TF-Attack: Transferable and Fast Adversarial Attacks
on Large Language Models
Abstract
With the great advancements in large language models (LLMs), adversarial attacks against LLMs have recently attracted increasing attention. We found that pre-existing adversarial attack methodologies exhibit limited transferability and are notably inefficient, particularly when applied to LLMs. In this paper, we analyze the core mechanisms of previous predominant adversarial attack methods, revealing that 1) the distributions of importance score differ markedly among victim models, restricting the transferability; 2) the sequential attack processes induces substantial time overheads. Based on the above two insights, we introduce a new scheme, named TF-Attack, for Transferable and Fast adversarial attacks on LLMs. TF-Attack employs an external LLM as a third-party overseer rather than the victim model to identify critical units within sentences. Moreover, TF-Attack introduces the concept of Importance Level, which allows for parallel substitutions of attacks. We conduct extensive experiments on 6 widely adopted benchmarks, evaluating the proposed method through both automatic and human metrics. Results show that our method consistently surpasses previous methods in transferability and delivers significant speed improvements, up to 20 faster than earlier attack strategies.
TF-Attack: Transferable and Fast Adversarial Attacks
on Large Language Models
Zelin Li, Kehai Chen, Lemao Liu, Xuefeng Bai, Mingming Yang, Yang Xiang and Min Zhang School of Computer Science and Technology, Harbin Institute of Technology, Shenzhen, China Pengcheng Laboratory, Shenzhen, China [email protected], {chenkehai,baixuefeng}@hit.edu.cn, {lemaoliu,shanemmyang}@gmail.com, [email protected], [email protected]
1 Introduction
Recently, large language models (LLMs) such as ChatGPT and LLaMA (Ouyang et al., 2022; Touvron et al., 2023) have demonstrated considerable promise across a range of downstream tasks (Kasneci et al., 2023; Thirunavukarasu et al., 2023; Liu et al., 2023). Subsequently, there has been increasing attention on the task of adversarial attack Xu et al. (2023); Yao et al. (2023), which aims to generate adversarial examples that confuse or mislead LLMs. This task is crucial for advancing reliable and robust LLMs in the AI community, emphasizing the paramount importance of security in AI systems (Marcus, 2020; Thiebes et al., 2021).
CNN | LSTM | BERT | LLaMA | Baichuan | |
CNN | 94.7 | 22.9 | 19.8 | 21.7 | 21.2 |
LSTM | 17.3 | 94.1 | 19.4 | 22.0 | 22.1 |
BERT | 12.6 | 14.6 | 91.0 | 16.6 | 18.3 |
LLaMA | 12.1 | 9.5 | 8.7 | 86.1 | 16.0 |
Baichuan | 21.8 | 24.0 | 19.6 | 27.2 | 89.3 |
ChatGPT | 11.2 | 14.4 | 12.6 | 16.2 | 14.8 |
Average | 14.6 | 17.0 | 18.0 | 20.7 | 18.1 |
Existing predominant adversarial attack approaches on LLMs typically adhere to a two-step process: initially, they rank token importance based on the victim model, and subsequently, they replace these tokens sequentially following specific rules (Cer et al., 2018; Oliva et al., 2011; Jin et al., 2020). Despite notable successes, recent studies highlight that current methods face two substantial limitations: 1) poor transferability of the generated adversarial samples. As depicted in Table 1, while adversarial samples generated by models can drastically reduce their own classification accuracy, they scarcely affect other models; 2) significant time overhead, particularly with Large Language Models (Spector and Re, 2023). For instance, the time required to conduct an attack on LLaMA is 30 slower than standard inference.
To tackle the above two issues, we begin by analyzing the causes of the poor transferability and the slow speed of existing adversarial attack methods. Specifically, we first study the impact of the importance score, which is the core mechanism of previous methods. Comparative analysis reveals distinct importance score distributions across various victim models. This discrepancy largely explains why the portability of adversarial samples generated by existing methods is poor, since perturbations generated according to a specific pattern of one model do not generalize well to other models with different importance assignments. In addition, analysis of time consumption in Figure 3 shows that over 80 of processing time is spent on sequential word-by-word operations when attacking LLMs.
Drawing on insights from the above observations, we propose a new scheme, named TF-Attack, for transferable and fast adversarial attacks over LLMs. TF-Attack follows the overall framework of BERT-Attack which generates adversarial samples by synonym replacement. Different from BERT-Attack, TF-Attack employs an external third-party overseer such as ChatGPT to identify important units with the input sentence, thus eliminating the dependency on the victim model. Moreover, TF-Attack introduces the concept of Important Level, which divides the input units into different groups based on their semantic importance. Specifically, we utilize the powerful abstract semantic understanding and efficient automatic extraction capabilities of ChatGPT to form an important level priority queue through human-crafted Ensemble Prompts. Based on this, TF-Attack can perform parallel replacement of entire words within the same priority queue, as opposed to the traditional approach of replacing them sequentially one by one, as shown in Figure 4. This approach markedly reduces the time of the attacking process, thereby resulting in a significant speed improvement. Furthermore, we employ two tricks, named Multi-Disturb and Dynamic-Disturb, to enhance the attack effectiveness and transferability of generated adversarial samples. The former involves three levels of disturbances within the same sentence, while the latter dynamically adjusts the proportions and thresholds of the three types of disturbances based on the sentence length of the input. They significantly boost attack effectiveness and transferability, and are adaptable to other attack methods.
We conduct experiments on six widely adopted benchmarks and compare the proposed method with several state-of-the-art methods. To verify the effectiveness of our method, we consider both automatic and human evaluation. Automatic evaluation shows that TF-Attack has surpassed the baseline method TextFooler, BERT-Attack and SDM-Attack. In addition, human evaluation results demonstrate that TF-Attack maintains a comparable consistency and achieves a comparable level of language fluency that does not cause much confusion for humans. Moreover, we compare the transferability of TF-Attack with BERT-Attack, demonstrating that TF-Attack markedly diminishes the accuracy of other models and exhibits robust migration attack capabilities. Furthermore, the time cost of TF-Attack is significantly lower than BERT-Attack, more than 10 speedup on average stats. Lastly, the adversarial examples generated by TF-Attack minimally impact the performance of model after adversarial training, significantly strengthening its defense against adversarial attacks. Overall, the main contributions of this work can be summarized as follows:
-
•
We investigate the underlying causes behind the slow speed and poor effectiveness of pre-existed adversarial attacks on LLMs.
-
•
We introduce TF-Attack, a novel approach leveraging an external LLM to identify critical units and facilitates parallelized adversarial attacks.
-
•
TF-Attack effectively enhances the transferability of generated adversarial samples and achieves a significant speedup compared to previous methods.
2 Related Work
2.1 Text Adversarial Attack
For NLP tasks, the adversarial attacks occur at various text levels including the character, word, or sentence level. Character-level attacks involve altering text by changing letters, symbols, and numbers. Word-level attacks (Wei and Zou, 2019) involve modifying the vocabulary with synonyms, misspellings, or specific keywords. Sentence-level attacks Coulombe (2018); Xie et al. (2020) involve adding crafted sentences to disrupt the output of model. Current adversarial attacks in NLP(Alzantot et al., 2018; Jin et al., 2020) employ substitution to generate adversarial examples through diverse strategies, such as genetic algorithms (Zang et al., 2020; Guo et al., 2021), greedy search (Sato et al., 2018; Yoo and Qi, 2021), or gradient-based methods (Ebrahimi et al., 2018a; Cheng et al., 2018), are employed to identify substitution words form synonyms (Jin et al., 2020) or language models (Li et al., 2020; Garg and Ramakrishnan, 2020; Li et al., 2021). Recent studies have refined sampling methods, yet these approaches continue to be time-intensive, highlighting a persistent challenge in efficiency. (Fang et al., 2023) apply reinforcement learning, showing promise on small models but facing challenges on LLMs (Ji et al., 2024; Zhong et al., 2024; Jiang et al., 2024) due to lengthy iterations, limiting large-scale adversarial samples.
2.2 Sample Transferability
Evaluating text adversarial attacks heavily depends on sample transferability, assessing the performance of attack samples across diverse environments and models to measure their broad applicability and consistency. In experiments Qi et al. (2021), adversarial samples generated from the Victim Model are directly applied to other models, testing transferability. Strongly transferable attack samples can hit almost all models in a black-box manner, which traditional white-box attacks Ebrahimi et al. (2018b) can not match. Evaluation datasets like adversarial tasks of Adv-Glue Wang et al. (2021) showcase this transferability, aiding in robustness assessment. Relevant research Liu et al. (2016) endeavors to enhance the capability of adversarial example transferability by attacking ensemble models. It has been demonstrated Zheng et al. (2020) that adversarial examples with better transferability can more effectively enhance the robustness of models in adversarial training. However, in the field of textual adversarial attacks, there has been a lack of in-depth research dedicated to how to improve the transferability of adversarial examples.
2.3 Synchronization Work
Prompt-Attack (Xu et al., 2023) leverages the exceptional comprehension of LLMs and diverges from traditional adversarial methods. It employs a manual approach of constructing rule-based prompt inputs, requiring LLMs to output adversarial attack samples that can deceive itself and meet the modification rule conditions. This attack method achieved fully automatic and efficient generation of attack samples using the local model. However, the drawback is that the model may not perform the whole attacking process properly, resulting in mediocre attack effectiveness. Additionally, different prompts can significantly influence the quality of the model-generated attack samples. These generated attack samples, though somewhat transferable, fail to consider the model’s internal reasoning, resulting in excessively high modification rates. Our work shares similarities in that we both leverage the understanding capabilities of LLMs. However, we solely employ ChatGPT’s language abstraction for Importance Level suggestions, maintaining the attack process within traditional text adversarial methods. Furthermore, our work is more focused on enhancing the transferability of attack samples and speeding up the attack, making them more widely applicable. This differs from the motivation of Prompt-Attack, which aims at the automated generation of samples that can deceive the model itself using LLMs.
3 Method
3.1 Preliminaries: Adversarial Attack
The task of adversarial attack aims at generating perturbations on inputs that can mislead the output of model. These perturbations can be very small, and imperceptible to human senses.
As for NLP tasks, given a corpus of N input texts, , and an output space containing K labels, the language model F() learns a mapping , which learns to classify each input sample to the ground-truth label :
(1) |
The adversary of text can be formulated as = + , where is a perturbation to the input . The goal is to mislead the victim language model F() within a certain constraint :
(2) | ||||
where is the coefficient, and C(, x) is usually calculated by the semantic or syntactic similarity (Cer et al., 2018; Oliva et al., 2011) between the input and its corresponding adversary .
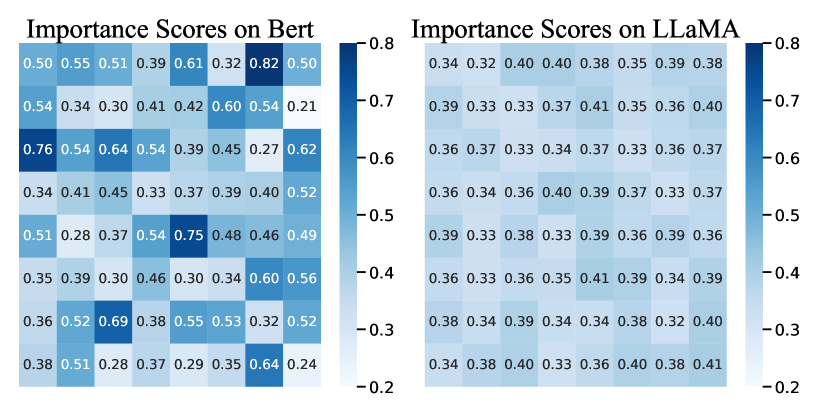
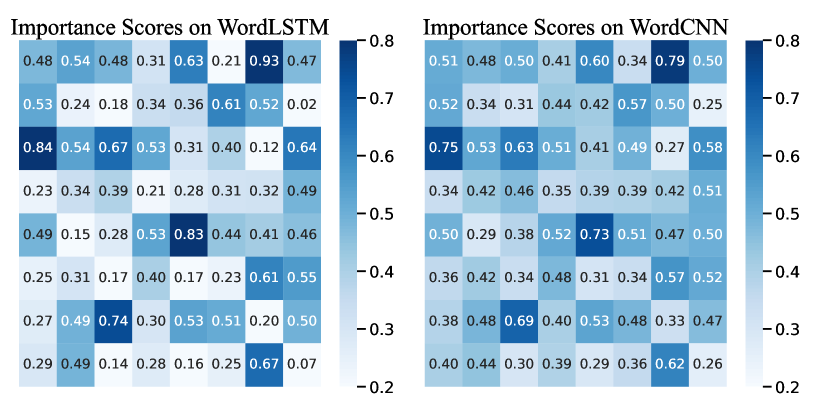
3.2 Limitations of Importance Score
Existing predominant adversarial attack systems Morris et al. (2020); Jin et al. (2020); Li et al. (2020) on LLMs typically adhere to a two-step process following BERT-Attack Li et al. (2020). We thus take BERT-Attack as a representative method for analysis. The core idea of BERT-Attack is to perform substitution according to Importance Score. BERT-Attack calculates the importance score by individually masking each word in the input text, performing a single inference for each, and using changes in the confidence score and label of Victim Models to determine the impact of each word. In BERT-Attack, importance score determine the subsequent attack sequence, which is crucial for the success of subsequent attacks and the times of attacks.
To explore the limited transferability of methods relying on importance scores, we analyze the importance score distribution among various models. As illustrated in Figure 1, there are substantial differences in the importance score derived from the same sentence when calculated using BERT and LLaMA. The former has a sharper distribution, while the latter essentially lacks substantial numerical differences. This phenomenon is consistent across multiple sentences. Figure 2 shows the different Importance Score distribution of the same sentence given by BERT-Attack on WordCNN and WordLSTM.
Given that importance score are crucial in the attack process of the aforementioned methods, variations in these scores can lead to entirely different adversarial samples. This variation explains the poor transferability of the generated attack samples, as demonstrated in Table 1.
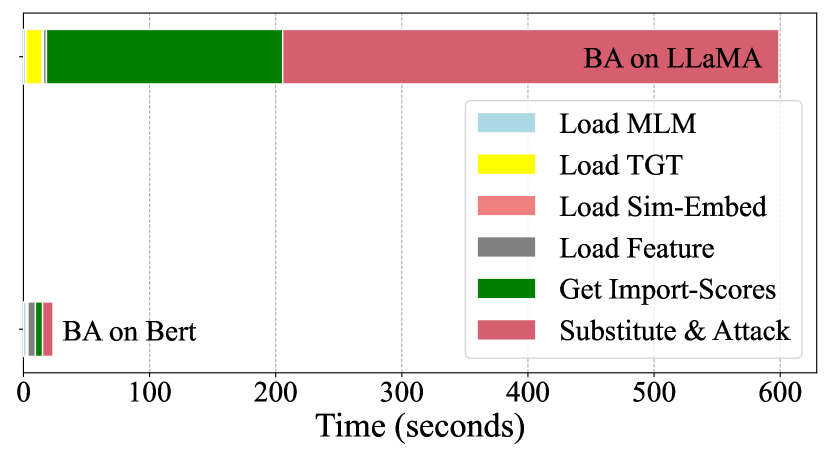
To investigate the causes of significant time overhead, we analyze the time consumption of various components involved when implementing a representative attacking method on both a small and a large model. As depicted in Figure 3, when applying BERT-Attack to attack small models like BERT, the average time spent per entry is very short, and the time consumption of various components is similar. However, the time cost per inference on LLaMA far exceeds that of BERT, disrupting this balance. It is evident that in LLMs, over 80 of the time spent per entry in the attack is consumed by the Get import-scores and substitute & Attack component. In addition, it is worth noting that this phenomenon is even more pronounced in successfully attacked samples. The underlying reason is that BERT-Attack necessitates performing the attack sequentially, according to the calculated importance score. This drawback becomes more pronounced when applied to large models, as both components require performing model inference, significantly increasing the time cost.
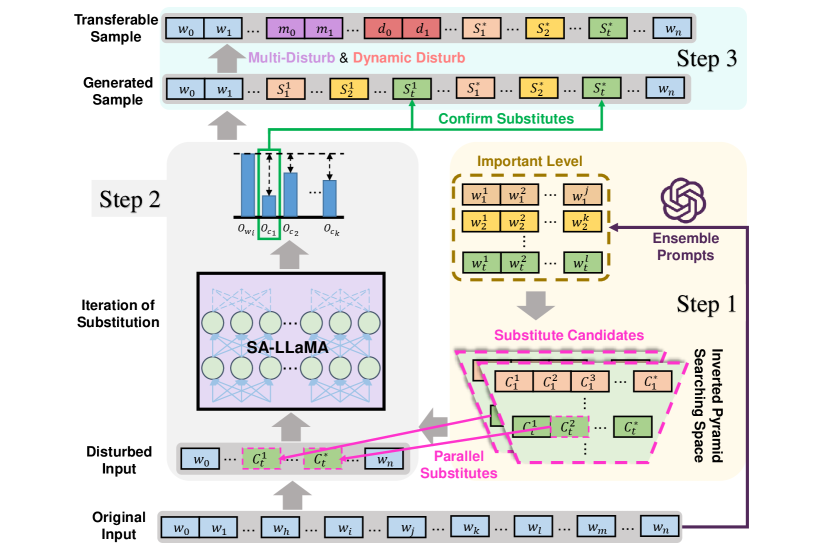
3.3 TF-Attack: Transferable and Fast Adversarial Attacks on LLMs
To address the above limitations caused by the importance score mechanism, we introduce TF-Attack as a solution for transferable and fast adversarial attacks. Firstly, as shown in Figure 4, TF-Attack employs an external model as a third-party overseer to identify important units. In this way, TF-Attack avoids excessive dependence on the victim model during the attack process. Specifically, we design several instructions in Table 11 for ChatGPT to partition all words in the original input according to their semantic importance. Another advantage of TF-Attack is its ability to utilize the rich semantic knowledge within ChatGPT, making the subsequent generation of attack sequences more universally semantic. In terms of speed, this approach does not require inference from the victim model, thus alleviating the problem of high inference costs for LLMs. TF-Attack only needs one inference to obtain a comprehensive Important Level priority queue, while the traditional approach requires inference times proportional to the length of the text.
Secondly, TF-Attack introduces the concept of Importance Level to facilitate parallel substitution. Specifically, TF-Attack takes the original sentence as input and outputs a priority queue with 5 levels, with a different number of words in each level. The concept of importance level assumes that words within the same level have no specific order, enabling parallel replacement of candidate words at the same level. This parallel replacement process markedly decreases both the search space and the number of required inferences, offering a substantial improvement over the previous approach that relied on greedy, sequential word replacements.
|
Abbre. | Perturbation Details | |
Character | C1 |
|
|
C2 | Change at most two letters in the sentence. | ||
C3 | Add at most two extraneous punctuation marks to the end of the sentence. | ||
Word | W1 | Replace at most two words in the sentence with synonyms. | |
W2 |
|
||
W3 | Add at most two semantically neutral words to the sentence. | ||
Sentence | S1 |
|
|
S2 | Change the syntactic structure and word order of the sentence. | ||
S3 | Paraphrase the sentence with ChatGPT. |
Additionally, we employ a reverse pyramid search space strategy for importance levels, optimizing the search space to reduce inefficient search expenditures. Words prioritized at higher levels are presumed to significantly influence sentence sentiment. Consequently, a larger search space is utilized to identify semantically similar words, with the aim of replacing them with synonyms that precipitate a notable decline in the performance of victim model. For words at lower priority levels, a smaller search space suffices, as alterations to these words minimally impact sentence sentiment. Excessive searching at these levels can lead to increased inference costs without substantially enhancing effectiveness.
3.4 Multi-Disturb & Dynamic-Disturb
Building on the aforementioned method, to further enhance the robustness of adversarial attack samples, we strategically propose two tricks for optimization. Following Xu et al. (2023), we use 9 ways of disturbance, including character-level, word-level, and sentence-level disturbances in Table 2. However, how to set the ratios of these three types of disturbances largely determines the quality of the transferability from generated attack samples. Therefore, the following strategy is proposed.
In the step of evaluating whether an attack sample is effective, traditional attack methods almost solely rely on the confidence of model output, a practice that undoubtedly promotes overfitting of attack samples to the model architecture. Therefore, in the process of determining the effectiveness of a replacement, we introduce random disturbance to the decrease in model confidence. This may result in the loss of some already successfully attacked samples, but it also prevents the occurrence of the phenomenon where the attack stops after succeeding on this particular Victim model. Traditional methods rely heavily on model confidence, leading to overfitting. To counter this, we introduce random disturbances during effectiveness assessment, reducing model confidence. This might sacrifice past successful attacks but prevents reliance on the success of the victim model.
These two strategies can be incorporated into traditional text adversarial attack methods as a post-processing step, significantly improving the transferability of adversarial samples. Specifically, the Multi-Disturb strategy refers to introducing a variety of disturbances within the same sentence. Table 2 outlines 9 ways of disturbance, including character-level, word-level, and sentence-level disturbances, which can greatly enhance the transferability of attack samples. Dynamic-Disturb refers to using an FFN+Softmax network to assess the length and structural distribution of the input sentence, outputting the ratios of these three types of disturbances.
In assessing attack sample effectiveness, traditional methods heavily depend on model output confidence, likely leading to overfitting to the model architecture. To rectify the problem, we incorporate random disturbance to diminish model confidence during replacement evaluation. Our experiments confirm that this two tricks can be adapted to almost all text adversarial attack methods, significantly enhancing transferability and increasing the ability of adversarial samples to confuse models through adaptive post-processing.
Dataset | Train | Test | Avg Len | Classes |
Yelp | 560k | 38k | 152 | 2 |
IMDB | 25k | 25k | 215 | 2 |
AG’s News | 120k | 7.6k | 73 | 4 |
MR | 9k | 1k | 20 | 2 |
SST-2 | 7k | 1k | 17 | 2 |
Dataset | Method | A-rate | Mod | Sim | Dataset | Method | A-rate | Mod | Sim |
Yelp | TextFooler | 78.9 | 9.1 | 0.73 | IMDB | TextFooler | 83.3 | 8.1 | 0.79 |
BERT-Attack | 80.5 | 11.5 | 0.69 | BERT-Attack | 84.2 | 9.6 | 0.78 | ||
SDM-Attack | 81.1 | 10.7 | 0.71 | SDM-Attack | 86.1 | 8.9 | 0.75 | ||
TF-Attack (Zero-Shot) | 81.3 | 10.3 | 0.71 | TF-Attack (Zero-Shot) | 86.1 | 8.7 | 0.81 | ||
TF-Attack (Few-Shot) | 83.7 | 9.0 | 0.77 | TF-Attack (Few-Shot) | 86.7 | 7.4 | 0.76 | ||
+MD | 84.6 | 12.3 | 0.71 | +MD | 87.1 | 10.7 | 0.77 | ||
+MD +DD | 84.5 | 11.9 | 0.72 | +MD +DD | 87.7 | 9.9 | 0.81 | ||
AG’s News | TextFooler | 73.2 | 16.1 | 0.54 | MR | TextFooler | 81.3 | 10.5 | 0.53 |
BERT-Attack | 76.6 | 17.3 | 0.59 | BERT-Attack | 82.8 | 10.2 | 0.51 | ||
SDM-Attack | 79.3 | 16.2 | 0.61 | SDM-Attack | 84.0 | 9.9 | 0.55 | ||
TF-Attack (Zero-Shot) | 77.1 | 18.3 | 0.52 | TF-Attack (Zero-Shot) | 84.6 | 8.9 | 0.58 | ||
TF-Attack (Few-Shot) | 81.9 | 16.1 | 0.58 | TF-Attack (Few-Shot) | 83.1 | 12.4 | 0.44 | ||
+MD | 82.8 | 19.4 | 0.53 | +MD | 83.0 | 13.2 | 0.40 | ||
+MD +DD | 83.0 | 19.1 | 0.55 | +MD +DD | 84.0 | 10.4 | 0.50 | ||
SNLI | TextFooler | 82.8 | 14.1 | 0.39 | MNLI | TextFooler | 77.1 | 11.5 | 0.51 |
BERT-Attack | 80.5 | 12.3 | 0.46 | BERT-Attack | 75.8 | 8.4 | 0.54 | ||
SDM-Attack | 83.1 | 14.6 | 0.42 | SDM-Attack | 79.3 | 10.2 | 0.55 | ||
TF-Attack (Zero-Shot) | 82.9 | 10.2 | 0.45 | TF-Attack (Zero-Shot) | 79.3 | 8.4 | 0.55 | ||
TF-Attack (Few-Shot) | 82.7 | 10.4 | 0.47 | TF-Attack (Few-Shot) | 78.2 | 7.9 | 0.57 | ||
+MD | 83.6 | 11.7 | 0.46 | +MD | 77.7 | 8.3 | 0.53 | ||
+MD +DD | 83.5 | 10.9 | 0.41 | +MD +DD | 79.4 | 8.5 | 0.52 |
4 Experiments
4.1 Experimental Setups
Tasks and Datasets
Following Li et al. (2020), we evaluate the effectiveness of the proposed TF-Attack on classification tasks upon diverse datasets covering news topics (AG’s News; Zhang et al., 2015), sentiment analysis at sentence (MR; Pang and Lee, 2005) and document levels (IMDB111https://datasets.imdbws.com/ and Yelp Polarity; Zhang et al., 2015). As for textual entailment, we use a dataset of sentence pairs (SNLI; Bowman et al., 2015) and a dataset with multi-genre (MultiNLI; Williams et al., 2018). Following (Jin et al., 2020; Alzantot et al., 2018), we attack 1k samples randomly selected from the test set of each task. The statistics of datasets and more details can be found in Table 3.
Baselines
We compare TF-Attack with recent studies: 1) TextFooler (Jin et al., 2020), which finds important words via probability-weighted word saliency and then applies substitution with counter-fitted word embeddings. 2) BERT-Attack (Li et al., 2020), which uses a mask-predict approach to generate adversaries. 3) SDM-Attack (Fang et al., 2023), which employs reinforcement learning to determine the attack sequence. We use the official codes BERT-Attack and TextAttack tools Morris et al. (2020) to perform attacks in our experiments. The TF-Attack (zero-shot) denotes that no demonstration examples were provided when generating the Important Level with ChatGPT. Conversely, the TF-Attack (few-shot) uses five demonstrations as context information. To ensure a fair comparison, we follow Morris et al. (2020) to apply constraints for TF-Attack.
Implementation Details
Following established training protocols, we fine-tuned a LLaMA-2-7B model to develop specialized Task-LLaMA models tailored for specific downstream tasks. Among them, the Task-LLaMA fine-tuned on the IMDB training set achieved an accuracy of 96.95% on the test set, surpassing XLNET with additional data, which achieved 96.21%. The model achieved 93.63% on another sentiment classification dataset, SST-2, indicating that Task-LLaMA is not overfitting to the training data but a strong baseline for experiments.
Automatic Evaluation Metrics
Following prior work (Jin et al., 2020; Morris et al., 2020), we assess the results with the following metrics: 1) attack success rate (A-rate): post-attack model performance decline; 2) Modification rate (Mod): percentage of altered words compared to the original; 3) Semantic similarity (Sim): cosine similarity between original and adversary texts via universal sentence encoder (USE; Cer et al., 2018); and 4) Transferability (Trans): the mean accuracy decreases across three models between adversarial and original samples.
Manual Evaluation Metrics
Following (Fang et al., 2023), We further manually validate the quality of the adversaries from three challenging properties. 1) Human prediction consistency (Con): how often human judgment aligns with the true label; 2) Language fluency (Flu): measured on a scale of 1 to 5 for sentence coherence Gagnon-Marchand et al. (2019); and 3) Semantic similarity (): gauging consistency between input-adversary pairs, with 1 indicating agreement, 0.5 ambiguity, and 0 inconsistency.
4.2 Overall Performance
Table 4 shows the performance of different systems on four benchmarks. As shown in Table 4, TF-Attack consistently achieves the highest attack success rate to attack LLaMA and has little negative impact on Mod and Sim. Additionally, TF-Attack mostly obtains the best performance of modification and similarity metrics, except for AG’s News, where TF-Attack achieves the second-best. In general, our method can simultaneously satisfy the high attack success rate with a lower modification rate and higher similarity. We additionally observe that TF-Attack achieves a better attack effect on the binary classification task. Empirically, when there exist more than two categories, the impact of each replacement word may be biased towards a different class, leading to an increase in the perturbation rate.
In Table 5, we evaluate the effectiveness of attack order. Utilizing a random attack sequence leads to a reduction in the success rate of attacks and a significant increase in the modification rate, as well as severe disruption of sentence similarity. This implies that each attack path is random, and a substantial amount of inference overhead is wasted on futile attempts. Although we adhere to the threshold constraints of the traditional adversarial text attack domain, the text can still be successfully attacked. However, under conditions of high modification rates and low similarity, the text has been altered significantly from its original semantics, contravening the purpose of the task. Furthermore, a random attack sequence incurs a substantial additional cost in terms of attack speed, resulting in a nearly doubled time delay.
Method | A-rate | Mod | Sim | Time Cost |
BA-IS | 84.2 | 9.9 | 0.78 | 11.1 |
TF-IS | 86.3 | 10.4 | 0.77 | 10.4 |
BA-IL | 86.9 | 9.2 | 0.83 | 3.3 |
TF-IL | 87.7 | 9.9 | 0.81 | 2.7 |
BA-RD | 75.3 | 18.4 | 0.47 | 27.6 |
TF-RD | 77.8 | 17.4 | 0.51 | 25.9 |
CNN | LSTM | BERT | LLaMA | Baichuan | |
CNN | -2.2 | +33.8 | +58.5 | +37.4 | +24.0 |
LSTM | +22.4 | -3.8 | +31.2 | +45.8 | +29.4 |
BERT | +21.4 | +26.0 | +2.2 | +25.4 | +25.6 |
LLaMA | +24.0 | +26.8 | +41.8 | +12.4 | +24.4 |
Baichuan | +22.2 | +20.6 | +22.4 | +21.6 | +4.4 |
ChatGPT | +14.8 | +18.6 | +22.4 | +22.4 | +18.8 |
Average | +21.2 | +23.6 | +35.2 | +30.0 | +27.6 |
4.3 Transferability
We evaluate the transferability of TF-Attack samples to detect whether the samples generated from TF-Attack can effectively attack other models. We conduct experiments on the IMDB datasets and use BERT-Attack as a baseline. Table 6 shows the improvement of the attack success rate of TF-Attack over BERT-Attack. It can be observed that TF-Attack achieves obvious improvements over BERT-Attack when applied to other models. In particular, the new samples generated by TF-Attack can lower the accuracy by over 10 percent on binary classification tasks, essentially confusing the victim model. Even a powerful baseline like ChatGPT would drop to only 68.6% accuracy. It is important to highlight that these samples do not necessitate attacks tailored to specific victim models.
4.4 Efficiency
We probe the efficiency according to varying sentence lengths in the IMDB dataset. As shown in Figure 5, the time cost of TF-Attack is surprisingly mostly better than BERT-Attack, which mainly targets obtaining cheaper computation costs with lower attack success rates in Table 4. Furthermore, with the increase of sentence lengths, TF-Attack maintains a stable time cost, while the time cost of BERT-Attack is exploding. The reason is that TF-Attack has the advantage of much faster parallel substitution, hence as the sentence grows, the increase in time cost will be much smaller. These phenomena verify the efficiency advantage of TF-Attack, especially in dealing with long texts.
BERT-Attack has emerged as the most widely adopted and efficacious technique for adversarial text attacks in the existing literature. Our TF-Attack has conducted a comprehensive analysis and introduced innovative enhancements to this approach. Consequently, we have chosen to benchmark our performance metrics against BERT-Attack to enable a more straightforward and direct comparative assessment. We have supplemented the speed experiments related to TextFooler and SDM-Attack on the IMDB dataset attacking SA-LLaMA, with the results pertaining to Figure 5 on Table 7.
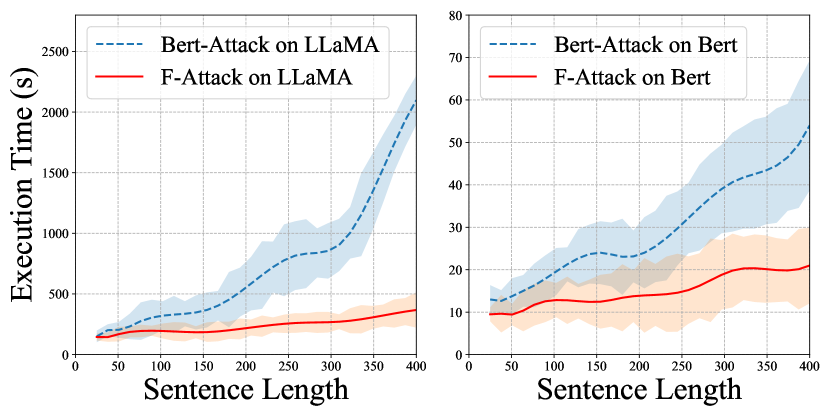
Method | on SA-LLaMA | on Bert |
TextFooler | 1034 | 32 |
Bert-Attack | 1126 | 29 |
SDM-Attack | 983 | 21 |
TF-Attack | 123 | 14 |
Method | A-rate | Mod | Sim |
TextBugger | 73.9 | 10.3 | 0.69 |
TextFooler | 78.9 | 9.1 | 0.73 |
Bert-Attack | 80.5 | 11.5 | 0.69 |
SDM-Attack | 81.1 | 10.7 | 0.71 |
Zero-Shot | 81.3 | 10.3 | 0.71 |
Few-Shot | 83.7 | 9.0 | 0.77 |
+MD | 84.6 | 12.3 | 0.71 |
+MD +DD | 84.5 | 11.9 | 0.72 |
Dataset | Con | Flu | ||
IMDB | Original | 0.88 | 4.0 | 0.82 |
TF-Attack | 0.79 | 3.8 | ||
MR | Original | 0.93 | 4.5 | 0.93 |
TF-Attack | 0.87 | 4.1 |
4.5 Manual evaluation
We follow Fang et al., 2023 to perform manual evaluation. At the beginning of manual evaluation, we provided some data to allow crowdsourcing workers to unify the evaluation standards. We also remove the data with large differences when calculating the average value to ensure the reliability and accuracy of the evaluation results. We first randomly select 100 samples from successful adversaries in IMDB and MR datasets and then ask ten crowd-workers to evaluate the quality of the original inputs and our generated adversaries. The results are shown in Table 9. For human prediction consistency, humans can accurately judge 93% of the original inputs on the IMDB dataset while maintaining an 87% accuracy rate with our generated adversarial examples. This suggests that TF-Attack can effectively mislead LLMs without altering human judgment. Regarding language fluency, the scores of our adversarial examples are comparable to the original inputs, with a minor score difference of no more than 0.3 across both datasets. Moreover, the semantic similarity scores between the original inputs and our generated adversarial examples stand at 0.93 for IMDB and 0.82 for MR, respectively. The result on NLI task is in Table 10. Overall, TF-Attack successfully preserves these three essential attributes.
4.6 More baseline experiments
BERT-Attack and TextFooler are currently the most widely used and powerful baselines in the field of text adversarial attacks, so we initially compared only these two methods. Due to time constraints, we conducted relevant experiments on the YELP dataset using TextBugger, with the victim model being SA-LLaMA. The results pertaining to Table 8 are supplemented in Table 8:
Dataset | Con | Flu | ||
MNLI | Original | 0.96 | 4.6 | 1.00 |
TextFooler | 0.86 | 4.1 | 0.85 | |
BERT-Attack | 0.82 | 4.3 | 0.91 | |
TF-Attack | 0.91 | 4.6 | 0.91 | |
SNLI | Original | 0.85 | 4.6 | 1.00 |
TextFooler | 0.77 | 3.9 | 0.84 | |
BERT-Attack | 0.78 | 3.7 | 0.84 | |
TF-Attack | 0.76 | 4.3 | 0.81 |
ASR | LLaMA-2b | LLaMA-2c | ChatGPT | Claude |
Prompt1 | / | / | 86.54% | 85.46% |
Prompt2 | / | 12.28% | 88.68% | 78.64% |
Prompt3 | / | / | 79.74% | 71.07% |
Prompt4 | / | 11.22% | 84.36% | 73.76% |
Prompt5 | 13.67% | / | 83.16% | 77.81% |
Prompt |
Rank each word in the input sentence into five levels based on its determining influence on the overall sentiment of the sentence. |
Determine the impact of each word on the overall sentiment of the sentence and categorise it into 5 levels. |
Rank the words from most to least influential in terms of their impact on the emotional tone of the sentence at 5 levels. |
Please classify each word into five levels, based on their importance to the overall emotional classification of the utterance. |
Assign each word to one of five levels of importance based on its contribution to the overall sentiment: Very High, High, Moderate, Low, Very Low. |
In fact, in earlier experiments Table 11, we attempted to use local LLMs or other different architectures of LLMs as selectors for attack order but found that open-source LLMs base models such as 7B, 13B, or even 30B could not understand our instructional intent well in this specific scenario, no matter Base models or Chat models. If we were to introduce a specially designed task fine-tuning process to achieve this functionality, it would not only require a large amount of manually labeled dataset but also incur even larger model training costs, which contradicts one of our motivations, accelerating adversarial attacks on large models. And we have supplemented the experimental results using Claude as a third-party selector, using the same Ensemble Prompt as ChatGPT. The experimental results have been added to Appendix. The following Table shows 100 samples attacked through different Prompts on different LLMs, ’/’ represents that LLM can not correctly output the Important Level. Table 12 shows all the 5 prompts we use in experiments.
Victim models | A-rate | Mod | Sim | Trans |
WordCNN | 96.3 | 9.1 | 0.84 | 78.5 |
WordLSTM | 92.8 | 9.3 | 0.85 | 75.1 |
BERT | 90.6 | 9.9 | 0.81 | 70.2 |
LLaMA | 91.8 | 13.1 | 0.74 | 68.6 |
Baichuan | 92.5 | 11.8 | 0.75 | 71.4 |
5 Analysis
5.1 Generalization to More Victim Models
Table 13 shows that TF-Attack not only has better attack effects against WordCNN and WordLSTM, but also misleads BERT and Baichuan, which are more robust models. For example, on the IMDB datasets, the attack success rate is up to 92.5% against Baichuan with a modification rate of only about 11.8% and a high semantic similarity of 0.75. Furthermore, the model generated by the Victim model created a decrease in accuracy to 71.4% on various black-box models of different scales.
5.2 Adversarial Training
Following Fang et al., 2023, we further investigate to improve the robustness of victim models via adversarial training using the generated adversarial samples. Specifically, we fine-tune the victim model with both original training datasets and our generated adversaries and evaluate it on the same test set. As shown in Table 14, compared to the results with the original training datasets, adversarial training with our generated adversaries can maintain close accuracy, while improving performance on attack success rates, modification rates, and semantic similarity. The victim models with adversarial training are more difficult to attack, which indicates that our generated adversaries have the potential to serve as supplementary corpora to enhance the robustness of victim models.
Dataset | Acc | A-rate | Mod | Sim |
Yelp | 97.4 | 81.3 | 8.5 | 0.73 |
+Adv Train | 95.9 | 65.7 | 12.3 | 0.67 |
IMDB | 97.2 | 86.1 | 4.6 | 0.81 |
+Adv Train | 95.5 | 70.2 | 7.3 | 0.78 |
AG-NEWS | 95.3 | 77.1 | 15.3 | 0.83 |
+Adv Train | 85.1 | 75.3 | 23.3 | 0.61 |
MR | 95.9 | 83.2 | 11.1 | 0.53 |
+Adv Train | 91.7 | 71.8 | 14.6 | 0.67 |
SST-2 | 97.1 | 89.7 | 14.3 | 0.85 |
+Adv Train | 92.2 | 68.6 | 16.8 | 0.83 |
Method | Text (MR; Negative) | Result | Mod | Sim | Flu |
Original | Davis is so enamored of her own creation that she can not see how insufferable the character is. | - | - | - | 5 |
TextFooler | Davis is well enamored of her own infancy that she could not admire how infernal the idiosyncrasies is. | Success | 33.3 | 0.23 | 3 |
BERT-Attack | Davis is often enamoted of her own generation that she can not see how insuffoure the queen is. | Failure | 27.8 | 0.16 | 2 |
TF-Attack | Davis is so charmed of her own crekation that she can’t see how indefensible the character is. @kjdjq2. | Success | 14.6 | 0.59 | 4 |
Table 14 displays adversarial training results of all datasets. Overall, after finetuned with both original training datasets and adversaries, victim model is more difficult to attack. Compared to original results, accuracy of all datasets is barely affected, while attack success rate meets an obvious decline. Meanwhile, attacking model with adversarial training leads to higher modification rate, further demonstrating adversarial training may help improve robustness of victim models.
5.3 Against Defense
Entropy threshold defense Yao et al. (2023) has been used to defend against the attack on LLMs recently. It employs the entropy of the first token prediction to refuse responding. Figure 6 demonstrates the probability of top-10 tokens in the first generated word of LLaMA. It can be observed that the raw inputs usually generates the first token with low entropy (i.e., the probability of argmax token is much higher, and the probability of other tokens is much lower). As shown in Figure 6, the adversarial samples from TF-Attack perform better than BERT-Attack with higher entropy. Attack samples generated through TF-Attack fare better against entropy-based filters compared to traditional text adversarial attack methods, indicating that the samples created by TF-Attack are harder to defend against.
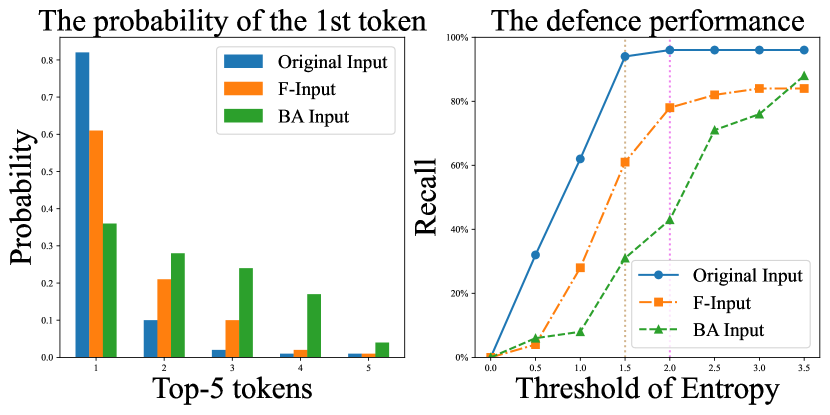
5.4 Case Study
Table 15 shows adversaries produced by TF-Attack and the baselines. Overall, the performance of TF-Attack is significantly better than other methods. For this sample from the MR dataset, only TextFooler and TF-Attack successfully mislead the victim model, i.e., changing the prediction from negative to positive. However, TextFooler modifies twice as many words as the TF-Attack, demonstrating our work has found a more suitable modification path. Adversaries generated by TextFooler and BERT-Attack are failed samples due to low semantic similarity. BERT-Attack even generates an invalid word "enamoted" due to its sub-word combination algorithm. We also ask crowd-workers to give a fluency evaluation.
Results show TF-Attack obtains the highest score of 4 as the original sentence, while other adversaries are considered difficult to understand, indicating TF-Attack can generate more natural sentences.
6 Conclusion
In this paper, we examined the limitations of current adversarial attack methods, particularly their issues with transferability and efficiency when applied to Large Language Models (LLMs). To address these issues, we introduced TF-Attack, a new approach that uses an external overseer model to identify key sentence components and allows for parallel processing of adversarial substitutions. Our experiments on six benchmarks demonstrate that TF-Attack outperforms current methods, significantly improving both transferability and speed. Furthermore, the adversarial examples made by TF-Attack do not significantly affect the performance of model after it has been trained to resist attacks, thus strengthening its defenses. We believe that TF-Attack is a significant improvement in creating strong defenses against adversarial attacks on LLMs, with potential benefits for future research in this field.
References
- Alzantot et al. (2018) Moustafa Alzantot, Yash Sharma, Ahmed Elgohary, Bo-Jhang Ho, Mani Srivastava, and Kai-Wei Chang. 2018. Generating natural language adversarial examples. In Proceedings of the 2018 Conference on Empirical Methods in Natural Language Processing, pages 2890–2896.
- Bowman et al. (2015) Samuel Bowman, Gabor Angeli, Christopher Potts, and Christopher D Manning. 2015. A large annotated corpus for learning natural language inference. In Proceedings of the 2015 Conference on Empirical Methods in Natural Language Processing, pages 632–642.
- Cer et al. (2018) Daniel Cer, Yinfei Yang, Sheng-yi Kong, Nan Hua, Nicole Limtiaco, Rhomni St John, Noah Constant, Mario Guajardo-Cespedes, Steve Yuan, Chris Tar, et al. 2018. Universal sentence encoder for english. In Proceedings of the 2018 conference on empirical methods in natural language processing: system demonstrations, pages 169–174.
- Cheng et al. (2018) Yong Cheng, Zhaopeng Tu, Fandong Meng, Junjie Zhai, and Yang Liu. 2018. Towards robust neural machine translation. In Proceedings of the 56th Annual Meeting of the Association for Computational Linguistics (Volume 1: Long Papers), pages 1756–1766.
- Coulombe (2018) Claude Coulombe. 2018. Text data augmentation made simple by leveraging nlp cloud apis. arXiv preprint arXiv:1812.04718.
- Ebrahimi et al. (2018a) Javid Ebrahimi, Daniel Lowd, and Dejing Dou. 2018a. On adversarial examples for character-level neural machine translation. In Proceedings of the 27th International Conference on Computational Linguistics, pages 653–663.
- Ebrahimi et al. (2018b) Javid Ebrahimi, Anyi Rao, Daniel Lowd, and Dejing Dou. 2018b. Hotflip: White-box adversarial examples for text classification. In Proceedings of the 56th Annual Meeting of the Association for Computational Linguistics (Volume 2: Short Papers). Association for Computational Linguistics.
- Fang et al. (2023) Xuanjie Fang, Sijie Cheng, Yang Liu, and Wei Wang. 2023. Modeling adversarial attack on pre-trained language models as sequential decision making. In Findings of the Association for Computational Linguistics: ACL 2023, pages 7322–7336.
- Gagnon-Marchand et al. (2019) Jules Gagnon-Marchand, Hamed Sadeghi, Md Haidar, Mehdi Rezagholizadeh, et al. 2019. Salsa-text: self attentive latent space based adversarial text generation. In Canadian Conference on Artificial Intelligence, pages 119–131. Springer.
- Garg and Ramakrishnan (2020) Siddhant Garg and Goutham Ramakrishnan. 2020. Bae: Bert-based adversarial examples for text classification. In Proceedings of the 2020 Conference on Empirical Methods in Natural Language Processing (EMNLP), pages 6174–6181.
- Guo et al. (2021) Chuan Guo, Alexandre Sablayrolles, Hervé Jégou, and Douwe Kiela. 2021. Gradient-based adversarial attacks against text transformers. In Proceedings of the 2021 Conference on Empirical Methods in Natural Language Processing, pages 5747–5757.
- Ji et al. (2024) Yixin Ji, Yang Xiang, Juntao Li, Wei Chen, Zhongyi Liu, Kehai Chen, and Min Zhang. 2024. Feature-based low-rank compression of large language models via bayesian optimization. arXiv e-prints, pages arXiv–2405.
- Jiang et al. (2024) Ruili Jiang, Kehai Chen, Xuefeng Bai, Zhixuan He, Juntao Li, Muyun Yang, Tiejun Zhao, Liqiang Nie, and Min Zhang. 2024. A survey on human preference learning for large language models. arXiv preprint arXiv:2406.11191.
- Jin et al. (2020) Di Jin, Zhijing Jin, Joey Tianyi Zhou, and Peter Szolovits. 2020. Is bert really robust? a strong baseline for natural language attack on text classification and entailment. In Proceedings of the AAAI conference on artificial intelligence, volume 34, pages 8018–8025.
- Kasneci et al. (2023) Enkelejda Kasneci, Kathrin Seßler, Stefan Küchemann, Maria Bannert, Daryna Dementieva, Frank Fischer, Urs Gasser, Georg Groh, Stephan Günnemann, Eyke Hüllermeier, et al. 2023. Chatgpt for good? on opportunities and challenges of large language models for education. Learning and individual differences, 103:102274.
- Li et al. (2021) Dianqi Li, Yizhe Zhang, Hao Peng, Liqun Chen, Chris Brockett, Ming-Ting Sun, and William B Dolan. 2021. Contextualized perturbation for textual adversarial attack. In Proceedings of the 2021 Conference of the North American Chapter of the Association for Computational Linguistics: Human Language Technologies, pages 5053–5069.
- Li et al. (2020) Linyang Li, Ruotian Ma, Qipeng Guo, Xiangyang Xue, and Xipeng Qiu. 2020. Bert-attack: Adversarial attack against bert using bert. In Proceedings of the 2020 Conference on Empirical Methods in Natural Language Processing (EMNLP), pages 6193–6202.
- Liu et al. (2016) Yanpei Liu, Xinyun Chen, Chang Liu, and Dawn Song. 2016. Delving into transferable adversarial examples and black-box attacks. In International Conference on Learning Representations.
- Liu et al. (2023) Yiheng Liu, Tianle Han, Siyuan Ma, Jiayue Zhang, Yuanyuan Yang, Jiaming Tian, Hao He, Antong Li, Mengshen He, Zhengliang Liu, et al. 2023. Summary of chatgpt-related research and perspective towards the future of large language models. Meta-Radiology, page 100017.
- Marcus (2020) Gary Marcus. 2020. The next decade in AI: four steps towards robust artificial intelligence. CoRR, abs/2002.06177.
- Morris et al. (2020) John Morris, Eli Lifland, Jin Yong Yoo, Jake Grigsby, Di Jin, and Yanjun Qi. 2020. Textattack: A framework for adversarial attacks, data augmentation, and adversarial training in nlp. In Proceedings of the 2020 Conference on Empirical Methods in Natural Language Processing: System Demonstrations, pages 119–126.
- Oliva et al. (2011) Jesús Oliva, José Ignacio Serrano, María Dolores Del Castillo, and Ángel Iglesias. 2011. Symss: A syntax-based measure for short-text semantic similarity. Data & Knowledge Engineering, 70(4):390–405.
- Ouyang et al. (2022) Long Ouyang, Jeffrey Wu, Xu Jiang, Diogo Almeida, Carroll Wainwright, Pamela Mishkin, Chong Zhang, Sandhini Agarwal, Katarina Slama, Alex Ray, et al. 2022. Training language models to follow instructions with human feedback. Advances in Neural Information Processing Systems, 35:27730–27744.
- Pang and Lee (2005) Bo Pang and Lillian Lee. 2005. Seeing stars: Exploiting class relationships for sentiment categorization with respect to rating scales. In Proceedings of the 43rd Annual Meeting of the Association for Computational Linguistics (ACL’05), pages 115–124.
- Qi et al. (2021) Fanchao Qi, Yangyi Chen, Xurui Zhang, Mukai Li, Zhiyuan Liu, and Maosong Sun. 2021. Mind the style of text! adversarial and backdoor attacks based on text style transfer. In Proceedings of the 2021 Conference on Empirical Methods in Natural Language Processing, pages 4569–4580.
- Sato et al. (2018) Motoki Sato, Jun Suzuki, Hiroyuki Shindo, and Yuji Matsumoto. 2018. Interpretable adversarial perturbation in input embedding space for text. In Proceedings of the 27th International Joint Conference on Artificial Intelligence, pages 4323–4330.
- Spector and Re (2023) Benjamin Frederick Spector and Christopher Re. 2023. Accelerating llm inference with staged speculative decoding. In Workshop on Efficient Systems for Foundation Models@ ICML2023.
- Thiebes et al. (2021) Scott Thiebes, Sebastian Lins, and Ali Sunyaev. 2021. Trustworthy artificial intelligence. Electronic Markets, 31(2):447–464.
- Thirunavukarasu et al. (2023) Arun James Thirunavukarasu, Darren Shu Jeng Ting, Kabilan Elangovan, Laura Gutierrez, Ting Fang Tan, and Daniel Shu Wei Ting. 2023. Large language models in medicine. Nature medicine, 29(8):1930–1940.
- Touvron et al. (2023) Hugo Touvron, Thibaut Lavril, Gautier Izacard, Xavier Martinet, Marie-Anne Lachaux, Timothee Lacroix, Baptiste Rozière, Naman Goyal, Eric Hambro, Faisal Azhar, et al. 2023. Llama: Open and efficient foundation language models. arXiv preprint arXiv:2302.13971.
- Wang et al. (2021) Boxin Wang, Chejian Xu, Shuohang Wang, Zhe Gan, Yu Cheng, Jianfeng Gao, Ahmed Hassan Awadallah, and Bo Li. 2021. Adversarial glue: A multi-task benchmark for robustness evaluation of language models. In Thirty-fifth Conference on Neural Information Processing Systems Datasets and Benchmarks Track (Round 2).
- Wei and Zou (2019) Jason Wei and Kai Zou. 2019. Eda: Easy data augmentation techniques for boosting performance on text classification tasks. In Proceedings of the 2019 Conference on Empirical Methods in Natural Language Processing and the 9th International Joint Conference on Natural Language Processing (EMNLP-IJCNLP), pages 6382–6388.
- Williams et al. (2018) Adina Williams, Nikita Nangia, and Samuel Bowman. 2018. A broad-coverage challenge corpus for sentence understanding through inference. In Proceedings of the 2018 Conference of the North American Chapter of the Association for Computational Linguistics: Human Language Technologies, Volume 1 (Long Papers), pages 1112–1122.
- Xie et al. (2020) Qizhe Xie, Zihang Dai, Eduard Hovy, Thang Luong, and Quoc Le. 2020. Unsupervised data augmentation for consistency training. Advances in Neural Information Processing Systems, 33:6256–6268.
- Xu et al. (2023) Xilie Xu, Keyi Kong, Ning Liu, Lizhen Cui, Di Wang, Jingfeng Zhang, and Mohan Kankanhalli. 2023. An llm can fool itself: A prompt-based adversarial attack. arXiv preprint arXiv:2310.13345.
- Yao et al. (2023) Jia-Yu Yao, Kun-Peng Ning, Zhen-Hui Liu, Mu-Nan Ning, and Li Yuan. 2023. Llm lies: Hallucinations are not bugs, but features as adversarial examples. arXiv preprint arXiv:2310.01469.
- Yoo and Qi (2021) Jin Yong Yoo and Yanjun Qi. 2021. Towards improving adversarial training of nlp models. In Findings of the Association for Computational Linguistics: EMNLP 2021, pages 945–956.
- Zang et al. (2020) Yuan Zang, Fanchao Qi, Chenghao Yang, Zhiyuan Liu, Meng Zhang, Qun Liu, and Maosong Sun. 2020. Word-level textual adversarial attacking as combinatorial optimization. In Proceedings of the 58th Annual Meeting of the Association for Computational Linguistics, pages 6066–6080.
- Zhang et al. (2015) Xiang Zhang, Junbo Zhao, and Yann LeCun. 2015. Character-level convolutional networks for text classification. Advances in neural information processing systems, 28.
- Zheng et al. (2020) Haizhong Zheng, Ziqi Zhang, Juncheng Gu, Honglak Lee, and Atul Prakash. 2020. Efficient adversarial training with transferable adversarial examples. In Proceedings of the IEEE/CVF Conference on Computer Vision and Pattern Recognition, pages 1181–1190.
- Zhong et al. (2024) Meizhi Zhong, Chen Zhang, Yikun Lei, Xikai Liu, Yan Gao, Yao Hu, Kehai Chen, and Min Zhang. 2024. Understanding the rope extensions of long-context llms: An attention perspective. arXiv preprint arXiv:2406.13282.