Storing Multi-model Data in RDBMSs based on Reinforcement Learning
Abstract.
How to manage various data in a unified way is a significant research topic in the field of databases. To address this problem, researchers have proposed multi-model databases to support multiple data models in a uniform platform with a single unified query language. However, since relational databases are predominant in the current market, it is expensive to replace them with others. Besides, due to the theories and technologies of RDBMSs having been enhanced over decades, it is hard to use few years to develop a multi-model database that can be compared with existing RDBMSs in handling security, query optimization, transaction management, etc. In this paper, we reconsider employing relational databases to store and query multi-model data. Unfortunately, the mismatch between the complexity of multi-model data structure and the simplicity of flat relational tables makes this difficult. Against this challenge, we utilize the reinforcement learning (RL) method to learn a relational schema by interacting with an RDBMS. Instead of using the classic Q-learning algorithm, we propose a variant Q-learning algorithm, called Double Q-tables, to reduce the dimension of the original Q-table and improve learning efficiency. Experimental results show that our approach could learn a relational schema outperforming the existing multi-model storage schema in terms of query time and space consumption.
1. Introduction
With the development of technology, different users may like to use different devices to collect relevant information from various perspectives for better describing or analyzing an identical phenomenon. For convenience, these devices with their supporting software would store the collected data in the way they like (e.g., using relational tables to preserve structured tabular data, using JSON document to record unstructured object-like data, and using RDF graph to store highly linked referential data), which is inevitable to cause data variety. Although choosing different databases to manage different data models is an ordinary operation, this would result in operational friction, latency, data inconsistency, etc., when using multiple databases in the same project.
Against this issue, researchers propose a multi-model database concept that manages multi-model data (see Figure 1) in a unified system (lu2019multi; lu2018multi). However, since researchers have spent decades strengthening RDBMS theories and technologies, it is not easy to use few years to develop a multi-model database to effectively and efficiently handle security, query optimization, transaction management, etc. Besides, since relational databases are still predominating the current market and storing mass legacy data, it is not easy to replace RDBMSs with multi-model databases at a low cost. Therefore, it fuels more and more interest to reconsider loading and processing multi-model data within RDBMSs.
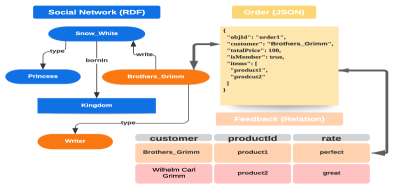
JSON, as the representative of semi-structured data, is proposed as a hierarchical, schema-less, and dynamic data interchange format on the web. Each JSON object comprises a set of structured key-value pairs, where key denotes attribute name, value is the attribute value. As for RDF data, it is a collection of statements where each statement is defined as subject-predicate-object (s,p,o) meaning that a subject has a predicate (property) with value . For storing them in RDBMSs, one straightforward method is to map the JSON document into several three-column tables (each table consists of object ID, key name, and value) (chasseur2013enabling) and parse the RDF graph into a series of triples stored in a three-column table (mcbride2002jena). Unfortunately, this method not only involves many self-joins, but it ignores the relationships among this multi-model data.
After reviewing the literature, we found there was still a blank for this research. The current works only focus on storing semi-structured document in RDBMSs (deutsch1999storingSTORED; chasseur2013enabling) or storing graph data in RDBMSs (zheng2020efficient; sun2015sqlgraph). Due to the mismatch between the complexity of multi-model data structure (as shown in Figure 1) and the simplicity of flat relational tables, we think it is a challenge to design a good schema for storing multi-model data in an RDBMS.
In this research, we attempt to generate a good relational schema based on the RL method to store and query multi-model data consisting of relational data, JSON documents, and RDF data in RDBMSs. It is not easy to employ the RL method to learn a relational schema for multi-model data. We need to define its states, actions, rewards, etc. And we propose a Double Q-tables method as a learning algorithm to help the agent choose actions, which extremely reduce the dimensions of the original Q-table’s action columns and improve learning efficiency.
The motivation for using RL is that RL is an optimization problem, and we could use Markov Decision Process to model the process of relational schema generation. Given an initial state (schema), RL could work with a dynamic environment (RDBMSs) and find the best sequence of actions (joins) that will generate the optimal outcome (relational schema collecting the most reward), where the most reward means the final generated schema have the minimum query time for a set of queries on the given workload. Specifically, we use the Q-learning algorithm to take in state observations (schemas) as the inputs and map them to actions (joins) as the outputs. In RL, this mapping is called the policy, which decides which action to take for collecting the most reward over time rather than a short-term benefit. It is just like supervised learning.
The rest of this paper is organized as follows. Section 2 introduces our approach. Section 3 shows the preliminary experimental results. Finally, the last section summarizes this paper.
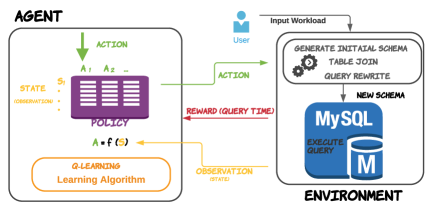
2. The Proposed Approach
2.1. The Overview of Framework
Since RL allows an agent to explore, interact with, and learn from the environment to maximize the cumulative reward, we propose a transforming multi-model data into relational tuples framework based on reinforcement Learning to store and query multi-model data in RDBMSs (see Figure 2). In this framework, we first generate an initial schema to be the initial state. Next, according to the current schema (state) and policy, we choose an action to generate a new (next) schema (state). Then, we rewrite the workload query statements to adapt the new schema and perform the rewritten query statements on the generated schema in MySQL databases. After that, the MySQL database returns the query time. And we regard the increase in negative query time compare to the previous state as a reward. Finally, the agent updates the Q-table based on the returned reward and observation (state). And it re-chooses an action to explore the potential relational schema or collect the most rewards that we already know about until the agent has tried all the actions in this episode (or the episode has reached the maximum number of iterations). After running the episode as many times as you want and collecting the generated states and their query time in this process, we could obtain a good relational schema for this multi-model data workload.
To formalize the problem of generating a relational schema for multi-model data as a reinforcement learning problem, we need to figure out input, goal, reward, policy, and observation. Next, we will introduce them separately.
objId | key | index | valStr |
1 | items | 0 | product1 |
1 | items | 1 | product2 |
2.2. Initial State
This framework uses a fully decomposed storage model (DSM) (copeland1985decomposition) to preserve multi-model data as the initial schema. Besides, we adopt the model-based method (florescu1999storing) to design a fixed schema for preserving JSON arrays. In detail, if the number of unique keys in the JSON document is ( + 1), we decompose a JSON document into two-column tables. Please note that we do not take JSON object id into account. We will use it to distinguish to which object these keys belong. For each table, the first column contains the JSON object id, and the second column holds the values for the corresponding key. Moreover, we use these keys as two-column table names. The table in Table 1 is called ArrayStringTable, which is designed to store array elements that have string type value. Similarly, we could also define ArrayNumberTable and ArrayBoolTable.
For the relational data, we split each table into multiple little tables whose amounts are equal to the number of unique attributes except the table keys. For each little table, the first several columns contain the original tuple keys, and the following column holds the values for the corresponding attribute (This attribute is also the name of this little table).
For the RDF graph, we break a triples table into multiple two-column tables whose amounts are equal to the number of unique predicates in this dataset. Within each table, the first column holds the subjects having the specific predicate, and its corresponding object values are preserved in the second column. Here, we use these predicates as two-column table names.
The benefits of using DSM are: (1) it could handle a dynamic dataset. If there is a new appearing attribute, we could add a new table to preserve it, and there is no need to change the current schema; (2) it could reduce the complexity of actions. In the next part, we will give the definition of actions.
2.3. Action
First, we collect and count all the keys (JSON), predicates (RDF), and attributes (relation) in the multi-model data. Next, we map each JSON key to an integer id from 1 to , map each predicate to an integer id from () to (), and map each attribute to an integer id from (