Spectrum of Heavy-Tailed Elliptic Random Matrices
Abstract.
An elliptic random matrix is a square matrix whose -entry is a random variable independent of every other entry except possibly . Elliptic random matrices generalize Wigner matrices and non-Hermitian random matrices with independent entries. When the entries of an elliptic random matrix have mean zero and unit variance, the empirical spectral distribution is known to converge to the uniform distribution on the interior of an ellipse determined by the covariance of the mirrored entries.
We consider elliptic random matrices whose entries fail to have two finite moments. Our main result shows that when the entries of an elliptic random matrix are in the domain of attraction of an -stable random variable, for , the empirical spectral measure converges, in probability, to a deterministic limit. This generalizes a result of Bordenave, Caputo, and Chafaï for heavy-tailed matrices with independent and identically distributed entries. The key elements of the proof are (i) a general bound on the least singular value of elliptic random matrices under no moment assumptions; and (ii) the convergence, in an appropriate sense, of the matrices to a random operator on the Poisson Weighted Infinite Tree.
1. Introduction
Let be the set of matrices over the field . For a matrix , the singular values of are the square roots of the eigenvalues of , where is the conjugate transpose of . We let denote the ordered singular values of , and be the eigenvalues of in no particular order. For a matrix we define the empirical spectral measure
and the empirical singular value measure
These measures are central objects in random matrix theory, and the goal of this paper is to study the asymptotic behavior of the empirical spectral measure for a class of heavy-tailed elliptic random matrices.
Elliptic random matrices can be thought of as interpolating between random matrices whose entries are independent and identically distributed (i.i.d.) and Wigner matrices. We now give a precise definition.
Definition 1.1 (Elliptic Random Matrix).
Let be a random vector with complex-valued random variable entries, a complex random variable, and be an random matrix. is an elliptic random matrix if
-
(i)
is a collection of independent random elements.
-
(ii)
the pairs are independent copies of .
-
(iii)
the diagonal elements are independent copies of .
We refer to as the atom variables of the matrix .
Elliptic random matrices were originally introduced by Girko [25, 26] in the 1980s, with the name coming from the limit of the empirical spectral measure. When the entries of the matrix have four finite moments, the limiting empirical spectral measures was investigated by Naumov [38]. The general case, when the entries are only assumed to have finite variance, was studied in [39].
Theorem 1.2 (Elliptic law for real random matrices, Theorem 1.5 in [39]).
Let be an elliptic random matrix with real atom variables . Assume have mean zero and unit variance, and for . Additionally assume has mean zero and finite variance. Then almost surely the empirical spectral measure of converges weakly to the uniform probability measure on
as .
Our main result, Theorem 1.6, gives an analogous result for heavy-tailed elliptic random matrices, i.e. when and are both infinite. In 1994, Cizeau and Bouchaud [18] introduced Lévy matrices as a heavy-tailed analogue of the Gaussian Orthogonal Ensemble (GOE). Instead of Gaussian entries, these matrices have entries in the domain of attraction of an -stable random variable, for . They predicted a deterministic limit , which depends only on , for the empirical spectral measures of these matrices when properly scaled. Convergence to a deterministic limit was first proved by Ben Arous and Guionnet [8] and later by Bordenave, Caputo, and Chafaï [12] with an alternative characterization in their study of random Markov matrices. In [13] Bordenave, Caputo, and Chafaï proved the empirical spectral measure of random matrices with i.i.d. entries in the domain of attraction of a complex -stable random variable converges almost surely to an isotropic measure on with unbounded support. Notably for Lévy matrices the limiting spectral measure inherits the tail behavior of the entries, while the limiting spectral measure of heavy-tailed random matrices with i.i.d. entries has a lighter tail and finite moments of every order.
Much of the work on heavy-tailed random matrices has been on the spectrum of symmetric matrices, either Lévy or sample covariance matrices [8, 4, 5, 7, 11, 2, 49, 12, 31]. Motivated by questions of delocalization from physics there has also been considerable work done in studying the eigenvectors of symmetric heavy-tailed matrices [16, 17, 1, 37, 18, 52, 9].
As is often the case in random matrix theory most of the work on heavy-tailed random matrices has focused on ensembles where the entries are independent up to symmetry conditions on the matrix. Work on matrices with dependent entries is still limited. Heavy-tailed matrices with normalized rows have been considered for random Markov chains in [12, 14] and sample correlation matrices in [31]. In [6] extreme eigenvalue statistics of symmetric heavy-tailed random matrices with -dependent entries were studied and shown to converge to a Poisson process. This -dependence can be thought of as a short range dependence meant to model stock returns that depend on stocks from the same sector of size determined by . To the best of our knowledge there are not any results on non-Hermitian heavy-tailed matrices with long range dependence between entries from different rows outside the random reversible Markov chains studied in [12].
The key question when approaching heavy-tailed elliptic random matrices is how to measure the dependence between and . Without two finite moments the covariance between and , which was the key parameter in Theorem 1.2, cannot be defined. Similar notions, such as covariation or codifference, exist for -stable random variables but they do not seem sufficient for our purposes. The difference is that the covariation does not provide as much information for -stable random vectors as the covariance does for Gaussian random vectors. If is a bivariate Gaussian random vector where and are standard real Gaussian random variables, then the correlation uniquely determines the distribution of . Thus one approach to measuring dependence is to find a parameter which uniquely determines the distribution of a properly normalized -stable random vector. The distribution of an -stable random vector in is determined uniquely through its characteristic function of the form
for a finite measure on the unit sphere and a deterministic vector . can be translated and scaled so that and is a probability measure uniquely determining the distribution of . is called the spectral measure of , and it turns out to be the appropriate explicit description of the dependence between the entries of . The definition of can be extended to random variables which are not stable but rather in the domain of attraction of an -stable random variable, see Definition 1.3.
If the components of are independent, then is supported entirely on the intersection of the axes and the unit sphere. Intuitively, when considering the mirrored entries of a random matrix, if is close to a measure supported on the intersection of the axes and the unit sphere, the entries are close to independent, after scaling. If is close to a measure supported on the set then the matrix is close to Hermitian. Numerical simulations seem to reflect this intuition in the spectrum of elliptic random matrices, see Figures 1, 2, and 3.
1.1. Matrix distribution
We will consider elliptic random matrices whose atom variables satisfy the following conditions.
Definition 1.3 (Condition C1).
We say the atom variables satisfy Condition C1 if
-
(i)
there exists a positive number , a sequence for a slowly varying function (i.e. for all ), and a finite measure on the unit sphere in such that for all Borel subsets of the unit sphere with and all ,
where is a measure on with density .
-
(ii)
there exists a constant such that for all .
We will reserve to denote the measure on a sphere associated to the atom variables of a heavy-tailed elliptic random matrix; it may be worth noting that stands for “dependence” and is not a parameter. As it turns out, Condition C1 is enough to prove convergence of the empirical singular value distribution, see Theorem 1.5. We will need more assumptions to prove convergence of the empirical spectral measure.
Definition 1.4 (Condition C2).
We say satisfy Condition C2 if the atom variables satisfy Condition C1 and if
-
(i)
there exists no such that
-
(ii)
, for some constant as .
1.2. Main results
For simplicity, let . Our first result gives the convergence of the singular values of , which we will denote as , for . Here and throughout, denotes the identity matrix. While interesting on its own, this is the first step in the method of Hermitization to establish convergence of the empirical spectral measure. Throughout we will use to denote weak convergence of probability measures and convergence in distribution of random variables.
Theorem 1.5 (Singular values of heavy-tailed elliptic random matrices).
Let be an elliptic random matrix with atom variables which satisfy Condition C1. Then for each there exists a deterministic probability measure , depending only on and , such that almost surely
as .
Under Condition C2 we prove the convergence of the empirical spectral measure of .
Theorem 1.6 (Eigenvalues of heavy-tailed elliptic random matrices).
Let be an elliptic random matrix with atom variables which satisfy Condition C2. Then there exists a deterministic probability measure , depending only on and , such that
in probability as . Moreover for any smooth with compact support
(1.1) |
where is as in Theorem 1.5 and is the Lebesgue measure on .
A distributional equation describing the Stieltjes transform of is given in Proposition 4.12, which, when combined with (1.1), gives a description of .
Remark 1.7.
If where and are probability measures with and , then in Theorem 1.5 and in Theorem 1.6 are the same measures as in the main results of Bordenave, Caputo, and Chafaï [13]. This can be seen by computing when and are independent and identically distributed. It is also worth noting that if the matrix is complex Hermitian but not real symmetric, then will satisfy Condition C2 (i), and thus Theorem 1.6 holds.
Numerical simulations seem to give weight to the reasoning that the support of determines how close is to being isotropic or supported on the real line. In Figure 3 we see as moves further from the mass of the spectrum moves further from the real line. A similar phenomenon appears in Figure 2: as moves further from the intersection of the axes and the sphere the spectrum becomes further from isotropic. We also see in Figure 1 that the tail behavior of the spectrum appears to depend on and may vary in different directions.
Spectrum similar to Figure 1 can be found in [30] where the authors study the spectral measure of where and are free random Lévy elements. The authors use tools in free probability to show the spectrum is supported inside the “hyperbolic cross”, which appears to be the case for heavy-tailed elliptic random matrices for certain . The limiting spectral measure in [13] is not contained in a “hyperbolic cross”, which suggests is not the heavy-tailed analogue of a circular element, in contrast with the case when and are free semicircular elements and is a circular element.
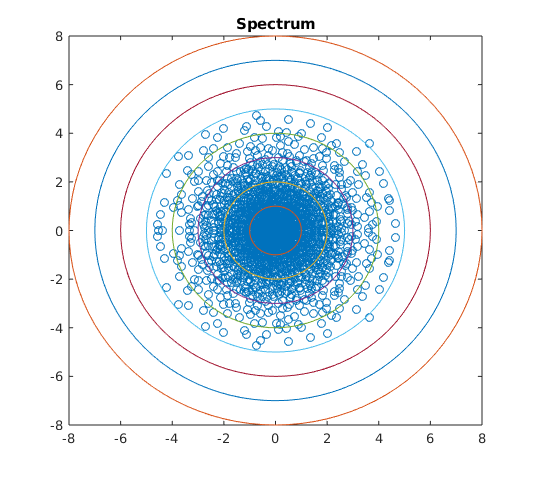
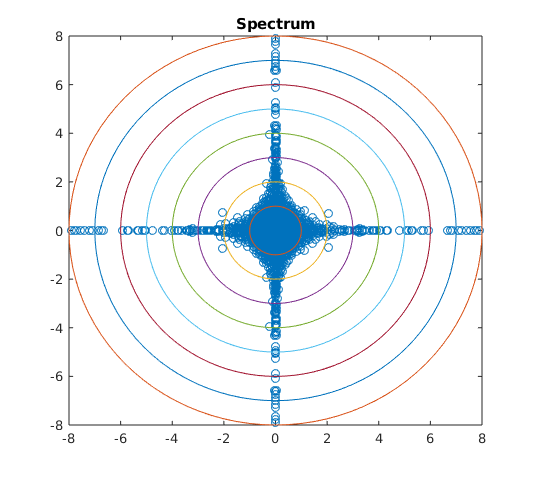
1.3. Further questions
Since our results capture both heavy-tailed Hermitian matrices and heavy-tailed matrices with i.i.d. entries, does actually depend on . However, as can be seen from [13], does not depend on every aspect of .
Question 1.8.
What properties of determine ?
In the case when has i.i.d. entries the limiting empirical spectral measure has an exponential tail [13] while in the case when is Hermitian it has the same tail behavior as the entries [8, 12]. This leads us to ask how the tail of depends on .
Question 1.9.
How does the tail behavior of vary with respect to ?
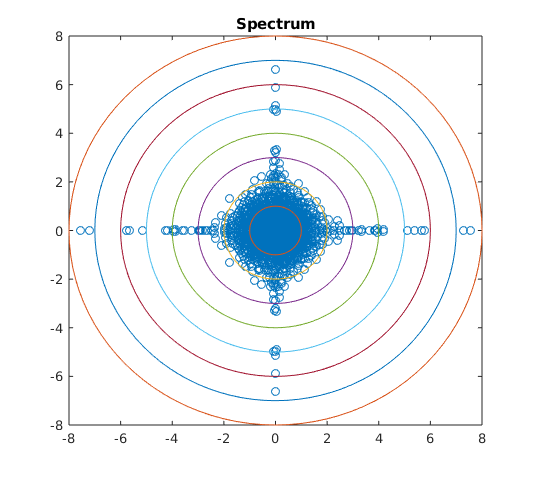
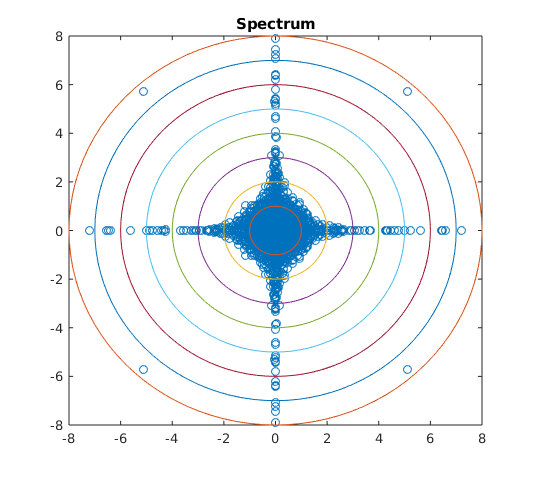
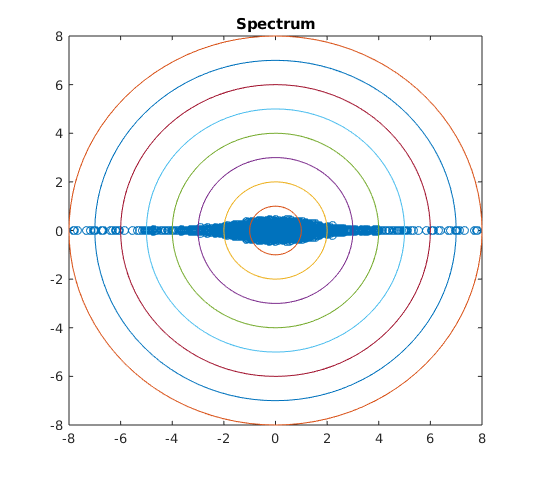
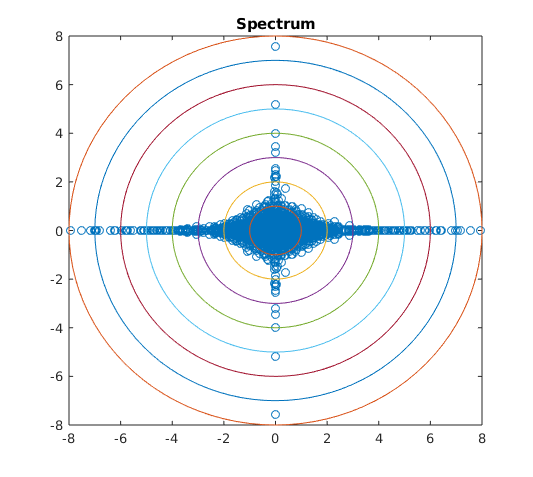
1.4. Outline
As expected with the empirical spectral measure of non-Hermitian random matrices, we make use of Girko’s Hermitization method. However, instead of considering the logarithmic potential directly, we follow the approach of Bordenave, Caputo, and Chafaï [12, 13] by using the objective method of Aldous and Steele [3] to get convergence of the matrices to an operator on Aldous’ Poisson Weighted Infinite Tree (PWIT). This objective method approach was expanded by Jung [34] to symmetric light-tailed random matrices, adjacency matrices of sparse random graphs, and symmetric random matrices whose column entries are some combination of heavy-tailed and light-tailed random variables.
In Sections 2 and 3 we give a collection of results and background for approaching the proof of Theorem 1.5. In Section 4 we define the PWIT, establish the local weak convergence of the matrices to an operator associated with the PWIT, and give a proof of Theorem 1.5. In Section 5 we give a very general bound on the least singular value of elliptic random matrices. In Section 6 we establish the uniform integrability of against and complete the proof of Theorem 1.6. The appendix contains some auxiliary results.
We conclude this section by establishing notation, giving a brief description of Hermitization, and stating some properties of and implied by Condition C2.
1.5. Notation
We now establish notation we will use throughout.
Let denote the discrete interval. For a vector and a subset , we let . Similarly, for an matrix and , we define . For a countable set we will let denote the identity on . In the case when we will simply write or if the dimension is clear.
For a vector , is the Euclidean norm of . For a matrix , is the transpose of and is the conjugate transpose of . In addition, is the spectral norm of and is the Hilbert–Schmidt norm of defined by the formula
For a linear, but not necessarily bounded, operator on a Hilbert space we let denote the domain of . We will often use the shorthand to denote where is the identity operator.
For two complex-valued square integrable random variables and , we define the correlation between and as
where is the covariance between and , and is the variance of . For two random elements and we say if and have the same distribution. We will also say a positive random variable stochastically dominates a positive random variable if for all
For a topological space , will always denote the Borel -algebra of . will denote the positive real numbers.
Throughout this paper we will use asymptotic notation (, etc.) under the assumption that . if for an absolute constant and all , if for , if for absolute constants and all , and if .
1.6. Hermitization
Let be the set of probability measures on which integrate in a neighborhood of infinity. For every the logarithmic potential of on is a function defined for every by
In one has , where is the set of Schwartz-Sobolev distributions on endowed with its usual convergence with respect to all infinitely differentiable functions with compact support.
Lemma 1.10 (Lemma A.1 in [13]).
For every , if a.e. then .
To see the connection between logarithmic potentials and random matrices consider an random matrix . If is the characteristic polynomial of , then
(1.2) |
Thus, through the logarithmic potential we can move from a question about eigenvalues of to singular values of . We refer the reader to [15] for more on the logarithmic potential in random matrix theory. One immediate issue is that is not a bounded function on , and thus we need more control on the integral of with respect to .
Definition 1.11 (Uniform integrability almost surely and in probability).
Let be a sequence of random probability measures on a measurable space . We say a measurable function is uniformly integrable almost surely with respect to if
with probability one. We say a measurable function is uniformly integrable in probability with respect to if for every
Lemma 1.12 (Lemma 4.3 in [15]).
Let be a sequence of complex random matrices where is for every . Suppose for Lebesgue almost all , there exists a probability measure on such that
-
•
a.s. tends weakly to
-
•
a.s. (resp. in probability) is uniformly integrable for .
Then there exists a probability measure such that
-
•
a.s. (resp. in probability) converges weakly to
-
•
for almost every ,
1.7. Individual entries and stable random vectors.
We now state some useful properties of and implied by Condition C2. First, if are i.i.d copies of , then Condition C1 (i) guarantees, see [46], there exists a sequence such that
for some -stable random vector with spectral measure . Condition C2 (i) guaranties neither nor is identically . We need the following theorem to get results on stable random vectors.
Theorem 1.13 (Theorem 2.3.9 in [47]).
Let be an -stable vector in . Then is an -stable random vector for any .
From this, with for real and for complex , we get that is in the domain of attraction of an -stable random variable and satisfies
(1.3) |
for some slowly varying function . In addition,
(1.4) |
for some probability measure , again see [46]. The same holds for with a possibly different probability measure . Also note
(1.5) |
for all and .
Acknowledgment
The second author thanks Djalil Chafaï for answering his questions concerning [15, Appendix A].
2. Poisson point processes and stable distributions
In this section we give a brief review of Poisson Point Processes (p.p.p.) and their relation to the order statistics of random variables in the domain of attraction of an -stable distribution. See [21, 43, 44], and the references therein for proofs.
2.1. Simple point processes
Throughout this section we will assume with the relative topology, where is the one point compactification of , but many of the results can be extended to other topological spaces.
Denote by the set of simple point Radon measures
where is such that is a finite set for any and is the ball of radius around the point . Denote by the set of supports corresponding to measures in . The elements of are called configurations.
Let denote the set of real-valued continuous functions on with compact support. The vague topology on is the topology where a sequence converges to if for any
with the vague topology is a Polish space, and thus complete and metrizable.
If one considers the one-to-one function given by , then the topology of can be pushed forward to . The vague convergence in can be stated in terms of a convergence of the supports. Let and give some labeling . Then this vague convergence implies there exists labeling such that for all , . This description will be particularly useful for our case.
A simple point process is a measurable mapping from a probability space to , where is the Borel -algebra defined by the vague topology. Weak convergence of simple point processes is defined by weak convergence of the measures in the vague topology.
2.2. Poisson point process
Definition 2.1.
Let be a Borel measure on . A point process is called a Poisson Point Process (p.p.p.) with intensity measure if for any pairwise disjoint Borel sets , the random variables are independent Poisson random variables with expected values .
Remark 2.2.
is a.s. simple if and only if is non-atomic.
The next proposition makes clear the connection between point processes and stable random variables. See Proposition 3.21 in [43] for a proof. First, we describe an important point process. Let be a finite measure on the unit sphere in , and be a measure with density on . We let denote the p.p.p. on with intensity measure .
Proposition 2.3.
Let be i.i.d. -valued random variables. Assume there exists a finite measure on the unit sphere in such that
for every and all Borel subsets of the unit sphere with , where for and a slowly varying function . Then
as .
Remark 2.4.
In [21], Davydov and Egorov prove this convergence in -type topologies, under a smoothness assumption on the .
2.3. Useful properties of
The following are useful and well known properties of the p.p.p. . Again, see Davydov and Egorov, [21], and the references therein for more information and proofs.
Proposition 2.5.
Let and be independent i.i.d. sequences where has exponential distribution with mean , and is distributed, with a finite nonzero measure on . Define . Then, for any ,
Lemma 2.6.
The p.p.p. has the following properties.
-
(1)
Almost surely there are only a finite number of points of outside a ball of positive radius centered at the origin.
-
(2)
is simple.
-
(3)
Almost surely, we can label the points from according to the decreasing order of their norms .
-
(4)
With probability one, for any ,
3. Bipartized resolvent matrix
In this section we follow the notation of Bordenave, Caputo, and Chafaï in [13] to define the bipartizations of matrices and operators.
3.1. Bipartization of a matrix
For an complex matrix we consider a symmetrized version of ,
Let and
For any and define the following matrices
Define the matrix by . As an element of , is Hermitian. We call the bipartization of the matrix . We define the resolvent matrix in by
so that for all , . For we write,
Letting we have
Let denote the Stieltjes transform of a probability measure on defined by
Theorem 3.1 (Theorem 2.1 in [13]).
Let . Then ,
and in , the set of Schwartz-Sobolev distributions on endowed with its usual convergence with respect to all infinitely differentiable functions with compact support,
3.2. Bipartization of an operator
Let be a countable set and let denote the Hilbert space defined by the inner product
where is the unit vector supported on . Let denote the dense subset of of vectors with finite support. Let be a collection of complex numbers such that for all ,
We then define a linear operator with domain by
(3.1) |
Let be a set in bijection with , and let be the image of under the bijection. Let , and define the bipartization of as the symmetric operator on by
Let denote the orthogonal projection onto the span of . is isomorphic to under the map . Under this isomorphism we may think of as a linear map from to with matrix representation
Let . For simplicity we will denote by the closure of . Recall the sum of an essentially self-adjoint operator and a bounded self-adjoint operator is essentially self-adjoint, thus if is (essentially) self-adjoint then is (essentially) self-adjoint. For , we define the resolvent operator
and
(3.2) |
Lemma 3.2.
If are defined by (3.2), then
-
•
for each , ,
-
•
the functions are analytic on ,
-
•
and
Moreover, if , then are pure imaginary and .
4. Convergence to the Poisson Weighted Infinite Tree
4.1. Operators on a tree
Consider a tree on a vertex set with edge set . We say if . Assume if then , in particular for all . We consider the operator defined by (3.1). We begin with useful sufficient conditions for a symmetric linear operator to be essentially self-adjoint, which will be very important for our use.
Lemma 4.1 (Lemma A.3 in [12]).
Let and be a tree. Assume that for all , and if then . Assume that there exists a sequence of connected finite subsets of , such that , , and for every and ,
Then is essentially self-adjoint.
Corollary 4.2 (Corollary 2.4 in [13]).
Let and be a tree. Assume that if then . Assume there exists a sequence of connected finite subsets of , such that , , and for every and ,
Then for all , is self-adjoint.
The advantages of defining an operator on a tree also include the following recursive formula for the resolvents, see Lemma 2.5 in [13] for a proof.
Proposition 4.3.
Assume is sellf-adjoint and let . Then
(4.1) |
where where is the bipartization of the operator restricted to , and is the subtree of of vertices whose path to contains .
4.2. Local operator convergence.
We now define a useful type of convergence.
Definition 4.4 (Local Convergence).
Suppose is a sequence of bounded operators on and is a linear operator on with domain . For any we say that converges locally to , and write
if there exists a sequence of bijections such that and, for all ,
in , as .
Here we use for the bijection on and the corresponding linear isometry defined in the obvious way. This notion of convergence is useful to random matrices for two reasons. First, we will make a choice on how to define the action of an matrix on , and the bijections help ensure the choice of location for the support of the matrix does not matter. Second, local convergence also gives convergence of the resolvent operator at the distinguished points . This comes down to the fact that local convergence is strong operator convergence, up to the isometries. See [13] for details.
Theorem 4.5 (Theorem 2.7 in [13]).
Assume for some . Let be the self-adjoint bipartized operator of . If the bipartized operator of is self-adjoint and is a core for (i.e., the closure restricted to is ), then for all
To apply this to random operators we say that in distribution if there exists a sequence of random bijections such that in distribution for every .
4.3. Poisson Weighted Infinite Tree (PWIT)
Let be a positive Radon measure on such that . is the random weighted rooted tree defined as follows. The vertex set of the tree is identified with by indexing the root as , the offspring of the root as and, more generally, the offspring of some as . Define as the tree on with edges between parents and offspring. Let be independent realizations of a Poisson point process with intensity measure . Let be ordered such that , and assign the weight to the edge between and , assuming such an ordering is possible. More generally assign the weight to the edge between and where where
Consider a realization of , with . Even though the measure has a more natural representation in polar coordinates, we let be the Cartesian coordinates of . Define an operator on by the formulas
(4.2) |
and otherwise. For , we know by Lemma 2.6 that the points in are almost surely square summable for every , and thus is actually a well defined linear operator on , though is possibly unbounded on . Before showing the local convergence of the random matrices to we will show the bipartization of is self-adjoint.
Proposition 4.6.
With probability one, for all , is self-adjoint, where is the bipartization of the operator defined by (4.2).
We begin with a lemma on point processes, proved in Lemma A.4 from [12], before checking our criterion for self-adjointness.
Lemma 4.7.
Let and let be a Poisson process of intensity on . Define Then is finite and goes to as goes to infinity.
Proof of Proposition 4.6.
For and define
Note if is a homogeneous Poisson process on with intensity 1 and , then is Poisson process with intensity measure . Thus by Lemma 4.7, can be chosen such that for any fixed . Since the random variables are i.i.d. this works for all . Fix such a . We now color the vertices red and green in an effort to build the sets in Corollary 4.2. Put a green color on all vertices such that and a red color otherwise. Define the sub-forest of where an edge between and is included if is green and . If the root is red let . Otherwise let be the subtree of containing . Let denote the number of vertices in at a depth from the root, then is a Galton-Watson process with offspring distribution . It is well known that if , then the tree is almost surely finite, see Theorem 5.3.7 in [22]. Let be the leaves of the tree . Set . It is clear for any ,
Now define the outer boundary of as and for set . For a connected set define its outer boundary as
Now for each apply the above process to get subtrees with roots and the leaves of tree denoted by . Set
Apply this procedure iteratively to get the sequence of subsets . Apply Corollary 4.2 to complete the proof. ∎
4.4. Local convergence
For an matrix we aim to define as a bounded operator on . For let . and otherwise.
Theorem 4.8.
Let be a sequence of uniformly distributed random permutation matrices, independent of . Then, in distribution, where is the operator defined by (4.2).
Remark 4.9.
This theorem holds for any sequence of permutation matrices regardless of independence or distribution, but for our use later it is important they are independent of and uniformly distributed. This is to get around the fact the entries of are not exchangeable.
The rest of this subsection is devoted to the proof of Theorem 4.8. The procedure follows along the same lines as Bordenave, Caputo, and Chafaï in [12] and [13]. We will define a network as a graph with edge weights taking values in some normed space. To begin let be the complete network on whose weight on edge equals for some collection of i.i.d. random variables taking values in some normed space. Now consider the rooted network with the distinguished vertex . For any realization , and for any such that , we will define a finite rooted subnetwork of whose vertex set coincides with a -ary tree of depth . To this end we partially index the vertices of as elements in
the indexing being given by an injective map from to . We set and the index of the root . The vertex is given the index , if has the -th largest norm value among , ties being broken by lexicographic order111To help keep track of notation in this section, note that if and .. This defines the first generation, and let be the union of and this generation. If repeat this process for the vertex labeled on to order to get . Define to be the union of and this new collection. Repeat again for to get the second generation and so on. Call this vertex set .
For a realization of , recall we assign the weight to the edge . Then is a rooted network. Call the finite rooted subnetwork obtained by restricting to the vertex set . If an edge is not present in assign the weight . We say a sequence , for fixed and , converges in distribution, as , to if the joint distribution of the weights converges weakly.
Let be the permutation on associated to the permutation matrix . We let
We now consider with weights and a realization, , of . We aim to show converges in distribution to , for fixed as .
Order the elements of lexicographically, i.e. . For let denote the offspring of in . By construction and , where must be strict in this union. Thus at every step of the indexing procedure we order the weights of neighboring edges not already considered at a previous step. Thus for all ,
Note that by independence, Proposition 2.3 still holds if you take the empirical sum over for any fixed finite set . Thus by Proposition 2.3 the weights from a fixed parent to its offspring in converge weakly to those of . By independence we can extend this to joint convergence. Because for any fixed , is still a complete network on , we must now check the weights connected to vertices not indexed above converge to zero, which is the weight given to edges not in the tree. For define
Also let denote independent variables distributed as . Let denote the set of edges that do not belong to the finite subtree . Because we have sorted out the largest elements, the vector is stochastically dominated by the vector (see [12] Lemma 2.7). Since is finite, the vector converges to as . Thus .
Let be the operator associated to defined by (4.2). For fixed let be the map above associated to , and arbitrarily extend to a bijection on , where is considered in the natural way as a subset of the offspring of . From the Skorokhod Representation Theorem we may assume converges almost surely to . Thus there is a sequences tending to infinity and such that for any pair , converges almost surely to
Thus almost surely
where depending on whether is an offspring of , vice versa, or we take the convention if neither in which case . To prove the local convergence of operators it is sufficient, by linearity, to prove point wise convergence for any . For convenience let . Thus all that remains to be shown to complete the proof of Theorem 4.8 is that almost surely as
4.5. Resolvent matrix
. Let be the resolvent of the bipartized random operator of . For , set
(4.3) |
We have the following result.
Theorem 4.10.
Let , , and be as in the Theorem 4.8. Since is almost surely self-adjoint we may almost surely define , the resolvent of . Let be the resolvent of the bipartized matrix of . For all ,
as .
As functions, the entries of the resolvent matrix are continuous, and by Lemma 3.2 are bounded. Thus
(4.4) |
Note that by independence of and
(4.5) |
This is the reason for the choice of uniformly distributed independent of .
Theorem 4.11.
For all , almost surely the measures converge weakly to a deterministic probability measure whose Stieltjes transform is given by
for and in (4.3).
Proof.
By Proposition 4.6 for every , is almost surely essentially self-adjoint. Thus, using the Borel functional calculus, there exists almost surely a random probability measure on such that
See Theorem VIII.5 in [42] for more on this measure and the Borel functional calculus for unbounded self-adjoint operators. Define as the resolvent matrix of , the bipartized matrix of . For , we write
By Theorem 3.1
It follows that converges to some deterministic probability measure . By Lemma A.2 concentrates around its expected value, and thus by the Borel-Cantelli Lemma converges almost surely to . ∎
Theorem 1.5 follows immediately from Theorem 4.11. We conclude this section with a recursive distributional equation describing .
Proposition 4.12.
Let be a probability measure. Let be a distributed random vector independent of the matrix given in (4.3). Additionally let be the measure on which is the distribution of the random vector
(4.6) |
where and are as in (4.3). Then the matrix given in (4.3) satisfies the following recursive distributional equation
(4.7) |
where is an -stable random vector with spectral measure is given by the image of the measure under the map .
5. Least singular values of elliptic random matrices
Now that we have proven Theorem 1.5, we move on to show that is uniformly integrable, in probability, with respect to . We begin with a bound on the least singular value of an elliptic random matrix under very general assumptions. This section is entirely self contained.
Theorem 5.1 (Least singular value bound).
Let be an complex-valued random matrix such that
-
(i)
(off-diagonal entries) is a collection of independent random tuples,
-
(ii)
(diagonal entries) the diagonal entries are independent of the off-diagonal entries (but can be dependent on each other),
-
(iii)
there exists such that the events
(5.1) defined for satisfy
and
Then there exists such that for , any , , ,
Remark 5.2.
5.1. Proof of Theorem 5.1
In this section we prove Theorem 5.1. Suppose and satisfy the assumptions of the theorem and denote . We will use throughout this section and it may be worth noting it is not the of Theorems 1.5 and 1.6. Throughout the section, we allow all constants to depend on without mentioning or denoting this dependence. Constants, however, will not depend on ; instead we will state all dependence on explicitly.
For the proof of Theorem 5.1, it suffices to assume that and every principle submatrix of is invertible with probability . To see this, define , where is the identity matrix and is a real-valued random variable uniformly distributed on the interval , independent of . It follows that satisfies the assumptions of Theorem 5.1. However, since is continuously distributed, it also follows that and every principle submatrix of is invertible with probability . By Weyl’s inequality for the singular values (see, for instance, [10, Problem III.6.13]), we find
Hence, we conclude that
In other words, it suffices to prove Theorem 5.1 under the additional assumption that and every principle submatrix of is invertible. We work under this additional assumption for the remainder of the proof.
5.2. Nets and a decomposition of the unit sphere
Consider a compact set and . A subset is called an -net of if for every point one has .
For some real positive parameters that will be determined later, we define the set of -sparse vectors as
We decompose the unit sphere into the set of compressible vectors and the complementary set of incompressible vectors by
and
We have the following result for incompressible vectors.
Lemma 5.3 (Incompressible vectors are spread; Lemma A.3 from [15]).
Let . There exists a subset such that and for all ,
5.3. Control of compressible vectors
The case of compressible vectors roughly follows the arguments from [15]. For , we let denote the -th column of and denote the -th column of with the -th entry removed.
Lemma 5.4 (Distance of a random vector to a deterministic subspace).
There exist constants such that for all and any deterministic subspace of with , we have
Proof.
The proof follows the same arguments as those given in the proof of [15, Theorem A.2]. Fix ; the arguments and bounds below are all uniform in . Recall the definitions of the events given in (5.1). By the assumptions on , the Chernoff bound gives
In other words, with high probability, at least of the events , occur. Thus, it suffices to prove the result by conditioning on the event
(5.2) |
There are two cases to consider. Either or . The arguments for these two cases are almost identical except some notations must be changed slightly to remove the -th index. For the remainder of the proof, let us only consider the case when ; the changes required for the other case are left to the reader.
Recall that it suffices to prove the result by conditioning on the event defined in (5.2). In fact, as , the definition of the event given in (5.2) can be stated as
Let denote the conditional expectation given the event and the filtration generated by , , . Let be the subspace spanned by and the vectors
and
By construction, and is measurable. In addition,
where
By assumption, the coordinates are independent random variables which satisfy
for . Thus, since the function is convex and -Lipschitz, Talagrand’s concentration inequality (see for instance [50, Theorem 2.1.13]) yields
(5.3) |
for every , where are absolute constants. In particular, this implies that
(5.4) |
for an absolute constant . Thus, if denotes the orthogonal projection onto the orthogonal complement of , we get
The last term is lower bounded by for all sufficiently large by taking . Combining this bound with (5.3) and (5.4) completes the proof. ∎
The next bound, which follows as a corollary of Lemma 5.4, will be useful when dealing with compressible vectors.
Corollary 5.5.
There exist such that for any deterministic subset with and any deterministic , we have
where .
Proof.
We will apply Lemma 5.4 to control . To this end, define to be the vector with the -th entry removed, and set . Then
for all . Note that is independent of . Hence, conditioning on and applying Lemma 5.4, we find the existence of such that for any with and all
Therefore, by the union bound,
and the proof is complete. ∎
Let be as in Corollary 5.5. From now on we set
(5.5) |
(Recall that is the upper bound for specified in the statement of Theorem 5.1.) In particular, this definition implies that . The parameter is still to be specified. Right now we only assume that .
Lemma 5.6 (Control of compressible vectors).
There exist constants such that for any deterministic vector and any
Proof.
Let be a constant to be chosen later. We decompose as
where
and
So by the union bound,
(5.6) | ||||
Fix with , and suppose there exists such that and . Then there exists with . In particular, this implies that . In addition, we have
by the assumption that and the definition of (5.5). Hence, we obtain
(5.7) |
We now bound from below. Indeed, we have
(5.8) |
where . In addition, we bound
Thus, combining (5.7) and (5.8) with the bound above, we find
To conclude, we have shown that
In view of Corollary 5.5, there exist such that for any with , we have
Returning to (5.6), we conclude that
for some constants by taking sufficiently small (in terms of ). ∎
We now fix to be the constant from Lemma 5.6. Thus, and are now completely determined. We will also need the following corollary of Lemma 5.6.
Corollary 5.7.
There exist constants such that for any deterministic vector and any
(5.9) |
Proof.
The proof is based on the arguments given in [53]. We first note that if , then the claim follows immediately from Lemma 5.6. Assume . Let denote the event on the left-hand side of (5.9) whose probability we would like to bound. Suppose that holds. Then there exists and such that and . In particular, this implies that
Let be a -net of the real interval . In particular, we can choose so that
(5.10) |
for some constant . Here, we have used the assumption that . In particular, there exists such that
By the triangle inequality, this implies that
To conclude, we have shown that
and thus, by the union bound, we have
The claim now follows by the cardinality bound for in (5.10) and Lemma 5.6. ∎
When dealing with incompressible vectors we will need the following corollary.
Corollary 5.8.
There exists constants , such that, for any with , and any , we have
Proof.
5.4. Anti-concentration bounds
In order to handle incompressible vectors, we will need several anti-concentration bounds. The main idea is to use the rate of convergence from the Berry–Esseen Theorem to obtain the estimates. This idea appears to have originated in [35] and has been used previously in many works including [15, 35, 45].
Lemma 5.9 (Small ball probability via Berry–Esseen; Lemma A.6 from [15]).
There exists such that if are independent centered complex-valued random variables, then for all ,
We begin the following anti-concentration bound for sums involving dependent random variables.
Lemma 5.10.
Let be a collection of independent complex-valued random tuples, and assume there exist such that the events
for satisfy
In addition, assume there exists such that
(5.11) |
Then for any , any , any with , any with , and any , we have
where is an absolute constant.
Proof.
The proof is based on the arguments from [15]. Since and , Lemma 5.3 implies the existence of such that and
for all . By conditioning on the random variables for and absorbing their contribution into the constant , it suffices to bound
We now proceed to truncate the random variables for . Indeed, by the Chernoff bound, it follows that
with probability at least . Therefore, taking , it suffices to show
where is the conditional probability given , the -algebra generated by all random variables except , and the event
By centering the random variables (and absorbing the expectations into the constant ), it suffices to bound
We again reduce to the case where we only need to consider a subset of the coordinates of and which are roughly comparable. Indeed, as the random tuples are jointly independent under the probability measure , we can condition on any subset of them (and again absorb their contribution into the constant ); hence, for any subset , we have
(5.12) | ||||
We now choose the subset in a sequence of two steps. First, define
and for set
By construction, partition the index set . Hence, by the pigeonhole principle, there exists such that . Second, we partition the set as follows. Define
and for set
and define
As by assumption, the sets form a partition of . By the pigeonhole principle, there exists such that
(5.13) |
Applying (5.12) to the set , it now suffices to show
(5.14) | ||||
for some absolute constant .
We will apply Lemma 5.9 to obtain (5.14). For , define
Then
by assumption (5.11). Thus, we deduce that
(5.15) |
In addition,
Hence, Lemma 5.9 implies the existence of an absolute constant such that
(5.16) |
We complete the proof by considering two separate cases. First, if , then using (5.13) and (5.15), we obtain
Hence returning to (5.16), we find that
(5.17) |
where is an absolute constant. Similarly, if , then
In this case, we again apply (5.16) to deduce the existence of an absolute constant such that
(5.18) |
Combining (5.17) and (5.18), we obtain the bound (5.14) (with the absolute constant ), and the proof of the lemma is complete. ∎
Lastly, we will need the following technical anti-concentration bound which is similar to Lemma 5.10.
Lemma 5.11.
Let be a collection of independent complex-valued random tuples with the property that has the same distribution as for , and assume there exist such that the events
for satisfy
In addition, let be i.i.d. -valued random variables with , and assume are independent of the random tuples . Then for any , any , any with , and any , we have
where is an absolute constant.
Lemma 5.11 is very similar to Lemma 5.10. The statement of the lemma is somewhat unusual since the hypotheses involve the variables , but the conclusion only involves the random variables . This is to match the assumptions of Theorem 5.1. The proof of Lemma 5.11 presented below follows the same framework as the proof of Lemma 5.10.
Proof of Lemma 5.11.
Since , Lemma 5.3 implies the existence of such that and
for all . By conditioning on the random variables for and absorbing their contribution into the constant , it suffices to bound
We now proceed to truncate the random variables for . Define the events
and observe that is independent of for . By the Chernoff bound, it follows that
with probability at least . Therefore, taking , it suffices to bound
(5.19) |
where is the conditional probability given , the -algebra generated by all random variables except , , , , , and the event
Here, we have exploited the fact that on the event , for , and so all factors of have been replaced by on the right-hand side of (5.19). By centering the random variables (and absorbing the expectations into the constant ), it suffices to bound
We again reduce to the case where we only need to consider a subset of the coordinates of and which are roughly comparable. Indeed, as the random vectors are jointly independent under the probability measure , we can condition on any subset of them (and again absorb their contribution into the constant ); hence, for any subset , we have
(5.20) | ||||
We now choose the subset in a sequence of two steps as was done in the proof of Lemma 5.10. First, define
and for set
By construction, partition the index set . Hence, by the pigeonhole principle, there exists such that . Second, we partition the set as follows. Define
and for set
and define
As by assumption, the sets form a partition of . By the pigeonhole principle, there exists such that
(5.21) |
Applying (5.20) to the set , it now suffices to show
(5.22) | ||||
for some absolute constant .
We will apply Lemma 5.9 to obtain (5.22). For , define
Then
(5.23) | ||||
since and are independent under the measure for . In addition,
Hence, Lemma 5.9 implies the existence of an absolute constant such that
(5.24) |
We complete the proof by considering two separate cases. First, if , then using (5.21) and (5.23), we obtain
Hence returning to (5.24), we find that
(5.25) |
where is an absolute constant. Similarly, if , then
In this case, we again apply (5.24) to deduce the existence of an absolute constant such that
(5.26) |
Combining (5.25) and (5.26), we obtain the bound (5.22) (with the absolute constant ), and the proof of the lemma is complete. ∎
5.5. Incompressible vectors
In order to control the set of incompressible vectors, we will require the following averaging estimate.
Lemma 5.12 (Invertibility via average distance; Lemma A.4 from [15]).
Let be a random matrix taking values in with columns . For any , let . Then, for any ,
Let be the matrix from Theorem 5.1. Let be the columns of and be as in Lemma 5.12. Our main result for controlling the set of incompressible vectors is the following.
Lemma 5.13.
There exists such that for every , any and any ,
The rest of the subsection is devoted to the proof of Lemma 5.13. We complete the proof of Theorem 5.1 in Subsection 5.6. We will also need the following result based on [53, Proposition 5.1] and [29, Statement 2.8].
Lemma 5.14 (Distance problem via bilinear forms).
Let , let denote the columns of , and fix . Let , be the -th row of with the -th entry removed, be with the -th entry removed, and let be the submatrix of formed from removing the -th row and -th column. If is invertible, then
(5.27) |
Proof.
The proof presented below is based on the arguments given in [29, 53]. By permuting the rows and columns, it suffices to assume that . Let denote any normal to the hyperplane . Then
We decompose
where and . Then
(5.28) |
Since is orthogonal to the columns of the matrix , we find
and hence
Returning to (5.28), we have
(5.29) |
In addition,
and so
(5.30) |
Our study of the bilinear form is based on the following general result, which will allow us to introduce some additional independence into the problem to deal with the fact that and are dependent. Similar decoupling techniques have also appeared in [19, 20, 27, 29, 53]. The lemma below is based on [53, Lemma 8.4] for quadratic forms.
Lemma 5.15 (Decoupling lemma).
Let , and let be random vectors in such that is a collection of independent random tuples. Let denote an independent copy of . Then for every subset and , we have
where is a random variable whose value is determined by .
Lemma 5.16 (Lemma 8.5 from [53]).
Let and be independent random vectors, and let be an independent copy of . Let be an event which is determined by the values of and . Then
Proof of Lemma 5.15.
Let be the random vector formed by the tuples , and let be the random vector formed from the tuples . Then and are independent by supposition, and we can apply Lemma 5.16. To this end, let be random vectors in defined by
An application of Lemma 5.16 yields
where the last inequality follows from the triangle inequality. We now note that
where depends only on . Since and , the claim follows. ∎
We now turn to the proof of Lemma 5.13. The arguments presented here follow the general framework of [53, Section 8.3]. Fix ; the arguments and bounds below will all be uniform in . Let be the -th row of with the -th entry removed. Let be the -th column of with the -th entry removed, and let be the matrix formed by removing the -th row and -th column from . In view of Lemma 5.14, it suffices to prove that
(5.31) |
for some constants . Our argument is based on applying Lemma 5.15 to decouple the bilinear form and then applying our anti-concentration bounds from Subsection 5.4 to bound the resulting expressions. We divide the proof of (5.31) into a number of sub-steps.
5.5.1. Step 1: Constructing a random subset
Following [53], we decompose into two random subsets and . Let be i.i.d. -valued random variables, independent of , with , where is a constant defined by
and was previously fixed. We then define . By the Chernoff bound, it follows that
(5.32) |
with probability at least for some constants .
5.5.2. Step 2: Estimating
Lemma 5.17 below will allow us to estimate the denominator appearing on the right-hand side of (5.27). To this end, let be an independent copy of , also independent of .
Lemma 5.17.
There exist constants such that, for any , the random matrix has the following properties with probability at least . If , one has:
-
(i)
for any , with probability at least in ,
-
(ii)
for any , with probability at least in ,
In order to prove the lemma, we will need the following elementary result.
Lemma 5.18 (Sums of dependent random variables; Lemma 8.3 from [53]).
Let be arbitrary non-negative random variables (not necessarily independent), and be non-negative numbers such that
Then, for every , we have
Proof of Lemma 5.17.
By Corollary 5.8 and the union bound, under the assumption , we have
for with probability at least . Here, are the standard basis elements of . Fix a realization of for which this property holds. We will prove that both properties hold with the desired probability for this fixed realization of .
5.5.3. Step 3: Working on the appropriate events
We have one last preparatory step before we can apply the decoupling lemma, Lemma 5.15. In this step, we define the events we will need to work on for the remainder of the proof. To this end, define the events
We note that and since and are sub-matrices of . Consider the random vectors
(5.33) |
It is possible that or , although we will show that these events happen with small probability momentarily.
Let . Consider the event
(5.34) |
By Lemma 5.17, we find
for some constants . In particular, since , it follows that when the event in (5.34) occurs, it must be the case that and are both nonzero. In order to avoid several different cases later in the proof, let us define and as follows. If is nonzero, we take , and if is zero, we define to be a fixed vector in . We define analogously in terms of . It follows that on the event (5.34), we have
(5.35) |
Next, consider the event
(5.36) |
Let us fix an arbitrary realization of and a realization of which satisfies (5.32). We will apply Corollary 5.8 to control the event in (5.36). Indeed, we only need to consider the cases when or . In these cases, it follows that or . Let us suppose this is the case. Then or , where is an orthogonal projection onto those coordinates specified by . Thus, from Corollary 5.8, we deduce that
for some constants . Combining the probabilities above, we conclude that
for some constants .
It follows that there exists a realization of that satisfies (5.32) and such that
We fix such a realization of for the remainder of the proof. Using Fubini’s theorem, we deduce that the random matrix has the following property with probability at least :
Since the event depends only on and not on , it follows that the random matrix has the following property with probability at least : either holds, or
(5.37) |
5.5.4. Step 4: Decoupling
Recall that we are interested in bounding , where
We first observe that
On the event , we have
In addition, if , then . Hence, on the event ,
Thus, we obtain
where
Thus, we find
The last probability is bounded above by by the previous step. We conclude that
We now begin to work with the random vectors (recall that are independent of ). To do so, we will work on a larger probability space which also includes the random vectors . Indeed, computing the probability above on the larger space which includes , we conclude that
For the remainder of the proof, we fix a realization of which satisfies (5.37) and fix an arbitrary realization of . By supposition, both and are independent of . It remains to bound the probability
where
To bound , we apply the decoupling lemma, Lemma 5.15. Indeed, by Lemma 5.15,
where
and is a complex number depending only on , . Using (5.37) (where the conditioning on is no longer required since is now fixed), we find
and hence
where
here, we used the fact that on the event (5.34), the event (5.35) holds.
5.5.5. Step 5: Applying the anti-concentration bounds
Recall that depend only on . In addition, and are independent of these random vectors. Let us fix a realization of the random vectors which satisfy (5.35) and (5.36). This completely determines and ; moreover, is also completely determined. Therefore, we conclude that
In order to bound this first term on the right-hand side, we will apply the anti-concentration bound given in Lemma 5.10. Without loss of generality, let us assume that . Then dividing through by , we find that
and
In view of Lemma 5.10 (where we recall that due to (5.32)), we conclude that
for some constant .
5.5.6. Step 6: Completing the proof
Combining the bounds from the previous steps, we obtain
We now proceed to simplify the expression to obtain (5.31). We still have the freedom to chose ; let us take . In addition, we may assume that the expression
(5.38) |
is less than one as the bound is trivial otherwise. In particular, this implies that . Among others, this means that the error term can be absorbed into terms of the form (5.38) (by increasing the constant if necessary). After some simplification, the bound for obtained in the previous step (with the substitution ) yields
for some constant . This completes the proof of (5.31), and hence the proof of Lemma 5.13 is complete.
5.6. Proof of Theorem 5.1
In this subsection, we complete the the proof of Theorem 5.1. Indeed, for any and any , we have
(5.39) | ||||
due to our decomposition of the unit sphere into compressible and incompressible vectors. It remains to bound each of the terms on the right-hand side.
6. Singular values of and uniform integrability
6.1. Tightness
We begin with a bound on the largest singular values of .
Lemma 6.1.
If Condition C1 holds, there exists such that the following hold.
-
•
For all , there exists such that almost surely
-
•
Almost surely
Proof.
We follow the approach of Lemma 3.1 in [13]. The tightness follows from the moment bound and Markov’s inequality. The moment bound on follows from the bound on and Weyl’s inequality, Lemma A.7. One has from Lemma A.3, for every and thus using the fact for any we can assume . We aim to work with matrices with independent entries, and thus decompose where is strictly lower triangular, and is upper triangular. Note for all , we have by Lemma A.3
We now restrict such that . Thus
We show only
as the proof that
follows in the exact same way.
6.2. Distance from a row and a vector space.
Throughout the rest of this section we assume the atom variables of satisfy Condition C2. The proof of Proposition 3.3 from [13] can be adapted in a straight forward way to get Proposition 6.2 below. We give a brief explanation of the changes to the proof needed for entries that are independent but not necessarily identically distributed.
Proposition 6.2.
Let , and be the -th row of with the -th entry set to zero. There exists depending on such that for all -dimensional subspaces of with , one has
Proof.
Assume is the -th row of with the -th entry set to zero. If is the -th row of with the -th entry set to zero, we have
where . Note the entries of are independent, but have two potentially different distributions, in contrast with [13] where the entries are independent and identically distributed. However, Lemma A.9 can be applied to either distribution. Under Condition C2 the slowly varying function, , in (1.3) is bounded and as for both entries. To adapt the proof of Proposition 3.3 in [13] apply Lemma A.9 to the entries of to get uniform bounds on the truncated moments without assuming the entries are identically distributed. ∎
We now give some results for stable random variables which will be helpful. For , let denote the one-sided positive -stable distribution such that for all ,
(6.1) |
Recall for ,
Thus for all ,
(6.2) |
and if are i.i.d. copies of and are non-negative real numbers then
(6.3) |
Lemma 6.3 (Lemma 3.5 in [13]).
Let and be a random variable such that as for some . Then there exists and such that the random variable dominates stochastically the random variable , where is a Bernoulli random variable, and and are independent.
Lemma 6.4.
Let be the -th row of the matrix , with the -th entry set to zero. Let be numbers such that for some . Let with . Then there exists and a coupling of and such that
for constants .
Proof.
Let and be two independent vectors of i.i.d. Bernoulli random variables given by Lemma 6.3 for with parameter and with parameter respectively. We know from Lemma 6.3 there exists , such that for independent random variables satisfying (6.1) such that for every , stochastically dominates . Then there exists a coupling (see Lemma 2.12 in [32]) such that
Let , and be the event for all . Then define the random variable pointwise on ,
for and on . From (6.3), we see satisfies (6.1) and the distribution of does not depend on . Thus it is sufficient to show there exists such that
Note , and thus . Therefore for ,
where the last bound follows from Hoeffding’s inequality. ∎
We now give another bound on the distance between a row of and a deterministic subspace.
Proposition 6.5.
Take . Let be the -th row of with the -th entry set to zero. There exists an event such that for any -dimensional subspace of with , we have for sufficiently large
where is an absolute constant which does not depend on the choice of row.
Proof.
We follow the approach of the proof of Proposition 3.7 in [13]. The only difference with Proposition 3.7 in [13] is the entries here are independent but not necessarily identically distributed. Assume that is the -th row of with the -th entry set to zero. Note
(6.4) |
where , is the -th basis vector, and . Though the -th entry of is not necessarily zero, inequality (6.4) still holds since the subspace spanned by is contained in . Let denote the set of indices such that . It is a straight forward application of the Chernoff bound to show there exists such that
We begin by showing for any set such that ,
for some event satisfying . Without loss of generality assume with . Let be the orthogonal projection onto the first canonical basis vectors. Let , set
Note . Define
One has
is a mean zero vector under . Note and , so we will work with both as objects in only and not the larger vector space . Let be the orthogonal projection matrix onto in . Note satisfies for sufficiently large
(6.5) |
By construction
(6.6) |
Let . Before beginning note by Lemma A.9 there exists such that for . Thus333We believe there is a small typo in the bound of in the proof of Proposition 3.7 in [13]
Thus
(6.7) |
Let be as in Lemma 6.4. Set and for , consider the event
where . From Lemma 6.4 there exists a coupling of and such that
(6.8) |
for some and some choice of . Since for we have where
and
From Lemma A.9 and (6.5) one has
where if and if . Let be the event that . There exists some such that
From the assumption with we have for some . From here, using the bound (6.2) on the negative second moment of , it is straightforward to show that for every there exists a constant such that
(6.9) |
Set . On , , and therefore
and thus using again the negative second moment bound on ,
(6.10) |
Let be the event . Note using Markov’s inequality and the Cauchy-Schwarz inequality leads to
(6.11) | ||||
Then by (6.7), (6.10), and (6.2)
(6.12) |
By the Cauchy-Schwarz inequality
To conclude take . Then
It is then straightforward to show using (6.8), (6.9), and (6.12)
Take where to complete the proof. ∎
6.3. Application of Theorem 5.1
We now show that Theorem 5.1 can be applied to to bound from below with high probability. The difficulty is connecting the hypothesis on the spectral measure in Condition C2 to the correlation of truncated random variables in the statement of Theorem 5.1.
Theorem 6.6.
For all , there exists such that
It is worth noting can be improved to for some sufficiently small . The proof of Theorem 6.6 will be an application of Theorem 5.1. First we need a bound on the operator norm, and hence the largest singular value, of .
Lemma 6.7.
For every , there exists depending on the distribution of the entries and such that for any and sufficiently large,
Proof.
Since
it is sufficient to bound , for some and sufficiently large. Note
Proof of Theorem 6.6.
Clearly satisfies conditions (i) and (ii) of Theorem 5.1, so it only remains to check condition (iii) before we can apply Theorem 5.1. Let . Since is a collection i.i.d. random tuples we focus on showing for some , satisfies the desired conditions. From the tail bounds on and ,
if and only if is constant on , and hence on any subset of . Thus if for some , then for all . Since is non-constant, there must be some sufficiently large such that is non-constant on . Thus for all sufficiently large. The same argument follows for .
Now assume for all sufficiently large,
Then there exists such that on ,
For , by Lemma A.9
as , for some slowly varying function . We assumed the atom variables satisfy Condition C2 (ii), specifically and thus for some constant as . For , is dominated by for any . Thus on
where is a sequence of complex numbers and
(6.13) |
since is slowly varying by Lemma A.9. If has a bounded subsequence , we shall take the corresponding sequences , and and for simplicity denote all three by and . If not, then we note on
where is bounded, and (6.13) still holds for . Thus we will assume is bounded and we take a subsequence which converges to . Let and be a Borel subset of the unit sphere in such that . Note
(6.14) | ||||
and
(6.15) | ||||
Thus we will consider only
(6.16) |
Define the set as
where is the limit of . Let be such that and . Let be a small open neighborhood of in such that . We will consider the limit
(6.17) |
Before we deal with this limit note that on
(6.18) |
(6.19) |
and
(6.20) |
We aim to show the unit vector in (6.17) is approaching the bad set , which will lead to a contradiction of Condition C2. To this end note by factoring out from (6.19) and (6.20), we get
and
Since , it follows from (6.20) that if , then for some . It then follows that on
and
Thus
(6.21) | ||||
since is a small perturbation of a vector in which is disjoint from . From (6.3), (6.3), and (6.3) we see
Thus for arbitrary , and , a contradiction of Condition C2 (i). Therefore there exists arbitrarily large such that
6.4. Uniform integrability
For we define . We aim to show that is uniformly integrable in probability with respect to , i.e. for all
(6.22) |
From Lemma 6.1 there exists a constant such that with probability 1
From this, the part of the integral in (6.22) over is not a concern. Thus it suffices to prove that for every sequence converging to ,
converges to in probability. By Theorem 6.6 we may, with probability , lower bound by for all . Take to be fixed later. Using the polynomial lower bound for , it follows that it is sufficient to prove that
converges in probability to .
We next aim to show there exists an event such that for some and
and
for . First to see why this implies convergence in probability to zero, note
It follows there exists a sequence tending to zero such that converges to . Hence it is sufficient to prove, given ,
(6.23) |
converges to zero in probability. Using the negative second moment bound on and the concavity of we have
where the last sum converges to zero by a Riemann Sum approximation. Thus, by Markov’s inequality we obtain convergence (given ) of (6.23) in probability to zero.
Now we finish with the construction of such an event . Let be the matrix formed by the first rows of . If are the singular values of , then by Cauchy interlacing
(6.24) |
By the Tao-Vu negative second moment lemma, Lemma A.4, we have
(6.25) |
where is the distance from the -th row of to the subspace spanned by the other rows. Using (6.24) and (6.25) we get
Now let be the distance from the -th row of with its -th entry removed and the subspace spanned by the other rows minus their -th entry. Since we have
(6.26) |
Let be the event that for all , . Since the span of all but 1 row of is at most , we can use Proposition 6.2 to obtain, for sufficiently large ,
for some (after a union bound). Let , then
for some .
We now aim to show the desired negative second moment bound on . Recall from Proposition 6.5 there exists an event independent from the rows of without their -th entry such that and for any subspace with dimension one has
where is the -th row of with the -th entry removed. Thus
for .
By taking the lower bound of on , we get
So for , not dependent on , sufficiently small one has
Thus by (6.26)
The result then follows from the assumption .
6.5. Proof of Theorem 1.6
We have shown that almost surely converges weakly to and that is uniformly integrable in probability with respect to . Thus by Lemma 1.12, converges weakly to some deterministic probability measure in probability.
Appendix A Additional lemmas
A.1. Concentration
Lemma A.1 (McDiarmid’s inequality, Theorem 3.1 in [36]).
Let be independent random variables taking values in respectively. Let be a function with the property that for every there exists a such that
for all , for . Then for any ,
for absolute constants and .
For the following lemma we need a way of breaking up an elliptic random matrix into independent pieces. For a matrix we let the -wedge of be the matrix with entries if either and or and , with otherwise. Note
and for all . Recall the total variation norm of a function is given by
where the supremum runs over all sequences with .
Lemma A.2.
Let be a random matrix with independent wedges. Then for any going to at with and every ,
for absolute constants .
Proof.
If let and be the cumulative distribution functions of and . By the Lidskii inequality (see Theorem 3.3.16 in [33]) for singular values
Assume is smooth. Integrating by parts we get
(A.1) |
Since depends on only finitely many points for any , we can extend the previous inequality to any function of finite total variation.
A.2. Singular value estimates
Lemma A.3 (See [33], Chapter 3).
If and are complex matrices then
for and . In addition,
Lemma A.4 (Tao-Vu Negative Second Moment, [51] Lemma A.4).
If is a full rank complex matrix with rows and , then
Lemma A.5 (Cauchy interlacing, see [33]).
Let be an complex matrix. If is an matrix obtained by deleting rows from , then for every ,
Lemma A.6 (See Remark 1 in [23]).
Let be an matrix and let denote the real part of . If and denote the singular values of and eigenvalues of respectively, then
(A.2) |
for every .
Lemma A.7 (Weyl’s inequality, [54], see also Lemma B.5 in [13]).
For every complex matrix with eigenvalues ordered as one has
for all . Moreover for
Lemma A.8 (Schatten Bound, see proof of Theorem 3.32 in [55]).
Let be an complex matrix with rows . Then for every ,
A.3. Moments of stable distributions
A.4. Proof of Proposition 4.12
From Proposition 4.3 one has
We will consider the point process in the polar form where is a Poisson point process with intensity measure and is a collection of independent identically distributed random variables independent of . We will denote the coordinates of by . In polar coordinates the recursive equation becomes
(A.3) |
where
To complete the proof that the matrix in (4.3) satisfies the distributional equation we need the following lemma, which is essentially Theorem 10.3 in [8].
Lemma A.10.
Let be a Poisson point process with intensity measure for and be a collection of bounded independent and identically distributed random vectors for some probability measure . Then
(A.4) |
where is an -stable random vector with spectral measure , where is the measure on the unit sphere obtained by the image of the measure under the map .
Proof.
Let be a sequence of i.i.d. non-negative random variables such that
(A.5) |
for some slowly varying function , and define the normalizing constants
(A.6) |
From Theorem 10.3 in [8], converges in distribution to . It also follows from Proposition 2.3 that
(A.7) |
as . It then follows from the almost sure uniform summability of the sequence that
(A.8) |
as . ∎
References
- [1] A. Aggarwal, P. Lopatto, and J. Marcinek. Eigenvector statistics of Lévy matrices. The Annals of Probability, 49(4):1778 – 1846, 2021.
- [2] A. Aggarwal, P. Lopatto, and H.-T. Yau. GOE statistics for Lévy matrices. Journal of the European Mathematical Society, 23(11):3707–3800, 2021.
- [3] D. Aldous and J. M. Steele. The objective method: Probabilistic combinatorial optimization and local weak convergence. In Probability on Discrete Structures, volume 110 of Encyclopaedia of Mathematical Sciences, page 1–72. Springer-Verlag, New York, 2004.
- [4] A. Auffinger, G. Ben Arous, and S. Péché. Poisson convergence for the largest eigenvalues of heavy tailed random matrices. Ann. Inst. Henri Poincaré, Probab. Stat., 45(3):589–610, 2009.
- [5] A. Auffinger and S. Tang. Extreme eigenvalues of sparse, heavy tailed random matrices. Stochastic Processes and their Applications, 126(11):3310–3330, Nov 2016.
- [6] B. Basrak, Y. Cho, J. Heiny, and P. Jung. Extreme eigenvalue statistics of m-dependent heavy-tailed matrices. Annales de l’Institut Henri Poincaré, Probabilités et Statistiques, 57(4):2100 – 2127, 2021.
- [7] S. Belinschi, A. Dembo, and A. Guionnet. Spectral measure of heavy tailed band and covariance random matrices. Communications in Mathematical Physics, 289(3):1023–1055, May 2009.
- [8] G. Ben Arous and A. Guionnet. The spectrum of heavy tailed random matrices. Communications in Mathematical Physics, 278(3):715–751, Dec 2007.
- [9] F. Benaych-Georges and A. Guionnet. Central limit theorem for eigenvectors of heavy tailed matrices. Electron. J. Probab., 19:27 pp., 2014.
- [10] R. Bhatia. Matrix analysis, volume 169 of Graduate Texts in Mathematics. Springer-Verlag, New York, 1997.
- [11] G. Biroli, J.-P. Bouchaud, and M. Potters. On the top eigenvalue of heavy-tailed random matrices. Europhysics Letters (EPL), 78(1):10001, Mar 2007.
- [12] C. Bordenave, P. Caputo, and D. Chafaï. Spectrum of large random reversible Markov chains: heavy-tailed weights on the complete graph. The Annals of Probability, 39(4):1544–1590, 2011.
- [13] C. Bordenave, P. Caputo, and D. Chafaï. Spectrum of non-Hermitian heavy tailed random matrices. Communications in Mathematical Physics, 307(2):513–560, Oct. 2011.
- [14] C. Bordenave, P. Caputo, D. Chafaï, and D. Piras. Spectrum of large random markov chains: Heavy-tailed weights on the oriented complete graph. Random Matrices: Theory and Applications, 06(02):1750006, 2017.
- [15] C. Bordenave and D. Chafaï. Around the circular law. Probab. Surveys, 9:1–89, 2012.
- [16] C. Bordenave and A. Guionnet. Localization and delocalization of eigenvectors for heavy-tailed random matrices. Probability Theory and Related Fields, 157(3-4):885–953, Jan 2013.
- [17] C. Bordenave and A. Guionnet. Delocalization at small energy for heavy-tailed random matrices. Communications in Mathematical Physics, 354(1):115–159, May 2017.
- [18] P. Cizeau and J. P. Bouchaud. Theory of Lévy matrices. Phys. Rev. E, 50:1810–1822, Sep 1994.
- [19] K. P. Costello. Bilinear and quadratic variants on the Littlewood-Offord problem. Israel Journal of Mathematics, 194(1):359–394, Mar 2013.
- [20] K. P. Costello, T. Tao, and V. Vu. Random symmetric matrices are almost surely nonsingular. Duke Math. J., 135(2):395–413, Nov 2006.
- [21] Y. Davydov and V. Egorov. On convergence of empirical point processes. Statistics & Probability Letters, 76(17):1836–1844, Nov. 2006.
- [22] R. Durrett. Probability: Theory and Examples. Cambridge Series in Statistical and Probabilistic Mathematics. Cambridge University Press, 4 edition, 2010.
- [23] K. Fan and A. J. Hoffman. Some metric inequalities in the space of matrices. Proceedings of the American Mathematical Society, 6(1):111–116, 1955.
- [24] W. Feller. An introduction to probability theory and its applications. Vol. II. Second edition. John Wiley & Sons Inc., New York, 1971.
- [25] V. Girko. Elliptic law. Theory of Probability & Its Applications, 30(4):677–690, 1986.
- [26] V. Girko. The elliptic law: ten years later I. Random Operators and Stochastic Equations, 3(3):257–302, 1995.
- [27] F. Götze. Asymptotic expansions for bivariate von Mises functionals. Zeitschrift für Wahrscheinlichkeitstheorie und Verwandte Gebiete, 50(3):333–355, Jan 1979.
- [28] F. Götze, A. Naumov, and A. Tikhomirov. On a generalization of the elliptic law for random matrices. Acta Physica Polonica B, 46(9):1737–1745, 2015.
- [29] F. Götze, A. Naumov, and A. Tikhomirov. On minimal singular values of random matrices with correlated entries. Random Matrices: Theory and Applications, 04(02):1550006, 2015.
- [30] E. Gudowska-Nowak, A. Jarosz, M. Nowak, and G. Papp. Towards non-hermitian random Lévy matrices. Acta Physica Polonica B, 38, 01 2007.
- [31] J. Heiny and J. Yao. Limiting distributions for eigenvalues of sample correlation matrices from heavy-tailed populations. Available at arXiv:2003.03857, 2020.
- [32] R. v. d. Hofstad. Random Graphs and Complex Networks, volume 1 of Cambridge Series in Statistical and Probabilistic Mathematics. Cambridge University Press, 2016.
- [33] R. Horn and C. R. Johnson. Topics in matrix analysis. Cambridge University Press.
- [34] P. Jung. Lévy-Khintchine random matrices and the poisson weighted infinite skeleton tree. Transactions of the American Mathematical Society, 370, 02 2014.
- [35] A. E. Litvak, A. Pajor, M. Rudelson, and N. Tomczak-Jaegermann. Smallest singular value of random matrices and geometry of random polytopes. Adv. Math., 195(2):491–523, 2005.
- [36] C. McDiarmid. Concentration. In M. Habib, C. McDiarmid, J. Ramirez-Alfonsin, and B. Reed, editors, Probabilistic Methods for Algorithmic Discrete Mathematics, Algorithms and Combinatorics. Springer-Verlag, New York, 1998.
- [37] C. Monthus. Localization transition in random Lévy matrices: multifractality of eigenvectors in the localized phase and at criticality. Journal of Statistical Mechanics: Theory and Experiment, 2016(9):093304, Sep 2016.
- [38] A. Naumov. Elliptic law for real random matrices. Available at arXiv:1201.1639, 2012.
- [39] H. H. Nguyen and S. O’Rourke. The elliptic law. International Mathematics Research Notices, 2015(17):7620–7689, Oct 2014.
- [40] S. O’Rourke and D. Renfrew. Central limit theorem for linear eigenvalue statistics of elliptic random matrices. Journal of Theoretical Probability, 29(3):1121–1191, Mar 2015.
- [41] S. O’Rourke, D. Renfrew, A. Soshnikov, and V. Vu. Products of independent elliptic random matrices. Journal of Statistical Physics, 160(1):89–119, Apr 2015.
- [42] M. Reed and B. Simon. Methods of modern mathematical physics, volume 1. Academic Press, 1972.
- [43] S. I. Resnick. Extreme Values, Regular Variation and Point Processes. Springer Series in Operations Research and Financial Engineering. Springer-Verlag, New York, 1 edition, 1987.
- [44] S. I. Resnick. Heavy-Tailed Phenomemena. Springer Series in Operations Research and Financial Engineering. Springer-Verlag, New York, 1 edition, 2007.
- [45] M. Rudelson and R. Vershynin. The Littlewood-Offord problem and invertibility of random matrices. Adv. Math., 218(2):600–633, 2008.
- [46] G. Sakovich. A single form for the conditions for attraction to stable laws. Theory of Probability & Its Applications, 1(3):322–325, 1956.
- [47] G. Samorodnitsky and M. S. Taqqu. Stable non-gaussian random processes. Stochastic Modeling. Chapman & Hall, New York, NY, 1994.
- [48] A. Sidorenko. A correlation inequality for bipartite graphs. Graphs and Combinatorics, 9(2):201–204, Jun 1993.
- [49] A. Soshnikov. Poisson statistics for the largest eigenvalues of Wigner random matrices with heavy tails. Electronic Communications in Probability, 9:82–91, 2004.
- [50] T. Tao. Topics in random matrix theory, volume 132 of Graduate Studies in Mathematics. American Mathematical Society, Providence, RI, 2012.
- [51] T. Tao, V. Vu, and M. Krishnapur. Random matrices: Universality of ESDs and the circular law. Ann. Probab., 38(5):2023–2065, 09 2010.
- [52] E. Tarquini, G. Biroli, and M. Tarzia. Level statistics and localization transitions of Lévy matrices. Physical Review Letters, 116(1), Jan 2016.
- [53] R. Vershynin. Invertibility of symmetric random matrices. Random Structures & Algorithms, 44(2):135–182, 2014.
- [54] H. Weyl. Inequalities between the two kinds of eigenvalues of a linear transformation. Proceedings of the National Academy of Sciences, 35(7):408–411, 1949.
- [55] X. Zhan. Matrix inequalities, volume 1790 of Lecture Notes in Mathematics. Springer-Verlag, New York, 2002.