S2GSL: Incorporating Segment to Syntactic Enhanced Graph Structure Learning for Aspect-based Sentiment Analysis
Abstract
Previous graph-based approaches in Aspect-based Sentiment Analysis(ABSA) have demonstrated impressive performance by utilizing graph neural networks and attention mechanisms to learn structures of static dependency trees and dynamic latent trees. However, incorporating both semantic and syntactic information simultaneously within complex global structures can introduce irrelevant contexts and syntactic dependencies during the process of graph structure learning, potentially resulting in inaccurate predictions. In order to address the issues above, we propose S2GSL, incorporating Segment to Syntactic enhanced Graph Structure Learning for ABSA. Specifically, S2GSL is featured with a segment-aware semantic graph learning and a syntax-based latent graph learning enabling the removal of irrelevant contexts and dependencies, respectively. We further propose a self-adaptive aggregation network that facilitates the fusion of two graph learning branches, thereby achieving complementarity across diverse structures. Experimental results on four benchmarks demonstrate the effectiveness of our framework.
S2GSL: Incorporating Segment to Syntactic Enhanced Graph Structure Learning for Aspect-based Sentiment Analysis
Bingfeng Chen1, Qihan Ouyang1, Yongqi Luo1, Boyan Xu1††thanks: Corresponding authors , Ruichu Cai1, Zhifeng Hao1,2 1School of Computer Science, Guangdong University of Technology 2College of Science, Shantou University [email protected] {ouyangqihan0720, lyongqi001, hpakyim, cairuichu}@gmail.com [email protected]
1 Introduction
Aspect-based Sentiment Analysis (ABSA) is a fine-grained sentiment analysis task that aims to recognize the sentiment polarities of multiple aspects within a given sentence. For example, in the sentence "The falafel was rather overcooked and dried but the chicken was fine," the sentiment polarity of the aspect words "falafel" and "chicken" is recognized as negative and positive, respectively. The ABSA task presents a notable challenge in accurately recognizing the sentiment polarity of specific aspect words, particularly when they are influenced by other aspect words with contrasting polarities within the given context.
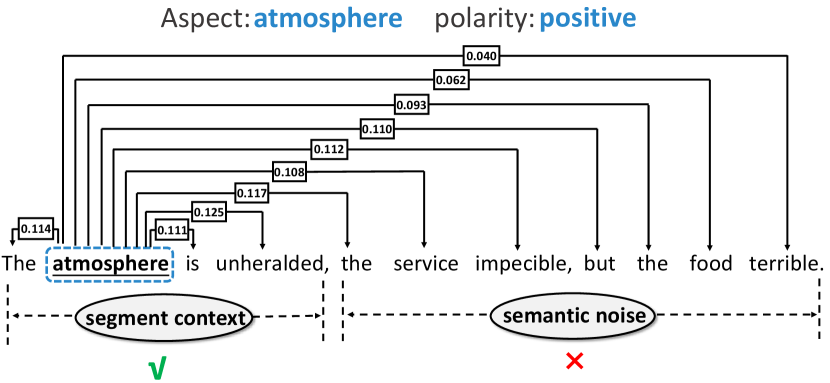
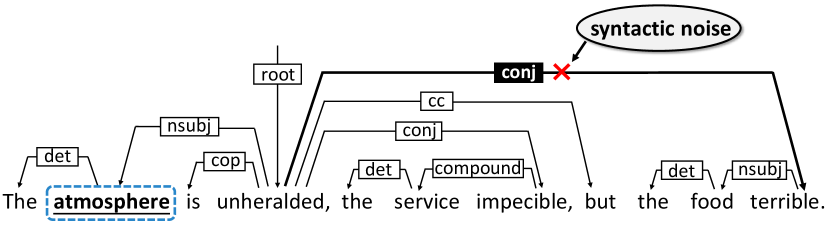
Leading graph-based approaches tackle ABSA tasks by learning either prior static structures and learnable dynamic semantic structures via graph neural networks and attention mechanisms. For instance, Chen et al. (2020) proposed combining external syntactic dependency tree and implicit graph to generate aspect-specific representations using GCN; Li et al. (2021) proposed to construct a SemGCN module and a SynGCN module using an attention mechanism and a syntactic dependency tree.
Although promising results were reported, we observe that existing graph structure learning approaches are still prone to incorrect predictions with hard samples containing multiple aspect words. Existing solutions introduce a heavy structure learning process leading to the following two main limitations: (i) Approaches reliant on attention mechanism are vulnerable to irrelevant context, potentially resulting in misalignment or weak linking. As shown in Figure 1, for the aspect word "atmosphere", except for the clause "The atmosphere is unheralded", the other parts are redundant for judging sentiment polarity, i.e., semantic noise. Negatively influenced by irrelevant context, this results in only weak linking to "atmosphere" and the corresponding opinion word "unheralded". (ii) The global structure of the dependency tree for parsing complex long sentences cannot avoid containing irrelevant dependency information for polarity judgment. As shown in Figure 2, the "conj" relation connecting "unheralded" and "terrible" is the syntactic noise for the aspect “atmosphere". Thus, the key to tackling these limitations is efficiently dividing a complex sentence into multiple local clauses in the graph structure learning process.
In this paper, we propose S2GSL, incorporating Segment to Syntactic enhanced Graph Structure Learning for ABSA. To minimize the negative impact of irrelevant structures, S2GSL introduces constituent trees to decompose the complex structure of the input sentences. To illustrate the role of constituent trees, Figure 3 presents a constituent tree and its third-layer segment matrix. This segment matrix can divide a sentence into three semantically complete paragraphs, which help to facilitate the alignment between each aspect and its corresponding opinion and filter out the contextual information unrelated to the respective paragraph. Specifically, we devise a segment-aware semantic graph(SeSG) branch by using a supervised dynamic local attention on the constituent tree, to learn the local semantic structure of each aspect. Sharing the same idea with leading graph-based approaches, S2GSL has also been designed with a syntax-based latent graph(SyLG) branch that utilizes syntactic dependency labels to enhance the latent tree construction. The difference from past work is that we introduce an attention-based learning mechanism in SyLG that effectively eliminates irrelevant dependency structures. Finally, the Self-adaptive Aggregation Network will fuse the SeSG branch and SyLG branch by cross-attention aggregation mechanism, which considers the complementarity across diverse structures. Our proposed S2GSL framework makes the following contributions:
-
•
In contrast to leading approaches in complex graph structure learning for ABSA, our proposed SeSG branch introduces constituent trees to decompose the global structure learning into multiple localized substructure learning processes.
-
•
In order to reduce dependence on prior structures, our proposed SyLG branch introduces a learnable method to incorporate syntactic dependencies into latent tree construction.
-
•
Within the two graph learning branch, we propose a Self-adaptive Aggregation Network to facilitate interactions and foster complementary across diverse structures.
-
•
We conduct extensive experiments to study the effectiveness of S2GSL. Experiments on four benchmarks demonstrate S2GSL outperforms the baselines. Additionally, the source code and preprocessed datasets used in our work are provided on GitHub111https://github.com/ouy7han/S2GSL.
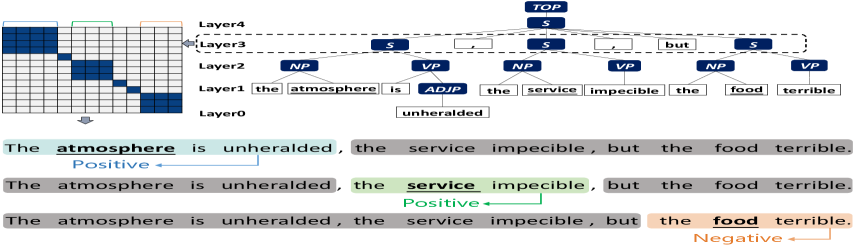
2 Proposed S2GSL
The overall architecture of S2GSL is shown in Figure 4 which is mainly composed of four modules: Context Encoding Module, Segment-aware Semantic Graph Learning(SeSG), Syntax-based Latent Graph Learning(SyLG), and Self-adaptive Aggregation Module. Next, components of S2GSL will be introduced separately in the rest of the sections.
2.1 Context Encoding Module
Given a sentence of words = , where the aspect = , we use the pre-trained language model BERT Devlin et al. (2019) as sentence encoder to extract contextual representations. For the BERT encoder, we follow BERT-SPC
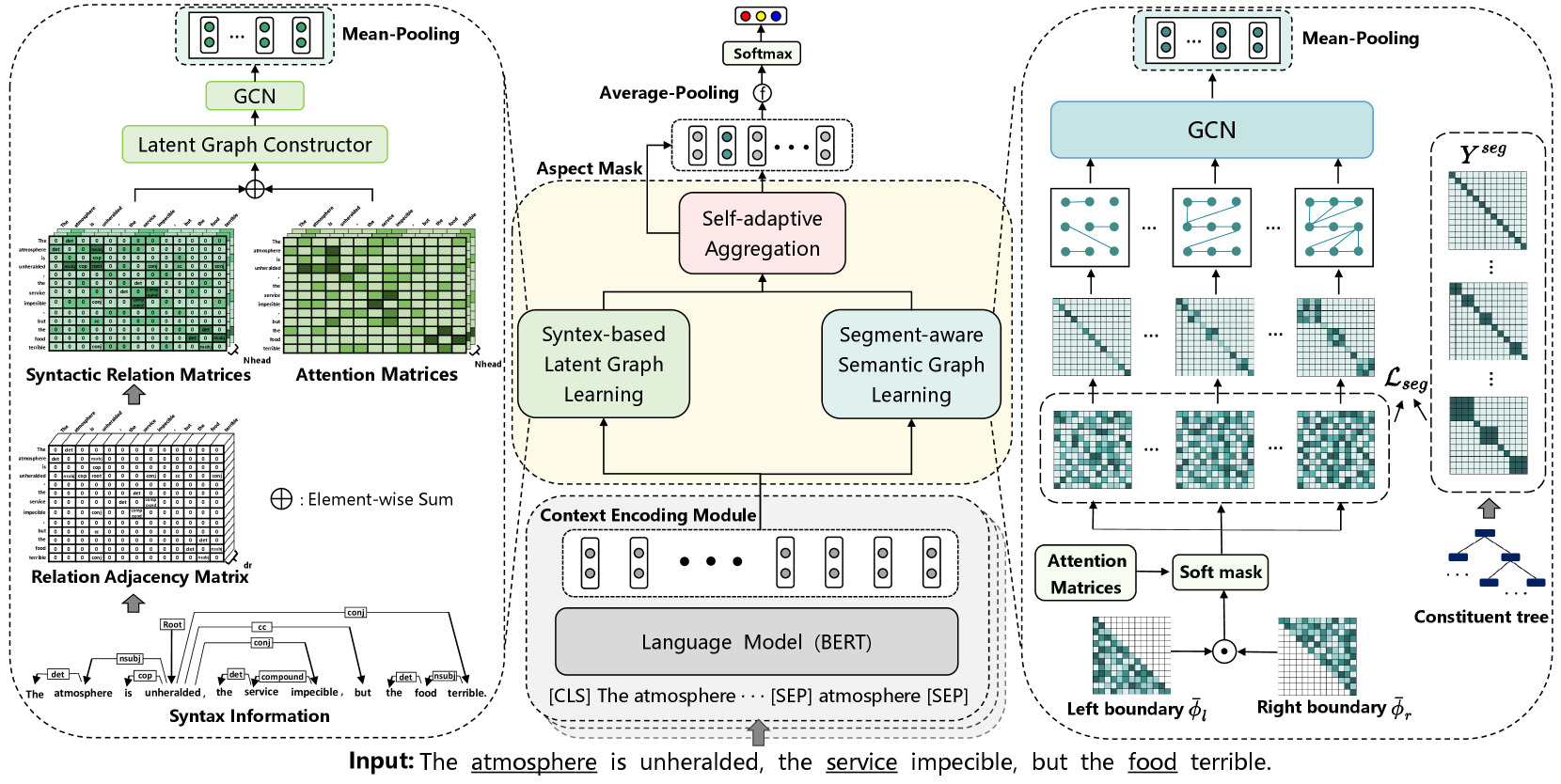
Song et al. (2019) to construct a BERT-based sequence = ([CLS] [SEP] [SEP]), if there are multiple aspects in the sentence, we would construct multiple inputs in the format of . Then the output representation = {} is obtained, where denotes the dimension of the representation and the denotes "context".
2.2 Segment-aware Semantic Graph Learning
Since aspects are vulnerable to irrelevant context, inspired by recent workNguyen et al. (2020); Shang et al. (2021), we use an end-to-end trainable soft masking dynamic local attention mechanism to construct a SeSG branch aiming to align each aspect and its corresponding opinion.
Attention Segment Masking Matrix We first generate the word-level attention segments for each sentence by training left and right boundary soft masking matrices , the formulations are calculated as below:
(1) |
(2) |
(3) |
where ==, is the element-wise product,and are trainable parameters. Notably, a mask matrix is introduced to ensure that the left boundary position and the right boundary position generated at position satisfy .
The attention segment masking matrix can be obtained by compositing the left and right boundary soft masking matrices and :
(4) |
where refers to the upper-triangular matrix.
Then we combine the attention segment masking matrix with the multi-head attention matrices to enable the model to more focus on the semantically relevant contextual information around each word:
(5) |
where , are the trainable parameters, is a multi-head attention matrix with the number of , where corresponds to the number of layers in the constituent tree.
Supervised Constraint In the absence of supervised signal, dynamic local attention may not be able to effectively comprehend the semantically complete segment information around each word, so we further introduce segment-supervised signal to facilitate the learning of dynamic local attention. Specifically, we use the binary cross-entropy loss to represent the distinction between the attention matrix and the segment-supervised signal :
(6) |
(7) |
where represents the sigmoid function, = 1 indicates the -th word and the -th word belong to the same segment, refers to the segment-supervised signal at each layer of the constituent tree.
To effectively learn the representation of each word, we utilize graph convolutional network Kipf and Welling (2017) to extract the segment-aware semantic features = { , , …, }, which is formulated as below:
(8) |
where represents the -th GCN output, is the initial input, denotes a nonlinear activation function, and and are the trainable parameters.
2.3 Syntax-based Latent Graph Learning
Sharing the same idea with past workTang et al. (2022), we also adopt syntactic dependency labels to enhance the latent tree construct. The difference from the past work is that we introduce an attention mechanism in the latent tree to effectively eliminate irrelevant dependency structures and construct a SyLG module.
Syntactically Enhanced Weight Matrix To leverage dependency label information, we first use an off-the-shelf toolkit to obtain dependency information, then we utilize this information to generate a dependency type matrix , where represents the types of dependency between and Tian et al. (2021). Subsequently, we embed each dependency type into the vector , and finally obtain the relational adjacency matrix , where refers to the embedding vector of dependency type between the -th word and the -th word. If and are not connected, we assign a "0" embedding vector to .
In order to induce a syntax-based latent tree, we need to generate a syntactically enhanced weight matrix. Specifically, we first use multi-head self-attention mechanism to compute a weight matrix . Then, we transform the relation adjacency matrix into a syntactic relation weight matrix through a linear transformation, which has the same number of heads as . Finally, the syntactically enhanced weight matrix can be obtained by summing and :
(9) |
(10) |
(11) |
where is the attention score matrix of the -th head, is the weight matrix for the linear transformation.
Syntax-based Latent Tree Construction Considering as the initial weight matrix, we follow Zhou et al. (2021) to generate a syntax-based latent tree. We firstly define a variant of the Laplacian matrix for the syntax-based latent tree:
(12) |
where is the non-normalized score that the -th node is selected as the root node, can be used to simplify the computation of weight sums. Therefore, the marginal probability of the syntax-based latent tree can be computed using :
(13) | ||||
where is Kronecker delta, can be regarded as the adjacency matrix of the syntax-based latent tree.
We employ a root constraint strategy Zhou et al. (2021) to direct the root node towards the aspect word:
(14) |
where denotes the probability of the -th word being the root node, and {0, 1} indicates whether the -th word is an aspect word.
Similar to the SeSG, we utilize graph convolutional network to extract the syntax-based latent Graph features = { , , …, }, which is formulated as below:
(15) |
where denotes the -th layer of GCN output, is the initial input, and are the trainable parameters.
2.4 Self-adaptive Aggregation Module
Considering the complementarity between SeSG and SyLG, we design a self-adaptive aggregation
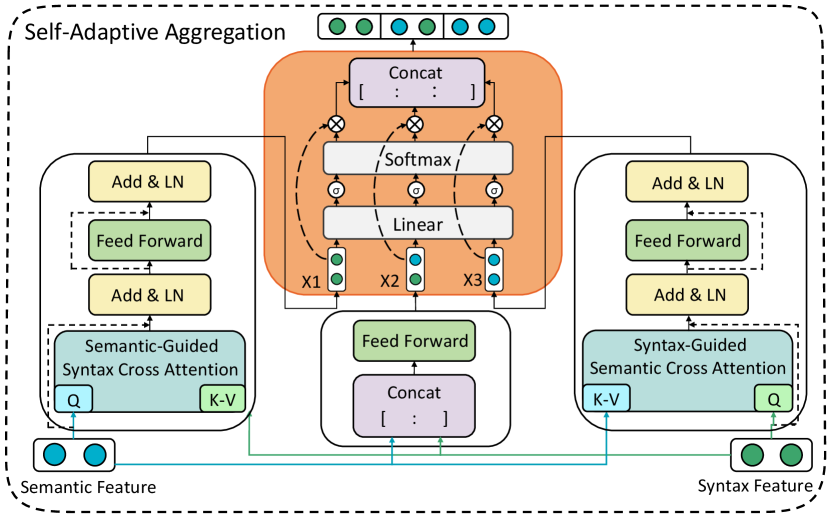
module, as shown in Figure 5, to realize their interaction. Specifically, this module consists of three streams, with two of them extended from the traditional Transformer Vaswani et al. (2017). These two streams can combine information from SeSG and SyLG and ultimately obtain semantic-guided syntax representations and syntax-guided semantic representations :
(16) | ||||
(17) |
(18) | ||||
(19) |
where , denotes multi-head attention, refers to layer normalization, and represents the feed-forward neural network.
To avoid bias towards specific module information, we introduce an additional channel to balance the information between SeSG and SyLG. The specific approach is as follows:
(20) |
where , represents the concatenation function.
Considering the different roles of the various module outputs, we assign different weights to these outputs to allow the model to more focus on the important module. Technically, given the input features . The weight of each module is calculated by the following equation:
(21) |
(22) |
where , , , and are the trainable parameters.The final output feature is generated as follows:
(23) |
where , with = 3.
2.5 Training
We use average pooling at the final aspect nodes of to obtain the aspect representation . Then, the sentiment probability distribution is calculated using a linear layer with a softmax function:
(24) |
where (,) represents the sentence-aspect pair. Our training objective is to minimize the following objective function:
(25) |
where denotes all trainable parameters of the model, and are hyper-parameters, and is the standard cross-entropy loss function:
(26) |
where contains all sentence-aspect pairs and is the collection of different sentiment polarities.
3 Experiments
3.1 Datasets
We conduct experiments on four public datasets. The Restaurant and Laptop reviews are from SemEval 2014 Task 4 Pontiki et al. (2014). The Twitter dataset is a collection of tweets Dong et al. (2014). The MAMS dataset is consisted of sentences with multiple aspectsJiang et al. (2019). Each aspect in the sentence is labeled with one of the three sentiment polarities: positive, neutral, and negative. The statistics for the four datasets are shown in Table 1.
Dataset | #Positive | #Negative | #Neutral | |||
---|---|---|---|---|---|---|
Train | Test | Train | Test | Train | Test | |
Laptop | 976 | 337 | 851 | 128 | 455 | 167 |
Restaurant | 2164 | 727 | 807 | 196 | 637 | 196 |
1507 | 172 | 1528 | 169 | 3016 | 336 | |
MAMS | 3380 | 400 | 2764 | 329 | 5042 | 607 |
3.2 Implementation Details
The Stanford parser222https://stanfordnlp.github.io/CoreNLP/ Manning et al. (2014) is used to obtain syntactic dependencies. Specifically, we use CRF constituency parser Zhang et al. (2020) to obtain the constituent tree. We use the bert-base-uncase333https://github.com/huggingface/transformers model as our context encoder. The model training is conducted using the Adam optimizer with a learning rate of and L2 regularization of . The GCN layers of SeSG and SyLG are set to 3. Our model is trained in 20 epochs with a batch size of 16. The hyper-parameters and for the four datasets are (0.1,0.5), (0.1,0.45), (0.35,0.3) and (0.4,0.75). All experiments are conducted on an NVIDIA 3090 GPU. The model with the highest accuracy or F1 score among all evaluation results is selected as the final model.
Model | Laptop | Restaurant | MAMS | |||||
Accuracy | Macro-F1 | Accuracy | Macro-F1 | Accuracy | Macro-F1 | Accuracy | Macro-F1 | |
RAM Chen et al. (2017) | 74.49 | 71.35 | 80.23 | 70.80 | 69.36 | 67.30 | - | |
MGAN Fan et al. (2018) | 75.39 | 72.47 | 81.25 | 71.94 | 72.54 | 70.81 | - | |
R-GAT Wang et al. (2020) | 78.21 | 74.07 | 86.60 | 81.35 | 76.15 | 74.88 | 84.52 | 83.74 |
KumaGCN Chen et al. (2020) | 81.98 | 78.81 | 86.43 | 80.30 | 77.89 | 77.03 | - | |
ACLT Zhou et al. (2021) | 79.68 | 75.83 | 85.71 | 78.44 | 75.48 | 74.51 | - | |
T-GCN Tian et al. (2021) | 80.88 | 77.03 | 86.16 | 79.95 | 76.45 | 75.25 | 83.38 | 82.77 |
DualGCN Li et al. (2021) | 81.80 | 78.10 | 87.13 | 81.16 | 77.40 | 76.02 | - | |
SSEGCN Zhang et al. (2022) | 81.01 | 77.96 | 87.31 | 81.09 | 77.40 | 76.02 | - | |
dotGCN Chen et al. (2022) | 81.03 | 78.10 | 86.16 | 80.49 | 78.11 | 77.00 | 84.95 | 84.44 |
MGFN Tang et al. (2022) | 81.83 | 78.26 | 87.31 | 82.37 | 78.29 | 77.27 | - | |
TF-BERT(dec) Zhang et al. (2023) | 81.49 | 78.30 | 86.95 | 81.43 | 77.84 | 76.23 | - | |
S2GSL(Ours) | 82.46 | 79.07 | 87.31 | 82.84 | 77.84 | 77.11 | 85.17 | 84.74 |
Model | Laptop | Restaurant | MAMS | |||||
Accuracy | Macro-F1 | Accuracy | Macro-F1 | Accuracy | Macro-F1 | Accuracy | Macro-F1 | |
S2GSL(Ours) | 82.46 | 79.07 | 87.31 | 82.84 | 77.84 | 77.11 | 85.17 | 84.74 |
w/o SyLG | 80.41 | 77.39 | 86.50 | 80.22 | 75.92 | 74.87 | 83.71 | 83.23 |
w/o SeSG | 79.46 | 76.33 | 86.10 | 79.66 | 76.51 | 75.23 | 83.42 | 82.76 |
w/o Self-Adaptive Aggregation | 80.88 | 77.07 | 86.32 | 80.08 | 76.07 | 75.54 | 83.60 | 83.05 |
3.3 Baselines
We compare our S2GSL with some mainstream and lasted models in ABSA, including Attention-based methods: RAM Chen et al. (2017), MGAN Fan et al. (2018). Syntactic-based methods: R-GATWang et al. (2020),T-GCNTian et al. (2021). Latent-graph methods: ACLT Zhou et al. (2021), dotGCN Chen et al. (2022). Multi-graph combined methods: KumaGCN Chen et al. (2020), DualGCN Li et al. (2021), SSEGCNZhang et al. (2022) MGFN Tang et al. (2022). Other method: TF-BERT Zhang et al. (2023).
3.4 Overall Performance
All baseline results on four datasets are shown in Table 2, we can find that our S2GSL outperforms all baselines on Laptop, Restaurant, and MAMS datasets. We got the second best on the Twitter dataset, reaching comparable with MGFN. We guess it is because the sentence structure of Twitter dataset is more complex than the other three datasets, basically samples containing only single aspect words, which cannot reflect the advantages of S2GSL. In contrast, the other three datasets, laptop, restaurant, and MAMS contain samples of multiple aspect words. The effect enhancement in Laptop, Restaurant, and MAMS effectively supports that S2GSL gets better sentiment recognition in the case of having multiple aspect words. Additionally, we conduct a parameter comparison between S2GSL and the GCN-based baseline methods. Notably, the number of parameters in S2GSL is comparable (detailed results can be found in A.2). These results demonstrate that S2GSL exhibits superior performance while incurring the same computational overhead.
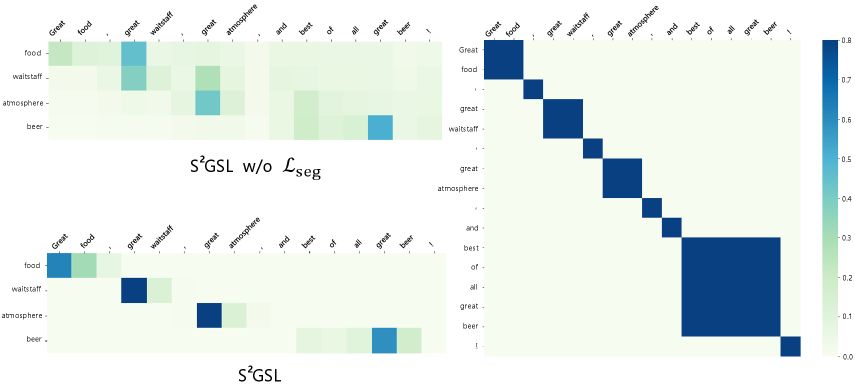
3.5 Ablation Study
We conduct ablation experiments to further investigate the effects of different modules, shown in Table 3. Excluding the syntax-based latent graph learning (w/o SyLG) results in a decrease in the model’s performance, which demonstrates the importance of the ability to adaptively capture syntactic relationships between words. We remove the segment-aware semantic graph learning module (w/o SeSG). Compared to the Twitter dataset, the performance of the Restaurant, Laptop and MAMS datasets decreases significantly, which is due to the fact that the sentence structures on these three datasets are more formal and each sentence can be constructed with multiple subordinate clauses. w/o Self-Adaptive Aggregation refers to the SyLG and SeSG modules cannot interact with each other, leading to a drop in performance on all four datasets. The study reveals that both SyLG and SeSG branches are crucial for handling complex sentences in all datasets, as removing either component leads to a noticeable drop in performance. However, compared to the other three datasets, the performance of the SeSG module on the Twitter dataset is not particularly significant, since each sentence on Twitter contains only one aspect word. This difference underscores the adaptability and effectiveness of S2GSL in varying complexity levels across datasets.
4 Discuss and Analysis
4.1 Effect of Dynamic Local Attention
To demonstrate the effectiveness of supervised dynamic local attention, we visualize the attention weight of all aspects in a sentence, as shown in Figure 6. We can find that in the model without the constraints of segment-supervised signal (S2GSL w/o ), the aspect "food" incorrectly assigns a higher attention weight to the opinion "great" of "waitstaff". In contrast, attention weights of each


aspect word constrained by the supervised signals are concentrated in its semantically coherent segment and each aspect assigns a higher attention weight to its corresponding opinion, which helps avoid the influence of noisy information.
4.2 Effect of Syntactic Dependency Label
To investigate the validity of syntactic dependency label information, we analyzed the weight change of each aspect word assigned to the corresponding opinion word in the latent tree. Figure 7(b) shows the weight changes of the aspect words "appetizers" and "service" assigned to the corresponding opinion "ok" and "slow". As can be seen from the figure, their respective weights have increased by 0.061 and 0.055. This is because in most cases, each aspect is connected to its corresponding opinion through the same syntactic dependency label, such as the "nsubj" label in Figure 7(a). The above analysis indicates that dependency labels can better capture the relationship between aspects and their corresponding opinions.
4.3 Effects of different fusion strategies
To validate the effectiveness of our proposed self-adaptive aggregation module, we compare it with several typical information fusion strategies: "concat", "sum" and "gate". As shown in Figure 8, we can find that "gate" outperforms better than "concat" and "sum" on all datasets. Furthermore, "con-
Model | Laptop14 | Restaurant14 |
---|---|---|
F1 | F1 | |
5-shot ICL Han et al. (2023) | 76.76 | 81.85 |
Prompt-setting 1 | 75.62 | 79.48 |
Prompt-setting 2 | 77.02 | 81.38 |
Prompt-setting 3 | 77.91 | 82.14 |
S2GSL(Ours) | 79.09 | 82.84 |
cat" performs better than "sum" on the laptop and restaurant, while "sum" performs better on Twitter. These results provide evidence that direct fusion strategies (e.g., concat and sum) are sub-optimal. In contrast, our proposed fusion module achieved the best performance on all datasets, which proves that our fusion module adaptively fuses the respective information in a multi-stream manner, which can fully utilize the complementarities between each stream.
4.4 Experiments With ChatGPT
ChatGPT OpenAI (2023a), powered by GPT-3.5 and GPT-4, can achieve significant zero-shot and few-shot in-context learning (ICL) Brown et al. (2020b) performance on unseen tasks, even without any parameter updates.
In this section, we investigate the performance of ChatGPT on ABSA tasks and its ability for fine-grained understanding of segmental context. We experimented with 3 different prompts and their settings as detailed in A.1. We also compared our results with Han et al. (2023), who investigated the performance of ChatGPT on various information extraction tasks, including ABSA. All results are shown in table 4. From the experiment, we find that when we prompt ChatGPT with some instructions (single aspect and multi-aspect sentences) can better improve the performance, but its best results are still not as good as our model. This situation suggests that ChatGPT possesses the ability to understand fine-grained segmental information to some extent. Perhaps there are ways to better harness this ability, such as incorporating constituent trees and dynamic local attention mechanisms as described in this paper (refer A.1 for details).
4.5 Impact of Constituent Tree Layer Number
To investigate the impact of different layer numbers of the constituent tree, we evaluate the performance of the model with 2 to 5 constituent tree layers on three different datasets. As shown in Figure 9, the best performance of the model is achieved when the number of layers of the constituent tree is 4. When the layer numbers of the constituent tree are lower than 4, the information from the constituent tree cannot fully cover the entire sentence, resulting in the model not being able to fully learn the complete segment information of a sentence. When the layer numbers are greater than 4, the model will repetitively learn redundant segment information, resulting in a decrease in model performance.
4.6 Case Study
We conduct a case study with a few examples, shown in Table 5. The first sentence only has one aspect word, so all models can easily determine the sentiment polarity of the aspect correctly. For the second comparative type of sentence, there is a certain syntactic dependency between the aspect "hamburger with special sauce" and the aspect "big mac", and the SeSG, which lacks syntactic information, cannot handle this type of sentence well. The last cases contain multiple aspects and opinions. DualGCN and SyLG, which lack segment structure awareness, cannot focus on local information around aspects, resulting in incorrect judgments. Our S2GSL correctly predicts all samples, indicating that it effectively considers the complementarity between segment structures and syntax correlations of a sentence.
5 Related Work
With the rapid development of ABSA, current research can be broadly divided into three main categories attention-based methods, syntactic-based methods, and multi-graph combined methods.
Attention-based methods Recently, various attention mechanisms have been proposed to implicitly construct the semantic relationships between aspects and their context. Wang et al. (2016); Tang et al. (2016); Ma et al. (2017); Chen et al. (2017); Gu et al. (2018); Fan et al. (2018); Hu et al. (2019); Tan et al. (2019). For instance, Wang et al. (2016) proposed an attention-based Long Short-Term Memory (LSTM) network for aspect-based sentiment classification. Ma et al. (2017) proposed an interactive attention network that can model the connection between the target aspect and the context simultaneously. Hu et al. (2019) propose orthogonal regularization and sparse regularization so that the attention weights of multiple aspects can focus
on different parts of a sentence.
Syntactic-based methods Several studies have explicitly used syntactic knowledge to explicitly build connections between aspects and opinions.Dong et al. (2014); Zhang et al. (2019); Sun et al. (2019); Huang and Carley (2019); Zheng et al. (2020); Wang et al. (2020); Zhou et al. (2021). Wang et al. (2020) reshape the conventional dependency tree with manual rules so that the root node of the dependency tree points to the aspect word. Zhou et al. (2021) constructed a task-oriented latent tree in an end-to-end fashion.
Multi-graph combined methods With the rapid growth of Graph Convolutional Neural Networks, some studies have explored the combination of different types of graphs for ABSA. For example, Chen et al. (2020) used a gating mechanism to combine a dependency graph and a latent graph to generate task-oriented representations. Li et al. (2021) constructed two graph convolutional neural networks using dependency tree and attention mechanism. Tang et al. (2022) by constructing a latent graph and a semantic graph to effectively capture the interaction between aspects and distant opinions. Liang et al. (2022) proposes to simultaneously utilize constituent tree and dependency tree of a sentence to model the sentiment relations between each aspect and its context.
However, these methods have primarily relied on the global graph structure learning process, which tends to introduce irrelevant contextual information and syntactic dependency unrelated to specific aspects. Our proposed method of using two graph branches can effectively align each aspect word with its corresponding opinion word.
Sentences | DualGCN | SyLG | SeSG | S2GSL | ||
---|---|---|---|---|---|---|
1. Much more reasonably too! | (P) | (P) | (P) | (P) | ||
|
(P,O) | (P,N) | (P,P) | (P,N) | ||
|
(P,N,P) | (P,P,P) | (P,O,P) | (P,O,P) |
6 Conclusion
In this paper, we propose an S2GSL model to tackle global structures that will introduce irrelevant contexts and syntactic dependencies during the process of graph structure learning. We propose a SeSG branch that decomposes the ABSA complex graph structure learning problem into multiple local sub-structure learning processes by utilizing constituent trees. Moreover, we propose a SyLG branch, a more learnable method to introduce syntactic dependencies into latent tree construction. Finally, we devise a Self-adaptive Aggregation Network to realize the interaction between two graph branches, achieving complementarity across diverse structures. Experiments on four benchmarks demonstrate S2GSL outperforms the baselines.
Limitations
S2GSL framework constructs different branches for syntactic and semantic structures which cannot encompass diverse structures in a unified graph modeling process. Therefore, the S2GSL framework further devises an adaptive aggregation to fuse diverse structural information.
Acknowledgements
This research was supported in part by the National Science and Technology Major Project (2021ZD0111501), the National Science Fund for Excellent Young Scholars (62122022), the Natural Science Foundation of China (62206064), the Guangzhou Basic and Applied Basic Research Foundation (2024A04J4384), the major key project of PCL (PCL2021A12), the Guangdong Basic and Applied Basic Research Foundation (2023B1515120020), and the Jihua laboratory scientific project (X210101UZ210).
References
- Brown et al. (2020a) Tom Brown, Benjamin Mann, Nick Ryder, Melanie Subbiah, Jared D Kaplan, Prafulla Dhariwal, Arvind Neelakantan, Pranav Shyam, Girish Sastry, Amanda Askell, Sandhini Agarwal, Ariel Herbert-Voss, Gretchen Krueger, Tom Henighan, Rewon Child, Aditya Ramesh, Daniel Ziegler, Jeffrey Wu, Clemens Winter, Chris Hesse, Mark Chen, Eric Sigler, Mateusz Litwin, Scott Gray, Benjamin Chess, Jack Clark, Christopher Berner, Sam McCandlish, Alec Radford, Ilya Sutskever, and Dario Amodei. 2020a. Language models are few-shot learners. In Advances in Neural Information Processing Systems, volume 33, pages 1877–1901. Curran Associates, Inc.
- Brown et al. (2020b) Tom B. Brown, Benjamin Mann, Nick Ryder, Melanie Subbiah, Jared Kaplan, Prafulla Dhariwal, Arvind Neelakantan, Pranav Shyam, Girish Sastry, Amanda Askell, Sandhini Agarwal, Ariel Herbert-Voss, Gretchen Krueger, T. J. Henighan, Rewon Child, Aditya Ramesh, Daniel M. Ziegler, Jeff Wu, Clemens Winter, Christopher Hesse, Mark Chen, Eric Sigler, Mateusz Litwin, Scott Gray, Benjamin Chess, Jack Clark, Christopher Berner, Sam McCandlish, Alec Radford, Ilya Sutskever, and Dario Amodei. 2020b. Language models are few-shot learners. ArXiv, abs/2005.14165.
- Chen et al. (2022) Chenhua Chen, Zhiyang Teng, Zhongqing Wang, and Yue Zhang. 2022. Discrete opinion tree induction for aspect-based sentiment analysis. In Proceedings of the 60th Annual Meeting of the Association for Computational Linguistics (Volume 1: Long Papers), pages 2051–2064, Dublin, Ireland. Association for Computational Linguistics.
- Chen et al. (2020) Chenhua Chen, Zhiyang Teng, and Yue Zhang. 2020. Inducing target-specific latent structures for aspect sentiment classification. In Proceedings of the 2020 Conference on Empirical Methods in Natural Language Processing (EMNLP), pages 5596–5607, Online. Association for Computational Linguistics.
- Chen et al. (2017) Peng Chen, Zhongqian Sun, Lidong Bing, and Wei Yang. 2017. Recurrent attention network on memory for aspect sentiment analysis. In Proceedings of the 2017 Conference on Empirical Methods in Natural Language Processing, pages 452–461, Copenhagen, Denmark. Association for Computational Linguistics.
- Chowdhery et al. (2022) Aakanksha Chowdhery, Sharan Narang, Jacob Devlin, Maarten Bosma, Gaurav Mishra, Adam Roberts, Paul Barham, Hyung Won Chung, Charles Sutton, Sebastian Gehrmann, Parker Schuh, Kensen Shi, Sasha Tsvyashchenko, Joshua Maynez, Abhishek Rao, Parker Barnes, Yi Tay, Noam M. Shazeer, Vinodkumar Prabhakaran, Emily Reif, Nan Du, Benton C. Hutchinson, Reiner Pope, James Bradbury, Jacob Austin, Michael Isard, Guy Gur-Ari, Pengcheng Yin, Toju Duke, Anselm Levskaya, Sanjay Ghemawat, Sunipa Dev, Henryk Michalewski, Xavier García, Vedant Misra, Kevin Robinson, Liam Fedus, Denny Zhou, Daphne Ippolito, David Luan, Hyeontaek Lim, Barret Zoph, Alexander Spiridonov, Ryan Sepassi, David Dohan, Shivani Agrawal, Mark Omernick, Andrew M. Dai, Thanumalayan Sankaranarayana Pillai, Marie Pellat, Aitor Lewkowycz, Erica Moreira, Rewon Child, Oleksandr Polozov, Katherine Lee, Zongwei Zhou, Xuezhi Wang, Brennan Saeta, Mark Díaz, Orhan Firat, Michele Catasta, Jason Wei, Kathleen S. Meier-Hellstern, Douglas Eck, Jeff Dean, Slav Petrov, and Noah Fiedel. 2022. Palm: Scaling language modeling with pathways. J. Mach. Learn. Res., 24:240:1–240:113.
- Devlin et al. (2019) Jacob Devlin, Ming-Wei Chang, Kenton Lee, and Kristina Toutanova. 2019. BERT: Pre-training of deep bidirectional transformers for language understanding. In Proceedings of the 2019 Conference of the North American Chapter of the Association for Computational Linguistics: Human Language Technologies, Volume 1 (Long and Short Papers), pages 4171–4186, Minneapolis, Minnesota. Association for Computational Linguistics.
- Dong et al. (2014) Li Dong, Furu Wei, Chuanqi Tan, Duyu Tang, Ming Zhou, and Ke Xu. 2014. Adaptive recursive neural network for target-dependent Twitter sentiment classification. In Proceedings of the 52nd Annual Meeting of the Association for Computational Linguistics (Volume 2: Short Papers), pages 49–54, Baltimore, Maryland. Association for Computational Linguistics.
- Fan et al. (2018) Feifan Fan, Yansong Feng, and Dongyan Zhao. 2018. Multi-grained attention network for aspect-level sentiment classification. In Proceedings of the 2018 Conference on Empirical Methods in Natural Language Processing, pages 3433–3442, Brussels, Belgium. Association for Computational Linguistics.
- Gu et al. (2018) Shuqin Gu, Lipeng Zhang, Yuexian Hou, and Yin Song. 2018. A position-aware bidirectional attention network for aspect-level sentiment analysis. In Proceedings of the 27th International Conference on Computational Linguistics, pages 774–784, Santa Fe, New Mexico, USA. Association for Computational Linguistics.
- Han et al. (2023) Ridong Han, Tao Peng, Chaohao Yang, Benyou Wang, Lu Liu, and Xiang Wan. 2023. Is information extraction solved by chatgpt? an analysis of performance, evaluation criteria, robustness and errors. ArXiv, abs/2305.14450.
- Hu et al. (2019) Mengting Hu, Shiwan Zhao, Li Zhang, Keke Cai, Zhong Su, Renhong Cheng, and Xiaowei Shen. 2019. CAN: Constrained attention networks for multi-aspect sentiment analysis. In Proceedings of the 2019 Conference on Empirical Methods in Natural Language Processing and the 9th International Joint Conference on Natural Language Processing (EMNLP-IJCNLP), pages 4601–4610, Hong Kong, China. Association for Computational Linguistics.
- Huang and Carley (2019) Binxuan Huang and Kathleen Carley. 2019. Syntax-aware aspect level sentiment classification with graph attention networks. In Proceedings of the 2019 Conference on Empirical Methods in Natural Language Processing and the 9th International Joint Conference on Natural Language Processing (EMNLP-IJCNLP), pages 5469–5477, Hong Kong, China. Association for Computational Linguistics.
- Jiang et al. (2019) Qingnan Jiang, Lei Chen, Ruifeng Xu, Xiang Ao, and Min Yang. 2019. A challenge dataset and effective models for aspect-based sentiment analysis. In Proceedings of the 2019 Conference on Empirical Methods in Natural Language Processing and the 9th International Joint Conference on Natural Language Processing (EMNLP-IJCNLP), pages 6280–6285, Hong Kong, China. Association for Computational Linguistics.
- Kipf and Welling (2017) Thomas N. Kipf and Max Welling. 2017. Semi-supervised classification with graph convolutional networks. In International Conference on Learning Representations.
- Li et al. (2021) Ruifan Li, Hao Chen, Fangxiang Feng, Zhanyu Ma, Xiaojie Wang, and Eduard Hovy. 2021. Dual graph convolutional networks for aspect-based sentiment analysis. In Proceedings of the 59th Annual Meeting of the Association for Computational Linguistics and the 11th International Joint Conference on Natural Language Processing (Volume 1: Long Papers), pages 6319–6329, Online. Association for Computational Linguistics.
- Liang et al. (2022) Shuo Liang, Wei Wei, Xian-Ling Mao, Fei Wang, and Zhiyong He. 2022. BiSyn-GAT+: Bi-syntax aware graph attention network for aspect-based sentiment analysis. In Findings of the Association for Computational Linguistics: ACL 2022, pages 1835–1848, Dublin, Ireland. Association for Computational Linguistics.
- Ma et al. (2017) Dehong Ma, Sujian Li, Xiaodong Zhang, and Houfeng Wang. 2017. Interactive Attention Networks for Aspect-Level Sentiment Classification. arXiv e-prints, page arXiv:1709.00893.
- Manning et al. (2014) Christopher Manning, Mihai Surdeanu, John Bauer, Jenny Finkel, Steven Bethard, and David McClosky. 2014. The Stanford CoreNLP natural language processing toolkit. In Proceedings of 52nd Annual Meeting of the Association for Computational Linguistics: System Demonstrations, pages 55–60, Baltimore, Maryland. Association for Computational Linguistics.
- Nguyen et al. (2020) Thanh-Tung Nguyen, Xuan-Phi Nguyen, Shafiq Joty, and Xiaoli Li. 2020. Differentiable window for dynamic local attention. In Proceedings of the 58th Annual Meeting of the Association for Computational Linguistics, pages 6589–6599, Online. Association for Computational Linguistics.
- OpenAI (2023a) OpenAI. 2023a. Introducing chatgpt.
- Pontiki et al. (2014) Maria Pontiki, Dimitris Galanis, John Pavlopoulos, Harris Papageorgiou, Ion Androutsopoulos, and Suresh Manandhar. 2014. SemEval-2014 task 4: Aspect based sentiment analysis. In Proceedings of the 8th International Workshop on Semantic Evaluation (SemEval 2014), pages 27–35, Dublin, Ireland. Association for Computational Linguistics.
- Shang et al. (2021) Xichen Shang, Qianli Ma, Zhenxi Lin, Jiangyue Yan, and Zipeng Chen. 2021. A span-based dynamic local attention model for sequential sentence classification. In Proceedings of the 59th Annual Meeting of the Association for Computational Linguistics and the 11th International Joint Conference on Natural Language Processing (Volume 2: Short Papers), pages 198–203, Online. Association for Computational Linguistics.
- Song et al. (2019) Youwei Song, Jiahai Wang, Tao Jiang, Zhiyue Liu, and Yanghui Rao. 2019. Attentional encoder network for targeted sentiment classification. arXiv preprint arXiv:1902.09314.
- Sun et al. (2019) Kai Sun, Richong Zhang, Samuel Mensah, Yongyi Mao, and Xudong Liu. 2019. Aspect-level sentiment analysis via convolution over dependency tree. In Proceedings of the 2019 Conference on Empirical Methods in Natural Language Processing and the 9th International Joint Conference on Natural Language Processing (EMNLP-IJCNLP), pages 5679–5688, Hong Kong, China. Association for Computational Linguistics.
- Tan et al. (2019) Xingwei Tan, Yi Cai, and Changxi Zhu. 2019. Recognizing conflict opinions in aspect-level sentiment classification with dual attention networks. In Proceedings of the 2019 Conference on Empirical Methods in Natural Language Processing and the 9th International Joint Conference on Natural Language Processing (EMNLP-IJCNLP), pages 3426–3431, Hong Kong, China. Association for Computational Linguistics.
- Tang et al. (2016) Duyu Tang, Bing Qin, and Ting Liu. 2016. Aspect level sentiment classification with deep memory network. In Proceedings of the 2016 Conference on Empirical Methods in Natural Language Processing, pages 214–224, Austin, Texas. Association for Computational Linguistics.
- Tang et al. (2022) Siyu Tang, Heyan Chai, Ziyi Yao, Ye Ding, Cuiyun Gao, Binxing Fang, and Qing Liao. 2022. Affective knowledge enhanced multiple-graph fusion networks for aspect-based sentiment analysis. In Proceedings of the 2022 Conference on Empirical Methods in Natural Language Processing, pages 5352–5362, Abu Dhabi, United Arab Emirates. Association for Computational Linguistics.
- Tian et al. (2021) Yuanhe Tian, Guimin Chen, and Yan Song. 2021. Aspect-based sentiment analysis with type-aware graph convolutional networks and layer ensemble. In Proceedings of the 2021 Conference of the North American Chapter of the Association for Computational Linguistics: Human Language Technologies, pages 2910–2922, Online. Association for Computational Linguistics.
- Touvron et al. (2023) Hugo Touvron, Louis Martin, Kevin R. Stone, Peter Albert, Amjad Almahairi, Yasmine Babaei, Nikolay Bashlykov, Soumya Batra, Prajjwal Bhargava, Shruti Bhosale, Daniel M. Bikel, Lukas Blecher, Cristian Cantón Ferrer, Moya Chen, Guillem Cucurull, David Esiobu, Jude Fernandes, Jeremy Fu, Wenyin Fu, Brian Fuller, Cynthia Gao, Vedanuj Goswami, Naman Goyal, Anthony S. Hartshorn, Saghar Hosseini, Rui Hou, Hakan Inan, Marcin Kardas, Viktor Kerkez, Madian Khabsa, Isabel M. Kloumann, A. V. Korenev, Punit Singh Koura, Marie-Anne Lachaux, Thibaut Lavril, Jenya Lee, Diana Liskovich, Yinghai Lu, Yuning Mao, Xavier Martinet, Todor Mihaylov, Pushkar Mishra, Igor Molybog, Yixin Nie, Andrew Poulton, Jeremy Reizenstein, Rashi Rungta, Kalyan Saladi, Alan Schelten, Ruan Silva, Eric Michael Smith, R. Subramanian, Xia Tan, Binh Tang, Ross Taylor, Adina Williams, Jian Xiang Kuan, Puxin Xu, Zhengxu Yan, Iliyan Zarov, Yuchen Zhang, Angela Fan, Melanie Kambadur, Sharan Narang, Aurelien Rodriguez, Robert Stojnic, Sergey Edunov, and Thomas Scialom. 2023. Llama 2: Open foundation and fine-tuned chat models. ArXiv, abs/2307.09288.
- Vaswani et al. (2017) Ashish Vaswani, Noam Shazeer, Niki Parmar, Jakob Uszkoreit, Llion Jones, Aidan N. Gomez, Łukasz Kaiser, and Illia Polosukhin. 2017. Attention is all you need. In Proceedings of the 31st International Conference on Neural Information Processing Systems, NIPS’17, page 6000–6010, Red Hook, NY, USA. Curran Associates Inc.
- Wang et al. (2020) Kai Wang, Weizhou Shen, Yunyi Yang, Xiaojun Quan, and Rui Wang. 2020. Relational graph attention network for aspect-based sentiment analysis. In Proceedings of the 58th Annual Meeting of the Association for Computational Linguistics, pages 3229–3238, Online. Association for Computational Linguistics.
- Wang et al. (2016) Yequan Wang, Minlie Huang, Xiaoyan Zhu, and Li Zhao. 2016. Attention-based LSTM for aspect-level sentiment classification. In Proceedings of the 2016 Conference on Empirical Methods in Natural Language Processing, pages 606–615, Austin, Texas. Association for Computational Linguistics.
- Zhang et al. (2019) Chen Zhang, Qiuchi Li, and Dawei Song. 2019. Aspect-based sentiment classification with aspect-specific graph convolutional networks. In Proceedings of the 2019 Conference on Empirical Methods in Natural Language Processing and the 9th International Joint Conference on Natural Language Processing (EMNLP-IJCNLP), pages 4568–4578, Hong Kong, China. Association for Computational Linguistics.
- Zhang et al. (2023) Mao Zhang, Yongxin Zhu, Zhen Liu, Zhimin Bao, Yunfei Wu, Xing Sun, and Linli Xu. 2023. Span-level aspect-based sentiment analysis via table filling. In Proceedings of the 61st Annual Meeting of the Association for Computational Linguistics (Volume 1: Long Papers), pages 9273–9284, Toronto, Canada. Association for Computational Linguistics.
- Zhang et al. (2020) Yu Zhang, Houquan Zhou, and Zhenghua Li. 2020. Fast and accurate neural crf constituency parsing. In Proceedings of the Twenty-Ninth International Joint Conference on Artificial Intelligence, IJCAI-20, pages 4046–4053. International Joint Conferences on Artificial Intelligence Organization. Main track.
- Zhang et al. (2022) Zheng Zhang, Zili Zhou, and Yanna Wang. 2022. SSEGCN: Syntactic and semantic enhanced graph convolutional network for aspect-based sentiment analysis. In Proceedings of the 2022 Conference of the North American Chapter of the Association for Computational Linguistics: Human Language Technologies, pages 4916–4925, Seattle, United States. Association for Computational Linguistics.
- Zheng et al. (2020) Yaowei Zheng, Richong Zhang, Samuel Mensah, and Yongyi Mao. 2020. Replicate, walk, and stop on syntax: an effective neural network model for aspect-level sentiment classification. In Proceedings of the AAAI conference on artificial intelligence, volume 34, pages 9685–9692.
- Zhou et al. (2021) Yuxiang Zhou, Lejian Liao, Yang Gao, Zhanming Jie, and Wei Lu. 2021. To be closer: Learning to link up aspects with opinions. In Proceedings of the 2021 Conference on Empirical Methods in Natural Language Processing, pages 3899–3909, Online and Punta Cana, Dominican Republic. Association for Computational Linguistics.
A Appendix
A.1 Experiments With ChatGPT
The rise of large language models (LLMs) such as GPT-3 Brown et al. (2020a), PaLM Chowdhery et al. (2022), Llama Touvron et al. (2023), etc, has greatly facilitated the rapid development of natural language processing (NLP). ChatGPT OpenAI (2023a), powered by GPT-3.5 and GPT-4, can achieve significant zero-shot and few-shot in-context learning (ICL) Brown et al. (2020b) performance on unseen tasks, even without any parameter updates.
In this section, we conducted exhaustive experiments to investigate the performance of ChatGPT on ABSA tasks and its ability for fine-grained understanding of segmental context.
Experiment setting : The version of ChatGPT we utilized in the experiment is gpt-3.5-turbo. To avoid variations in ChatGPT-generated outputs, the temperature parameter was set to 0. For the number of response words, the max tokens parameter was set to 512. We experimented with 3 different prompts and their respective settings as shown in Figure10. We take the laptop dataset as example to explain our prompt settings:
-
•
Prompt-setting 1: Zero-shot. In this prompt setting, we have only given definition: "Recognize the sentiment polarity for the given aspect term in the given review. Answer from the options [ “positive”, “negative”, “neutral” ] without any explanation.", then we sent a test review and all aspect words in this review to ChatGPT, and ChatGPT will give the answer.
-
•
Prompt-setting 2: 5-Shot ICL with single aspect short sentences. The given definition is same as setting 1. What differs from setting 1 is that we randomly selected 5 single-aspect word sentences from the training set as examples to better leverage ChatGPT’s In-Context Learning capability. Sequentially, we provide a test review and all aspect words, asking ChatGPT to output the sentiment polarity for each aspect word.
-
•
Prompt-setting 3: 5-Shot ICL with multiple aspects long sentences. What differs from setting 2 is that the examples chosen from the training set are all multiple aspects long sentences. The purpose of doing this is to explore whether ChatGPT possesses the ability to understand fine-grained segmental context and the strength of this ability.
Result analysis : The results using ChatGPT and our model are shown in table6. Firstly, from the result of prompt-setting 2, we can infer that using a few examples to guide ChatGPT can effectively improve its performance, demonstrating the powerful In-Context-Learning capability of ChatGPT. Secondly, we compared our experimental results with Han et al. (2023), who investigated the performance of ChatGPT on various information extraction tasks, including ABSA. We found that our model has better performance. Finally, from prompt-setting 3, we can observe that providing ChatGPT with long examples containing multiple aspect words can lead to better performance. However, the results still fall short compared to our model. This situation suggests that ChatGPT possesses the ability to understand fine-grained segmental information to some extent. Perhaps there are ways to better harness this ability, such as incorporating constituent trees and dynamic local attention mechanisms as described in this paper.
A.2 Paramer Comparison
We conduct a parameter comparison between S2GSL and other GCN-based baseline methods, shown in table 7. Notably, the number of parameters in S2GSL is comparable while the two graph learning branch design of S2GSL does lead to an increase in the parameters, it’s important to note that this does not result in an unnecessary escalation in computational costs when compared with the baselines.
Model | Laptop14 | Restaurant14 |
---|---|---|
F1 | F1 | |
5-shot ICL Han et al. (2023) | 76.76 | 81.85 |
Prompt-setting 1 | 75.62 | 79.48 |
Prompt-setting 2 | 77.02 | 81.38 |
Prompt-setting 3 | 77.91 | 82.14 |
S2GSL(Ours) | 79.09 | 82.84 |
Model | Parameter Count | Laptop | Restaurant | |
F1 | F1 | F1 | ||
ACLT(2021) | 110M | 75.83 | 78.44 | 74.51 |
T-GCN(2021) | 113M | 77.03 | 79.95 | 75.25 |
DualGCN(2021) | 111M | 78.10 | 81.16 | 76.02 |
SSEGCN(2022) | 110M | 77.96 | 81.09 | 76.02 |
S2GSL | 114M | 79.07 | 82.84 | 77.11 |
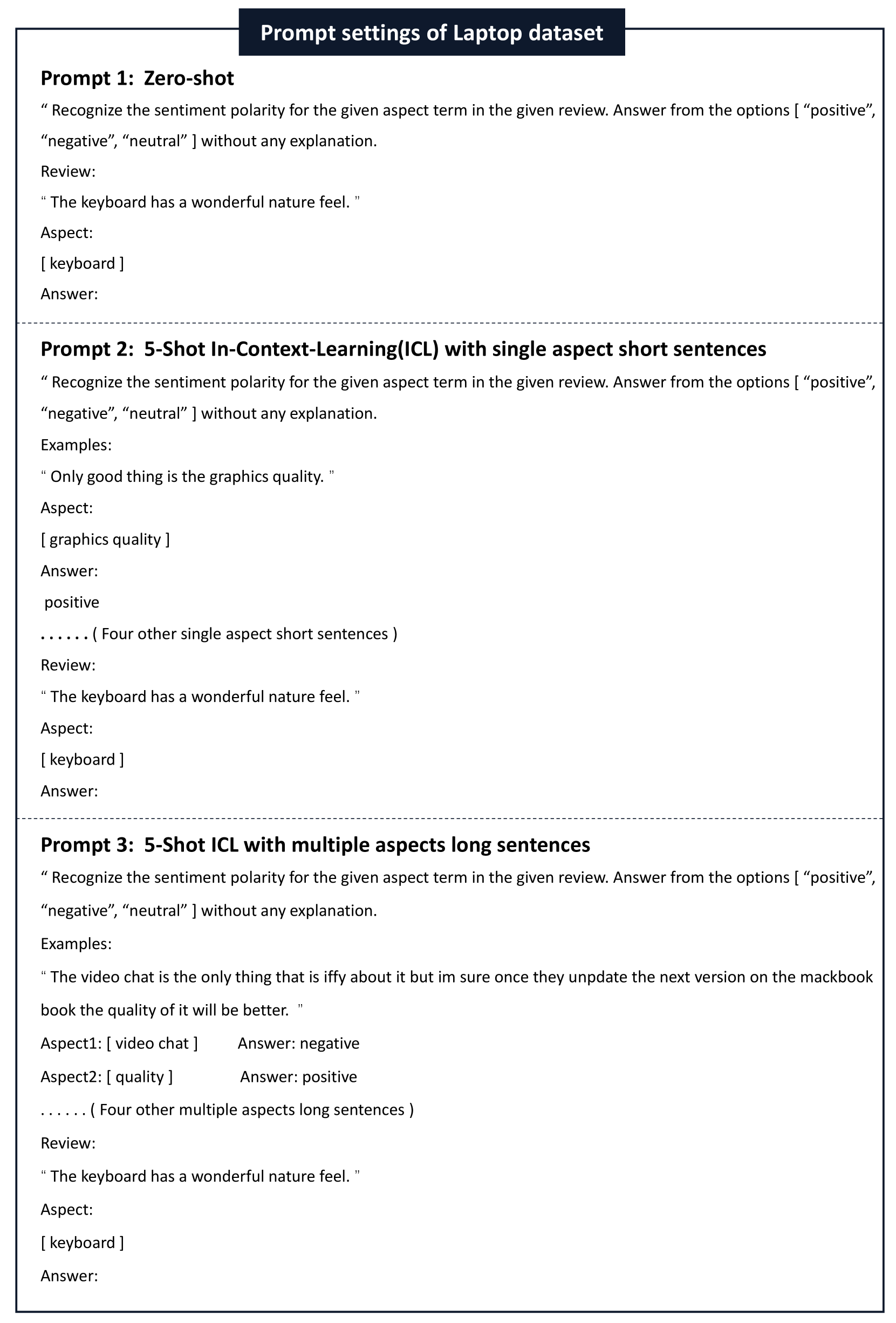