can be exploited with ML to obtain a user’s credentials and vulnerabilities in AI systems can be used to avoid biometric security.
4.3.1. Biometric spoofing
Biometric security is used for access to terminals (such as smartphones) and for performing automated surveillance (Mozur, 2018; Wang et al., 2017; Ding et al., 2018). Recent works have shown how AI can generate “Master Prints” which are deepfakes of fingerprints that can open nearly any partial print scanner (such as on a smartphone) (Bontrager et al., 2018). Face recognition systems can be fooled or evaded with the use of adversarial samples. For example, in (Sharif et al., 2016) where the authors generated colorful glasses that alters the perceived identity. Moreover, ‘sponge’ samples (Shumailov et al., 2020) can be used to slow down a surveillance camera until it is unresponsive or out of batteries (when remote). Voice authentication can also be evaded through adversarial samples, spoofed voice (Wang et al., 2019c), and by cloning the target’s voice with deep learning (Wang et al., 2019c).
4.3.2. Cache mining
Information on credentials can be found in a system’s cache and log dumps, but the large amount of data makes finding it a difficult task. However, the authors of (Wang et al., 2019b) showed how ML can be used to identify credentials in cache dumps from graphic libraries. Another example is the work of (Calzavara et al., 2015) where an ML system was used to identify cookies containing session information.
4.3.3. Implicit key logging
Over the last few years researchers have shown how AI can be used as an implicit key-logger by sensing side channel information from a physical environment. The side channels comes in one or a combination of the following aspects:
- Motion.:
-
When tapping on a phone screen or typing on a keyboard, the device and nearby surfaces move and vibrate. A malware can use the smartphone’s motion sensors to decipher the touch strokes on the phone (Hussain et al., 2016; Javed et al., 2020) and keystrokes on nearby keyboards (Marquardt et al., 2011). Wearable devices can be exploited in a similar way as well (Liu et al., 2015b; Maiti et al., 2018).
- Audio.:
-
Researchers have shown that, when pressed, each key gives of it’s own unique sound which can be used to infer what is being typed (Liu et al., 2015a; Compagno et al., 2017). Timing between key strokes is also a revealing factor due to the structure of the language and keyboard layout. Similar approaches have also been shown for inferring touches on smartphones (Shumailov et al., 2019; Yu et al., 2019; Lu et al., 2019).
- Video.:
-
In some cases, a nearby smartphone or compromised surveillance camera can be used to observe keystrokes, even when the surface is obscured. For example, via eye movements (Chen et al., 2018a; Wang et al., 2018, 2019a), device motion (Sun et al., 2016), and hand motion (Balagani et al., 2018; Lim et al., 2020).
4.3.4. Password Guessing
Humans tend to select passwords with low entropy or with personal information such as dates. GANs can be used to intelligently brute-force passwords by learning from leaked password databases (Hitaj et al., 2019). Researchers have improved on this approach by using RNNs in the generation process (Nam et al., 2020). However, the authors of (Garg and Ahuja, 2019) found that models like (Hitaj et al., 2019) do not work well on Russian passwords. Instead, adversaries may pass the GAN personal information on the user to improve the performance (Seymour and Tully, 2018).
4.3.5. Side Channel Mining
ML algorithms are adept at extracting latent patterns in noisy data. Adversaries can leverage ML to extract secrets from side channels emitted from cryptographic algorithms. This has been accomplished on a variety of side channels including power consumption (Kocher et al., 1999; Lerman et al., 2014), electromagnetic emanations (Gandolfi et al., 2001), processing time (Brumley and Boneh, 2005), cache hits/misses(Perianin et al., 2020). In general, ML can be used to mine nearly any kind of side channel (Lerman et al., 2013; Weissbart et al., 2019; Picek et al., 2019; Cagli et al., 2017; Heuser et al., 2016; Picek et al., 2018; Maghrebi et al., 2016; Perin et al., 2020). For example, credentials can be extracted from the timing of network traffic (Song et al., 2001).
4.4. Exploit Development
Adversaries work hard to understand the content and inner-workings of compiled software to (1) steal intellectual property, (2) share trade secrets, (3) and identify vulnerabilities which they can exploit.
4.4.1. Reverse Engineering
While interpreting compiled code, an adversary can use ML to help identify functions and behaviors, and guide the reversal process. For example binary code similarity can be used to identify well-known or reused behaviors (Shin et al., 2015; Xu et al., 2017; Bao et al., 2014; Liu et al., 2018; Ding et al., 2019; Duan et al., 2020; Ye et al., 2020) and autoencoder networks can be used to segment and identify behaviors in code, similar to the work of (272, 2021). Furthermore, DL can potentially be used to lift compiled code up to a higher-level representation using graph transformation networks (Yun et al., 2019), similar to semantic analysis in language processing. Protocols and state machines can also be reversed using ML. For example, CAN bus data in a vehicles (Huybrechts et al., 2017), network protocols (Li et al., 2015), and commands (Bossert et al., 2014; Wang et al., 2011).
4.4.2. Vulnerability Detection
There are a wide variety of software vulnerability detection techniques which can be broken down into static and dynamic approaches:
- Static.:
-
For open source applications and libraries, the attacker can use ML tools for detecting known types of vulnerabilities in source code (Mokhov et al., 2014; Feng et al., 2016; Li et al., 2018; Li et al., 2019c; Chakraborty et al., 2020). If its a commercial product (compiled as a binary) then methods such as (272, 2021) can be used to identify vulnerabilities by comparing parts of the program’s control flow graph to known vulnerabilities.
- Dynamic.:
-
ML can also be used to perform guided input ‘fuzzing’ which can reach buggy code faster (She et al., 2019, 2020; Wang et al., 2020; Li et al., 2020a; Lin et al., 2020; Cheng et al., 2019; Atlidakis et al., 2020). Many works have also shown how AI can mitigate the issue of symbolic execution’s massive state space (Janota, 2018; Samulowitz and Memisevic, 2007; Liang et al., 2018; Kurin et al., 2019; Jiang et al., 2019).
4.5. Information Gathering
AI scales well and is very good at data mining and language processing. These capabilities can be used by an adversary to collect and distil actionable intel for a campaign.
4.5.1. Mining OSINT
In general, there are three ways in which AI can improve an adversary’s OSINT.
- Stealth.:
-
The adversary can use AI to camouflage its probe traffic to resemble benign services like Google’s web crawler (Cohen et al., 2020). Unlike heavy tools like Metagoofil (Martorella, 2020), ML can be used to minimize interactions by prioritizing sites and data elements (Ghazi et al., 2018; Guo et al., 2019).
- Gathering.:
-
Network structure and elements can be identified using cluster analysis or graph-based anomaly detection (Akoglu et al., 2015). Credentials and asset information can be found using methods like reinforcement learning on other organizations (Schwartz and Kurniawati, 2019). Finally, personnel structure can be extracted from social media using NLP-based web scrappers like Oxylabs(Oxylabs, 2021).
- Extraction.:
4.5.2. Model Theft
An adversary may want to steal an AI model to (1) obtain it as intellectual property, (2) extract information about members of its training set (Shokri et al., 2017; Narayanan and Shmatikov, 2008; Hidano et al., 2017), or (3) use it to perform a white-box attack against an organization. As described in section 2.3, if the model can be queried (e.g., model as a service -MAAS), then its parameters (Juuti et al., 2019; Jia et al., 2021) and hyperparameters (Wang and Gong, 2018) can be copied by observing the model’s responses. This can also be done through side-channel (Batina et al., 2019) or hardware-level analysis (Breier et al., 2020).
4.5.3. Spying
DL is extremely good at processing audio and video, and therefore can be used in spyware. For example, a compromised smartphone can map an office by (1) modeling each room with ultrasonic echo responses (Zhou et al., 2017), (2) using object recognition (Jiao et al., 2019) to obtain physical penetration info (control terminals, locks, guards, etc), and (3) automatically mine relevant information from overheard conversations (Ren et al., 2019; Nasar et al., 2019). ML can also be used to analyze encrypted traffic. For example it can extract transcripts from encrypted voice calls (White et al., 2011), identify applications (Al-Hababi and Tokgoz, 2020), and reveal internet searches (Monaco, 2019).
4.6. Social Engineering
The weakest links in an organization’s security are its humans. Adversaries have long targeted humans by exploiting their emotions and trust. AI provides adversaries will enhanced capabilities to exploit humans further.
4.6.1. Impersonation (Identity Theft)
An adversary may want to impersonate someone for a scam, blackmail attempt, a defamation attack, or to perform a spear phishing attack with their identity. This can be accomplished using deepfake technologies which enable the adversary to reenact (puppet) the voice and face of a victim, or alter existing media content of a victim (Mirsky and Lee, 2021). Recently, the technology has advanced to the state where reenactment can be performed in real-time (Nirkin et al., 2019), and training only requires a few images (Siarohin et al., 2019) or seconds of audio (Jia et al., 2018) from the victim. For high quality deepfakes, large amounts of audio/video data is still needed. However, when put under pressure, a victim may trust a deepfake even if it has a few abnormalities (e.g., in a phone call) (Workman, 2008). Moreover, the audio/video data may be an end-goal and inside the organization (e.g., customer data).
4.6.2. Persona Building
Adversaries build fake personas on online social networks (OSN) to connect with their targets. To evade fake profile detectors, a profile can be cloned and slightly altered using AI (Salminen et al., 2019; Salminen et al., 2020; Spiliotopoulos et al., 2020) so that they will appear different yet reflect the same personality. The adversary can then use a number of AI techniques to alter or mask the photos from detection (Shan et al., 2020; Sun et al., 2018; Li et al., 2019a; shaoanlu, 2020). To build connections, a link prediction model can be used to maximize the acceptance rate (Wang et al., 2019d; Kong and Tong, 2020) and a DL chatbot can be used to maintain the conversations (Roller et al., 2020).
4.6.3. Spear Phishing
Call-based spear phishing attacks can be enhanced using real-time deepfakes of someone the victim trusts. For example, this occured in 2019 when a CEO was scammed out $240k (Stupp, [n.d.]). For text-based phishing, tweets (zerofox, 2020) and emails (Seymour and Tully, 2016, 2018; Das and Verma, 2019) can be generated to attract a specific victim, or style transfer techniques can be used to mimic a colleague (Fu et al., 2017; Yang et al., 2018).
4.6.4. Target Selection
An adversary can use AI to identify victims in the organization who are the most susceptible to social engineering attacks (Abid et al., 2018). A regression model based on the target’s social attributes (conversations, attended events, etc) can be used as well. Moreover, sentiment analysis can be used to find disgruntled employees to be recruited as insiders (Panagiotou et al., 2019; Abd El-Jawad et al., 2018; Dhaoui et al., 2017; Ghiassi and Lee, 2018; Rathi et al., 2018).
4.6.5. Tracking
To study members of an organization, adversaries may track the member’s activities. With ML, an adversary can trace personnel across different social media sites by content (Malhotra et al., 2012) and through facial recognition (bla, [n.d.]). ML models can also be used on OSN content to track a member’s location (Pellet et al., 2019). Finally, ML can also be used to discover hidden business relationships (Zhang et al., 2012; Ma et al., 2009) from the news and from OSNs as well (Kumar and Rathore, 2016; Zhang and Chen, 2018).
4.7. Stealth
In multi step attacks, covert operations are necessary to ensure success. An adversary can either use or abuse AI to evade detection.
4.7.1. Covering tracks
To hide traces of the adversary’s presence, anomaly detection can be performed on the logs to remove abnormal entries (Cao et al., 2017; Debnath et al., 2018). CryptoNets (Gilad-Bachrach et al., 2016) can also be used to hide malware logs and onsite training data for later use. To avoid detection onsite, trojans can be planted in DL intrusion detection systems (IDS) in a supply chain attack at both the hardware (Breier et al., 2018b, a) and software (Liu et al., 2017; Li et al., 2020b) levels. DL hardware trojans can use adversarial machine learning to avoid being detected (Hasegawa et al., 2020).
4.7.2. Evading HIDS (Malware Detectors)
The struggle between security analysts and malware developers is a never-ending battle, with the malware quickly evolving and defeating detectors. In general, state-of-the-art detectors are vulnerable to evasion (Kolosnjaji et al., 2018; Demontis et al., 2019b; Maiorca et al., 2020). For example, adversary can evade an ML-based HIDS that performs dynamic analysis by splitting the malware’s code into small components executed by different processes (Ispoglou and Payer, 2016). They can also evade ML-based detectors that perform static analysis by adding bytes to the executable (Suciu et al., 2019) or code that does not affect the malware behavior (Demetrio et al., 2020; Pierazzi et al., 2020; Anderson et al., 2018; Zhiyang et al., 2019; Fang et al., 2019). Modifying the malware without breaking its malicious functionality is not easy. Attackers may use AI explanation tools like LIME (Ribeiro et al., 2016) to understand which parts of malware are being recognized by the detector and change them manually. Tools for evading ML-based detection can be found freely online 666 https://github.com/zangobot/secml_malware.
4.7.3. Evading NIDS (Network Intrusion Detection Systems)
There are several ways an adversary can use AI to avoid detection while entering, traversing, and communicating over an organization’s network. Regarding URL-based NIDSs, attackers can avoid phishing detectors by generating URLS that do not match known examples (Bahnsen et al., 2018). Bots trying to contact their C2 server can generate URLs that appear legitimate to humans (Peck et al., 2019), or that can evade malicious-URL detectors(Sidi et al., 2020). To evade traffic-based NIDSs, adversaries can shape their traffic (Novo and Morla, 2020; Han et al., 2020) or change their timing to hide it(Sharon et al., 2021).
4.7.4. Evading Insider Detectors
To avoid insider detection mechanisms, adversaries can mask their operations using ML. For example, given some user’s credentials, they can use information on the user’s role and the organization’s structure to ensure that operation performed looks legitimate (Sutro, 2020).
4.7.5. Evading Email Filter
Many email services use machine learning to detect malicious emails. However, adversaries can use adversarial machine learning to evade detection (Dalvi et al., 2004; Lowd and Meek, 2005a, b; Gao et al., 2018). Similarly, malicious documents attached to emails, containing malware, can evade detection as well (e.g., (Li et al., 2020c)). Finally, an adversary may send emails to be intentionally detected so that they will be added to the defender’s training set, as part of a poisoning attack (Biggio et al., 2011).
4.7.6. Exfiltration
Similar to evading NIDSs, adversaries must evade detection when trying to exfiltrate data outside of the network. This can be accomplished by shaping traffic to match the outbound traffic (Li et al., 2019b) or by encoding the traffic within a permissible channel like Facebook chat (Rigaki and Garcia, 2018). To hide the transfer better, an adversary could use DL to compress (Patel et al., 2019) and even encrypt (Abadi and Andersen, 2016) the data being exfiltrated. To minimize throughput, audio and video media can be summarized to textual descriptions onsite with ML before exfiltration. Finally, if the network is air gapped (isolated from the Internet) (Guri and Elovici, 2018) then DL techniques can be used to hide data within side channels such as noise in audio (Jiang et al., 2020).
4.7.7. Propagation & Scanning
For stealthy lateral movement, an adversary can configure their Petri nets or attack graphs (see section 4.1.3) to avoid assets and subnets with certain IDSs and favour networks with more noise to hide in. Moreover, AI can be used to scan hosts and networks covertly by modeling its search patterns and network traffic according to locally observed patterns (Li et al., 2019b).
5. User Study & Threat Ranking
In our literature review (section 4) we identified the potential offensive AI capabilities (OAC) which an adversary can use to attack an organization. However, some OACs may be impractical, where others may pose much larger threats. Therefore, we performed a user study to rank these threats and understand their impact on the cyber kill chain.
5.1. Survey Setup
We surveyed 22 experts in both subjects of AI and cybersecurity. Our participants were CISOs, researchers, ethics experts, company founders, research managers, and other relevant professions. Exactly half of the participants were from academia and the other half were from industry (companies and government agencies). For example, some of our participants were from MITRE, IBM Research, Microsoft, Airbus, Bosch (RBEI), Fujitsu Ltd., Hitachi Ltd., Huawei Technologies, Nord Security, Institute for Infocomm Research (I2R), Purdue University, Georgia Institute of Technology, Munich Research Center, University of Cagliari, and the Nanyang Technological University (NTU). The responses of the participants have been anonymized and reflect their own personal views and not the views of their employers.
The survey consisted of 204 questions which asked the participants to (1) rate different aspects of each OAC, (2) give their opinion on the utility of AI to the adversary in the cyber kill chain, and (3) give their opinion on the balance between the attacker and defender when both have AI. We used these responses to produce threat rankings and to gain insights on the threat of offensive AI to organizations.
Only 22 individuals participated in the survey because AI-cybersecurity experts are very busy and hard to reach. However, assuming there are 100k eligible respondents in the population, with a confidence level of 95% we calculate that we have a margin of error of about 20%. Moreover, since we have sampled a variety of major universities and companies, and since deviation in the responses is relatively small, we believe that the results capture a fair and meaningful view of the subject matter.
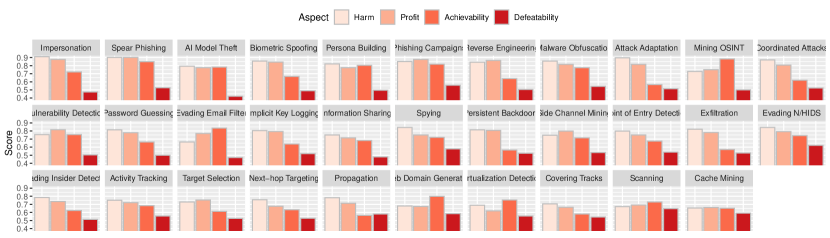
5.2. Threat Ranking
In this section we measure and rank the various threats of an adversary which can utilize or exploit AI technologies to enhance their attacks. For each OAC the participants were asked to rate four aspects on the range of 1-7 (low to high):
- Profit ()::
-
The amount of benefit which a threat agent gains by using AI compared to using non-AI methods. For example, attack success, flexibility, coverage, automation, and persistence. Here profit assumes that the AI tool has already been implemented.
- Achievability ()::
-
How easy is it for the attacker to use AI for this task considering that the adversary must implement, train, test and deploy the AI.
- Defeatability ()::
-
How easy is it for the defender to detect or prevent the AI-based attack. Here, a higher score is bad for the adversary (1=hard to defeat, 7=easy to defeat).
- Harm ()::
-
The amount of harm which an AI-capable adversary can inflict in terms of physical, physiological, or monetary damage (including effort put into mitigating the attack).
We say that an adversary is motivated to perform an attack if there is high profit and high achievability . Moreover, if there is high but low or vice versa, some actors may be tempted to try anyways. Therefore, we model the motivation of using an OAC as . However, just because there is motivation, it does not mean that there is a risk. If the AI attack can be easily detected or prevented, then no amount of motivation will make the OAC a risk. Therefore, we model risk as where a low defeatability (hard to prevent) increases and a high defeatability (easy to prevent) lowers . Risk can also be viewed as the likelihood of the attack occurring, or the likelihood of an attack success. Finally, to model threat, we must consider the amount of harm done to the organization. An OAC with high but no consequences is less of a threat. Therefore, we model our threat score as
(1) |
Before computing , we normalize , , , and from the range 1-7 to 0-1. This way, a threat score greater than 1 indicates a significant threat because for these scores (1) the adversary will attempt the attack (), and (2) the level of harm will be greater than the ability to prevent the attack (). We can also see from our model that as an adversary’s motivation increases over defeatability, the amount of harm deemed threatening decreases. This is intuitive because if an attack is easy to achieve and highly profitable, then it will be performed more often. Therefore, even if it is less harmful, attacks will occur frequently so the damage will be higher in the long run.
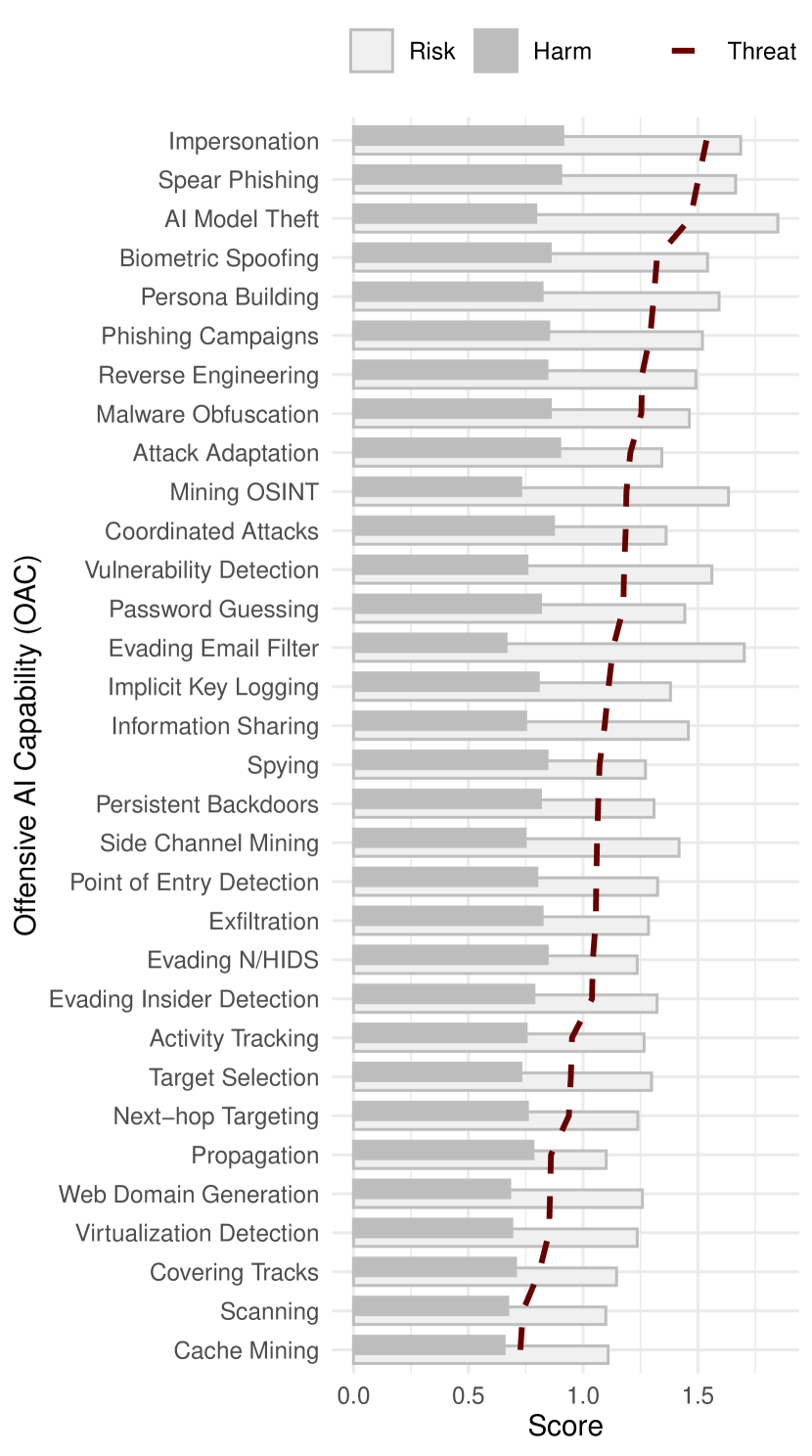
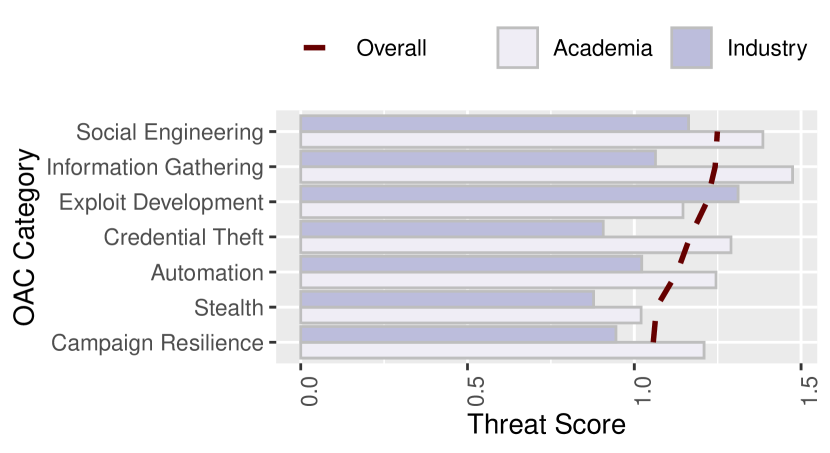
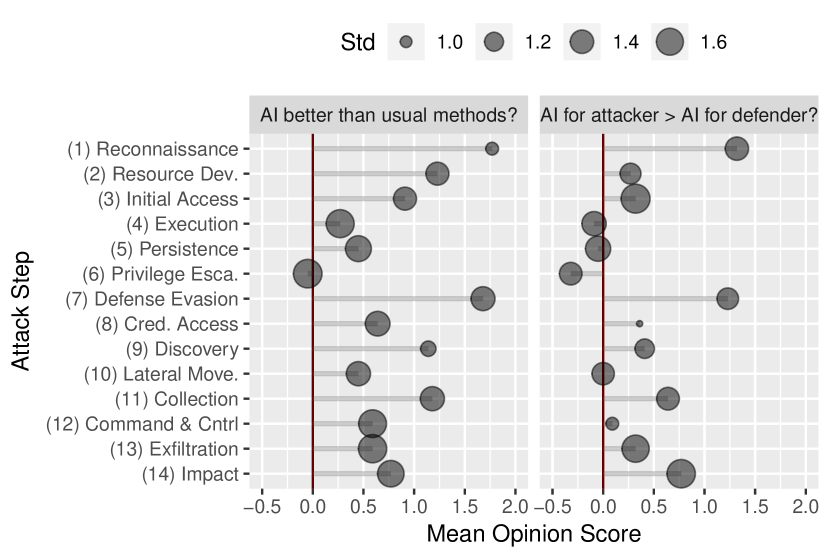
5.2.1. OAC Threat Ranking
In Fig. 2 we present the average , , , and scores for each OAC. In Fig. 5 we present the OACs ranked according to their threat score , and contrast their risk scores to their harm scores .
The results show that 23 of the OACs (72%) are considered to be significant threats (have a ). In general we observe that the top threats mostly relate to social engineering and malware development. The top three OACs are impersonation, spear phishing, and model theft. These OACs have significantly larger threat scores than the others because they are (1) easy to achieve, (2) have high payoffs, (3) are hard to prevent, and (4) cause the most harm (top left of Fig. 2). Interestingly, the use of AI to run phishing campaigns is considered a large threat even though it has a relatively high score. We believe this is because, with AI, an adversary can both increase the number and quality of the phishing attacks. Therefore, even if 99% of the attempts fail, some will get through and cause the organization damage. The least significant threats were scanning and cache mining which are perceived to have have little benefit for the adversary because they pose a high risk of detection. Other low ranked threats include some on-site automation for propagation, target selection, lateral movement, and covering tracks.
5.2.2. Industry vs Academia
In Fig. 5 we look at the average threat scores for each OAC category, and contrast the opinions of members from academia to those from industry.
In general, academia views AI as a more significant threat to organizations than industry. One can argue that the discrepancy is because industry tends to be more practical and grounded in the present, where academia considers potential threats thus considering the future. For example, when looking at the threat scores from academia, all of the categories are considered significant threats (). However, when looking at the industry’s responses, the categories of stealth, credential theft, and campaign resilience are not. This may be because these concepts have presented (proven) themselves less in the wild than the others.
Regardless, both industry and academia agree on the top three most threatening OAC categories: (1) exploit development, (2) social engineering, and (3) information gathering. This is because, for these categories, the attacker benefits greatly from using AI (), can easy implement the relevant AI tools (), the attack causes considerable damage (), and there is little the defender can do to prevent them () (indicated in Fig. 2). For example, deepfakes are easy to implement yet hard to detect in practice (e.g., in a phone call), and extracting private information from side channels and online resources can be accomplished with little intervention.
Surprisingly, both academia and industry consider the use of AI for stealth as the least threatening OAC category in general. Even though there has been a great deal of work showing how IDS models are vulnerable (Suciu et al., 2019; Novo and Morla, 2020), IDS evasion approaches were considered the second most defeatable OAC after intelligent scanning. This may have to do with the fact that the adversary cannot evaluate its AI-based evasion techniques inside the actual network, and thus risks detection.
Overall, there were some disagreements between industry and academia regarding the most threatening OACs. The top-10 most threatening OACs for organizations (out of 33) were ranked as follows:
Industry’s Perspective (1) Reverse Engineering (2) Impersonation (3) AI Model Theft (4) Spear Phishing (5) Persona Building (6) Phishing Campaigns (7) Information Sharing (8) Malware Obfuscation (9) Vulnerability Detection (10) Password Guessing Academia’s Perspective (1) Biometric Spoofing (2) Impersonation (3) Spear Phishing (4) AI Model Theft (5) Mining OSINT (6) Spying (7) Target Selection (8) Side Channel Mining (9) Coordinated Attacks (10) Attack Adaptation
We note that academia views biometric spoofing as the top threat, where industry doesn’t consider it in their top 10. We think this is because the latest research on this topic involves ML which can be evaded (e.g., (Sharif et al., 2016; Bontrager et al., 2018)). In contrast to academia, industry views this OAC as less harmful to the organization and less profitable to the adversary, perhaps because biometric security is not a common defense used in organization. Regardless, biometric spoofing is still considered the 4-th highest threat overall (Fig. 5). Another insight is that academia is more concerned about the use of ML for spyware, side-channels, target selection, and attack adaptation than industry. This may be because these are topics which have long been discussed in academia, but have yet to cause major disruptions in the real-world. For industry, they are more concerned with the use of AI for exploit development and social engineering, likely because these are threats which are out of their control.
Additional figures which compare the responses of industry to academia can be found online777https://tinyurl.com/t735m6st.
5.3. Impact on the Cyber Kill Chain
For each of the 14 MITRE ATT&CK steps, we asked the participants whether they agree or disagree888Measured using a 7-step likert scale ranging from strongly disagree (-3) to neutral (0) to strongly agree (+3). to the following statements: (1) It more beneficial for the attacker to use AI than conventional methods in this attack step, and (2) AI benefits the attacker more than AI benefits the defender. The objective of these questions were to identify how AI impacts the kill chain and whether AI forms any asymmetry between the attacker and defender.
In Fig. 5 we present the mean opinion scores along with their standard deviations (additional histograms can be found online7). Overall, our participants felt that AI enhances the adversary’s ability to traverse the kill chain. In particular, we observe that adversary benefits considerably from AI during the first three steps. One explanation is that these attacks are maintained offsite and thus are easier to develop and have less risk. Moreover, we understand from the results that there is a general feeling that defenders do not have a good way to preventing adversarial machine learning attacks. Therefore, AI not only improves defense evasion but also gives the attacker a considerable advantage over the defender in this regard.
Our participants also felt that an adversary with AI has a somewhat greater advantage over a defender with AI for most attack steps. In particular, the defender cannot effectively utilize AI to prevent reconnaissance except for mitigating a few kinds of social engineering attacks. Moreover, the adversary has many new uses for AI during the impact step, such as the tampering of records, where the defender does not. However, the participants felt that the defender has an advantage when using AI to detect execution, persistence, and privilege escalation. This is understandable since the defender can train and evaluate models onsite whereas the attacker cannot.
6. Discussion
In this section, we share our insights on our findings and discuss the road ahead.
6.1. Insights, Observations, & Limitations
Top Threats. It is understandable why the highest ranked threats to organizations relate to social engineering attacks and software analysis (vulnerability detection and reverse engineering). It is because these attacks are out of the defender’s control. For example, humans are the weakest link, even with security awareness training. However, with deepfakes, even less can be done to mitigate these social engineering attacks. The same holds for software analysis where ML has proven itself to work well with languages and even compiled binaries (Ye et al., 2020). As mentioned earlier, we believe the reason academia is the most concerned with biometrics is because it almost exclusively uses ML, and academia is well aware of ML’s flaws. On the other hand, industry members know that organizations do not often employ biometric security. Therefore, they perceive AI attacks on their software and personnel as the greatest threats.
The Near Future. Over the next few years, we believe that there will be an increase of offensive AI incidents, but only at the front and back of the attack model (recon., resource development, and impact –such as record tampering). This is because currently AI cannot effectively learn on its own. Therefore, we aren’t likely to see botnets that can autonomously and dynamically interact with a diverse set of complex systems (like an organization’s network) in the near future. Therefore, since modern adversaries have limited information on the organizations’ network, they are restricted to attacks where the data collection, model development, training, and evaluation occur offsite. In particular, we note that DL models are large and require a considerable amount of resources to run. This makes them easy to detect when transferred into the network or executed onsite. However, the model’s footprint will become less anomalous over time as DL proliferates. In the near future, we also expect that phishing campaigns will become more rampant and dangerous as humans and bots are given the ability to make convincing deepfake phishing calls.
AI is a Double Edged Sword. We observed that AI technologies for security can also be used in an offensive manner. Some technologies are dual purpose. For example, the ML research into disassembly, vulnerability detection, and penetration testing. Some technologies can be repurposed. For example, instead of using explainable AI to validate malware detection, it can be used to hide artifacts. And some technologies can be inverted. For example, an insider detection model can be used to help cover tracks and avoid detection. To help raise awareness, we recommend that researchers note the implications of their work, even for defensive technologies. One caveat is that the ‘sword’ is not symmetric depending on the wielder. For example, generative AI (deepfakes) is better for the attacker, but anomaly detection is better for the defender.
6.2. The Industry’s Perspective
Using logic to automate attacks is not new to industry – for instance, in 2015, security researchers from FireEye (Intelligence, 2015) found that advanced Russian cyber threat groups built a malware called HAMMERTOSS that used rules based automation to blend its traffic into normal traffic by checking for regular office hours in the time zone and then operating only in that time range. However, the scale and speed that offensive AI capabilities can endow attackers can be damaging.
According to 2019 Verizon Data Breach report analysis of 140 security breaches (dbi, 2019), the meantime to compromising an organization and exfiltrating the data ranges is already in the order of minutes. Organizations are already finding it difficult to combat automated offensive tactics and anticipate attacks to get stealthier in the future. For instance, according to the final report released by the US National Security Commission on AI in 2021 (rep, 2021a), the warning is clear “The U.S. government is not prepared to defend the United States in the coming artificial intelligence (AI) era.” The final report reasons that this is “Because of AI, adversaries will be able to act with micro-precision, but at macro-scale and with greater speed. They will use AI to enhance cyber attacks and digital disinformation campaigns and to target individuals in new ways.”
Most organizations see offensive AI as an imminent threat – 49% of 102 cybersecurity organizations surveyed by Forrester market research in 2020(rep, 2020), anticipate offensive AI techniques to manifest in the next 12 months. As a result, more organizations are turning to ways to defend against these attacks. A 2021 survey (rep, 2021b) of 309 organizations’ business leaders, C-Suite executives found that 96% of the organizations surveyed are already making investments to guard against AI-powered attacks as they anticipate more automation than what their defenses can handle.
6.3. What’s on the Horizon
With AI’s rapid pace of development and open accessibility, we expect to see a noticeable shift in attack strategies on organizations. First, we foresee that the number of deepfake phishing incidents will increase. This is because the technology (1) is mature, (2) is harder to mitigate than regular phishing, (3) is more effective at exploiting trust, (4) can expedite attacks, and (5) is new as phishing tactic so people are not expecting it. Second, we expect that AI will enable adversaries to target more organizations in parallel and more frequently. As a result, instead of being covert, adversaries may chose to overwhelm the defender’s response teams with thousands of attempts for the chance of one success. Finally, as adversaries begin to use AI-enabled bots, defenders will be forced to automate their defences with bots as well. Keeping humans in the loop to control and determine high level strategies is a practical and ethical requirement. However, further discussion and research is necessary to form safe and agreeable policies.
6.4. What can be done?
Attacks Using AI. Industry and academia should focus on developing solutions for mitigating the top threats. Personnel can be shown what to expect from AI-powered social engineering and further research can be done on detecting deepfakes, but in a manner which is robust to a dynamic adversary (Mirsky and Lee, 2021). Moreover, we recommend research into post-processing tools that can protect software from analysis after development (i.e., anti-vulnerability detection).
Attacks Against AI. The advantages and vulnerabilities of AI have profoundly questioned their widespread adoption, especially in mission-critical and cybersecurity-related tasks. In the meantime, organizations are working on automating the development and operations of ML models (MLOps), without focusing too much on ML security-related issues. To bridge this gap, we argue that extending the current MLOps paradigm to also encompass ML security (MLSecOps) may be a relevant way towards improving the security posture of such organizations. To this end, we envision the incorporation of security testing, protection and monitoring of AI/ML models into MLOps. Doing so will enable organizations to seamlessly deploy and maintain more secure and reliable AI/ML models.
7. Conclusion
In this survey we first explored, categorized, and identified the threats of offensive AI against organizations (sections 2 and 3). We then detailed the threats and ranked them through a user study with experts from the domain (sections 4 and 5). Finally, we provided insights into our results and gave directions for future work (section 6). We hope this survey will be meaningful and helpful to the community in addressing the imminent threat of offensive AI.
8. Acknowledgments
The authors would like to thank Laurynas Adomaitis, Sin G. Teo, Manojkumar Parmar, Charles Hart, Matilda Rhode, Dr. Daniele Sgandurra, Dr. Pin-Yu Chen, Evan Downing, and Didier Contis for taking the time to participate in our survey. We note that the views reflect the participant’s personal experiences and does not reflect the view of the participant’s employer. This material is based upon work supported by the Zuckerman STEM Leadership Program.
References
- (1)
- bla ([n.d.]) [n.d.]. Black Hat USA 2018. https://www.blackhat.com/us-18/arsenal.html#social-mapper-social-media-correlation-through-facial-recognition
- git ([n.d.]) [n.d.]. Telegram Contest. https://github.com/IlyaGusev/tgcontest. (Accessed on 10/14/2020).
- dbi (2019) 2019. 2019 Data Breach Investigations Report. Verizon, Inc (2019).
- rep (2020) 2020. The Emergence of Offensive AI. Forrester (2020).
- cro (2020) 2020. Our Work with the DNC: Setting the record straight. https://www.crowdstrike.com/blog/bears-midst-intrusion-democratic-national-committee/
- 272 (2021) 2021. DeepReflect: Discovering Malicious Functionality through Binary Reconstruction. In 30th USENIX Security Symposium (USENIX Security 21). USENIX Association. https://www.usenix.org/conference/usenixsecurity21/presentation/downing
- rep (2021a) 2021a. Final Report - National Security Commission on Artificial Intelligence. National Security Commission on Artificial Intelligence (2021).
- rep (2021b) 2021b. Preparing for AI-enabled cyberattacks. MIT Technology Review Insights (2021).
- Abadi and Andersen (2016) Martín Abadi and David G. Andersen. 2016. Learning to Protect Communications with Adversarial Neural Cryptography. arXiv (2016). https://arxiv.org/abs/1610.06918
- Abd El-Jawad et al. (2018) M. H. Abd El-Jawad, R. Hodhod, and Y. M. K. Omar. 2018. Sentiment Analysis of Social Media Networks Using Machine Learning. In 2018 14th International Computer Engineering Conference (ICENCO). 174–176. https://doi.org/10.1109/ICENCO.2018.8636124
- Abid et al. (2018) Y. Abid, Abdessamad Imine, and Michaël Rusinowitch. 2018. Sensitive Attribute Prediction for Social Networks Users. In EDBT/ICDT Workshops.
- Aghakhani et al. (2020) Hojjat Aghakhani, Dongyu Meng, Yu-Xiang Wang, Christopher Kruegel, and Giovanni Vigna. 2020. Bullseye Polytope: A Scalable Clean-Label Poisoning Attack with Improved Transferability. arXiv preprint arXiv:2005.00191 (2020).
- Akoglu et al. (2015) Leman Akoglu, Hanghang Tong, and Danai Koutra. 2015. Graph based anomaly detection and description: a survey. Data mining and knowledge discovery 29, 3 (2015), 626–688.
- Al-Hababi and Tokgoz (2020) Abdulrahman Al-Hababi and Sezer C Tokgoz. 2020. Man-in-the-Middle Attacks to Detect and Identify Services in Encrypted Network Flows using Machine Learning. In 2020 3rd International Conference on Advanced Communication Technologies and Networking (CommNet). IEEE, 1–5.
- Alshamrani et al. (2019) Adel Alshamrani, Sowmya Myneni, Ankur Chowdhary, and Dijiang Huang. 2019. A survey on advanced persistent threats: Techniques, solutions, challenges, and research opportunities. IEEE Communications Surveys & Tutorials 21, 2 (2019), 1851–1877.
- Anderson (2017) H. Anderson. 2017. Evading Machine Learning Malware Detection.
- Anderson et al. (2018) Hyrum S. Anderson, Anant Kharkar, Bobby Filar, David Evans, and Phil Roth. 2018. Learning to Evade Static PE Machine Learning Malware Models via Reinforcement Learning. arXiv:1801.08917 [cs.CR]
- Arsene (2020) L. Arsene. 2020. Oil & Gas Spearphishing Campaigns Drop Agent Tesla Spyware in Advance of Historic OPEC+ Deal. https://labs.bitdefender.com/2020/04/oil-gas-spearphishing-campaigns-drop-agent-tesla-spyware-in-advance-of-historic-opec-deal/
- Atlidakis et al. (2020) Vaggelis Atlidakis, Roxana Geambasu, Patrice Godefroid, Marina Polishchuk, and Baishakhi Ray. 2020. Pythia: Grammar-Based Fuzzing of REST APIs with Coverage-guided Feedback and Learning-based Mutations. arXiv preprint arXiv:2005.11498 (2020).
- Bagdasaryan and Shmatikov (2020) Eugene Bagdasaryan and Vitaly Shmatikov. 2020. Blind Backdoors in Deep Learning Models.
- Bahnsen et al. (2018) Alejandro Correa Bahnsen, Ivan Torroledo, Luis David Camacho, and Sergio Villegas. 2018. DeepPhish: Simulating Malicious AI. In 2018 APWG Symposium on Electronic Crime Research (eCrime). 1–8.
- Balagani et al. (2018) Kiran S Balagani, Mauro Conti, Paolo Gasti, Martin Georgiev, Tristan Gurtler, Daniele Lain, Charissa Miller, Kendall Molas, Nikita Samarin, Eugen Saraci, et al. 2018. Silk-tv: Secret information leakage from keystroke timing videos. In European Symposium on Research in Computer Security. Springer, 263–280.
- Bao et al. (2014) Tiffany Bao, Jonathan Burket, Maverick Woo, Rafael Turner, and David Brumley. 2014. BYTEWEIGHT: Learning to recognize functions in binary code. In 23rd USENIX Security Symposium (USENIX Security 14). 845–860.
- Barreno et al. (2010) Marco Barreno, Blaine Nelson, Anthony Joseph, and J. Tygar. 2010. The security of machine learning. Machine Learning 81 (2010), 121–148.
- Batina et al. (2019) Lejla Batina, Shivam Bhasin, Dirmanto Jap, and Stjepan Picek. 2019. CSI NN: Reverse Engineering of Neural Network Architectures Through Electromagnetic Side Channel. In 28th USENIX Security Symposium (USENIX Security 19). USENIX Association, Santa Clara, CA, 515–532. https://www.usenix.org/conference/usenixsecurity19/presentation/batina
- Beni (2020) Gerardo Beni. 2020. Swarm intelligence. Complex Social and Behavioral Systems: Game Theory and Agent-Based Models (2020), 791–818.
- Biggio et al. (2011) Battista Biggio, Igino Corona, Giorgio Fumera, Giorgio Giacinto, and Fabio Roli. 2011. Bagging Classifiers for Fighting Poisoning Attacks in Adversarial Classification Tasks. In 10th International Workshop on Multiple Classifier Systems (MCS) (Lecture Notes in Computer Science, Vol. 6713), Carlo Sansone, Josef Kittler, and Fabio Roli (Eds.). Springer-Verlag, 350–359.
- Biggio et al. (2013) B. Biggio, I. Corona, D. Maiorca, B. Nelson, N. Šrndić, P. Laskov, G. Giacinto, and F. Roli. 2013. Evasion attacks against machine learning at test time. In Machine Learning and Knowledge Discovery in Databases (ECML PKDD), Part III (LNCS, Vol. 8190), Hendrik Blockeel, Kristian Kersting, Siegfried Nijssen, and Filip Železný (Eds.). Springer Berlin Heidelberg, 387–402.
- Biggio et al. (2012) Battista Biggio, Blaine Nelson, and Pavel Laskov. 2012. Poisoning attacks against support vector machines, In 29th Int’l Conf. on Machine Learning, John Langford and Joelle Pineau (Eds.). Int’l Conf. on Machine Learning (ICML), 1807–1814.
- Biggio and Roli (2018) B. Biggio and F. Roli. 2018. Wild Patterns: Ten Years After the Rise of Adversarial Machine Learning. Pattern Recognition 84 (2018), 317–331.
- Bitton et al. (2018) Ron Bitton, Tomer Gluck, Orly Stan, Masaki Inokuchi, Yoshinobu Ohta, Yoshiyuki Yamada, Tomohiko Yagyu, Yuval Elovici, and Asaf Shabtai. 2018. Deriving a cost-effective digital twin of an ICS to facilitate security evaluation. In European Symposium on Research in Computer Security. Springer, 533–554.
- Bland et al. (2020) John A. Bland, Mikel D. Petty, Tymaine S. Whitaker, Katia P. Maxwell, and Walter Alan Cantrell. 2020. Machine Learning Cyberattack and Defense Strategies. Computers & Security 92 (2020), 101738. https://doi.org/10.1016/j.cose.2020.101738
- Bontrager et al. (2018) Philip Bontrager, Aditi Roy, Julian Togelius, Nasir Memon, and Arun Ross. 2018. Deepmasterprints: Generating masterprints for dictionary attacks via latent variable evolution. In 2018 IEEE 9th International Conference on Biometrics Theory, Applications and Systems (BTAS). IEEE, 1–9.
- Bossert et al. (2014) Georges Bossert, Frédéric Guihéry, and Guillaume Hiet. 2014. Towards automated protocol reverse engineering using semantic information. In Proceedings of the 9th ACM symposium on Information, computer and communications security. 51–62.
- Breier et al. (2018a) Jakub Breier, Xiaolu Hou, Dirmanto Jap, Lei Ma, Shivam Bhasin, and Yang Liu. 2018a. Deeplaser: Practical fault attack on deep neural networks. arXiv preprint arXiv:1806.05859 (2018).
- Breier et al. (2018b) Jakub Breier, Xiaolu Hou, Dirmanto Jap, Lei Ma, Shivam Bhasin, and Yang Liu. 2018b. Practical fault attack on deep neural networks. In Proceedings of the 2018 ACM SIGSAC Conference on Computer and Communications Security. 2204–2206.
- Breier et al. (2020) Jakub Breier, Dirmanto Jap, Xiaolu Hou, Shivam Bhasin, and Yang Liu. 2020. SNIFF: Reverse Engineering of Neural Networks with Fault Attacks. arXiv preprint arXiv:2002.11021 (2020).
- Brumaghin et al. (2018) Edmund Brumaghin, Holger Unterbrink, and Emmanuel Tacheau. 2018. Old dog, new tricks - Analysing new RTF-based campaign distributing Agent Tesla, Loki with PyREbox. https://blog.talosintelligence.com/2018/10/old-dog-new-tricks-analysing-new-rtf_15.html.
- Brumley and Boneh (2005) David Brumley and Dan Boneh. 2005. Remote timing attacks are practical. Computer Networks 48, 5 (2005), 701–716.
- Brundage et al. (2018) Miles Brundage, Shahar Avin, Jack Clark, Helen Toner, Peter Eckersley, Ben Garfinkel, Allan Dafoe, Paul Scharre, Thomas Zeitzoff, Bobby Filar, et al. 2018. The malicious use of artificial intelligence: Forecasting, prevention, and mitigation. arXiv preprint arXiv:1802.07228 (2018).
- Cagli et al. (2017) Eleonora Cagli, Cécile Dumas, and Emmanuel Prouff. 2017. Convolutional neural networks with data augmentation against jitter-based countermeasures. In International Conference on Cryptographic Hardware and Embedded Systems. Springer, 45–68.
- Caldwell et al. (2020) M Caldwell, JTA Andrews, T Tanay, and LD Griffin. 2020. AI-enabled future crime. Crime Science 9, 1 (2020), 1–13.
- Calzavara et al. (2015) Stefano Calzavara, Gabriele Tolomei, Andrea Casini, Michele Bugliesi, and Salvatore Orlando. 2015. A Supervised Learning Approach to Protect Client Authentication on the Web. ACM Trans. Web 9, 3, Article 15 (June 2015), 30 pages. https://doi.org/10.1145/2754933
- Cao et al. (2017) Q. Cao, Y. Qiao, and Z. Lyu. 2017. Machine learning to detect anomalies in web log analysis. In 2017 3rd IEEE International Conference on Computer and Communications (ICCC). 519–523.
- Castiglione et al. (2014) Aniello Castiglione, Roberto De Prisco, Alfredo De Santis, Ugo Fiore, and Francesco Palmieri. 2014. A botnet-based command and control approach relying on swarm intelligence. Journal of Network and Computer Applications 38 (2014), 22–33. https://doi.org/10.1016/j.jnca.2013.05.002
- Chakraborty et al. (2018) Anirban Chakraborty, Manaar Alam, Vishal Dey, Anupam Chattopadhyay, and Debdeep Mukhopadhyay. 2018. Adversarial Attacks and Defences: A Survey. arXiv:1810.00069 [cs, stat] ACM Computing Survey (Sept. 2018). http://arxiv.org/abs/1810.00069 arXiv: 1810.00069.
- Chakraborty et al. (2020) Saikat Chakraborty, Rahul Krishna, Yangruibo Ding, and Baishakhi Ray. 2020. Deep Learning based Vulnerability Detection: Are We There Yet? arXiv preprint arXiv:2009.07235 (2020).
- Chen et al. (2018b) Jiageng Chen, Chunhua Su, Kuo-Hui Yeh, and Moti Yung. 2018b. Special issue on advanced persistent threat.
- Chen et al. (2014) Wei Chen, Xiaoqiang Qiao, Jun Wei, Hua Zhong, and Xiang Huang. 2014. Detecting inter-component configuration errors in proactive: a relation-aware method. In 2014 14th International Conference on Quality Software. IEEE, 184–189.
- Chen et al. (2017) X. Chen, C. Liu, B. Li, K. Lu, and D. Song. 2017. Targeted Backdoor Attacks on Deep Learning Systems Using Data Poisoning. ArXiv e-prints abs/1712.05526 (2017).
- Chen et al. (2018a) Yimin Chen, Tao Li, Rui Zhang, Yanchao Zhang, and Terri Hedgpeth. 2018a. Eyetell: Video-assisted touchscreen keystroke inference from eye movements. In 2018 IEEE Symposium on Security and Privacy (SP). IEEE, 144–160.
- Cheng et al. (2019) Liang Cheng, Yang Zhang, Yi Zhang, Chen Wu, Zhangtan Li, Yu Fu, and Haisheng Li. 2019. Optimizing seed inputs in fuzzing with machine learning. In 2019 IEEE/ACM 41st International Conference on Software Engineering: Companion Proceedings (ICSE-Companion). IEEE, 244–245.
- Cohen et al. (2020) Dvir Cohen, Yisroel Mirsky, Manuel Kamp, Tobias Martin, Yuval Elovici, Rami Puzis, and Asaf Shabtai. 2020. DANTE: A framework for mining and monitoring darknet traffic. In European Symposium on Research in Computer Security. Springer, 88–109.
- Compagno et al. (2017) Alberto Compagno, Mauro Conti, Daniele Lain, and Gene Tsudik. 2017. Don’t Skype & Type! Acoustic Eavesdropping in Voice-Over-IP. In Proceedings of the 2017 ACM on Asia Conference on Computer and Communications Security. 703–715.
- Croce and Hein (2020) Francesco Croce and Matthias Hein. 2020. Reliable evaluation of adversarial robustness with an ensemble of diverse parameter-free attacks. In ICML.
- Dabre et al. (2020) Raj Dabre, Chenhui Chu, and Anoop Kunchukuttan. 2020. A survey of multilingual neural machine translation. ACM Computing Surveys (CSUR) 53, 5 (2020), 1–38.
- Dalvi et al. (2004) Nilesh Dalvi, Pedro Domingos, Mausam, Sumit Sanghai, and Deepak Verma. 2004. Adversarial classification. In Tenth ACM SIGKDD International Conference on Knowledge Discovery and Data Mining (KDD). Seattle, 99–108.
- Das and Verma (2019) Avisha Das and Rakesh Verma. 2019. Automated email Generation for Targeted Attacks using Natural Language. arXiv:1908.06893 [cs.CL]
- Datta (2020) Siddhartha Datta. 2020. DeepObfusCode: Source Code Obfuscation Through Sequence-to-Sequence Networks. arXiv:1909.01837 [cs.CR]
- Debnath et al. (2018) B. Debnath, M. Solaimani, M. A. G. Gulzar, N. Arora, C. Lumezanu, J. Xu, B. Zong, H. Zhang, G. Jiang, and L. Khan. 2018. LogLens: A Real-Time Log Analysis System. In 2018 IEEE 38th International Conference on Distributed Computing Systems (ICDCS). 1052–1062.
- Demetrio et al. (2020) Luca Demetrio, Battista Biggio, Giovanni Lagorio, Fabio Roli, and Alessandro Armando. 2020. Functionality-preserving Black-box Optimization of Adversarial Windows Malware. arXiv:2003.13526 [cs] (Sept. 2020). http://arxiv.org/abs/2003.13526 arXiv: 2003.13526.
- Demontis et al. (2019a) Ambra Demontis, Marco Melis, Maura Pintor, Matthew Jagielski, Battista Biggio, Alina Oprea, Cristina Nita-Rotaru, and Fabio Roli. 2019a. Why Do Adversarial Attacks Transfer? Explaining Transferability of Evasion and Poisoning Attacks. In 28th USENIX Security Symposium (USENIX Security 19). USENIX Association.
- Demontis et al. (2019b) Ambra Demontis, Marco Melis, Maura Pintor, Matthew Jagielski, Battista Biggio, Alina Oprea, Cristina Nita-Rotaru, and Fabio Roli. 2019b. Why Do Adversarial Attacks Transfer? Explaining Transferability of Evasion and Poisoning Attacks. In 28th USENIX Security Symposium (USENIX Security 19). USENIX Association.
- Dhaoui et al. (2017) Chedia Dhaoui, Cynthia M Webster, and Lay Peng Tan. 2017. Social media sentiment analysis: lexicon versus machine learning. Journal of Consumer Marketing (2017).
- Ding et al. (2018) Changxing Ding, Kaiqi Huang, Vishal M Patel, and Brian C Lovell. 2018. Special issue on Video Surveillance-oriented Biometrics. Pattern Recognition Letters 107 (2018), 1–2.
- Ding et al. (2019) Steven HH Ding, Benjamin CM Fung, and Philippe Charland. 2019. Asm2vec: Boosting static representation robustness for binary clone search against code obfuscation and compiler optimization. In 2019 IEEE Symposium on Security and Privacy (SP). IEEE, 472–489.
- Duan et al. (2020) Yue Duan, Xuezixiang Li, Jinghan Wang, and Heng Yin. 2020. DEEPBINDIFF: Learning Program-Wide Code Representations for Binary Diffing. In Proceedings of the 27th Annual Network and Distributed System Security Symposium (NDSS’20).
- Evangelista et al. (2020) João Rafael Gonçalves Evangelista, Renato José Sassi, Márcio Romero, and Domingos Napolitano. 2020. Systematic Literature Review to Investigate the Application of Open Source Intelligence (OSINT) with Artificial Intelligence. Journal of Applied Security Research (2020), 1–25.
- Fang et al. (2019) Z. Fang, J. Wang, B. Li, S. Wu, Y. Zhou, and H. Huang. 2019. Evading Anti-Malware Engines With Deep Reinforcement Learning. IEEE Access 7 (2019), 48867–48879. https://doi.org/10.1109/ACCESS.2019.2908033
- Feng et al. (2016) Qian Feng, Rundong Zhou, Chengcheng Xu, Yao Cheng, Brian Testa, and Heng Yin. 2016. Scalable graph-based bug search for firmware images. In Proceedings of the 2016 ACM SIGSAC Conference on Computer and Communications Security. 480–491.
- Fu et al. (2017) Zhenxin Fu, Xiaoye Tan, Nanyun Peng, Dongyan Zhao, and Rui Yan. 2017. Style transfer in text: Exploration and evaluation. arXiv preprint arXiv:1711.06861 (2017).
- Fuller et al. (2020) Aidan Fuller, Zhong Fan, Charles Day, and Chris Barlow. 2020. Digital twin: Enabling technologies, challenges and open research. IEEE Access 8 (2020), 108952–108971.
- Gandolfi et al. (2001) Karine Gandolfi, Christophe Mourtel, and Francis Olivier. 2001. Electromagnetic analysis: Concrete results. In International workshop on cryptographic hardware and embedded systems. Springer, 251–261.
- Gao et al. (2018) J. Gao, J. Lanchantin, M. L. Soffa, and Y. Qi. 2018. Black-Box Generation of Adversarial Text Sequences to Evade Deep Learning Classifiers. In 2018 IEEE Security and Privacy Workshops (SPW). 50–56. https://doi.org/10.1109/SPW.2018.00016
- Garg and Ahuja (2019) Vernit Garg and Laxmi Ahuja. 2019. Password Guessing Using Deep Learning. In 2019 2nd International Conference on Power Energy, Environment and Intelligent Control (PEEIC). IEEE, 38–40.
- Ghazi et al. (2018) Yumna Ghazi, Zahid Anwar, Rafia Mumtaz, Shahzad Saleem, and Ali Tahir. 2018. A supervised machine learning based approach for automatically extracting high-level threat intelligence from unstructured sources. In 2018 International Conference on Frontiers of Information Technology (FIT). IEEE, 129–134.
- Ghiassi and Lee (2018) Manoochehr Ghiassi and S Lee. 2018. A domain transferable lexicon set for Twitter sentiment analysis using a supervised machine learning approach. Expert Systems with Applications 106 (2018), 197–216.
- Gilad-Bachrach et al. (2016) Ran Gilad-Bachrach, Nathan Dowlin, Kim Laine, Kristin Lauter, Michael Naehrig, and John Wernsing. 2016. Cryptonets: Applying neural networks to encrypted data with high throughput and accuracy. In International Conference on Machine Learning. 201–210.
- Goodfellow et al. (2014) Ian J. Goodfellow, Jean Pouget-Abadie, Mehdi Mirza, Bing Xu, David Warde-Farley, Sherjil Ozair, Aaron Courville, and Yoshua Bengio. 2014. Generative Adversarial Nets. In Proceedings of the 27th International Conference on Neural Information Processing Systems - Volume 2 (Montreal, Canada) (NIPS’14). MIT Press, Cambridge, MA, USA, 2672–2680.
- Goodfellow et al. (2015) Ian J. Goodfellow, Jonathon Shlens, and Christian Szegedy. 2015. Explaining and Harnessing Adversarial Examples. In International Conference on Learning Representations.
- Gu et al. (2017) Tianyu Gu, Brendan Dolan-Gavitt, and Siddharth Garg. 2017. BadNets: Identifying Vulnerabilities in the Machine Learning Model Supply Chain. In NIPS Workshop on Machine Learning and Computer Security, Vol. abs/1708.06733.
- Guo et al. (2019) Jiafeng Guo, Yixing Fan, Liang Pang, Liu Yang, Qingyao Ai, Hamed Zamani, Chen Wu, W Bruce Croft, and Xueqi Cheng. 2019. A deep look into neural ranking models for information retrieval. Information Processing & Management (2019), 102067.
- Guri and Elovici (2018) Mordechai Guri and Yuval Elovici. 2018. Bridgeware: The air-gap malware. Commun. ACM 61, 4 (2018), 74–82.
- Hajipour et al. (2020) Hossein Hajipour, Mateusz Malinowski, and Mario Fritz. 2020. IReEn: Iterative Reverse-Engineering of Black-Box Functions via Neural Program Synthesis. arXiv:2006.10720 [cs.LG]
- Han et al. (2020) Dongqi Han, Zhiliang Wang, Ying Zhong, Wenqi Chen, Jiahai Yang, Shuqiang Lu, Xingang Shi, and Xia Yin. 2020. Practical traffic-space adversarial attacks on learning-based nidss. arXiv preprint arXiv:2005.07519 (2020).
- Hasegawa et al. (2020) Kento Hasegawa, Masao Yanagisawa, and Nozomu Togawa. 2020. Trojan-Net Classification for Gate-Level Hardware Design Utilizing Boundary Net Structures. IEICE TRANSACTIONS on Information and Systems 103, 7 (2020), 1618–1622.
- Heuser et al. (2016) Annelie Heuser, Stjepan Picek, Sylvain Guilley, and Nele Mentens. 2016. Side-channel analysis of lightweight ciphers: Does lightweight equal easy?. In International Workshop on Radio Frequency Identification: Security and Privacy Issues. Springer, 91–104.
- Hidano et al. (2017) S. Hidano, T. Murakami, S. Katsumata, S. Kiyomoto, and G. Hanaoka. 2017. Model Inversion Attacks for Prediction Systems: Without Knowledge of Non-Sensitive Attributes. In 2017 15th Annual Conference on Privacy, Security and Trust (PST). 115–11509. https://doi.org/10.1109/PST.2017.00023
- Hitaj et al. (2019) Briland Hitaj, Paolo Gasti, Giuseppe Ateniese, and Fernando Perez-Cruz. 2019. Passgan: A deep learning approach for password guessing. In International Conference on Applied Cryptography and Network Security. Springer, 217–237.
- Huang et al. (2011) L. Huang, A. D. Joseph, B. Nelson, B. Rubinstein, and J. D. Tygar. 2011. Adversarial Machine Learning. In 4th ACM Workshop on Artificial Intelligence and Security (AISec 2011). Chicago, IL, USA, 43–57.
- Hussain et al. (2016) Muzammil Hussain, Ahmed Al-Haiqi, AA Zaidan, BB Zaidan, ML Mat Kiah, Nor Badrul Anuar, and Mohamed Abdulnabi. 2016. The rise of keyloggers on smartphones: A survey and insight into motion-based tap inference attacks. Pervasive and Mobile Computing 25 (2016), 1–25.
- Huybrechts et al. (2017) Thomas Huybrechts, Yon Vanommeslaeghe, Dries Blontrock, Gregory Van Barel, and Peter Hellinckx. 2017. Automatic reverse engineering of CAN bus data using machine learning techniques. In International Conference on P2P, Parallel, Grid, Cloud and Internet Computing. Springer, 751–761.
- Ilin ([n.d.]) Ivan Ilin. [n.d.]. Building a news aggregator from scratch: news filtering, classification, grouping in threads and ranking. https://towardsdatascience.com/building-a-news-aggregator-from-scratch-news-filtering-classification-grouping-in-threads-and-7b0bbf619b68. (Accessed on 10/14/2020).
- Intelligence (2015) Fire Eye Threat Intelligence. 2015. HAMMERTOSS: stealthy tactics define a Russian cyber threat group. Milpitas, CA: FireEye, Inc (2015).
- Ispoglou and Payer (2016) Kyriakos K. Ispoglou and Mathias Payer. 2016. malWASH: Washing Malware to Evade Dynamic Analysis. In 10th USENIX Workshop on Offensive Technologies (WOOT 16). USENIX Association, Austin, TX. https://www.usenix.org/conference/woot16/workshop-program/presentation/ispoglou
- Janota (2018) Mikolás Janota. 2018. Towards Generalization in QBF Solving via Machine Learning.. In AAAI. 6607–6614.
- Javed et al. (2020) Abdul Rehman Javed, Mirza Omer Beg, Muhammad Asim, Thar Baker, and Ali Hilal Al-Bayatti. 2020. AlphaLogger: Detecting motion-based side-channel attack using smartphone keystrokes. Journal of Ambient Intelligence and Humanized Computing (2020), 1–14.
- Jia et al. (2021) Hengrui Jia, Christopher A. Choquette-Choo, Varun Chandrasekaran, and Nicolas Papernot. 2021. Entangled Watermarks as a Defense against Model Extraction. arXiv:2002.12200 [cs.CR]
- Jia et al. (2018) Ye Jia, Yu Zhang, Ron Weiss, Quan Wang, Jonathan Shen, Fei Ren, zhifeng Chen, Patrick Nguyen, Ruoming Pang, Ignacio Lopez Moreno, and Yonghui Wu. 2018. Transfer Learning from Speaker Verification to Multispeaker Text-To-Speech Synthesis. In Advances in Neural Information Processing Systems 31, S. Bengio, H. Wallach, H. Larochelle, K. Grauman, N. Cesa-Bianchi, and R. Garnett (Eds.). Curran Associates, Inc., 4480–4490. http://papers.nips.cc/paper/7700-transfer-learning-from-speaker-verification-to-multispeaker-text-to-speech-synthesis.pdf
- Jiang et al. (2019) Jian Jiang, Xiangzhan Yu, Yan Sun, and Haohua Zeng. 2019. A Survey of the Software Vulnerability Discovery Using Machine Learning Techniques. In International Conference on Artificial Intelligence and Security. Springer, 308–317.
- Jiang et al. (2020) Shunzhi Jiang, Dengpan Ye, Jiaqing Huang, Yueyun Shang, and Zhuoyuan Zheng. 2020. SmartSteganogaphy: Light-weight generative audio steganography model for smart embedding application. Journal of Network and Computer Applications 165 (2020), 102689.
- Jiao et al. (2019) Licheng Jiao, Fan Zhang, Fang Liu, Shuyuan Yang, Lingling Li, Zhixi Feng, and Rong Qu. 2019. A survey of deep learning-based object detection. IEEE Access 7 (2019), 128837–128868.
- Joseph et al. (2018) Anthony D. Joseph, Blaine Nelson, Benjamin I. P. Rubinstein, and J.D. Tygar. 2018. Adversarial Machine Learning. Cambridge University Press.
- Juuti et al. (2019) M. Juuti, S. Szyller, S. Marchal, and N. Asokan. 2019. PRADA: Protecting Against DNN Model Stealing Attacks. In 2019 IEEE European Symposium on Security and Privacy (EuroS P). 512–527. https://doi.org/10.1109/EuroSP.2019.00044
- Knake (2017) Robert K. Knake. 2017. A Cyberattack on the U.S. Power Grid. Technical Report. Council on Foreign Relations. http://www.jstor.org/stable/resrep05652
- Kocher et al. (1999) Paul Kocher, Joshua Jaffe, and Benjamin Jun. 1999. Differential power analysis. In Annual international cryptology conference. Springer, 388–397.
- Koh and Liang (2017) P. W. Koh and P. Liang. 2017. Understanding Black-box Predictions via Influence Functions. In International Conference on Machine Learning (ICML).
- Kolosnjaji et al. (2018) Bojan Kolosnjaji, Ambra Demontis, Battista Biggio, Davide Maiorca, Giorgio Giacinto, Claudia Eckert, and Fabio Roli. 2018. Adversarial Malware Binaries: Evading Deep Learning for Malware Detection in Executables. In 26th European Signal Processing Conf. (EUSIPCO). IEEE, Rome, 533–537.
- Kong and Tong (2020) Ru Kong and Xiangrong Tong. 2020. Dynamic Weighted Heuristic Trust Path Search Algorithm. IEEE Access 8 (2020), 157382–157390.
- Krebs (2014) Brian Krebs. 2014. Target Hackers Broke in Via HVAC Company – Krebs on Security. https://krebsonsecurity.com/2014/02/target-hackers-broke-in-via-hvac-company/. (Accessed on 04/15/2021).
- Kumar et al. (2018) Ashutosh Kumar, Arijit Biswas, and Subhajit Sanyal. 2018. eCommerceGAN : A Generative Adversarial Network for E-commerce. arXiv:1801.03244 [cs.LG]
- Kumar and Rathore (2016) Ashish Kumar and NC Rathore. 2016. Improving attribute inference attack using link prediction in online social networks. In Recent Advances in Mathematics, Statistics and Computer Science. World Scientific, 494–503.
- Kurin et al. (2019) Vitaly Kurin, Saad Godil, Shimon Whiteson, and Bryan Catanzaro. 2019. Improving SAT solver heuristics with graph networks and reinforcement learning. arXiv preprint arXiv:1909.11830 (2019).
- Leetaru (2019) Kalev Leetaru. 2019. Deep Fakes’ Greatest Threat Is Surveillance Video. https://www.forbes.com/sites/kalevleetaru/2019/08/26/deep-fakes-greatest-threat-is-surveillance-video/?sh=73c35a6c4550. (Accessed on 04/15/2021).
- Leong et al. (2019) R. Leong, D. Perez, and T. Dean. 2019. MESSAGETAP: Who’s Reading Your Text Messages? https://www.fireeye.com/blog/threat-research/2019/10/messagetap-who-is-reading-your-text-messages.html
- Lerman et al. (2014) Liran Lerman, Gianluca Bontempi, and Olivier Markowitch. 2014. Power analysis attack: an approach based on machine learning. International Journal of Applied Cryptography 3, 2 (2014), 97–115.
- Lerman et al. (2013) Liran Lerman, Gianluca Bontempi, Souhaib Ben Taieb, and Olivier Markowitch. 2013. A time series approach for profiling attack. In International Conference on Security, Privacy, and Applied Cryptography Engineering. Springer, 75–94.
- Leslie et al. (2019) Nandi O. Leslie, Richard E. Harang, Lawrence P. Knachel, and Alexander Kott. 2019. Statistical Models for the Number of Successful Cyber Intrusions. CoRR abs/1901.04531 (2019). arXiv:1901.04531 http://arxiv.org/abs/1901.04531
- Leviathan and Matias (2018) Yaniv Leviathan and Yossi Matias. 2018. Google Duplex: an AI system for accomplishing real-world tasks over the phone. (2018).
- Li et al. (2015) Haifeng Li, Bo Shuai, Jian Wang, and Chaojing Tang. 2015. Protocol reverse engineering using LDA and association analysis. In 2015 11th International Conference on Computational Intelligence and Security (CIS). IEEE, 312–316.
- Li et al. (2019b) J. Li, L. Zhou, H. Li, L. Yan, and H. Zhu. 2019b. Dynamic Traffic Feature Camouflaging via Generative Adversarial Networks. In 2019 IEEE Conference on Communications and Network Security (CNS). 268–276. https://doi.org/10.1109/CNS.2019.8802772
- Li et al. (2020b) Shaofeng Li, Shiqing Ma, Minhui Xue, and Benjamin Zi Hao Zhao. 2020b. Deep Learning Backdoors. arXiv preprint arXiv:2007.08273 (2020).
- Li et al. (2020a) Yuwei Li, Shouling Ji, Chenyang Lyu, Yuan Chen, Jianhai Chen, Qinchen Gu, Chunming Wu, and Raheem Beyah. 2020a. V-Fuzz: Vulnerability Prediction-Assisted Evolutionary Fuzzing for Binary Programs. IEEE Transactions on Cybernetics (2020).
- Li et al. (2020c) Yuanzhang Li, Yaxiao Wang, Ye Wang, Lishan Ke, and Yu-an Tan. 2020c. A feature-vector generative adversarial network for evading PDF malware classifiers. Information Sciences 523 (2020), 38–48.
- Li et al. (2019a) Yuezun Li, Xin Yang, Baoyuan Wu, and Siwei Lyu. 2019a. Hiding faces in plain sight: Disrupting ai face synthesis with adversarial perturbations. arXiv preprint arXiv:1906.09288 (2019).
- Li et al. (2019c) Zhen Li, Deqing Zou, Jing Tang, Zhihao Zhang, Mingqian Sun, and Hai Jin. 2019c. A comparative study of deep learning-based vulnerability detection system. IEEE Access 7 (2019), 103184–103197.
- Li et al. (2018) Zhen Li, Deqing Zou, Shouhuai Xu, Xinyu Ou, Hai Jin, Sujuan Wang, Zhijun Deng, and Yuyi Zhong. 2018. Vuldeepecker: A deep learning-based system for vulnerability detection. arXiv preprint arXiv:1801.01681 (2018).
- Liang et al. (2018) Jia Hui Liang, Chanseok Oh, Minu Mathew, Ciza Thomas, Chunxiao Li, and Vijay Ganesh. 2018. Machine learning-based restart policy for CDCL SAT solvers. In International Conference on Theory and Applications of Satisfiability Testing. Springer, 94–110.
- Lim et al. (2020) John Lim, True Price, Fabian Monrose, and Jan-Michael Frahm. 2020. Revisiting the Threat Space for Vision-based Keystroke Inference Attacks. arXiv preprint arXiv:2009.05796 (2020).
- Lin et al. (2020) Guanjun Lin, Sheng Wen, Qing-Long Han, Jun Zhang, and Yang Xiang. 2020. Software Vulnerability Detection Using Deep Neural Networks: A Survey. Proc. IEEE 108, 10 (2020), 1825–1848.
- Liu et al. (2018) Bingchang Liu, Wei Huo, Chao Zhang, Wenchao Li, Feng Li, Aihua Piao, and Wei Zou. 2018. diff: cross-version binary code similarity detection with dnn. In Proceedings of the 33rd ACM/IEEE International Conference on Automated Software Engineering. 667–678.
- Liu and Lang (2019) Hongyu Liu and Bo Lang. 2019. Machine learning and deep learning methods for intrusion detection systems: A survey. applied sciences 9, 20 (2019), 4396.
- Liu et al. (2015a) Jian Liu, Yan Wang, Gorkem Kar, Yingying Chen, Jie Yang, and Marco Gruteser. 2015a. Snooping keystrokes with mm-level audio ranging on a single phone. In Proceedings of the 21st Annual International Conference on Mobile Computing and Networking. 142–154.
- Liu et al. (2015b) Xiangyu Liu, Zhe Zhou, Wenrui Diao, Zhou Li, and Kehuan Zhang. 2015b. When good becomes evil: Keystroke inference with smartwatch. In Proceedings of the 22nd ACM SIGSAC Conference on Computer and Communications Security. 1273–1285.
- Liu et al. (2017) Yingqi Liu, Shiqing Ma, Yousra Aafer, Wen-Chuan Lee, Juan Zhai, Weihang Wang, and Xiangyu Zhang. 2017. Trojaning attack on neural networks. (2017).
- Lowd and Meek (2005a) Daniel Lowd and Christopher Meek. 2005a. Adversarial Learning. In Proc. 11th ACM SIGKDD International Conference on Knowledge Discovery and Data Mining (KDD). ACM Press, Chicago, IL, USA, 641–647.
- Lowd and Meek (2005b) Daniel Lowd and Christopher Meek. 2005b. Good word attacks on statistical spam filters. In Second Conference on Email and Anti-Spam (CEAS). Mountain View, CA, USA.
- Lu et al. (2019) Li Lu, Jiadi Yu, Yingying Chen, Yanmin Zhu, Xiangyu Xu, Guangtao Xue, and Minglu Li. 2019. KeyLiSterber: Inferring keystrokes on QWERTY keyboard of touch screen through acoustic signals. In IEEE INFOCOM 2019-IEEE Conference on Computer Communications. IEEE, 775–783.
- Lunghi et al. (2017) Daniel Lunghi, Jaromir Horejsi, and Cedric Pernet. 2017. Untangling the Patchwork Cyberespionage Group. https://documents.trendmicro.com/assets/tech-brief-untangling-the-patchwork-cyberespionage-group.pdf
- Ma et al. (2009) Zhongming Ma, Olivia Sheng, and Gautam Pant. 2009. Discovering company revenue relations from news: A network approach. Decision Support Systems 47 (11 2009), 408–414. https://doi.org/10.1016/j.dss.2009.04.007
- Maghrebi et al. (2016) Houssem Maghrebi, Thibault Portigliatti, and Emmanuel Prouff. 2016. Breaking cryptographic implementations using deep learning techniques. In International Conference on Security, Privacy, and Applied Cryptography Engineering. Springer, 3–26.
- Mahadi et al. (2018) Nurul Afnan Mahadi, Mohamad Afendee Mohamed, Amirul Ihsan Mohamad, Mokhairi Makhtar, Mohd Fadzil Abdul Kadir, and Mustafa Mamat. 2018. A survey of machine learning techniques for behavioral-based biometric user authentication. Recent Advances in Cryptography and Network Security (2018), 43–54.
- Maiorca et al. (2020) Davide Maiorca, Ambra Demontis, Battista Biggio, Fabio Roli, and Giorgio Giacinto. 2020. Adversarial Detection of Flash Malware: Limitations and Open Issues. Computers & Security 96 (2020), 101901. https://doi.org/10.1016/j.cose.2020.101901
- Maiti et al. (2018) Anindya Maiti, Murtuza Jadliwala, Jibo He, and Igor Bilogrevic. 2018. Side-channel inference attacks on mobile keypads using smartwatches. IEEE Transactions on Mobile Computing 17, 9 (2018), 2180–2194.
- Malhotra et al. (2012) Anshu Malhotra, Luam Totti, Wagner Meira Jr., Ponnurangam Kumaraguru, and Virgilio Almeida. 2012. Studying User Footprints in Different Online Social Networks. In Proceedings of the 2012 International Conference on Advances in Social Networks Analysis and Mining (ASONAM 2012) (ASONAM ’12). IEEE Computer Society, USA, 1065–1070. https://doi.org/10.1109/ASONAM.2012.184
- Manning et al. (2018) Matthew Manning, Gabriel TW Wong, Timothy Graham, Thilina Ranbaduge, Peter Christen, Kerry Taylor, Richard Wortley, Toni Makkai, and Pierre Skorich. 2018. Towards a ‘smart’cost–benefit tool: using machine learning to predict the costs of criminal justice policy interventions. Crime Science 7, 1 (2018), 12.
- Marquardt et al. (2011) Philip Marquardt, Arunabh Verma, Henry Carter, and Patrick Traynor. 2011. (sp) iphone: Decoding vibrations from nearby keyboards using mobile phone accelerometers. In Proceedings of the 18th ACM conference on Computer and communications security. 551–562.
- Martorella (2020) Christian Martorella. 2020. laramies/metagoofil: Metadata harvester. https://github.com/laramies/metagoofil. (Accessed on 10/20/2020).
- Matta et al. (2019) Marco Matta, Gian Carlo Cardarilli, Luca Di Nunzio, Rocco Fazzolari, Daniele Giardino, Marco re, Francesca Silvestri, and Sergio Spanò. 2019. Q-RTS: a Real-Time Swarm Intelligence based on Multi-Agent Q-Learning. Electronics Letters (03 2019). https://doi.org/10.1049/el.2019.0244
- Mattei (2017) Tobias A Mattei. 2017. Privacy, Confidentiality, and Security of Health Care Information: Lessons from the Recent WannaCry Cyberattack. World neurosurgery 104 (August 2017), 972—974. https://doi.org/10.1016/j.wneu.2017.06.104
- Melis et al. (2019) Marco Melis, Ambra Demontis, Maura Pintor, Angelo Sotgiu, and Battista Biggio. 2019. secml: A Python Library for Secure and Explainable Machine Learning. arXiv preprint arXiv:1912.10013 (2019).
- Messaoud et al. (2016) Brahim ID Messaoud, Karim Guennoun, Mohamed Wahbi, and Mohamed Sadik. 2016. Advanced persistent threat: new analysis driven by life cycle phases and their challenges. In 2016 International Conference on Advanced Communication Systems and Information Security (ACOSIS). IEEE, 1–6.
- Mirsky et al. (2018) Yisroel Mirsky, Tomer Doitshman, Yuval Elovici, and Asaf Shabtai. 2018. Kitsune: an ensemble of autoencoders for online network intrusion detection. arXiv preprint arXiv:1802.09089 (2018).
- Mirsky and Lee (2021) Yisroel Mirsky and Wenke Lee. 2021. The creation and detection of deepfakes: A survey. ACM Computing Surveys (CSUR) 54, 1 (2021), 1–41.
- Mirsky et al. (2019) Yisroel Mirsky, Tom Mahler, Ilan Shelef, and Yuval Elovici. 2019. CT-GAN: Malicious Tampering of 3D Medical Imagery using Deep Learning. In 28th USENIX Security Symposium (USENIX Security 19). USENIX Association, Santa Clara, CA, 461–478. https://www.usenix.org/conference/usenixsecurity19/presentation/mirsky
- Mokhov et al. (2014) Serguei A. Mokhov, Joey Paquet, and Mourad Debbabi. 2014. The Use of NLP Techniques in Static Code Analysis to Detect Weaknesses and Vulnerabilities. In Advances in Artificial Intelligence, Marina Sokolova and Peter van Beek (Eds.). Springer International Publishing, Cham, 326–332.
- Monaco (2019) John V. Monaco. 2019. What Are You Searching For? A Remote Keylogging Attack on Search Engine Autocomplete. In 28th USENIX Security Symposium (USENIX Security 19). USENIX Association, Santa Clara, CA, 959–976. https://www.usenix.org/conference/usenixsecurity19/presentation/monaco
- Mozur (2018) Paul Mozur. 2018. Looking Through the Eyes of China’s Surveillance State. https://www.nytimes.com/2018/07/16/technology/china-surveillance-state.html accessed: June 2018.
- Mueller (2018) R. Mueller. 2018. Indictment - United States of America vs. VIKTOR BORISOVICH NETYKSHO, et al. {https://www.justice.gov/file/1080281/download}
- Muñoz-González et al. (2017) Luis Muñoz-González, Battista Biggio, Ambra Demontis, Andrea Paudice, Vasin Wongrassamee, Emil C. Lupu, and Fabio Roli. 2017. Towards Poisoning of Deep Learning Algorithms with Back-gradient Optimization. In 10th ACM Workshop on Artificial Intelligence and Security (AISec ’17), Bhavani M. Thuraisingham, Battista Biggio, David Mandell Freeman, Brad Miller, and Arunesh Sinha (Eds.). ACM, New York, NY, USA, 27–38.
- Nam et al. (2020) Sungyup Nam, Seungho Jeon, Hongkyo Kim, and Jongsub Moon. 2020. Recurrent GANs Password Cracker For IoT Password Security Enhancement. Sensors 20, 11 (2020), 3106.
- Narayanan and Shmatikov (2008) A. Narayanan and V. Shmatikov. 2008. Robust De-anonymization of Large Sparse Datasets. In 2008 IEEE Symposium on Security and Privacy (sp 2008). 111–125. https://doi.org/10.1109/SP.2008.33
- Nasar et al. (2019) Zara Nasar, Syed Waqar Jaffry, and Muhammad Kamran Malik. 2019. Textual keyword extraction and summarization: State-of-the-art. Information Processing & Management 56, 6 (2019), 102088.
- Nicolae et al. (2018) Maria-Irina Nicolae, Mathieu Sinn, Minh Ngoc Tran, Beat Buesser, Ambrish Rawat, Martin Wistuba, Valentina Zantedeschi, Nathalie Baracaldo, Bryant Chen, Heiko Ludwig, Ian Molloy, and Ben Edwards. 2018. Adversarial Robustness Toolbox v1.2.0. CoRR 1807.01069 (2018). https://arxiv.org/pdf/1807.01069
- Nirkin et al. (2019) Yuval Nirkin, Yosi Keller, and Tal Hassner. 2019. Fsgan: Subject agnostic face swapping and reenactment. In Proceedings of the IEEE/CVF International Conference on Computer Vision. 7184–7193.
- Novo and Morla (2020) Carlos Novo and Ricardo Morla. 2020. Flow-based Detection and Proxy-based Evasion of Encrypted Malware C2 Traffic. In Proceedings of the 13th ACM Workshop on Artificial Intelligence and Security (AISec’20). Association for Computing Machinery, New York, NY, USA, 83–91. https://doi.org/10.1145/3411508.3421379
- Otsuka et al. (2015) Hiroshi Otsuka, Yukihiro Watanabe, and Yasuhide Matsumoto. 2015. Learning from before and after recovery to detect latent misconfiguration. In 2015 IEEE 39th Annual Computer Software and Applications Conference, Vol. 3. IEEE, 141–148.
- Ou et al. (2005) X. Ou, Sudhakar Govindavajhala, and Andrew W. Appel. 2005. MulVAL: A Logic-based Network Security Analyzer. In USENIX Security Symposium.
- Oxylabs (2021) Oxylabs. 2021. Innovative Proxy Service to Gather Data at Scale. https://oxylabs.io/. (Accessed on 04/14/2021).
- Panagiotou et al. (2019) Aimilia Panagiotou, Bogdan Ghita, Stavros Shiaeles, and Keltoum Bendiab. 2019. FaceWallGraph: Using Machine Learning for Profiling User Behaviour from Facebook Wall. In Internet of Things, Smart Spaces, and Next Generation Networks and Systems, Olga Galinina, Sergey Andreev, Sergey Balandin, and Yevgeni Koucheryavy (Eds.). Springer International Publishing, Cham, 125–134.
- Papernot et al. (2018) Nicolas Papernot, Fartash Faghri, Nicholas Carlini, Ian Goodfellow, Reuben Feinman, Alexey Kurakin, Cihang Xie, Yash Sharma, Tom Brown, Aurko Roy, Alexander Matyasko, Vahid Behzadan, Karen Hambardzumyan, Zhishuai Zhang, Yi-Lin Juang, Zhi Li, Ryan Sheatsley, Abhibhav Garg, Jonathan Uesato, Willi Gierke, Yinpeng Dong, David Berthelot, Paul Hendricks, Jonas Rauber, and Rujun Long. 2018. Technical Report on the CleverHans v2.1.0 Adversarial Examples Library. arXiv preprint arXiv:1610.00768 (2018).
- Papernot et al. (2018) N. Papernot, P. McDaniel, A. Sinha, and M. P. Wellman. 2018. SoK: Security and Privacy in Machine Learning. In 2018 IEEE European Symposium on Security and Privacy (EuroS P). 399–414. https://doi.org/10.1109/EuroSP.2018.00035
- Pasandi et al. (2019) Ghasem Pasandi, Shahin Nazarian, and Massoud Pedram. 2019. Approximate logic synthesis: A reinforcement learning-based technology mapping approach. In 20th International Symposium on Quality Electronic Design (ISQED). IEEE, 26–32.
- Patel et al. (2019) Manish I Patel, Sirali Suthar, and Jil Thakar. 2019. Survey on Image Compression using Machine Learning and Deep Learning. In 2019 International Conference on Intelligent Computing and Control Systems (ICCS). IEEE, 1103–1105.
- Peck et al. (2019) Jonathan Peck, Claire Nie, Raaghavi Sivaguru, Charles Grumer, Femi Olumofin, Bin Yu, Anderson Nascimento, and Martine De Cock. 2019. CharBot: A simple and effective method for evading DGA classifiers. IEEE Access 7 (2019), 91759–91771.
- Pellet et al. (2019) Hector Pellet, Stavros Shiaeles, and Stavros Stavrou. 2019. Localising social network users and profiling their movement. Computers & Security 81 (2019), 49–57.
- Perianin et al. (2020) Thomas Perianin, Sebastien Carré, Victor Dyseryn, Adrien Facon, and Sylvain Guilley. 2020. End-to-end automated cache-timing attack driven by Machine Learning. Journal of Cryptographic Engineering (2020), 1–12.
- Perin et al. (2020) Guilherme Perin, Łukasz Chmielewski, Lejla Batina, and Stjepan Picek. 2020. Keep it Unsupervised: Horizontal Attacks Meet Deep Learning. IACR Transactions on Cryptographic Hardware and Embedded Systems 2021, 1 (Dec. 2020), 343–372. https://doi.org/10.46586/tches.v2021.i1.343-372
- Picek et al. (2019) Stjepan Picek, Annelie Heuser, Alan Jovic, Shivam Bhasin, and Francesco Regazzoni. 2019. The curse of class imbalance and conflicting metrics with machine learning for side-channel evaluations. IACR Transactions on Cryptographic Hardware and Embedded Systems 2019, 1 (2019), 1–29.
- Picek et al. (2018) Stjepan Picek, Ioannis Petros Samiotis, Jaehun Kim, Annelie Heuser, Shivam Bhasin, and Axel Legay. 2018. On the performance of convolutional neural networks for side-channel analysis. In International Conference on Security, Privacy, and Applied Cryptography Engineering. Springer, 157–176.
- Pierazzi et al. (2020) F. Pierazzi, F. Pendlebury, J. Cortellazzi, and L. Cavallaro. 2020. Intriguing Properties of Adversarial ML Attacks in the Problem Space. In 2020 IEEE Symposium on Security and Privacy (SP). 1332–1349. https://doi.org/10.1109/SP40000.2020.00073
- Rahman et al. (2019) T. Rahman, M. Rochan, and Y. Wang. 2019. Video-Based Person Re-Identification using Refined Attention Networks. In 2019 16th IEEE International Conference on Advanced Video and Signal Based Surveillance (AVSS). 1–8. https://doi.org/10.1109/AVSS.2019.8909869
- Rathi et al. (2018) M. Rathi, A. Malik, D. Varshney, R. Sharma, and S. Mendiratta. 2018. Sentiment Analysis of Tweets Using Machine Learning Approach. In 2018 Eleventh International Conference on Contemporary Computing (IC3). 1–3. https://doi.org/10.1109/IC3.2018.8530517
- Rebryk and Beliaev (2020) Yurii Rebryk and Stanislav Beliaev. 2020. ConVoice: Real-Time Zero-Shot Voice Style Transfer with Convolutional Network. arXiv preprint arXiv:2005.07815 (2020).
- Ren et al. (2019) Yi Ren, Xu Tan, Tao Qin, Sheng Zhao, Zhou Zhao, and Tie-Yan Liu. 2019. Almost unsupervised text to speech and automatic speech recognition. arXiv preprint arXiv:1905.06791 (2019).
- Ribeiro et al. (2016) Marco Tulio Ribeiro, Sameer Singh, and Carlos Guestrin. 2016. “Why Should I Trust You?”: Explaining the Predictions of Any Classifier. In 22nd ACM SIGKDD Int’l Conf. Knowl. Disc. Data Mining (KDD ’16). ACM, New York, NY, USA, 1135–1144.
- Rigaki and Garcia (2018) M. Rigaki and S. Garcia. 2018. Bringing a GAN to a Knife-Fight: Adapting Malware Communication to Avoid Detection. In 2018 IEEE Security and Privacy Workshops (SPW). 70–75. https://doi.org/10.1109/SPW.2018.00019
- Roller et al. (2020) Stephen Roller, Emily Dinan, Naman Goyal, Da Ju, Mary Williamson, Yinhan Liu, Jing Xu, Myle Ott, Kurt Shuster, Eric M. Smith, Y.-Lan Boureau, and Jason Weston. 2020. Recipes for building an open-domain chatbot. arXiv:2004.13637 [cs] (April 2020). http://arxiv.org/abs/2004.13637 arXiv: 2004.13637.
- Salminen et al. (2019) Joni Salminen, Soon-gyo Jung, and Bernard J Jansen. 2019. The Future of Data-driven Personas: A Marriage of Online Analytics Numbers and Human Attributes.. In ICEIS (1). 608–615.
- Salminen et al. (2020) Joni Salminen, Rohan Gurunandan Rao, Soon-gyo Jung, Shammur A Chowdhury, and Bernard J Jansen. 2020. Enriching Social Media Personas with Personality Traits: A Deep Learning Approach Using the Big Five Classes. In International Conference on Human-Computer Interaction. Springer, 101–120.
- Samulowitz and Memisevic (2007) Horst Samulowitz and Roland Memisevic. 2007. Learning to solve QBF. In AAAI, Vol. 7. 255–260.
- Schreyer et al. (2019) Marco Schreyer, Timur Sattarov, Bernd Reimer, and Damian Borth. 2019. Adversarial Learning of Deepfakes in Accounting. arXiv:1910.03810 [cs.LG]
- Schwartz and Kurniawati (2019) Jonathon Schwartz and Hanna Kurniawati. 2019. Autonomous penetration testing using reinforcement learning. arXiv preprint arXiv:1905.05965 (2019).
- Seymour and Tully (2016) John Seymour and Philip Tully. 2016. Weaponizing data science for social engineering: Automated E2E spear phishing on Twitter. Black Hat USA 37 (2016), 1–39.
- Seymour and Tully (2018) John Seymour and Philip Tully. 2018. Generative models for spear phishing posts on social media. arXiv preprint arXiv:1802.05196 (2018).
- Shafahi et al. (2018) Ali Shafahi, W. Ronny Huang, Mahyar Najibi, Octavian Suciu, Christoph Studer, Tudor Dumitras, and Tom Goldstein. 2018. Poison Frogs! Targeted Clean-Label Poisoning Attacks on Neural Networks. In Proceedings of the 32nd International Conference on Neural Information Processing Systems (Montréal, Canada) (NIPS’18). Curran Associates Inc., Red Hook, NY, USA, 6106–6116.
- Shan et al. (2020) Shawn Shan, Emily Wenger, Jiayun Zhang, Huiying Li, Haitao Zheng, and Ben Y Zhao. 2020. Fawkes: Protecting Privacy against Unauthorized Deep Learning Models. In 29th USENIX Security Symposium (USENIX Security 20). 1589–1604.
- shaoanlu (2020) shaoanlu. 2020. shaoanlu/faceswap-GAN: A denoising autoencoder + adversarial losses and attention mechanisms for face swapping. https://github.com/shaoanlu/faceswap-GAN. (Accessed on 10/19/2020).
- Sharif et al. (2016) Mahmood Sharif, Sruti Bhagavatula, Lujo Bauer, and Michael K Reiter. 2016. Accessorize to a crime: Real and stealthy attacks on state-of-the-art face recognition. In Proceedings of the 2016 ACM SIGSAC Conference on Computer and Communications Security. ACM, 1528–1540.
- Sharon et al. (2021) Yam Sharon, David Berend, Yang Liu, Asaf Shabtai, and Yuval Elovici. 2021. TANTRA: Timing-Based Adversarial Network Traffic Reshaping Attack. arXiv preprint arXiv:2103.06297 (2021).
- She et al. (2020) Dongdong She, Rahul Krishna, Lu Yan, Suman Jana, and Baishakhi Ray. 2020. MTFuzz: Fuzzing with a Multi-Task Neural Network. arXiv preprint arXiv:2005.12392 (2020).
- She et al. (2019) Dongdong She, Kexin Pei, Dave Epstein, Junfeng Yang, Baishakhi Ray, and Suman Jana. 2019. NEUZZ: Efficient fuzzing with neural program smoothing. In 2019 IEEE Symposium on Security and Privacy (SP). IEEE, 803–817.
- Shin et al. (2015) Eui Chul Richard Shin, Dawn Song, and Reza Moazzezi. 2015. Recognizing functions in binaries with neural networks. In 24th USENIX Security Symposium (USENIX Security 15). 611–626.
- Shokri et al. (2017) R. Shokri, M. Stronati, C. Song, and V. Shmatikov. 2017. Membership Inference Attacks Against Machine Learning Models. In 2017 IEEE Symposium on Security and Privacy (SP). 3–18.
- Shumailov et al. (2019) Ilia Shumailov, Laurent Simon, Jeff Yan, and Ross Anderson. 2019. Hearing your touch: A new acoustic side channel on smartphones. arXiv preprint arXiv:1903.11137 (2019).
- Shumailov et al. (2020) Ilia Shumailov, Yiren Zhao, Daniel Bates, Nicolas Papernot, Robert Mullins, and Ross Anderson. 2020. Sponge Examples: Energy-Latency Attacks on Neural Networks. arXiv preprint arXiv:2006.03463 (2020).
- Siarohin et al. (2019) Aliaksandr Siarohin, Stéphane Lathuilière, Sergey Tulyakov, Elisa Ricci, and Nicu Sebe. 2019. First Order Motion Model for Image Animation. In Conference on Neural Information Processing Systems (NeurIPS).
- Sidi et al. (2020) Lior Sidi, Asaf Nadler, and Asaf Shabtai. 2020. MaskDGA: An Evasion Attack Against DGA Classifiers and Adversarial Defenses. IEEE Access 8 (2020), 161580–161592.
- Singh and Thakur (2020) Siddhant Singh and Hardeo K Thakur. 2020. Survey of Various AI Chatbots Based on Technology Used. In 2020 8th International Conference on Reliability, Infocom Technologies and Optimization (Trends and Future Directions)(ICRITO). IEEE, 1074–1079.
- Song et al. (2001) Dawn Xiaodong Song, David A Wagner, and Xuqing Tian. 2001. Timing analysis of keystrokes and timing attacks on ssh.. In USENIX Security Symposium, Vol. 2001.
- Spiliotopoulos et al. (2020) Dimitris Spiliotopoulos, Dionisis Margaris, and Costas Vassilakis. 2020. Data-Assisted Persona Construction Using Social Media Data. Big Data and Cognitive Computing 4, 3 (2020), 21.
- Stupp ([n.d.]) Catherine Stupp. [n.d.]. Fraudsters Used AI to Mimic CEO’s Voice in Unusual Cybercrime Case. https://www.wsj.com/articles/fraudsters-use-ai-to-mimic-ceos-voice-in-unusual-cybercrime-case-11567157402. (Accessed on 10/14/2020).
- Suciu et al. (2019) O. Suciu, S. E. Coull, and J. Johns. 2019. Exploring Adversarial Examples in Malware Detection. In 2019 IEEE Security and Privacy Workshops (SPW). 8–14. https://doi.org/10.1109/SPW.2019.00015
- Sun et al. (2016) Jingchao Sun, Xiaocong Jin, Yimin Chen, Jinxue Zhang, Yanchao Zhang, and Rui Zhang. 2016. VISIBLE: Video-Assisted Keystroke Inference from Tablet Backside Motion.. In NDSS.
- Sun et al. (2018) Qianru Sun, Ayush Tewari, Weipeng Xu, Mario Fritz, Christian Theobalt, and Bernt Schiele. 2018. A hybrid model for identity obfuscation by face replacement. In Proceedings of the European Conference on Computer Vision (ECCV). 553–569.
- Sutro (2020) Alejo Grigera Sutro. 2020. Machine-Learning Based Evaluation of Access Control Lists to Identify Anomalies. https://www.tdcommons.org/dpubs_series/2870
- Szegedy et al. (2014) Christian Szegedy, Wojciech Zaremba, Ilya Sutskever, Joan Bruna, Dumitru Erhan, Ian Goodfellow, and Rob Fergus. 2014. Intriguing properties of neural networks. In International Conference on Learning Representations. http://arxiv.org/abs/1312.6199
- Tariq (2018) N. Tariq. 2018. IMPACT OF CYBERATTACKS ON FINANCIAL INSTITUTIONS. The Journal of Internet Banking and Commerce 23 (2018), 1–11.
- Truong et al. (2019) Thanh Cong Truong, Ivan Zelinka, and Roman Senkerik. 2019. Neural swarm virus. In Swarm, Evolutionary, and Memetic Computing and Fuzzy and Neural Computing. Springer, 122–134.
- Ucci et al. (2019) Daniele Ucci, Leonardo Aniello, and Roberto Baldoni. 2019. Survey of machine learning techniques for malware analysis. Computers & Security 81 (2019), 123–147.
- Wang and Gong (2018) Binghui Wang and Neil Zhenqiang Gong. 2018. Stealing hyperparameters in machine learning. In 2018 IEEE Symposium on Security and Privacy (SP). IEEE, 36–52.
- Wang et al. (2019b) Daimeng Wang, Ajaya Neupane, Zhiyun Qian, Nael B Abu-Ghazaleh, Srikanth V Krishnamurthy, Edward JM Colbert, and Paul Yu. 2019b. Unveiling your keystrokes: A Cache-based Side-channel Attack on Graphics Libraries.. In NDSS.
- Wang et al. (2019d) Qi Wang, Weiliang Zhao, Jian Yang, Jia Wu, Wenbin Hu, and Qianli Xing. 2019d. DeepTrust: A Deep User Model of Homophily Effect for Trust Prediction. In 2019 IEEE International Conference on Data Mining (ICDM). IEEE, 618–627.
- Wang et al. (2020) S. Wang, S. Nepal, C. Rudolph, M. Grobler, S. Chen, and T. Chen. 2020. Backdoor Attacks against Transfer Learning with Pre-trained Deep Learning Models. IEEE Transactions on Services Computing (2020), 1–1. https://doi.org/10.1109/TSC.2020.3000900
- Wang et al. (2019c) Xin Wang, Junichi Yamagishi, Massimiliano Todisco, Hector Delgado, Andreas Nautsch, Nicholas Evans, Md Sahidullah, Ville Vestman, Tomi Kinnunen, Kong Aik Lee, et al. 2019c. The ASVspoof 2019 database. arXiv preprint arXiv:1911.01601 (2019).
- Wang et al. (2017) Ya Wang, Tianlong Bao, Chunhui Ding, and Ming Zhu. 2017. Face recognition in real-world surveillance videos with deep learning method. In Image, Vision and Computing (ICIVC), 2017 2nd International Conference on. IEEE, 239–243.
- Wang et al. (2019a) Yao Wang, Wandong Cai, Tao Gu, and Wei Shao. 2019a. Your eyes reveal your secrets: an eye movement based password inference on smartphone. IEEE transactions on mobile computing (2019).
- Wang et al. (2018) Yao Wang, Wandong Cai, Tao Gu, Wei Shao, Ibrahim Khalil, and Xianghua Xu. 2018. GazeRevealer: Inferring password using smartphone front camera. In Proceedings of the 15th EAI International Conference on Mobile and Ubiquitous Systems: Computing, Networking and Services. 254–263.
- Wang et al. (2020) Yan Wang, Peng Jia, Luping Liu, Cheng Huang, and Zhonglin Liu. 2020. A systematic review of fuzzing based on machine learning techniques. PloS one 15, 8 (2020), e0237749.
- Wang et al. (2020) Yaqing Wang, Quanming Yao, James T. Kwok, and Lionel M. Ni. 2020. Generalizing from a Few Examples: A Survey on Few-Shot Learning. ACM Comput. Surv. 53, 3, Article 63 (June 2020), 34 pages. https://doi.org/10.1145/3386252
- Wang et al. (2011) Yipeng Wang, Zhibin Zhang, Danfeng Daphne Yao, Buyun Qu, and Li Guo. 2011. Inferring protocol state machine from network traces: a probabilistic approach. In International Conference on Applied Cryptography and Network Security. Springer, 1–18.
- Weissbart et al. (2019) Léo Weissbart, Stjepan Picek, and Lejla Batina. 2019. One Trace Is All It Takes: Machine Learning-Based Side-Channel Attack on EdDSA. In Security, Privacy, and Applied Cryptography Engineering, Shivam Bhasin, Avi Mendelson, and Mridul Nandi (Eds.). Springer International Publishing, Cham, 86–105.
- White et al. (2011) Andrew White, Austin Matthews, Kevin Snow, and Fabian Monrose. 2011. Phonotactic Reconstruction of Encrypted VoIP Conversations: Hookt on Fon-iks. Proceedings - IEEE Symposium on Security and Privacy, 3 – 18. https://doi.org/10.1109/SP.2011.34
- Woh and Lee (2018) S. Woh and J. Lee. 2018. Game State Prediction with Ensemble of Machine Learning Techniques. In 2018 Joint 10th International Conference on Soft Computing and Intelligent Systems (SCIS) and 19th International Symposium on Advanced Intelligent Systems (ISIS). 89–92. https://doi.org/10.1109/SCIS-ISIS.2018.00025
- Workman (2008) Michael Workman. 2008. Wisecrackers: A theory-grounded investigation of phishing and pretext social engineering threats to information security. Journal of the American Society for Information Science and Technology 59, 4 (2008), 662–674.
- Wu et al. (2021) Runze Wu, Jinxin Gong, Weiyue Tong, and Bing Fan. 2021. Network Attack Path Selection and Evaluation Based on Q-Learning. Applied Sciences 11, 1 (2021). https://doi.org/10.3390/app11010285
- Xu et al. (2017) Xiaojun Xu, Chang Liu, Qian Feng, Heng Yin, Le Song, and Dawn Song. 2017. Neural Network-based Graph Embedding for Cross-Platform Binary Code Similarity Detection. Proceedings of the 2017 ACM SIGSAC Conference on Computer and Communications Security (Oct 2017). https://doi.org/10.1145/3133956.3134018
- Yager (1984) R. R. Yager. 1984. Approximate reasoning as a basis for rule-based expert systems. IEEE Transactions on Systems, Man, and Cybernetics SMC-14, 4 (1984), 636–643. https://doi.org/10.1109/TSMC.1984.6313337
- Yang et al. (2018) Zichao Yang, Zhiting Hu, Chris Dyer, Eric P Xing, and Taylor Berg-Kirkpatrick. 2018. Unsupervised text style transfer using language models as discriminators. In Advances in Neural Information Processing Systems. 7287–7298.
- Yao et al. (2019) Yuanshun Yao, Huiying Li, Haitao Zheng, and Ben Y. Zhao. 2019. Latent Backdoor Attacks on Deep Neural Networks. In Proceedings of the 2019 ACM SIGSAC Conference on Computer and Communications Security (London, United Kingdom) (CCS ’19). Association for Computing Machinery, New York, NY, USA, 2041–2055. https://doi.org/10.1145/3319535.3354209
- Ye et al. (2020) Fangke Ye, Shengtian Zhou, Anand Venkat, Ryan Marucs, Nesime Tatbul, Jesmin Jahan Tithi, Paul Petersen, Timothy Mattson, Tim Kraska, Pradeep Dubey, et al. 2020. MISIM: An End-to-End Neural Code Similarity System. arXiv preprint arXiv:2006.05265 (2020).
- Yousefi et al. (2018) M. Yousefi, N. Mtetwa, Y. Zhang, and H. Tianfield. 2018. A Reinforcement Learning Approach for Attack Graph Analysis. In 2018 17th IEEE International Conference On Trust, Security And Privacy In Computing And Communications/ 12th IEEE International Conference On Big Data Science And Engineering (TrustCom/BigDataSE). 212–217. https://doi.org/10.1109/TrustCom/BigDataSE.2018.00041
- Yu et al. (2019) Jiadi Yu, Li Lu, Yingying Chen, Yanmin Zhu, and Linghe Kong. 2019. An indirect eavesdropping attack of keystrokes on touch screen through acoustic sensing. IEEE Transactions on Mobile Computing (2019).
- Yun et al. (2019) Seongjun Yun, Minbyul Jeong, Raehyun Kim, Jaewoo Kang, and Hyunwoo J Kim. 2019. Graph transformer networks. arXiv preprint arXiv:1911.06455 (2019).
- Zelinka et al. (2018) Ivan Zelinka, Swagatam Das, Lubomir Sikora, and Roman Šenkeřík. 2018. Swarm virus-Next-generation virus and antivirus paradigm? Swarm and Evolutionary Computation 43 (2018), 207–224.
- Zeng and Church (2009) W. Zeng and R. L. Church. 2009. Finding Shortest Paths on Real Road Networks: The Case for A*. Int. J. Geogr. Inf. Sci. 23, 4 (April 2009), 531–543. https://doi.org/10.1080/13658810801949850
- zerofox (2020) zerofox. 2020. zerofox-oss/SNAP_R: A machine learning based social media pentesting tool. https://github.com/zerofox-oss/SNAP_R. (Accessed on 10/21/2020).
- Zhang et al. (2019) Huan Zhang, Hongge Chen, Zhao Song, Duane S. Boning, Inderjit S. Dhillon, and Cho-Jui Hsieh. 2019. The Limitations of Adversarial Training and the Blind-Spot Attack. In 7th International Conference on Learning Representations, ICLR 2019, New Orleans, LA, USA, May 6-9, 2019. OpenReview.net. https://openreview.net/forum?id=HylTBhA5tQ
- Zhang and Chen (2018) Muhan Zhang and Yixin Chen. 2018. Link prediction based on graph neural networks. In Advances in Neural Information Processing Systems. 5165–5175.
- Zhang et al. (2012) W. Zhang, R.Y.K. Lau, S.S.Y. Liao, and R.C.-W Kwok. 2012. A probabilistic generative model for latent business networks mining. International Conference on Information Systems, ICIS 2012 2 (01 2012), 1102–1118.
- Zhang (2018) X. Zhang. 2018. Analysis of New Agent Tesla Spyware Variant. {https://www.fortinet.com/blog/threat-research/analysis-of-new-agent-tesla-spyware-variant.html}
- Zhang et al. (2016) Y. Zhang, J. E. Meng, and M. Pratama. 2016. Extractive document summarization based on convolutional neural networks. In IECON 2016 - 42nd Annual Conference of the IEEE Industrial Electronics Society. 918–922. https://doi.org/10.1109/IECON.2016.7793761
- Zhiyang et al. (2019) Fang Zhiyang, Junfeng Wang, Boya Li, Siqi Wu, Yingjie Zhou, and Haiying Huang. 2019. Evading Anti-Malware Engines With Deep Reinforcement Learning. IEEE Access PP (03 2019), 1–1. https://doi.org/10.1109/ACCESS.2019.2908033
- Zhou et al. (2017) Bing Zhou, Mohammed Elbadry, Ruipeng Gao, and Fan Ye. 2017. BatMapper: Acoustic sensing based indoor floor plan construction using smartphones. In Proceedings of the 15th Annual International Conference on Mobile Systems, Applications, and Services. 42–55.
- Zhu et al. (2018) X. Zhu, X. Jing, X. You, X. Zhang, and T. Zhang. 2018. Video-Based Person Re-Identification by Simultaneously Learning Intra-Video and Inter-Video Distance Metrics. IEEE Transactions on Image Processing 27, 11 (2018), 5683–5695. https://doi.org/10.1109/TIP.2018.2861366