The doubly asymmetric simple exclusion process, the colored Boolean process, and the restricted random growth model
Abstract.
The multispecies asymmetric simple exclusion process (mASEP) is a Markov chain in which particles of different species hop along a one-dimensional lattice. This paper studies the doubly asymmetric simple exclusion process in which particles with species hop along a circular lattice with n sites, but also the particles are allowed to spontaneously change from one species to another. In this paper, we introduce two related Markov chains called the colored Boolean process and the restricted random growth model, and we show that the DASEP lumps to the colored Boolean process, and the colored Boolean process lumps to the restricted random growth model. This allows us to generalize a theorem of David Ash on the relations between sums of steady state probabilities. We also give explicit formulas for the stationary distribution of .
Key words and phrases:
Asymmetric exclusion process, Markov chain1991 Mathematics Subject Classification:
05E99, 60J101. Introduction
The asymmetric simple exclusion process (ASEP) is a Markov chain for particles hopping on a one-dimensional lattice such that each site contains at most one particle. The ASEP was introduced independently in biology by Macdonald-Gibbs-Pipkin [13], and in mathematics by Spitzer [20]. There are many versions of the ASEP: the lattice can have open boundaries, or be a ring, not necessarily finite (see Liggett [11],[12]). Particles can have different species, and this variation is called the multispecies ASEP (mASEP). The asymmetry can be partial, so that particles are allowed to hop both left and right, but one side is times more probable, and this is called the partially asymmetric exclusion process (PASEP). The ASEP is closely related to a growth model defined by Kardar-Parizi-Zhang [9], and various methods have been invented to study the ASEP, such as the matrix ansatz introduced by Derrida et al. in [6]. The combinatorics of the ASEP was studied by many people, see [7][3][4][14][5].
A partition is a weakly decreasing sequence of nonnegative integers . We denote the sum of all parts by . We will write a partition as an -tuple . Let be the number of parts of that equal . As in [21, Section 7.2], we also denote a partition by . Let denote the number of nonzero (positive) parts of , or the length of . We have . We write as the set of all permutations of . The mASEP can be thought of a Markov chain on .
Let be the number of positions on the lattice, be number of types of species, and be the number of particles. David Ash [2] defined the doubly asymmetric simple exclusion process . The DASEP is a variant of the mASEP but also allows particles to change from one species to another. If , is the usual 1-species PASEP on a ring.
Definition 1.1.
[2] For all positive integers with , the doubly asymmetric simple exclusion process is a Markov process on the following set
Let be constants. The transition probability on two permutations and is as follows:
-
•
If and with , then if and if .
-
•
If and with , then if and if .
-
•
If and with , then .
-
•
If and with , then .
-
•
If none of the above conditions apply but then . If then .
As we are interested in the stationary distribution of DASEP, we denote the un-normalised stationary distribution by , which is uniquely defined if we require the to be polynomials with greatest common divisor equal to 1.
Remark 1.2.
There is an inherent cyclic symmetry in the definition of DASEP.
Our first main result is about the ratio between the sums of certain groups of . For each partition and each binary word , define
as the set of all permutations of whose zeros are aligned with the binary word . Then if and , we have and .
Example 1.3.
For the partition , we have . For , we have . For , we have .
Theorem 1.4.
Consider for any positive integers with .
-
(1)
For any two binary words , we have .
-
(2)
For any binary word and partition such that
we have
In other words, the sum of steady state probabilities of states within one equivalence class is proportional to each other and the ratio only depends on the sum of all parts and the multiplicities in the partition; all steady state probabilities with respect to binary words are equal.
011 | |
---|---|
012 | |
021 | |
022 |
0011 | |
---|---|
0101 | |
0022 | |
0202 | |
0012 | |
0102 | |
0021 |
Example 1.6.
To prove 1.4, we also introduce a new Markov chain that we call the colored Boolean process (see 2.1), and we show that the DASEP lumps to the colored Boolean process. This gives a relationship between the stationary distribution of colored Boolean process and the DASEP, see 2.3.
Corollary 1.7.
For the defined by positive integers , and two partitions with , we have
In 4.1, we also give explicit formulas for the stationary distributions of the infinite family for any . The formulas depend on whether is odd or even. Both are described by polynomial sequences given by a second-order homogeneous recurrence relation (see 4.1).
When we specialize to , the polynomial sequences specialize to the trinomial transform of Lucas number A082762 or the binomial transform of the denominators of continued fraction convergents to A084326 [19].
The structure of this paper is as follows. In Section 2, we define the colored Boolean process, and we show that the DASEP lumps to the colored Boolean process. In Section 3, we define the restricted random growth model, which is a Markov chain on Young diagrams, and we show that the colored Boolean process lumps to the restricted random growth model. In Section 4, we give explicit formulas for the stationary distributions of the infinite family .
Acknowledgements
We thank Lauren Williams for suggesting the problem and helping me better understand ASEP.
2. The DASEP lumps to the colored Boolean process
In this section, we define the colored Boolean process, and we show that the DASEP lumps to the colored Boolean process. We compute the ratios between steady states probabilities in the colored Boolean process, leading us to prove 1.4.
Definition 2.1.
The colored Boolean process is a Markov chain dependent on three positive integers with on states space
with the following transition probabilities:
-
•
if contains parts equal to and is obtained from by changing a part equal to to a part equal to .
-
•
if contains parts equal to and is obtained from by changing a part equal to to a part equal to .
-
•
if is obtained from by at a unique position (allowing wrap-around at the end).
-
•
if is obtained from by at a unique position (allowing wrap-around at the end).
-
•
If none of the above conditions apply but or , then .
-
•
We denote the stationary distribution of by .
We think of parts of different sizes as particles of different colors, or species, hence the name.
The relation between the colored Boolean process and the DASEP is captured by the following notion.
Definition 2.2.
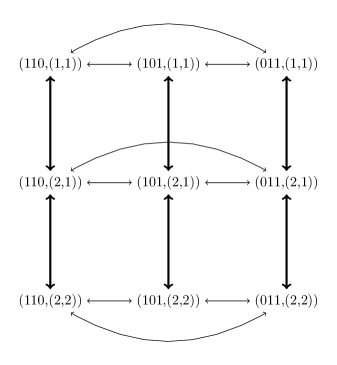
Theorem 2.3.
The projection map sending each to is a lumping of onto the colored Boolean process .
Proof.
Fix and , we want to show that for any , the quantity
is independent of the choice of and equal to . We may assume , because the probabilities add up to 1.
This quantity is nonzero only in the following cases:
-
•
and if there exists a unique with such that . We upgrade the species of a particle from to , and there are again ways to do it, where each transition probability , so the quantity is equal to .
-
•
and if there exists a unique with such that . We downgrade the species of a particle from to , and there are ways to do it, so there are number of ’s in with nonzero , so the quantity is equal to .
-
•
and is obtained from by at a unique position (allowing wrap-around at the end). This quantity is equal to .
-
•
and is obtained from by at a unique position (allowing wrap-around at the end). This quantity is equal to .
The nonzero transition probabilities of in each of the four cases above is the same as we defined. ∎
We may use the stationary distribution of to compute that of its lumping.
Proposition 2.4.
[10, Section 6.3] Suppose is a stationary distribution for , and let be the measure on defined by . Then is a stationary distribution for .
Thus, we may use the stationary distribution of to study that of . We have the following corollary due to 2.4 and 2.3.
Corollary 2.5.
The unnormalized steady state probabilities of the colored Boolean process and the unnormalized steady state probabilities of the DASEP are related as follows:
Theorem 2.6.
Consider the colored Boolean process .
-
(1)
The steady state probabilities of all binary words are equal, i.e.,
for any .
-
(2)
The steady state probability of an arbitrary state can be expressed in terms of the steady state probability (of the corresponding state with the trivial partition ) as follows:
(1)
Proof.
The colored Boolean process is again an ergodic markov chain, so we only need to show that the above relations satisfy the balance equations given by the transition matrix of .
Let us first check it for . For any binary word , denote by . Let be the number of blocks of consecutive ’s in (allowing wrap-around). Notice that any occurrence of in must begin a block, and any occurrence of must signify the end of a block. We have
(2) |
Expanding the multinomial coefficient, we are left with
which will be satisfied if we set all ’s to be equal.
For a generic , Equation 2 is modified on the left hand side such that becomes where and . On the right hand side pf Equation 2, we change the first term to
Expand this using Equation 1, the multinomial coefficients gives the ratios
for all . Then we have a term by term equality for each where corresponds to the first summation and corresponds to the second. ∎
3. The colored Boolean process lumps to the restricted random growth model
In this section, we define the restricted random growth model, which is a Markov chain on the set of Young diagrams fitting inside a rectangle, and we show it to be a lumping of the colored Boolean process.
For partitions and , we write if there exists a unique such that and for all we have . We write if there exists a unique such that and for all we have . In both cases, we have where is the number of parts of equal to .
Example 3.1.
and .
Definition 3.2.
Define the restricted random growth model on the the state space which includes all partitions that fit inside a rectangle but do not fit inside a shorter rectangle, with transition probabilities as follows:
-
•
If , then .
-
•
If , then .
-
•
In all other cases where , .
-
•
.
Recall that we also denote the number of parts of the partition that equal to by . We denote the unnormalized steady state probabilities of the restricted random growth model by .
Remark 3.3.
In other words, the transitions randomly add or remove a box from the right of a random chosen row of the Young diagram of the partition (conditioned on rightly fitting in the rectangle) as shown on the right hand side of Figure 3, then rearrange the parts in weakly decreasing order as shown on the left hand side of Figure 3.
The 1d random growth model [8] is a growth model on diagrams not necessarily arranged in weakly decreasing order. The diagrams do not need to fit in a rectangle, and the times between arrivals are independent i.i.d..
Theorem 3.4.
The projection map on state spaces sending to (forgetting the positions of 0’s) is a lumping of the colored Boolean process to the restricted random growth .
Proof.
By 2.2, we need to show that for any and binary word the following equation holds:
It suffices to check this for all . Then only if by 2.1, and this quantity is either when or when . ∎
Corollary 3.5.
The steady state probabilities of the restricted random growth and the unnormalized steady state probabilities of the colored Boolean process are related as follows:
Theorem 3.6.
The steady state probabilities of the restricted random growth model satisfy the following relations for all partitions :
4. The stationary distribution of DASEP(n,2,2)
If there were only one species of particle, i.e. , the stationary distribution of is uniform. If there were only one particle, i.e., , then the unnormalized steady state probabilities of are given by powers of , not involving due to cyclic symmetry. We now study the first nontrivial case of in more detail, namely . In this section, we give a complete description of the stationary distributions when there are two particles and two species, while the number of sites can be arbitrary.
Theorem 4.1.
Let and be polynomial sequences in satisfying the recurrence relation
(3) | ||||
(4) |
with initial conditions .
We can fully describe the unnormalized steady state probabilities of the infinite family as follows. When is odd,
When is even,
Proof.
We will denote by for simplicity in the proof. We need to show that these formulae satisfy balance equation at each state. Let for any binary word be , then for would be equal to by 2.6. Let be and be for or . The following equations hold.
(5) | ||||
(6) | ||||
(7) | ||||
(8) |
When is odd, Equation 7 is true for , and we have an additional equation
(9) |
When is even, Equation 7 is true for , and we have an additional equation
(10) |
By 1.4, we have for all . Applying this to Equation 5, Equation 8, and Equation 10, we see that all of them hold trivially.
To show Equation 6, we may assume is odd, since the even case would be the same. Deduce from both sides then divide both sides by , we are left with
which is the same as
Since , this is true by Equation 3.
To show Equation 7, we rewrite the recurrence relations Equation 3 and Equation 4 into
eliminating out of the equations, hence proving Equation 7.
To show Equation 9, we deduce from both sides then divide both sides by so that we are left with
which is true since . ∎
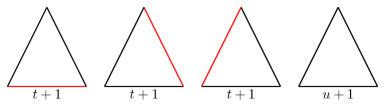

Remark 4.2.
A matching in a graph is a set of pairwise non-adjacent edges. Consider matchings in the cycle graph or line graph with vertices. Assign each matching a weight of . Then is the sum of weights over all matchings of the cycle , i.e.,
(11) |
and is that of the line , i.e.
(12) |
These can be seen via induction. The base cases can be seen in Figure 4, and Figure 5 which equal to the first rows of Table 1.
Proof.
We prove that the right hand side of Equation 11 satisfies the recurrence relation Equation 3 and the right hand side of Equation 12 satisfies Equation 4.
For the line graph , we label the edges by from left to right. Take a matching in the first edges (or, in the subgraph), then are both matchings in . However, is only a matching if , and if , then is a matching in (or, on the subgraph) because implies that . Therefore, counts for all matchings in , and counts the those set of edges given by that are not matchings.
For the cycle graph , we label the edges by . The argument is very similar, but we have to subtract another copy of which comes from the possible non-matchings given by . However, if we take a matching on , then is a matching in , which is counted by and added back. ∎
5. Homomesy
1.4 can be viewed as a statement about taking average of some statistic over the orbit of a group acting on the particles, which is an instance of a phenomenon called homomesy by Propp and Roby [17]. This phenomenon was first noticed by Panyushev [16] in 2007 in the context of the rowmotion action on the set of antichains of a root poset; Armstrong, Stump, and Thomas [1] proved Panyushev’s conjecture in 2011.
Definition 5.1 ([17]).
Given a set , an invertible map such that each -orbit is finite, and a function (or “statistic”) taking values in some field of characteristic zero, we say the triple exhibits homomesy if there exists a constant such that for every -orbit
In this situation we say that is homomesic under the action of on , or more specifically -mesic.
Although the original definition concerns the action of the cyclic group generated by a single map , this can be generalized to the action of any finite group, as pointed out in [18, Section 2.1]. We can also generalize the definition such that the statistic takes value in a ring of polynomials (or even Laurent polynomials) over some field of characteristic zero, and the average of this statistic is equal up to a monic monomial. We make the generalized definition more precise as follows.
Definition 5.2.
Given a set , a finite group acting on with finite orbits, and a function for a field of characteristic zero, we say the triple exhibits homomesy if there exists a polynomial such that for every -orbit , there exist nonnegative integers for such that
Corollary 5.3.
Let the symmetric group acts on the states of by permuting the positive parts (the particles). Then the triple exhibits homomesy with statistic in the more general sense defined above.
Proof.
Corollary 5.4.
Let the symmetric group acts on the states of by permuting the sites. Then the triple exhibits homomesy with statistic .
References
- [1] Drew Armstrong, Christian Stump and Hugh Thomas “A uniform bijection between nonnesting and noncrossing partitions” In Trans. Amer. Math. Soc. 365.8, 2013, pp. 4121–4151 DOI: 10.1090/S0002-9947-2013-05729-7
- [2] David W. Ash “Introducing DASEP: the doubly asymmetric simple exclusion process”, 2023 arXiv:2201.00040 [math.CO]
- [3] R. A. Blythe, W. Janke, D. A. Johnston and R. Kenna “The grand-canonical asymmetric exclusion process and the one-transit walk” In J. Stat. Mech. Theory Exp., 2004, pp. 001, 10
- [4] R. Brak and J. W. Essam “Simple asymmetric exclusion model and lattice paths: bijections and involutions” In J. Phys. A 45.49, 2012, pp. 494007, 22 DOI: 10.1088/1751-8113/45/49/494007
- [5] Sylvie Corteel, Olya Mandelshtam and Lauren Williams “From multiline queues to Macdonald polynomials via the exclusion process” In Amer. J. Math. 144.2, 2022, pp. 395–436 DOI: 10.1353/ajm.2022.0007
- [6] B Derrida, M R Evans, V Hakim and V Pasquier “Exact solution of a 1D asymmetric exclusion model using a matrix formulation” In Journal of Physics A: Mathematical and General 26.7, 1993, pp. 1493 DOI: 10.1088/0305-4470/26/7/011
- [7] Enrica Duchi and Gilles Schaeffer “A combinatorial approach to jumping particles: the parallel TASEP” In Random Structures Algorithms 33.4, 2008, pp. 434–451 DOI: 10.1002/rsa.20229
- [8] P. L. Ferrari and H. Spohn “Random growth models” In The Oxford handbook of random matrix theory Oxford Univ. Press, Oxford, 2011, pp. 782–801
- [9] Mehran Kardar, Giorgio Parisi and Yi-Cheng Zhang “Dynamic Scaling of Growing Interfaces” In Phys. Rev. Lett. 56 American Physical Society, 1986, pp. 889–892 DOI: 10.1103/PhysRevLett.56.889
- [10] John G. Kemeny and J. Laurie Snell “Finite Markov chains” Reprinting of the 1960 original, Undergraduate Texts in Mathematics Springer-Verlag, New York-Heidelberg, 1976, pp. ix+210
- [11] Thomas M. Liggett “Ergodic theorems for the asymmetric simple exclusion process” In Trans. Amer. Math. Soc. 213, 1975, pp. 237–261 DOI: 10.2307/1998046
- [12] Thomas M. Liggett “Interacting particle systems” 276, Grundlehren der mathematischen Wissenschaften [Fundamental Principles of Mathematical Sciences] Springer-Verlag, New York, 1985, pp. xv+488 DOI: 10.1007/978-1-4613-8542-4
- [13] Carolyn T. MacDonald, Julian H. Gibbs and Allen C. Pipkin “Kinetics of biopolymerization on nucleic acid templates” In Biopolymers 6.1, 1968, pp. 1–25 DOI: https://doi.org/10.1002/bip.1968.360060102
- [14] James B. Martin “Stationary distributions of the multi-type ASEP” In Electron. J. Probab. 25, 2020, pp. Paper No. 43, 41 DOI: 10.1214/20-ejp421
- [15] C. Y. Amy Pang “Lumpings of algebraic Markov chains arise from subquotients” In J. Theoret. Probab. 32.4, 2019, pp. 1804–1844 DOI: 10.1007/s10959-018-0834-0
- [16] Dmitri I. Panyushev “On orbits of antichains of positive roots” In European J. Combin. 30.2, 2009, pp. 586–594 DOI: 10.1016/j.ejc.2008.03.009
- [17] James Propp and Tom Roby “Homomesy in products of two chains” In Electron. J. Combin. 22.3, 2015, pp. Paper 3.4, 29 DOI: 10.37236/3579
- [18] Tom Roby “Dynamical algebraic combinatorics and the homomesy phenomenon” In Recent trends in combinatorics 159, IMA Vol. Math. Appl. Springer, [Cham], 2016, pp. 619–652 DOI: 10.1007/978-3-319-24298-9“˙25
- [19] Neil J. A. Sloane and The OEIS Foundation Inc. “The on-line encyclopedia of integer sequences”, 2020 URL: http://oeis.org/?language=english
- [20] Frank Spitzer “Interaction of Markov processes” In Advances in Math. 5, 1970, pp. 246–290 DOI: 10.1016/0001-8708(70)90034-4
- [21] Richard P. Stanley “Enumerative combinatorics. Vol. 2” With an appendix by Sergey Fomin 208, Cambridge Studies in Advanced Mathematics Cambridge University Press, Cambridge, [2024] ©2024, pp. xvi+783