11email: [email protected] 22institutetext: Thrust of Financial Technology, The Hong Kong University of Science and Technology (Guangzhou), Guangzhou, China
22email: [email protected] 33institutetext: Department of Computer Science and Engineering, The Hong Kong University of Science and Technology, Hong Kong, China
33email: [email protected]
The Impact of Generative Artificial Intelligence on Market Equilibrium: Evidence from a Natural Experiment
Abstract
Generative artificial intelligence (AI) exhibits the capability to generate creative content akin to human output with greater efficiency and reduced costs. This groundbreaking capability, however, has ignited a debate regarding its potential to displace human creators. In light of these discussions, this paper empirically investigates the impact of generative AI on market equilibrium, in the context of China’s leading art outsourcing platform. We overcome the challenge of causal inference by identifying an unanticipated and sudden leak of an advanced image-generative AI as a natural experiment. This leak precipitated a notable reduction in the production costs of anime-style images compared to other genres, thereby providing a unique opportunity for difference-in-differences comparisons. Our analysis shows that the advent of generative AI led to a 64% reduction in average prices, yet it simultaneously spurred a 121% increase in order volume and a 56% increase in overall revenue. This growth is primarily driven by the rising demand for "low-end" personal orders, rather than commercial orders. Moreover, incumbent creators retain the majority of the market share and reap the most benefits of generative AI. Our research highlights the potential of generative AI to benefit all stakeholders across the platform economy, yielding both scholarly contributions and practical implications.
Keywords:
Artificial Intelligence Generative AI Market Equilibrium Online Platform Platform Economy1 Introduction
Generative artificial intelligence (AI) appears to propel our world closer to the realm of Cyberpunk—a futuristic milieu characterized by the coexistence of "high tech and low life": Generative AI demonstrates its ability to generate text, images, and videos that are similar to content created by human experts – but in much less time, at a fraction of the cost, and with amazing creativity [43]. Such advances, however, have raised significant concerns about the potential for job displacement. One notable manifestation is the organized opposition of artists, who have taken to social media to voice their opposition through campaigns such as "NO to AI-generated images" [20].
However, the potential impact of generative AI remains controversial in academia. Distinguished AI scientists such as Turing Award winners Yoshua Bengio, Yann LeCun, and Geoffrey Hinton - recognized with what is often called the "Nobel Prize of computer science" - hold divergent views. While Bengio and Hinton have publicly expressed concern, warning of "the risk of extinction by AI," LeCun dismisses such fears as unfounded, citing his understanding of the limitation of current AI systems. This debate echoes the broader discourse found in the economics literature, which suggests that while information technology may carry the risk of rendering certain jobs obsolete [1, 6, 17, 5, 45], it may also benefit workers by increasing their productivity [7, 29, 28].
To this end, this paper investigates the impact of generative AI on market equilibrium, with empirical data from China’s leading art outsourcing platform. Specifically, we seek to answer the following research questions: RQ1: In what ways does generative AI shape market equilibrium in terms of average price, order volume, and overall revenue? RQ2: What types of demand are eliminated or created by generative AI? RQ3: Who emerges as the primary suppliers in the market—are they incumbents or entrants utilizing generative AI?
The main challenge in assessing the impact of generative AI lies in achieving causal inference. This involves establishing a true cause-and-effect relationship between generative AI and the focal outcomes, rather than mere correlation. One straightforward approach is to contrast the outcome variable (e.g., the number of orders) after and before the emergence of generative AI. However, this method lacks the capacity for causal inference. For example, the demand for anime images could naturally fluctuate due to the seasonal effect of a new semester for students. This intrinsic variability could potentially bias our estimates of the impact of generative AI, leading to incorrect estimates. Another approach is randomized experiments (or A/B testing), which offer the most compelling evidence for causality. However, using experiments to study the impact of generative AI on markets is not feasible. Unlike individuals, markets cannot be randomly assigned to different groups, which limits the ability to implement generative AI effects only on the treated group while leaving the control group unaffected. Macro-level analyses involving countries, cities, and industries face similar limitations.
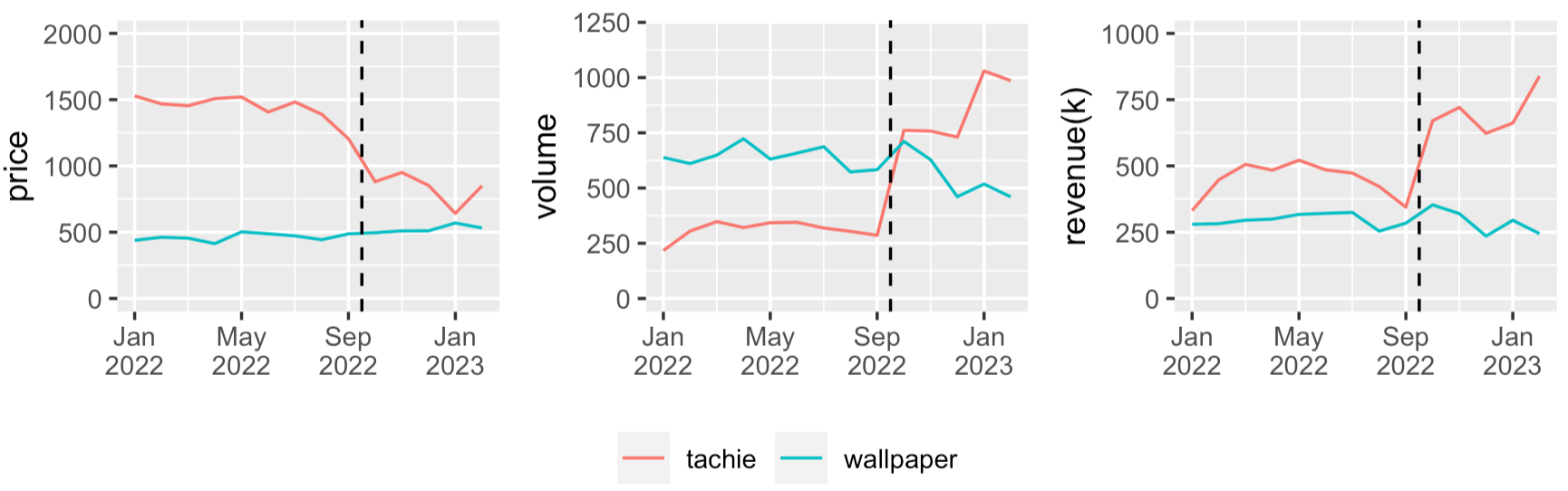
To overcome the challenge of causal inference, we identify an unanticipated and sudden leak of a highly proficient image-generative AI as a natural experiment, where markets inadvertently encounter experimental and control conditions, resembling a scenario wherein nature orchestrates an experiment.
Among the pioneer platforms in the field, NovelAI stands out as an early entrant, offering text-to-image generative AI services. These services are available for a subscription fee ranging from $10 to $25 per month per user. Powered by Stable Diffusion [34], NovelAI’s AI can quickly generate anime-style images in response to specific keyword-based requests. However, in early October 2022, hackers attacked NovelAI and leaked the AI model and training codes used by NovelAI. As a result, the leaked resources are available for download and local implementation, effectively reducing the cost of image generation to nearly zero.
The inadvertent leak, which originated in the Western world, has inadvertently reverberated throughout the Eastern world. Prior to October 2022, China lacked domestic providers of generative AI, while access to foreign alternatives was also impeded. After October 2022, however, the leaked AI, along with its accompanying tutorials, quickly spread throughout China’s social media landscape. As a result, this leak has expeditiously transitioned China from a state of being unaffected by generative AI to one of being affected by it.
This leak served as an ideal natural experiment. First, China constitutes an environment where painting markets remained unaffected by generative AI prior to the leak due to policy restrictions, language restrictions, and payment restrictions. Second, the leaked AI specializes exclusively in generating anime-style images, thus affecting only the anime painting markets while leaving other painting styles unaffected, allowing for a comparison between treated and control units. Third, the unanticipated and sudden nature of the leak is exogenous. This feature ensures that the focal markets are unlikely to have been affected by unobserved confounding factors as stakeholders did not have time to react to the leak in advance. Thus, alternative explanations are effectively ruled out.
We collected 197,068 records from a leading art outsourcing platform in China from January 2022 to February 2023. This platform allows consumers to place orders with specifications and budgets, and artists as freelancers to fulfill them. The orders are segmented into distinct markets, such as anime, UI, live2d, and wallpaper. We transformed these order records into a market-week panel dataset, focusing on average price, order volume, and overall revenue. Using a difference-in-differences research design, we compare the anime market to the wallpaper market, before and after the unintended leak of NovelAI’s anime generative AI. Our main findings are summarized in Figure 1.
Our analysis reveals a promising effect on market equilibrium following the emergence of generative AI. Specifically, although this emergence leads to a 64% reduction in the average price, the generative AI also leads to a remarkable 121% increase in order volume and a consequent 56% increase in overall revenue. All effects are statistically significant at the 1% level. In addition, the effects are also economically significant, due to their substantial magnitude. The results suggest that generative AI can contribute to a more prosperous market, where each stakeholder benefits: consumers get cheaper services, and human creators and the platform get more orders and revenue.
We then examine what types of demand are created by generative AI. Orders on the platform are categorized into personal orders and commercial orders, with different licenses. We conduct a heterogeneous analysis, showing that the growth of generative AI is mainly driven by personal orders, rather than commercial orders. The "tachie" market achieved significantly more revenue through more personal orders at low prices, while the price, volume, and revenue of commercial orders are relatively stable. Our results indicate the potential of generative AI for business success by satisfying the "low-end" needs of individual users.
Finally, we examine who emerges as the primary suppliers in the market. Our results show that the incumbents, who registered before the leak, won 97% of the orders after the leak. As a result, they reap most of the benefits of increased order volume and revenue. The results rule out the possibility that new entrants using generative AI have taken over the market share of real human creators.
Our work provides valuable managerial implications. First, the increased order volume and overall revenue indicate more jobs and taxes, providing favorable economic evidence of generative AI for policymakers to make supportive AI policies. Second, generative AI mainly benefits incumbent creators, suggesting that they should welcome and incorporate generative AI into their workflow to further increase their productivity. Third, online platforms can conduct marketing campaigns and improve services specifically for individual users to better exploit the blue ocean driven by generative AI.
In conclusion, this paper makes the following contributions:
-
•
To the best of our knowledge, we pioneer the estimation of the impact of generative AI on market equilibrium, making scholarly contributions to the growing literature on the economics of (generative) AI.
-
•
We provide promising empirical evidence that generative AI can promote market prosperity and explain the reason. We also provide valuable managerial insights for various stakeholders.
-
•
Our identified natural experiment facilitates causal inference for future research on generative AI—its effects on customer satisfaction, on employment, and beyond.
2 Related Literature
This paper adopts empirical research methods to study generative AI, which is related to the field of generative AI and the economics of AI.
2.1 Generative AI
Generative AI is defined as artificial intelligence (AI) algorithms that generate original outputs based on prompt inputs, which is distinguished from discriminative AI in that generative AI synthesizes the data it has been trained on and creates similar content created by human experts [43].
Generative AI has many applications, which their output format can categorize. For text, the applications include machine translation [3], code generation [38], natural language generation [11], etc. For images, the applications include text-to-image generation [31], style transfer [12], image translation [19], etc. For audio, the applications include text-to-speech [32], music composition [9], singing voice conversion [26], etc.
Generative AI’s underlying generative models include Autoregressive Model (AR) [25], Generative Adversarial Network (GAN) [14], Normalizing Flow (Flow) [33], Variational Auto-Encoder (VAE) [22], and Denoising Diffusion Probabilistic Model (Diffusion) [16]. For example, OpenAI’s ChatGPT adopts an autoregressive model [4] with Transformer architecture [41]. The focal generative AI in this paper, NovelAI’s model, adopts stable diffusion [34].
Stable diffusion is a cutting-edge text-to-image deep learning model that generates images based on textual descriptions. Training Stable Diffusion takes two steps: initially, a large dataset of text-image pairs is used to teach the model the complex relationships between textual descriptions and visual content; subsequently, the model learns to iteratively refine images from noise, given these text inputs.
The technical foundations of stable diffusion are relevant to our work in the following ways. First, stable diffusion can only generate images from the same distribution of its training set. Since NovelAI’s model is trained on anime images, it can only generate anime images, allowing for comparison across genres. Second, stable diffusion may struggle with maintaining fidelity to specific details in the text prompts, especially for complex or highly detailed descriptions, resulting in images that are somewhat generic or miss nuanced aspects of the desired output. This limitation can qualitatively explain why generative AI mainly affects low-end orders and cannot replace incumbent artists.
2.2 The Economics of AI
The economics of artificial intelligence (AI) refers to the study of how AI affects markets, organizations, and individuals, and how it changes the way businesses operate and make strategic decisions.
The main research method for studying the economics of AI is empirical research, which meticulously examines the impact of AI adoption on a focal area through the careful analysis of observational data and controlled experiments. Past research has examined the impact of AI on areas such as innovation [2], human resource management [39], sales [23], consumer behavior [37], international trade [13], education [30] and healthcare [44].
A stream of papers on the impact of AI on the labor market [42, 10] is closely related to our work. These papers mainly focus on how AI affects the employment and wages of workers, and who is more or less likely to be affected by AI. The literature reveals two contradictory aspects: on the one hand, AI may carry the risk of job displacement [1, 6, 17, 5, 45]; on the other hand, AI may also benefit workers by increasing their productivity [7, 29, 28].
Our paper contributes to the literature on the economics of (generative) artificial intelligence. First, we pioneer the estimation of the impact of generative AI, the new era of AI whose effects are still unclear and understudied. Second, our research target, a marketplace, is unique and provides perspectives from both the supplier and consumer sides, providing more insights into the impact of generative AI. Third, our identified natural experiment facilitates future research on generative AI by identifying an ideal scenario for causal inference.
3 Empirical Strategy
In this section, we compare different empirical methods for estimating the causal effects of generative AI. We apply our analysis under the potential outcome framework [35], the most widely used framework for causal inference.
3.1 Pretest-Posttest Design and Experimental Design
Let us consider the case of analyzing a set of units, labeled from to . For each unit , there are two potential outcomes depending on whether the unit receives treatment or not (). The individual treatment effect for unit is then defined as . In this paper, the units are markets of different art genres, the treatment is the emergence of generative AI and the outcomes are market equilibrium variables, i.e., price, volume, and revenue.
The main challenge in making causal inferences is posed by the fact that there is only one potential outcome that can be observed for each unit. For treated units (), we can only observe ; for control units(), we can only observe . As a result, individual treatment effects can never be estimated unless strong assumptions about the data generation process are made. As an alternative, researchers estimate the average treatment effect (ATE, ) or the average treatment effect on the treated (ATT, ).
We further extend our demonstration to two time periods. In the pretest period (), all units are not treated, where we can observe and . In the posttest period (), the treated units () have received the treatment while the control units () remain untreated. We can observe and . Our goal is to estimate ATE () or ATT (). Note that we still cannot observe another potential outcome ( and ).
A straightforward yet unsophisticated method is the pretest-posttest design, which compares the outcome variable before and after the treatment. Its estimator is . However, ATT equals , which indicates that the estimator of the pretest-posttest design is unbiased only if , i.e., the outcome variable does not change for the treated units if they do not receive the treatment.
However, the equation does not hold in the context of this study. A plausible explanation for this discrepancy could be the influence of seasonal effects. For example, the demand for anime images could naturally fluctuate after October 2022 due to the start of a new academic semester for students. This intrinsic variability could potentially skew our estimates of the impact of generative AI, leading to underestimates, overestimates, or even fundamentally incorrect estimates. As a result, it’s clear that the pretest-posttest design is not an appropriate approach for this study.
Another method is randomized experiments, also called a/b tests in some literature. This method randomly assigns units to a treated group () and a control group (), ensuring that the population of the treated group is the same as the population of the control group. So we have . We can then derive ATT by subtracting from , since we can observe both parts. Also, ATT is equal to ATE in randomized experiments. Thus, randomized experiments are considered the best method for causal inference.
However, randomized experiments are not feasible in this study. While the individual level of analysis allows for the division of subjects into treatment and control groups, the challenge arises at the market level of analysis. Unlike individuals, markets cannot be randomly assigned to different groups, which limits the ability to implement generative AI effects only on the treated group while leaving the control group unaffected. Macro-level analyses involving countries, cities, and industries face similar limitations. In these contexts, researchers have to gain valuable insights from observational data rather than from controlled interventions.
3.2 Difference-In-Differences Design
Difference-in-differences (DID) is arguably the most popular research design in the quantitative social sciences. It can be traced back to 1855 [36] and has also made great strides in recent years [15].
The DID estimator is . DID first calculates the differences between the pretest and posttest periods for each group. DID then takes the difference between these two differences as the estimator.
The DID design is based on the parallel trend assumption that the trends in the outcome variable for the treatment and control groups would have been parallel in the absence of the treatment. Formally, the DID estimator equals ATT if . The parallel trend assumption can be deemed satisfied if the treatment and control groups exhibit comparable pre-treatment trends in the outcome variable.
The application of the difference-in-differences to examine the impact of generative AI on market equilibrium hinges on three pivotal conditions: an appropriate treatment that affects treated markets subsequent to the treatment, not prior to it; minimal impact on control markets; and the fulfillment of the parallel trend assumption between treated and control markets.
This study employs the difference-in-differences design, utilizing an unanticipated leak of an advanced generative AI as the treatment. Prior to the leak, the trends of the treated and control markets were stable and parallel, as illustrated in Figure 1, which lends support to the validity of the parallel trend assumption.
4 Research Context and Data
4.1 The NovelAI "experiment"
NovelAI is one of the first commercial websites offering generative AI services. NovelAI was initially launched on June 15, 2021, when it only offered AI story-writing services. After Stable Diffusion, a state-of-the-art text-to-image algorithm, was released on March 2, 2022, NovelAI began offering its text-to-image services. NovelAI put a lot of effort into collecting anime-style images and used them to train an anime-style generative AI model based on Stable Diffusion. NovelAI’s text-to-image service was implemented on October 3, 2022 [27].
NovelAI’s generative AI takes a series of prompts, or keywords, such as "masterpiece, magical girl, fantasy, caustic" as input. The generative AI will generate images according to the prompts. Figure 4 in the Appendix presents the official examples of NovelAI. Note that NovelAI’s AI only generates anime-style images because it is trained on anime-style images.
NovelAI’s generative AI can generate an image in a few seconds and generate multiple images simultaneously. The cost of generating an image depends on its quality and resolution size. NovelAI uses a monthly subscription scheme ranging from $10 to $25. $10 users can generate approximately 200 images of normal quality per month. $25 users can generate an unlimited number of images.
However, on October 6, 2022, a few days after NovelAI’s text-to-image service was released, hackers attacked NovelAI’s server and released its AI model and associated training code. Anyone can download the leaked material via a shared seed and implement the same AI locally, allowing people to generate an image in a few seconds for free.
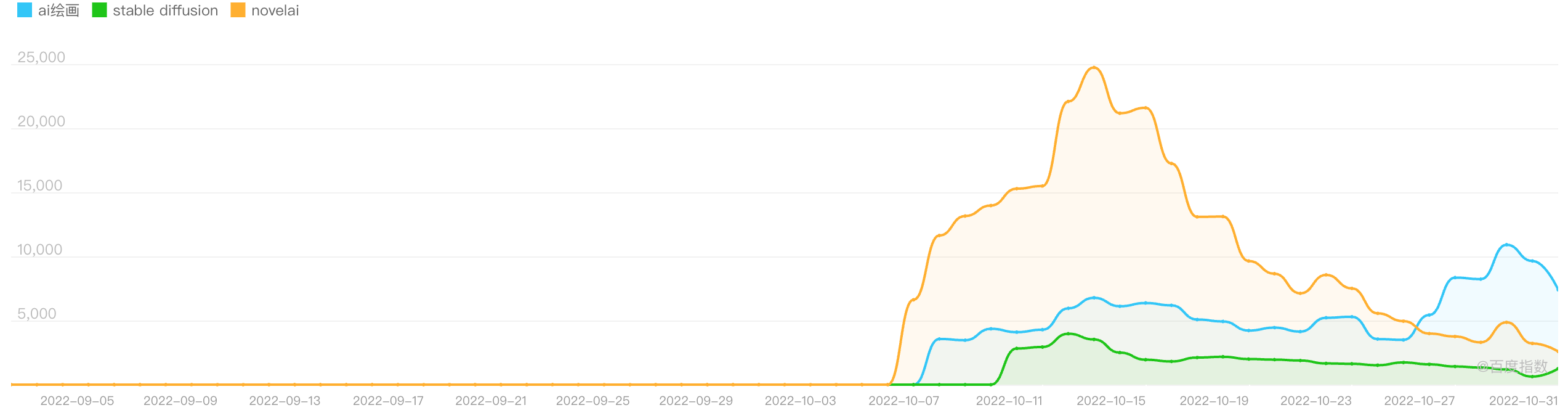
This leak served as an ideal natural experiment for several reasons. First, China constitutes an environment where painting markets remained unaffected by generative AI before the leak. China did not yet have a local generative AI provider, and people cannot access foreign generative AI providers due to policy restrictions, language restrictions, and payment restrictions. Figure 2 shows the search trend from Baidu, the largest search engine in China. The trend verifies that painting markets had not been affected by generative AI before the leak. Second, the leaked AI exclusively specializes in generating anime-style images, thereby exerting an impact solely on the anime painting markets while leaving other painting styles unaffected, allowing for a comparison between treated and control units. Third, the leak caused by hackers is unanticipated and sudden, which contributes to exogeneity. This attribute ensures that the focal markets are unlikely to have been affected by unobserved confounding factors since stakeholders had no time to react to the leak in advance. As a result, alternative explanations beyond the emergence of generative AI are effectively ruled out.
4.2 Data Description
We collect data from Mihuashi, the leading art outsourcing platform in China. Consumers, as demanders, can post an order on the focal platform, and artists, as producers, can express their interest in accepting the order. The consumer can then select one of the interested artists and enter into a contract with the selected artist. Once the artist has completed the task, the consumer makes the payment. The platform charges a commission of 5% of the price of the order.
An order on the focal platform is comprised of an art category, a price range, and a description. The art category includes "original character", "artwork", "avatar", "Q style", "tachie", "wallpaper", "live2d", "scenario", "cover", "poster", "UI", "character design", and "CG". The price range is defined by a lower and an upper bound, such as 50 to 200 yuan. The order description specifies the requirement, such as "a wallpaper for Vtuber live streaming background".
We collected data on the focal platform from January 2022 to February 2023, which contains 197,068 orders in total. We removed 408 outlier orders whose price is more than 70,000 yuan (about $10,000) because they are too expensive to become real orders. We then aggregate the data to the category-week level. For each category and each week, we calculate the average price (price), order volume (volume), and overall revenue (revenue). We calculate the average price and overall revenue based on the lower bound because the lower bound may better reflect the true willingness of consumers to accept their orders than the upper bound.
Mean | SD | Min | Median | Max | |
---|---|---|---|---|---|
Panel A: All Orders | |||||
Price | 843.7 | 460.7 | 334.6 | 628.0 | 2473.2 |
Volume | 123.7 | 54.5 | 12.0 | 130.0 | 318.0 |
Revenue | 92362.1 | 45096.0 | 6000.0 | 78950.0 | 264104.0 |
Price_upper | 2237.5 | 869.3 | 1212.2 | 1949.5 | 4689.1 |
Revenue_upper | 251996.5 | 103591.0 | 21550.0 | 230810.0 | 656074.0 |
Panel B: Completed Orders | |||||
Price | 885.9 | 472.3 | 327.9 | 721.5 | 2321.6 |
Volume | 43.2 | 18.1 | 1.0 | 43.0 | 89.0 |
Revenue | 34718.8 | 19238.0 | 500.0 | 30501.0 | 111170.0 |
Price_upper | 2266.1 | 901.7 | 1152.0 | 2010.9 | 5154.8 |
Revenue_upper | 92299.1 | 46196.5 | 1500.0 | 81650.0 | 286370.0 |
We select the category "tachie" as the treated market. The "tachie" is a Japanese term in the context of anime, manga, and video games that refers to the full-body standing drawing of a character.111https://en.wiktionary.org/wiki/%E7%AB%8B%E3%81%A1%E7%B5%B5#Japanese The "tachie" is precisely the style that NovelAI’s AI produces. In contrast, we select the "wallpaper" as the control market. A control unit should be comparable to the treated unit; thus, "live2d," "UI," and "CG" were excluded from consideration because they are beyond the scope of paintings. With regard to the remaining categories, they are likely to be partially affected by NovelAI’s generative AI, because the generative AI may only be useful with careful prompt engineering and post-processing, which could result in an underestimation of the effects. The "wallpaper" may be the best control unit because it is unlikely that NovelAI’s generative AI will generate elaborate wallpapers.
Table 1 presents the summary statistics for the category-week panel dataset in this paper. Price refers to the average price. Volume refers to order volume. Revenue refers to the overall revenue. The currency of Price and Revenue is Chinese yuan (7 yuan = $1). Also, we show the price and revenue based on the upper bound, which is Price_upper and Revenue_upper. Panel A presents the statistical data for all orders, while Panel B displays the statistical data for only completed orders.
5 Main Analysis
In this section, we answer the research question RQ1, by examining the impact of generative AI on market equilibrium in terms of average price, order volume, and overall revenue.
We employ the standard multi-periods model specification of difference-in-differences with two-way fixed effects [8]:
(1) |
In this model, the variable represents one of our outcome variables by category in week . is a dummy variable indicating whether category belongs to the treated group () or the control group (). is also a dummy variable indicating whether week is after the natural experiment () or before the natural experiment (). The model includes a fixed effect for each week to control for observed and unobserved nonparametric time trends. Additionally, the model includes a fixed effect for each category to account for time-invariant differences between categories. The parameter of interest is , which captures the impact of generative AI on the focal outcome variable.
Dependent Variables: | price | volume | revenue | log(price) | log(volume) | log(revenue) |
---|---|---|---|---|---|---|
Model: | (1) | (2) | (3) | (4) | (5) | (6) |
Variables | ||||||
treated after | -646.5∗∗∗ | 140.3∗∗∗ | 56,674.4∗∗∗ | -0.643∗∗∗ | 1.21∗∗∗ | 0.567∗∗∗ |
() | () | () | () | () | () | |
Fixed-effects | ||||||
category | Yes | Yes | Yes | Yes | Yes | Yes |
week | Yes | Yes | Yes | Yes | Yes | Yes |
Fit statistics | ||||||
Observations | 126 | 126 | 126 | 126 | 126 | 126 |
R2 | 0.88131 | 0.89730 | 0.84262 | 0.91405 | 0.93921 | 0.88433 |
Within R2 | 0.49208 | 0.79015 | 0.36957 | 0.53003 | 0.83278 | 0.35453 |
Clustered (category) standard-errors in parentheses | ||||||
Signif. Codes: ***: 0.01, **: 0.05, *: 0.1 |
Table 2 shows the estimates for the change in price, volume, and revenue in columns (1) to (3), respectively. The coefficient estimate of price is -646, indicating generative AI lowered the average price by 646 yuan. Notably, the coefficient estimates of volume and revenue are 140 and 56,674, showing that generative AI increased the number of orders by 140 per week and increased the total revenue by 56,674 yuan per week. In addition, columns (4) to (6) take the logarithm of price, volume, and revenue and show their percentage change. The results show that price decreased by 64%, volume increased by 121%, and revenue increased by 56%. All effects are statistically significant at the 1% level.
Public concern about generative AI is often rooted in negative anticipation of the economic ramifications associated with its adoption. In particular, there is an understandable concern among various segments, including artists, that generative AI could lead to feelings of displacement, given the fear of rapid and unceremonious replacement after years of dedicated study and creative toil.
However, our empirical findings provide a counterintuitive insight, showing that contrary to initial impressions, generative AI can benefit all stakeholders across the platform economy. Consumers can fulfill their orders 64% cheaper than before, indicating an improvement in consumer welfare. Furthermore, artists receive 56% more revenue, as each monetary transaction completed by consumers is considered revenue for the artist. They receive 121% more orders, which underscores the increased opportunities that accelerate the evolution of their artistic endeavors by providing an invaluable platform for rapid prototyping and iterative refinement. Moreover, the platform’s revenue also increases, as their commission is proportional to the total revenue. The substantial increase in order volume could further benefit the platform economy through the network effect [40, 24].
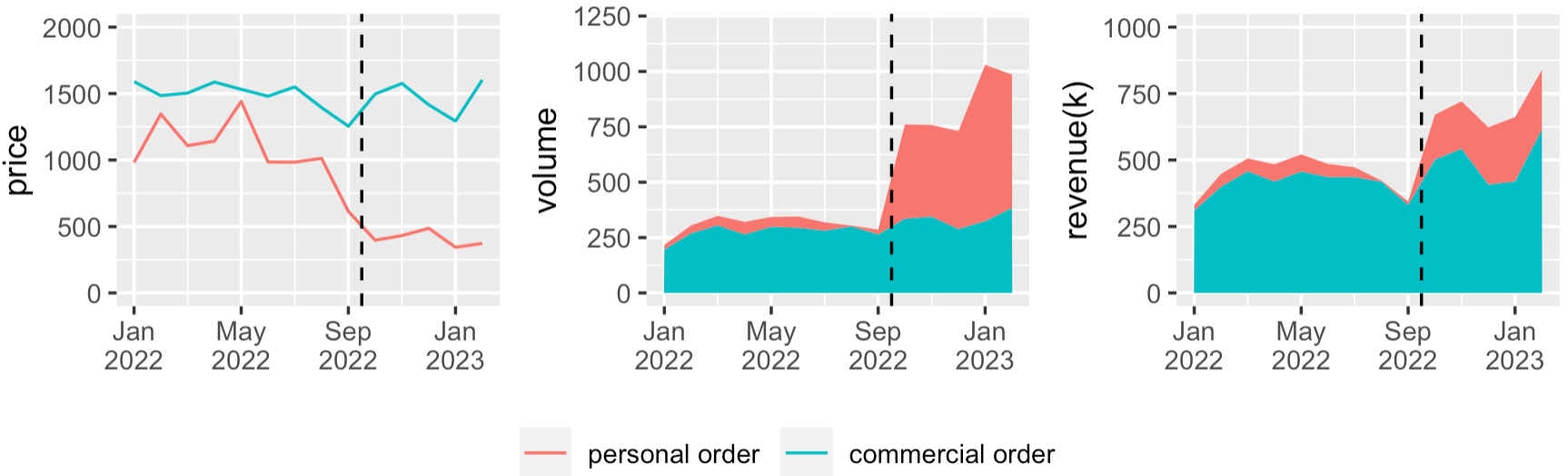
6 Demand Side Analysis
In this section, we answer research question RQ2, which aims to identify the specific demand growth that drives market prosperity222”Once you start thinking about growth, it’s hard to think about anything else,” said Robert Lucas, a Nobel Prize-winning economist.. To this end, the heterogeneous effects on order licenses are examined.
The orders on the focal platform are classified into two categories based on licenses: personal orders and commercial orders. The artwork of personal orders is for personal use only, whereas the artwork of commercial orders is permitted for commercial purposes. In particular, commercial orders are granted more extensive property rights than personal orders, such as distribution rights and rental rights.
Figure 3 shows the market equilibrium outcomes of personal orders and commercial orders in the ”tachie” market. Prior to and following the advent of generative AI, the price, volume, and revenue of commercial orders remain relatively stable. In contrast, personal orders exhibit a substantial increase in volume and revenue with a corresponding decrease in price, which aligns with the overall "tachie" market. This suggests that the growth in the "tachie" market is primarily driven by personal orders.
Dependent Variables: | price | volume | revenue | log(price) | log(volume) | log(revenue) |
---|---|---|---|---|---|---|
Model: | (1) | (2) | (3) | (4) | (5) | (6) |
Variables | ||||||
personal_treated after | -746.0∗∗ | 129.5∗∗∗ | 41,244.4∗∗∗ | -0.9892∗∗∗ | 2.953∗∗∗ | 1.964∗∗∗ |
(17.61) | (1.520) | (195.6) | (0.0101) | (0.0162) | (0.0061) | |
commercial_treated after | -50.57∗∗∗ | 37.25∗∗∗ | 21,115.6∗∗∗ | -0.0967∗∗∗ | 0.3903∗∗∗ | 0.2936∗∗∗ |
() | () | () | () | () | () | |
Fixed-effects | ||||||
art_category | Yes | Yes | Yes | Yes | Yes | Yes |
year_week | Yes | Yes | Yes | Yes | Yes | Yes |
Fit statistics | ||||||
Observations | 185 | 185 | 185 | 185 | 185 | 185 |
R2 | 0.78796 | 0.91291 | 0.82473 | 0.79054 | 0.92775 | 0.83418 |
Within R2 | 0.59778 | 0.73866 | 0.77730 | 0.63074 | 0.85464 | 0.76433 |
Clustered (art_category) standard-errors in parentheses | ||||||
Signif. Codes: ***: 0.01, **: 0.05, *: 0.1 |
Formally, we replace the term, , in Equation 1 with and to capture heterogeneous treatment effects for different order licenses:
(2) |
Table 3 presents the heterogeneous impact of Generative AI with different licenses. The results indicate that both personal and commercial orders exhibit a similar trend with regard to the primary average effects, namely a reduction in price and an increase in volume and revenue. Furthermore, the results demonstrate that the magnitude of the estimators for personal orders is notably higher than that of commercial orders, suggesting that generative AI benefits the "tachie" market by increasing the demand for personal orders with lower prices.
Our findings indicate that, as a blue ocean strategy [21], generative AI can achieve its success by fulfilling the "low-end" needs of individual users, which were previously unmet due to the high cost. In contrast, the requisite quality for commercial orders exceeds the capabilities of current generative AI. For example, there is a notable disparity between the demo images produced by NovelAI (Figure 4 in Appendix) and successful commercial anime images (such as [18]) that feature considerably more intricate and detailed designs. Conversely, individual users may not require a very high level of quality for their orders. Generative AI can fulfill their demand directly or with a slight modification by human creators. Furthermore, generative AI may present potential copyright issues that limit its commercial applications. This is because the training of generative AI often involves the use of a substantial number of images collected from the Internet without copyright permission.
Panel A: All Completed Orders | Panel B: Incumbent Completed Orders | |||||
Dependent Variables: | log(price) | log(volume) | log(revenue) | log(price) | log(volume) | log(revenue) |
Model: | (1) | (2) | (3) | (4) | (5) | (6) |
Variables | ||||||
treated after | -0.4915∗∗∗ | 1.171∗∗∗ | 0.6790∗∗∗ | -0.5127∗∗∗ | 1.168∗∗∗ | 0.6556∗∗∗ |
() | () | () | () | () | () | |
Fixed-effects | ||||||
art_category | Yes | Yes | Yes | Yes | Yes | Yes |
year_week | Yes | Yes | Yes | Yes | Yes | Yes |
Fit statistics | ||||||
Observations | 126 | 126 | 126 | 126 | 126 | 126 |
R2 | 0.79706 | 0.89378 | 0.73652 | 0.77257 | 0.89462 | 0.73014 |
Within R2 | 0.21484 | 0.67848 | 0.19868 | 0.20654 | 0.67798 | 0.17595 |
Clustered (art_category) standard-errors in parentheses | ||||||
Signif. Codes: ***: 0.01, **: 0.05, *: 0.1 |
7 Supply Side Analysis
Our previous findings indicate that generative AI has the potential to benefit artists by increasing their order volume and revenue. However, it remains unclear whether incumbents or new entrants utilizing generative AI will reap most of the benefits. In this section, we answer research question RQ3, by examining the incumbents in the market.
We focus on the completed orders in order to observe which artists won the orders. We define incumbents as the group of artists who registered prior to the leak, i.e., before October 2022. We will examine the market equilibrium before and after the leak with the orders completed by incumbents.
We employ the standard difference-in-differences in Equation (1). Table 4 presents the estimation results. Panel A’s dataset comprises all completed orders, whereas Panel B’s dataset comprises only orders completed by incumbents. The results in Panel B are consistent with our main effects, indicating that incumbents benefit from generative AI by gaining more orders (116%) and more revenue (65%). Furthermore, the estimators in Panel B is close to those in Panel A, indicating that the majority of the orders were completed by incumbents, resulting in a similar sample for Panel A and B. According to our data, the incumbents won 97.41% of the orders and 97.96% of the revenue after the leak.
One potential explanation for this result is that the current generative AI is unable to fulfill orders without human artists. This is because the generation of content is an iterative process between consumers and artists, with artists required to make ongoing modifications in response to consumer feedback. The current generative AI, which outputs images, audio, and videos, lacks the capacity to make slight modifications independently due to the limitations of its underlying algorithms.
Our results rule out the possibility that new entrants using generative AI have taken over the market share of genuine human creators. In contrast, generative AI serves to expand the market itself, with the incumbent creators deriving benefits from such expansion. The findings provide empirical evidence of a favorable economic consequence of generative AI for artists and suggest that they should embrace generative AI rather than resist it. The incorporation of generative AI into one’s workflow has the potential to increase productivity and leverage the novelty and enhanced capabilities provided by generative AI to attract new customers who previously were not engaging with the art market, thereby "growing the pie."
8 Conclusions
This paper examines the impact of the advent of generative AI on market equilibrium, in terms of price, volume, and revenue. We identify an unanticipated and sudden leak of an advanced image-generative AI as a natural experiment, which facilitates difference-in-differences comparisons for causal inference. The results indicate that generative AI substantially increases order volume and overall revenue with a corresponding decrease in average price. The increase in volume and revenue is primarily driven by the rising demand for personal orders with lower prices, rather than expensive commercial orders. Moreover, the incumbent creators maintained the majority of the market share, reaping most of the benefits from generative AI.
This paper makes a significant scholarly contribution to the growing literature on the economics of AI, by providing one of the first empirical evidence on how generative AI affects the platform economy through diverse perspectives. Moreover, the findings also offer valuable practical insights. Creators should embrace generative AI rather than resist it to gain greater benefits from enhanced productivity. Online platforms can conduct marketing campaigns and improve services specifically for "low-end" personal orders, the emerging demand driven by generative AI. Policymakers may also implement supportive policies for generative AI to create more jobs and tax revenue.
It is our contention that generative AI is having and will continue to have a profound impact on our world. In the context of the marketplace, future research can explore how generative AI affects product quality, consumer satisfaction, and beyond through our identified natural experiment.
Acknowledgments. We would like to thank Reviewer HvRd, QbGb, and Area Chair Y8tc for their constructive feedback. This work was supported by the National Natural Science Foundation of China (Grant No.92370204), National Key R&D Program of China (Grant No.2023YFF0725001), Guangzhou-HKUST(GZ) Joint Funding Program (Grant No.2023A03J0008), Education Bureau of Guangzhou Municipality.
References
- [1] Acemoglu, D., Autor, D.: Skills, tasks and technologies: Implications for employment and earnings. In: Handbook of labor economics, vol. 4, pp. 1043–1171. Elsevier (2011)
- [2] Allam, S.: The impact of artificial intelligence on innovation-an exploratory analysis. Sudhir Allam," The Impact of Artificial Intelligence on Innovation-An Exploratory Analysis", International Journal of Creative Research Thoughts (IJCRT), ISSN pp. 2320–2882 (2016)
- [3] Bahdanau, D., Cho, K., Bengio, Y.: Neural machine translation by jointly learning to align and translate. arXiv preprint arXiv:1409.0473 (2014)
- [4] Brown, T., Mann, B., Ryder, N., Subbiah, M., Kaplan, J.D., Dhariwal, P., Neelakantan, A., Shyam, P., Sastry, G., Askell, A., et al.: Language models are few-shot learners. Advances in neural information processing systems 33, 1877–1901 (2020)
- [5] Brynjolfsson, E., Hui, X., Liu, M.: Does machine translation affect international trade? evidence from a large digital platform. Management Science 65(12), 5449–5460 (2019)
- [6] Brynjolfsson, E., Mitchell, T., Rock, D.: What can machines learn and what does it mean for occupations and the economy? In: AEA papers and proceedings. vol. 108, pp. 43–47. American Economic Association 2014 Broadway, Suite 305, Nashville, TN 37203 (2018)
- [7] Brynjolfsson, E., Rock, D., Syverson, C.: Artificial intelligence and the modern productivity paradox. The economics of artificial intelligence: An agenda 23, 23–57 (2019)
- [8] Callaway, B., Sant’Anna, P.H.: Difference-in-differences with multiple time periods. Journal of econometrics 225(2), 200–230 (2021)
- [9] Fernández, J.D., Vico, F.: Ai methods in algorithmic composition: A comprehensive survey. Journal of Artificial Intelligence Research 48, 513–582 (2013)
- [10] Frank, M.R., Autor, D., Bessen, J.E., Brynjolfsson, E., Cebrian, M., Deming, D.J., Feldman, M., Groh, M., Lobo, J., Moro, E., et al.: Toward understanding the impact of artificial intelligence on labor. Proceedings of the National Academy of Sciences 116(14), 6531–6539 (2019)
- [11] Gatt, A., Krahmer, E.: Survey of the state of the art in natural language generation: Core tasks, applications and evaluation. Journal of Artificial Intelligence Research 61, 65–170 (2018)
- [12] Gatys, L.A., Ecker, A.S., Bethge, M.: Image style transfer using convolutional neural networks. In: Proceedings of the IEEE conference on computer vision and pattern recognition. pp. 2414–2423 (2016)
- [13] Goldfarb, A., Trefler, D., et al.: Artificial intelligence and international trade. The economics of artificial intelligence: an agenda pp. 463–492 (2019)
- [14] Goodfellow, I., Pouget-Abadie, J., Mirza, M., Xu, B., Warde-Farley, D., Ozair, S., Courville, A., Bengio, Y.: Generative adversarial nets. Advances in neural information processing systems 27 (2014)
- [15] Goodman-Bacon, A.: Difference-in-differences with variation in treatment timing. Journal of Econometrics 225(2), 254–277 (2021)
- [16] Ho, J., Jain, A., Abbeel, P.: Denoising diffusion probabilistic models. Advances in neural information processing systems 33, 6840–6851 (2020)
- [17] Horton, J.J., Tambe, P., Wharton, U.P.: The death of a technical skill. Unpublished Manuscript p. 299 (2019)
- [18] Hoyoverse: Genshin impact characters. https://genshin.hoyoverse.com/en/character/liyue?char=12 (2024)
- [19] Isola, P., Zhu, J.Y., Zhou, T., Efros, A.A.: Image-to-image translation with conditional adversarial networks. In: Proceedings of the IEEE conference on computer vision and pattern recognition. pp. 1125–1134 (2017)
- [20] Jonas, J.: No to ai-generated images. https://twitter.com/JoJoesArt/status/1603071104528023553 (2022)
- [21] Kim, W.C., Mauborgne, R.A.: Blue ocean strategy, expanded edition: How to create uncontested market space and make the competition irrelevant. Harvard business review Press (2014)
- [22] Kingma, D.P., Welling, M.: Auto-encoding variational bayes. arXiv preprint arXiv:1312.6114 (2013)
- [23] Luo, X., Qin, M.S., Fang, Z., Qu, Z.: Artificial intelligence coaches for sales agents: Caveats and solutions. Journal of Marketing 85(2), 14–32 (2021)
- [24] McIntyre, D.P., Srinivasan, A.: Networks, platforms, and strategy: Emerging views and next steps. Strategic management journal 38(1), 141–160 (2017)
- [25] MODEL, A.: System identification and. In: Instrumentation, Control and Automation of Water and Wastewater Treatment and Transport Systems: Proceedings of the 5th IAWPRC Workshop Held in Yokohama and Kyoto, Japan, 26 July–3 August 1990. p. 121. Elsevier (2013)
- [26] Mohammadi, S.H., Kain, A.: An overview of voice conversion systems. Speech Communication 88, 65–82 (2017)
- [27] NovelAI: Image generation announcement. https://blog.novelai.net/image-generation-announcement-807b3cf0afec (2022)
- [28] Noy, S., Zhang, W.: Experimental evidence on the productivity effects of generative artificial intelligence. Science 381(6654), 187–192 (2023)
- [29] Peng, S., Kalliamvakou, E., Cihon, P., Demirer, M.: The impact of ai on developer productivity: Evidence from github copilot. arXiv preprint arXiv:2302.06590 (2023)
- [30] Popenici, S.A., Kerr, S.: Exploring the impact of artificial intelligence on teaching and learning in higher education. Research and Practice in Technology Enhanced Learning 12(1), 1–13 (2017)
- [31] Ramesh, A., Pavlov, M., Goh, G., Gray, S., Voss, C., Radford, A., Chen, M., Sutskever, I.: Zero-shot text-to-image generation. In: International Conference on Machine Learning. pp. 8821–8831. PMLR (2021)
- [32] Ren, Y., Ruan, Y., Tan, X., Qin, T., Zhao, S., Zhao, Z., Liu, T.Y.: Fastspeech: Fast, robust and controllable text to speech. Advances in neural information processing systems 32 (2019)
- [33] Rezende, D., Mohamed, S.: Variational inference with normalizing flows. In: International conference on machine learning. pp. 1530–1538. PMLR (2015)
- [34] Rombach, R., Blattmann, A., Lorenz, D., Esser, P., Ommer, B.: High-resolution image synthesis with latent diffusion models. In: Proceedings of the IEEE/CVF conference on computer vision and pattern recognition. pp. 10684–10695 (2022)
- [35] Rubin, D.B.: Causal inference using potential outcomes: Design, modeling, decisions. Journal of the American Statistical Association 100(469), 322–331 (2005)
- [36] Snow, J.: On the mode of communication of cholera. Edinburgh medical journal 1(7), 668 (1856)
- [37] Sun, C., Shi, Z.J., Liu, X., Ghose, A., Li, X., Xiong, F.: The effect of voice ai on consumer purchase and search behavior. NYU Stern School of Business (2019)
- [38] Svyatkovskiy, A., Deng, S.K., Fu, S., Sundaresan, N.: Intellicode compose: Code generation using transformer. In: Proceedings of the 28th ACM Joint Meeting on European Software Engineering Conference and Symposium on the Foundations of Software Engineering. pp. 1433–1443 (2020)
- [39] Tambe, P., Cappelli, P., Yakubovich, V.: Artificial intelligence in human resources management: Challenges and a path forward. California Management Review 61(4), 15–42 (2019)
- [40] Uzzi, B.: The sources and consequences of embeddedness for the economic performance of organizations: The network effect. American sociological review pp. 674–698 (1996)
- [41] Vaswani, A., Shazeer, N., Parmar, N., Uszkoreit, J., Jones, L., Gomez, A.N., Kaiser, Ł., Polosukhin, I.: Attention is all you need. Advances in neural information processing systems 30 (2017)
- [42] Webb, M.: The impact of artificial intelligence on the labor market. Available at SSRN 3482150 (2019)
- [43] Wessel, M., Adam, M., Benlian, A., Thies, F.: Generative ai and its transformative value for digital platforms
- [44] Wolff, J., Pauling, J., Keck, A., Baumbach, J.: The economic impact of artificial intelligence in health care: systematic review. Journal of medical Internet research 22(2), e16866 (2020)
- [45] Yilmaz, E.D., Naumovska, I., Aggarwal, V.A.: Ai-driven labor substitution: Evidence from google translate and chatgpt (2023)
Appendix 0.A Appendix
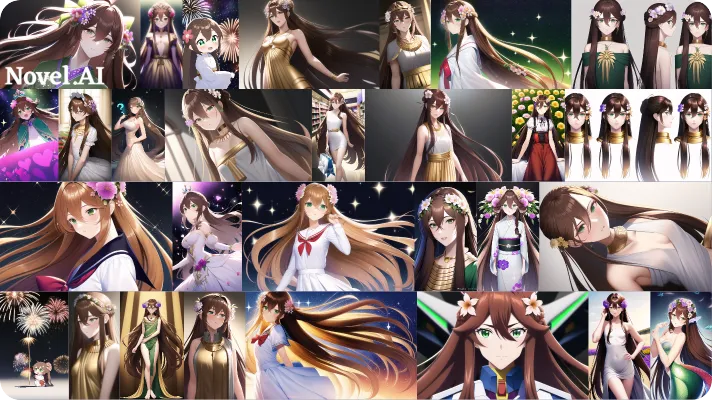