RVD: A Handheld Device-Based Fundus Video Dataset for Retinal Vessel Segmentation
Abstract
Retinal vessel segmentation is generally grounded in image-based datasets collected with bench-top devices. The static images naturally lose the dynamic characteristics of retina fluctuation, resulting in diminished dataset richness, and the usage of bench-top devices further restricts dataset scalability due to its limited accessibility. Considering these limitations, we introduce the first video-based retinal dataset by employing handheld devices for data acquisition. The dataset comprises 635 smartphone-based fundus videos collected from four different clinics, involving 415 patients from 50 to 75 years old. It delivers comprehensive and precise annotations of retinal structures in both spatial and temporal dimensions, aiming to advance the landscape of vasculature segmentation. Specifically, the dataset provides three levels of spatial annotations: binary vessel masks for overall retinal structure delineation, general vein-artery masks for distinguishing the vein and artery, and fine-grained vein-artery masks for further characterizing the granularities of each artery and vein. In addition, the dataset offers temporal annotations that capture the vessel pulsation characteristics, assisting in detecting ocular diseases that require fine-grained recognition of hemodynamic fluctuation. In application, our dataset exhibits a significant domain shift with respect to data captured by bench-top devices, thus posing great challenges to existing methods. Thanks to rich annotations and data scales, our dataset potentially paves the path for more advanced retinal analysis and accurate disease diagnosis. In the experiments, we provide evaluation metrics and benchmark results on our dataset, reflecting both the potential and challenges it offers for vessel segmentation tasks. We hope this challenging dataset would significantly contribute to the development of eye disease diagnosis and early prevention. The dataset is available at \faGithub RVD.
1 Introduction
The observation of the retinal vasculature patterns serves as a reliable approach to tracking the morphological changes of eyes over time. These morphological changes have been found to be closely associated with a spectrum of ocular diseases, e.g., diabetic retinopathy, age-related macular degeneration, and glaucoma [83, 14]. Retinal vessel segmentation aims to provide pixel-level extraction of the visible vasculature from a fundus image [56]. It is the initial yet fundamental step in objectively assessing vasculature in fundus images and quantitatively interpreting associated morphometrics. Thus, this task plays a pivotal role in understanding and diagnosing ocular diseases.
Existing methods for retinal vessel segmentation are designed on image-based datasets [38, 72, 69, 25], as shown in Fig. 1 (a). Although these datasets have contributed valuable vessel annotations for studying retinal segmentation, the static nature of images inherently limits their ability to portray dynamic retinal characteristics, e.g., vessel pulsations. These dynamic phenomena play a vital role in facilitating comprehensive and in-depth understanding of retinal functionality and vasculature structure. Moreover, image-based datasets are captured by expensive bench-top ophthalmic equipment, which is operated by professionally trained clinicians [31, 34]. Such requirements potentially limit the scale of the datasets and data diversity, thereby adversely affecting the generalization ability of the models trained on these datasets.
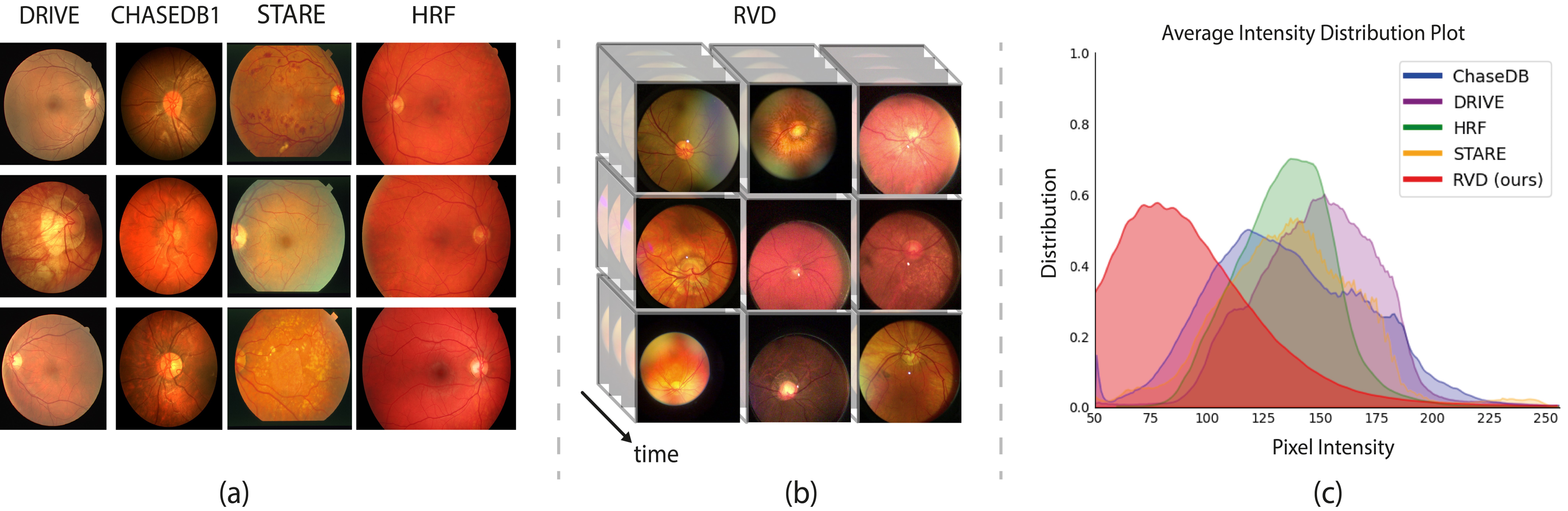
In recent years, advances in imaging technology have enabled the usage of smartphone-based devices for retinal observation [79, 32]. They offer better flexibility and portability, allowing for scalable data collection. In this paper, we introduce the first video-based retinal vessel dataset (RVD), a collection of 635 smartphone-based videos with detailed vessel annotation. These videos are recorded from four clinics, including patients from 50 to 75 years old. Some examples of our dataset are shown in Fig. 1 (b). The sequential frames capture the continuous changes in retinal vessels and thus significantly facilitate the analysis of subtle fluctuations in the retinal structure. Therefore, the use of portable devices for data acquisition and the provision of the video modality remarkably overcome the limitations of existing datasets.
The annotations provided in our dataset span two dimensions: spatial and temporal. In the spatial dimension, we offer three distinct levels of annotations: binary vessel masks, general vein-artery masks, and fine-grained vein-artery masks. Each kind of annotation is tailored to specific clinical purposes. Specifically, for the binary vessel masks, we identify the sharpest and most representative frame from the video clip and generate binary masks representing the skeletal structure of the vessels. This mask primarily targets the holistic vessel structure but neglects the difference between arteries and veins. For general vein-artery masks, we differentiate veins and arteries based on their respective vessel calibres and generate separate masks for them respectively. Lastly, in contrast to the general differentiation between arteries and veins, the fine-grained vein-artery masks further divide each retinal artery and vein into sections based on a set of pre-defined vessel widths. We thus generate eight different vein-artery masks for each sample and these masks precisely reflect the granularities of retinal vessels. These sophisticated masks are highly demanded when detecting ocular diseases [4, 10].
In the temporal dimension, we enrich our dataset with annotations of the complex dynamics of retinal vasculature. For each video, we focus on the optic disk regions where the retinal vessel fluctuation normally occurs. We then select and annotate frames with the maximal and minimal pulse widths as well as label the existence of spontaneous retinal venous pulsations (SVP). The existence and extent of vessel changes signify vascular pulsations and cranial pressure-related alterations. Clinically, the signals of pulsation facilitate the detection of abnormalities in retinal vessels, while precise identification of pressure-related alterations aids in detecting temporally-dependent ocular diseases. Our integration of temporal annotations thus increases its potential for ocular disease diagnosis.
The distinction between smartphone-based and benchtop devices and data modality differences result in domain gaps, as illustrated in Fig. 1. Furthermore, since our data are collected by handheld devices in clinics, our dataset also involves more realistic factors, e.g., the operations of the clinicians, surrounding illumination conditions, and eye movements of patients during video capture. Consequently, our dataset presents more challenges for existing vessel segmentation methods. More importantly, the large number of training samples and detailed annotations in our dataset will likely pave the way toward more advanced yet portable retinal analysis and more accurate disease diagnosis. In the experiments, we delve into an in-depth analysis of our dataset and provide benchmark results of different tasks on our newly curated dataset.
The main contributions of our paper are summarized as follows:
-
•
Dataset construction: We construct a new video-based retinal vessel dataset (RVD) with rich spatial and temporal annotations for vessel segmentation tasks. To the best of our knowledge, RVD is the first mobile-device based dataset for retinal vessel segmentation.
-
•
Three-level spatial annotations: Our dataset introduces three levels of annotations in spatial, comprising binary vessel masks, general vein-artery masks, and fine-grained vein-artery masks. The hierarchical and diverse descriptions of spatial annotations enable us to better analyze the vessel structure.
-
•
Temporal annotations: Our dataset also provides temporal annotations of spontaneous retinal venous pulsations (SVP) to reveal the dynamic changes in retinal vessels. This enables the assessment of pulsatile variations in retinal vessels.
-
•
Benchmarking: We investigate the gap between our dataset and previous retinal datasets by assessing the performance of several state-of-the-art methods. The experimental results will shed some light on mobile-device based retinal vessel segmentation.
2 Related Work
Existing Retinal Datasets: In the realm of retinal vessel segmentation, various retinal vessel datasets have been proposed. Existing datasets can be roughly categorized into two streams: binary vessel based ones and artery-vein based ones. Among the datasets with binary vessel masks, DRIVE [72], STARE [69], HRF [38], and CHASE_DB1 [25] have emerged as the most frequently used datasets. In fact, each of these datasets only comprises dozens of images. For example, DRIVE consists of 40 images captured in the 45-degree field of view, with an image size of 584565 pixels. Besides, DRiDB [59], ARIA [23], IOSTAR [84], and RC-SLO [2] are another publicly available datasets for retinal vessel segmentation. However, they are less used in recent years considering their data quality and maintenance. Recently, the FIVES dataset has been introduced [34], with data distributed across four categories: normal retinas, retinas affected by Diabetic Retinopathy, Glaucoma, and Age-related Macular Degeneration. It comprises 800 retinal images.
Regarding the datasets with artery-vein masks, RITE [31], AV-DRIVE [60], INSPIRE-AVR [57], and WIDE [21] are the available ones. The AV-DRIVE dataset, derived from DRIVE, consists of 40 images and offers separate ground truth masks for arteries and veins. The INSPIRE-AVR is an independently constructed dataset with artery-vein ground truth masks. It consists of 40 color images in total. The WIDE dataset provides 30 scanning laser ophthalmoscope (SLO) images.
In contrast to existing datasets which are collected with cumbersome bench-top devices and are composed of static images, our dataset is constructed with portable handheld devices and is video-based. Our dataset preserves the dynamic characteristics of vessels. Besides, existing datasets typically provide only one type of annotation for a specific research purpose, whereas our dataset offers annotations in both spatial and temporal dimensions. The spatial annotations include binary vessel masks, general vein-artery masks, and fine-grained vein-artery masks, respectively. The temporal annotations reveal the state of SVP, an important signal for diagnosing various diseases.
Methods for Retinal Vessel Segmentation: In the past years, a variety of methods have been developed for retinal vessel segmentation. Traditional methods mainly depend on handcrafted features [7, 16, 88, 76, 61, 28, 17, 36], which are less discriminative and effective [51]. With the unprecedented breakthroughs of deep neural networks (DNNs) in the image classification, detection, and segmentation tasks, researchers have explored the potential of DNNs in retinal vessel segmentation [22, 43, 35, 81, 89]. Many works [6, 42, 71, 58, 33, 15] adopt fully convolutional networks [46] to produce more accurate segmentation of retinal images by combining semantic information from deep layers with appearance information from shallow layers. Several works have focused on modifying the U-Net structure [74, 64, 85] for vessel segmentation. [86] first introduces the residual connection into U-net to detect vessels. This idea has been adopted in later studies [5, 41, 26, 78]. [82] introduces the local-region and cross-dataset contrastive learning losses in training to explore a more powerful feature embedding space. Besides, several other methods employ various networks and strategies for retinal vessel segmentation, such as generative and adversarial networks [40, 70], ensemble learning [49, 75, 44], and graph convolutional network [68].
The aforementioned methods are mainly conducted on datasets DRIVE, STARE, CHASE_DB1, and HRF, with binary vessel masks as supervision. Thanks to INSPIRE-AVR, AV-DRIVE, and WIDE, many works have been proposed to distinguish artery and vein [18, 21, 87, 50]. In [47], a multi-task deep neural network with spatial activation is proposed. The constructed network is able to segment full retinal vessels, arteries, and veins simultaneously. More recently, transformer based models, e.g., ViT [20], Swin transformer [45], and Mask2Former [13], have been proposed. These models have demonstrated their superior performance in capturing visual concepts and become popular backbones in visual understanding tasks [54]. We thus choose these models in the experiments to study the characteristics of our proposed dataset.
3 Our Proposed RVD
In this section, we first describe our data collection process and data sources. Concerning privacy and ethic, we perform this study in accordance with the guidelines of the Tenets of Helsinki. Written consent was obtained from all participants prior to any data collection, and all examination protocols adhered to the tenets of the Declaration of Helsinki. Once clinical data have been collected, we need to clean and pre-process the data in order to facilitate clinicians’ annotations and neural network training. In our work, the annotations are provided by professionally well-trained clinicians, and they have been asked to not only annotate conventional spatial segmentation masks but also temporal segmentation masks for dynamic biomarkers, such as Spontaneous retinal Venous Pulsations (SVPs). Last, we will introduce the data split and relevant tasks that are supported by our dataset.
Dataset | Resolution | Modality | Device | Num | Dimension | Annotation type |
STARE [69] | 605700 | Image | Benchtop | 20 | Spatial | Binary |
DRIVE [72] | 768584 | Image | Benchtop | 40 | Spatial | Binary |
ARIA [23] | 576768 | Image | Benchtop | 161 | Spatial | Binary |
CHASEDB1 [25] | 990960 | Image | Benchtop | 28 | Spatial | Binary |
INSPIRE-AVR [57] | 23922048 | Image | Benchtop | 40 | Spatial | Multi-class |
HRF [38] | 33042336 | Image | Benchtop | 45 | Spatial | Binary |
RITE [31] | 768584 | Image | Benchtop | 40 | Spatial | Multi-class |
FIVES [34] | 20482048 | Image | Benchtop | 800 | Spatial | Binary |
RAVIR [29] | 768768 | Image | IR Laser | 42 | Spatial | Multi-class |
RVD (ours) | 18001800 | Video | Hand-held | 1,270 | Spatial + Temporal | Multi-class |
3.1 Data Collection
For data collection, the employed hand-held fundus imaging devices are constructed by connecting a smartphone to the fundus camera lens. Then, clinicians are trained to operate the hand-held devices to examine patients’ retinas while collecting fundus videos. These participants are fully aware of data collection when they undergo their annual medical examinations. With the help of clinicians, a total of 415 patients from four different clinics participate in the data collection process. As data are collected in different clinics over the past five years, the employed smartphones are different, thus increasing the diversity of data sources. More specifically, 264 males and 151 females are included here. Their ages range from 50 to 75. People of these ages are commonly considered to be at high risk for eye-related diseases, such as glaucoma and hypertension [37]. Our dataset involves both videos recorded from healthy eyes and videos from eyes with ocular diseases.
During the collection, one eye of each patient is recorded at a time. In this manner, at least one fundus video of each patient has been recorded and some participate multiple times in video recording. As a result, a total of 635 RGB videos have been captured. All captured videos have a frame rate of 25 frames per second, with the duration varying between 2 to 30 seconds. The total number of frames in our dataset is over 130,000. This collection process ensures the generality and diversity of our dataset for retinal vessel analysis. The detailed information of our dataset is shown in Table 1 and some examples could be found in Fig. 1 (b).
3.2 Data Cleaning and Preprocessing
Although we have tried our best to minimize environmental interference during collection, the original videos still exhibit various noise, such as video jittering and motion blur. Such noise will severely degrade the quality of collected videos and impose more difficulties in annotations. Hence, we eliminate the noise in the footage to improve the quality of our dataset and facilitate annotations.
Data cleaning: Considering that blood vessel dynamics mostly appear in ODR, we remove the video segments without ODR and ensure the existence of ODR in all videos. To this end, we employ an ODR detection method to localize ODR. Specifically, we label ODR regions by bounding-boxes, and for each video, we only annotate one frame per 25 frames (i.e., 1 second), similar to [67]. Then, we leverage the labeled ODR as supervision to train the Faster-RCNN detection network [62]. After that, the remaining frames are labeled by the trained Faster-RCNN. Manual check is also conducted to modify erroneous detection results by annotators. We only select video segments in the dataset if their ODRs are detectable in a minimum of 30 continuous frames. Such operations help maintain the overall quality of our video data.
To further improve the data quality, we leverage the optical flow to pinpoint frames with a high level of blur. Optical flow captures the spatial alterations between distinct frames, and thus it could serve as an indicator of spatial sharpness. Frames with large optical flow are subsequently discarded, as they likely correspond to instances of blurring. Similar to ODR detection, annotators (non-experts) also manually remove frames that undergo severe blur but have not been spotted by optical flow.
Data Preprocessing: In retinal vessel segmentation, ODR and its surrounding area are the most representative regions of the eye and provide extensive details about retinal vessels. However, the inherent ocular movement results in varying ODR positions across different frames. Such variations can impede the precise observation and annotation of SVP by clinicians. To tackle this issue, we employ the template matching algorithm [8] to stabilize the ODRs across video segments, ensuring a consistent ODR placement and a fixed field of view across frames. This facilitates human observation and machine perception of dynamic changes surrounding the ODR, thus greatly enhancing annotations and clinical diagnosis.
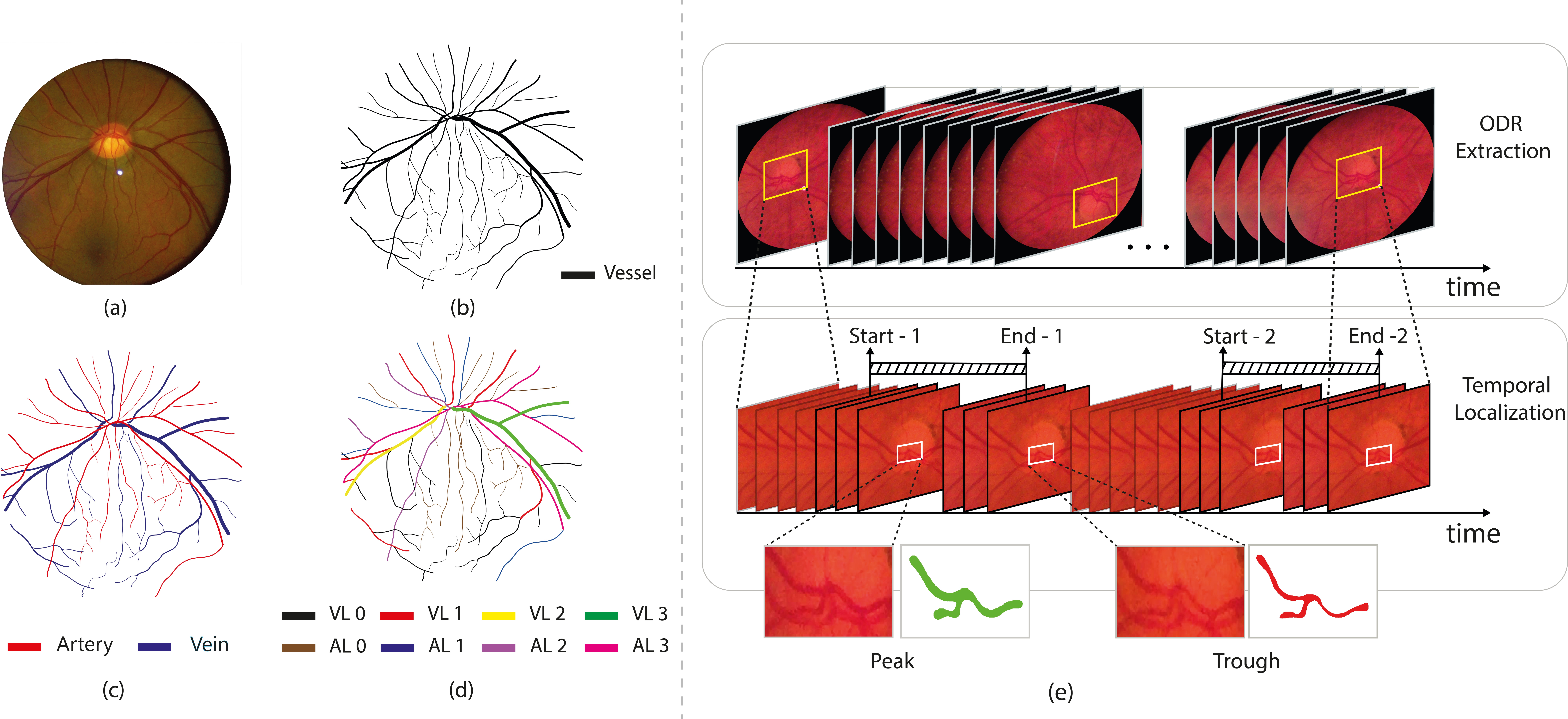
3.3 Data Annotation
After data cleaning and preprocessing of the initial videos, we detail the annotation process and provide the annotations for both spatial vessel segmentation and temporal SVP localization and classification tasks. To ensure the annotation quality, six clinicians are involved in this process.
3.3.1 Spatial segmentation
To mitigate the redundancy of annotating similar video frames, we select two keyframes from each video and generate subsequent annotations for them. Here, we adopt a three-fold strategy to identify the most representative frame from a video. (1) A frame contains the most vessels in a video; (2) A frame can cover ODR, fovea, and macula regions since these regions pathologically have a significant number of capillaries; (3) After one frame is selected, we choose another frame that not only has visible ODR with high-density vessels but also exhibits maximum spatial distance between its ODR and the previously selected ones. In this way, the selected frames will cover most of the retinal vasculature and any pathological regions of the retina. We then elaborate on creating three types of spatial annotations: binary vessel masks, general vein-artery masks, and fine-grained vein-artery masks, as follows:
Binary vessel masks: To generate the binary vessel masks, we adopt a similar method proposed in [48]. For each frame, we first draft a centerline-level annotation using the ImageJ software [65] and generate the delineation of vessel boundaries to obtain the main structure of vessels. Then we employ our experts to manually refine the structure by correcting the boundaries and improving the details of small capillaries. We can obtain the binary vessel masks by assigning the label to the refined structure (see Fig. 2 (b)).
General vein-artery masks: Many intracranial vascular diseases are found to be related to retinal vessels and affect the arteries and veins differently [3]. Thus, distinguishing between the retinal artery and vein plays a critical role in the clinical biomarker study of how various systemic and cardiovascular diseases affect the retinal vessels. In practice, the arteries and veins can be distinguished based on their difference in three aspects: color, light reflection, and calibres. The veins generally have a darker color than arteries and show a smaller central light reflex. Meanwhile, the veins are also wider than adjacent arteries. Then, clinicians only need to assign labels (i.e., vein and artery) to the vessels and obtain the vein-artery masks, as shown in Fig. 2 (c).
Fine-grained vein-artery masks: Vascular morphology holds substantial clinical significance, as alterations in vessel diameters frequently signify the presence of various diseases. For example, damage of the small retinal vessels could result in diabetic retinopathy [39]. Similarly, glaucoma pathogenesis is postulated to be linked to alterations in the retinal vasculature, such as retinal arteriolar narrowing and decreased fractal dimension [1]. Despite the clinical importance of such information, existing datasets scarcely provide this type of labels. Therefore, we consider the morphological characteristics of each artery and vein in our dataset and thus provide fine-grained vein-artery masks based on the vessel widths.
Specifically, we first measure the vessel diameters automatically via “Vessel Diameters” plugin in ImageJ.111https://imagej.net/software/imagej/ Then, we divide the arteries into multiple small vessel segments based on the diameters of the vessels. Based on the largest diameter among these artery segments, we define four levels of widths according to specific ratios. More specifically, a vessel segment within the range of 0-25% of the largest diameter is categorized as level 0. Similarly, levels 1, 2, and 3 correspond to vessel widths in the ranges of 25%-50%, 50%-75%, and 75%-100% of the largest diameter, respectively. Afterward, we obtain four-class masks for arteries based on vessel widths. The same operation is also applied to veins. After the automatic processing, clinicians will validate the quality of the fine-grained segmentation masks. This process ultimately yields eight-class masks for both arteries and veins (see Fig. 2 (d)). Those masks significantly enrich the granularity of our dataset.
3.3.2 Temporal localization
Existence of SVP: Based on the results of data cleaning and preprocessing, we utilize the stabilized videos and further annotate the dynamic state of vessel pulsations. Spontaneous retinal Venous Pulsation (SVP) plays a crucial role as a biomarker in retina assessments. Specifically, SVP is characterized by rhythmic pulsations evident in the central retinal vein and its branches, typically observable within the optic disc region (ODR) of the retinas. The absence of SVP holds substantial clinical significance, as it is correlated with certain pathologies. For example, the absence of SVP is associated with progressive glaucoma [53], and it is indicative of increased intracranial pressure [52]. Considering the needs of the specialty, we invite multiple clinicians to annotate the presence or absence of SVP in each video of our dataset. Once the annotation process completes, we obtain 335 “SVP-present” videos and 300 “SVP-absent” videos respectively. This annotation establishes a fundamental task of SVP detection, facilitating further analysis and investigation on the relationship between SVP and eye diseases.
Temporal duration of SVP: After annotating the existence of SVP in the stabilized videos, some “SVP-present” videos may not contain SVP throughout the whole video. This means in some frames SVP is not visible. Using these videos to train an SVP classification model would suffer ambiguity especially when an entire video cannot be fed into a neural network. Therefore, we further provide temporal emergence annotations of SVP by indicating the starting and ending frames of retinal vessel fluctuation (see Fig. 2 (e)). The detailed duration of SVP serves two purposes: it acts as a valuable signal to improve the performance of SVP detection tasks and concurrently sets a new task for SVP temporal localization. We obtain videos in three distinct groups: 156 videos containing intermittent SVPs, 179 videos demonstrating persistent SVPs, and the remaining 300 videos without SVPs. These temporal annotations allow us to better understand retinal vessel dynamics.
“Peak” and “Trough” annotations of SVP: As discussed above, SVP reflects the temporal dilation and contraction in retinal vessels. The state with maximal dilation is characterized as “peak”, whereas those with maximal contraction are termed “trough”. Here, we select frames corresponding to the “peak” and “trough” states from each “SVP-present” video. Subsequently, we generate corresponding masks for these selected frames, yielding a total of 670 annotated masks. This annotation allows us to quantitatively measure the extent of pulsations and occurring positions of vessel pulsations.
3.4 Data Protocols
Data split: When partitioning our dataset for training and evaluation, we also take into account the similarity of the recorded videos of the same person. Specifically, we ensure that videos captured from the same patient are allocated to the same subset during the partitioning process. This strategy aims to decrease the similarity between training data and testing data and thus minimizes performance bias. In practice, we divide the data based on patient IDs. In this manner, the same patient’s videos will not appear simultaneously in both the training and testing sets. Then, we select 517 videos from the 635 videos for training and validation, and the rest 118 videos are used for testing. We also cross-validate a method with three different data splits and will release the dataset and data splits.
Metrics: Based on our annotations, we can conduct tasks in two major categories. (1) Retinal vessel segmentation metrics: Since the binary, general artery-vein and fine-grained vessel segmentation tasks are essentially semantic segmentation, we adopt the mean Intersection over Union (mIoU), mean Accuracy (mAcc), and mean F-score (mFscore) to evaluate the performance of models on our dataset. Note that mFscore is of special interest since we have provided annotations for multi-class segmentation. (2) SVP recognition and temporal localization metrics: SVP recognition is to classify whether SVP exists in a video. SVP localization task is to identify the time period where SVP appears in a video. We adopt the Accuracy (Acc), Area Under the Receiver Operating Characteristic Curve (AUROC), and Recall for SVP recognition. The frame-mAP (F-mAP), video-mAP (V-mAP) [27] under IoU threshold of 0.5 and mean Intersection over Union (mIOU) are adopted for the task of SVP localization.
4 Experiments
In this section, we employ state-of-the-art (SOTA) segmentation methods to examine the contributions and challenges of our newly curated RVD as well as establish a new benchmark for the dynamic vessel segmentation and localization tasks. As our data are collected from hand-held fundus imaging devices, we also investigate whether domain gaps between our dataset and existing ones which are captured by benchtop based devices.
Method | Backbone | Binary | General Artery-Vein | Fine-grained Artery-Vein | ||||||
mIoU | mAcc | mFscore | mIoU | mAcc | mFscore | mIoU | mAcc | mFscore | ||
DLV3 [11] | UNet [63] | |||||||||
ResNet50 [30] | ||||||||||
ResNet101 | ||||||||||
M2F [12] | ResNet50 | |||||||||
ResNet101 | ||||||||||
Swin-T [45] | ||||||||||
Swin-S | ||||||||||
Swin-B-1k | ||||||||||
Swin-B-22k | ||||||||||
Swin-L |
4.1 Overall Results
We first focus on spatial segmentation tasks on our dataset. We conduct experiments of binary vessel segmentation, general artery-vein segmentation, and fine-grained artery-vein segmentation, respectively. We employ several popular used segmentation methods, including FCN [66], DeepLabV3 [11], Segmentor [73], and Mask2Former [12]. We also apply different backbones to these segmentation methods. The adopted backbones involves convolutional UNet [63], ResNet [30], ViT [20], and Swin Transformer [45]. We use the pre-trained parameters as initialization and train the networks on our training set. Due to the space limit, we only present the results of DeepLabV3 and Mask2Former in Table 2 and others are reported in the Appendix.
Even for the binary segmentation task, the highest mIoU results barely reach around 70%. For the more complex fine-grained artery-vein segmentation, the mIoU values further decline to approximately 25%. Some visual results are shown in Fig. 3. It is observed that current methods in general struggle to localize thin vessels. However, these thin vessels always play an important role in reflecting some diseases, e.g., atherosclerosis [80]. The performance of the SOTA methods implies the challenges of our dataset, but this also emphasizes the potential of our dataset for future studies.
Method | Recognition | Localization | ||||
Acc | AUROC | Recall | F-mAP | V-mAP | mIOU | |
LRCN [19] | 52.68 | 56.79 | 45.00 | 64.62 | 59.06 | 50.62 |
I3D [9] | 60.71 | 61.83 | 61.67 | 67.49 | 60.63 | 51.89 |
X3D [24] | 52.68 | 51.60 | 75.00 | 61.12 | 52.60 | 50.53 |
TSN [77] | 50.89 | 64.39 | 30.00 | 67.60 | 56.85 | 50.89 |
VTN [55] | 58.93 | 65.58 | 86.67 | 68.08 | 57.64 | 51.25 |
We also conduct experiments on temporal SVP recognition and localization with our provided annotations. In SVP recognition, we train the models to predict whether SVP exists in a video. In SVP localization, we train the models to identify the time period where SVP appears in a video. We employ the models of LRCN [19], I3D [9], X3D [24], TSN [77], and VTN [55]. We use the metrics in Section 3.4 and report the results in Table 3. To the best of our knowledge, we are the first to provide data and annotations for SVP recognition and localization. However, it is found that existing methods fail to recognize and localize SVP precisely on our real-clinic video data. For example, in SVP localization task, VTN only achieves 51.25% mIoU, which might not meet the needs of real-world applications. Such results indicate that more specific-designed methods are highly demanded.
4.2 Domain Gaps between RVD and Existing Datasets
We conduct a two-way evaluation process where models trained on our dataset are tested on previous datasets and models trained on existing datasets are also evaluated on our dataset. First, to evaluate binary vessel segmentation performance, we include the following datasets: CHASE DB1 (C-DB.), DRIVE (DRI.), HRF, and STARE (STA.). Then, we also conduct general artery-vein segmentation on the RITE dataset. The results are shown in Table 4. Note that existing datasets do not support fine-grained eight-class segmentation, and thus we did not test our data in this setting. Due to the domain gap, the models suffer performance drop. The results also indicate that our dataset provides unique data samples. The visualization is illustrated in the Appendix. From our experimental results above, we can tell that the retinal vessel segmentation is far from being solved. Our RVD dataset will serve as a valuable resource, motivating future explorations in retinal vessel segmentation.
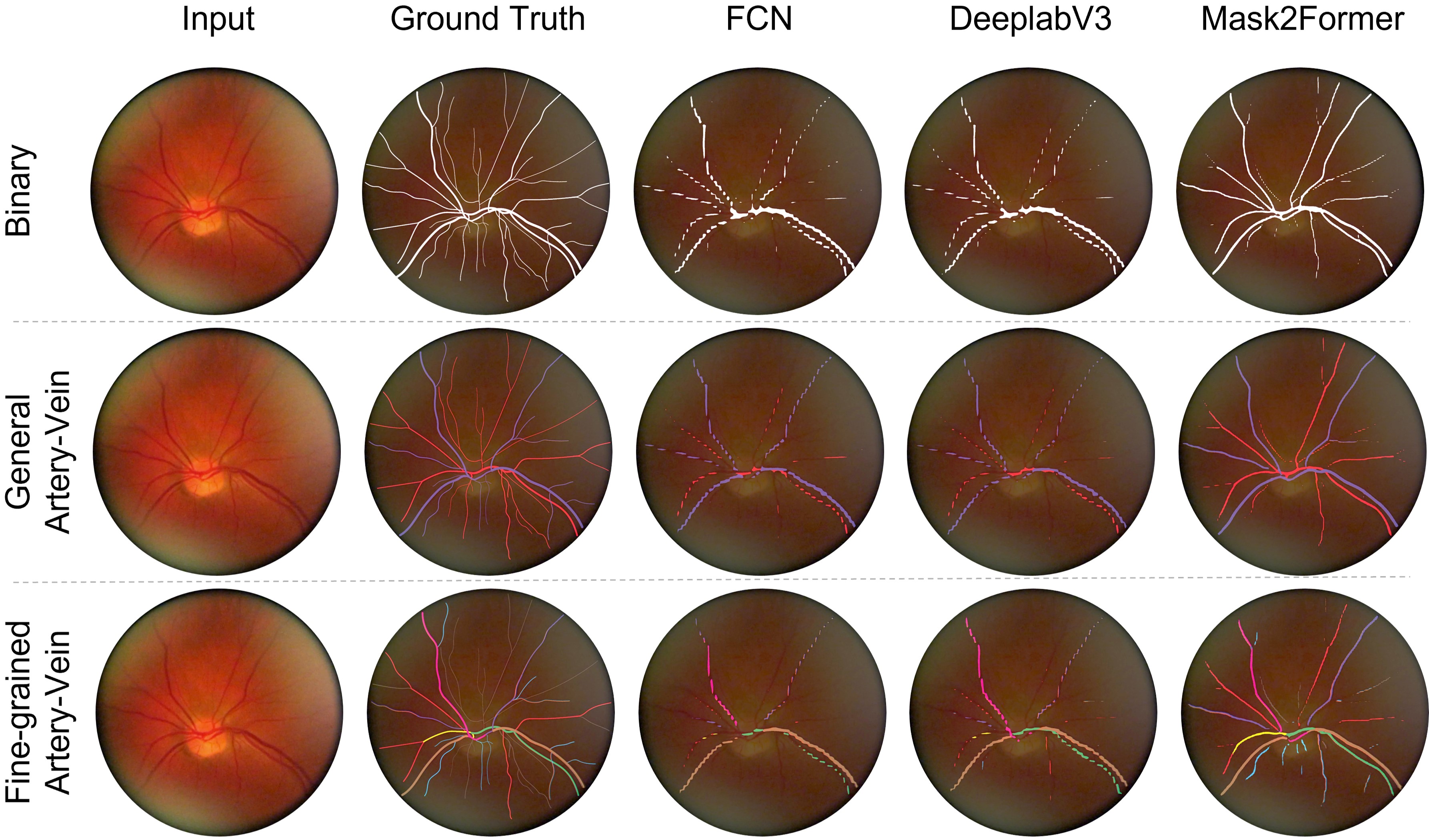
Method | Backbone | Binary Segmentation | General Artery-Vein | ||||||||
RVD C-DB. | C-DB. RVD | RVD DRI. | DRI. RVD | RVD HRF | HRF RVD | RVD STA. | STA. RVD | RVD RITE | RITE RVD | ||
DLV3 | UNet | ||||||||||
ResNet50 | |||||||||||
ResNet101 | |||||||||||
M2F | ResNet50 | ||||||||||
ResNet101 | |||||||||||
Swin-T | |||||||||||
Swin-S | |||||||||||
Swin-B-1k | |||||||||||
Swin-B-22k | |||||||||||
Swin-L |
5 Conclusion
In this work, we propose the first video-based retinal vessel segmentation dataset by employing handheld devices for data acquisition. Our dataset significantly complements the current benchtop-based datasets for retinal vessel segmentation and enables SVP detection and localization. More importantly, it offers rich annotations for both spatial vessel segmentation and temporal SVP localization. In comparison to existing datasets, our dataset is not only the largest scale one with the most diverse annotations but also more challenging. The domain gaps between our dataset and existing ones allow researchers to investigate how to minimize the domain gaps in vessel segmentation. Therefore, our curated dataset RVD is valuable for retinal vessel segmentation and would facilitate the clinical diagnosis of eye-related diseases.
References
- bmj [2018] Correction: Retinal vasculature in glaucoma: a review. BMJ Open Ophthalmology, 3(1), 2018. doi: 10.1136/bmjophth-2016-000032corr1. URL https://bmjophth.bmj.com/content/3/1/bmjophth-2016-000032corr1.
- Abbasi-Sureshjani et al. [2015] Samaneh Abbasi-Sureshjani, Iris Smit-Ockeloen, Jiong Zhang, and Bart Ter Haar Romeny. Biologically-inspired supervised vasculature segmentation in slo retinal fundus images. In Image Analysis and Recognition: 12th International Conference, ICIAR 2015, Niagara Falls, ON, Canada, July 22-24, 2015, Proceedings 12, pages 325–334. Springer, 2015.
- Abràmoff et al. [2010] Michael D Abràmoff, Mona K Garvin, and Milan Sonka. Retinal imaging and image analysis. IEEE reviews in biomedical engineering, 3:169–208, 2010.
- Ali et al. [2021] Aziah Ali, W Mimi Diyana W Zaki, Aini Hussain, and Wan Haslina Wan Abdul Halim. Retinal width estimation of high-resolution fundus images for diabetic retinopathy detection. In 2020 IEEE-EMBS Conference on Biomedical Engineering and Sciences (IECBES), pages 460–465, 2021. doi: 10.1109/IECBES48179.2021.9398752.
- Alom et al. [2018] Md Zahangir Alom, Mahmudul Hasan, Chris Yakopcic, Tarek M Taha, and Vijayan K Asari. Recurrent residual convolutional neural network based on u-net (r2u-net) for medical image segmentation. arXiv preprint arXiv:1802.06955, 2018.
- Atli and Gedik [2021] Ibrahim Atli and Osman Serdar Gedik. Sine-net: A fully convolutional deep learning architecture for retinal blood vessel segmentation. Engineering Science and Technology, an International Journal, 24(2):271–283, 2021.
- Barkana et al. [2017] Buket D Barkana, Inci Saricicek, and Burak Yildirim. Performance analysis of descriptive statistical features in retinal vessel segmentation via fuzzy logic, ann, svm, and classifier fusion. Knowledge-Based Systems, 118:165–176, 2017.
- Brunelli [2009] Roberto Brunelli. Template matching techniques in computer vision: theory and practice. John Wiley & Sons, 2009.
- Carreira and Zisserman [2017] Joao Carreira and Andrew Zisserman. Quo vadis, action recognition? a new model and the kinetics dataset. In proceedings of the IEEE Conference on Computer Vision and Pattern Recognition, pages 6299–6308, 2017.
- Chalakkal et al. [2020] Renoh Johnson Chalakkal, Waleed Habib Abdulla, and Sheng Chiong Hong. 3 - fundus retinal image analyses for screening and diagnosing diabetic retinopathy, macular edema, and glaucoma disorders. In Ayman S. El-Baz and Jasjit S. Suri, editors, Diabetes and Fundus OCT, Computer-Assisted Diagnosis, pages 59–111. Elsevier, 2020. ISBN 978-0-12-817440-1. doi: https://doi.org/10.1016/B978-0-12-817440-1.00003-6. URL https://www.sciencedirect.com/science/article/pii/B9780128174401000036.
- Chen et al. [2017] Liang-Chieh Chen, George Papandreou, Florian Schroff, and Hartwig Adam. Rethinking atrous convolution for semantic image segmentation. arXiv preprint arXiv:1706.05587, 2017.
- Cheng et al. [2021] Bowen Cheng, Anwesa Choudhuri, Ishan Misra, Alexander Kirillov, Rohit Girdhar, and Alexander G Schwing. Mask2former for video instance segmentation. arXiv preprint arXiv:2112.10764, 2021.
- Cheng et al. [2022] Bowen Cheng, Ishan Misra, Alexander G Schwing, Alexander Kirillov, and Rohit Girdhar. Masked-attention mask transformer for universal image segmentation. In Proceedings of the IEEE/CVF Conference on Computer Vision and Pattern Recognition, pages 1290–1299, 2022.
- Cheung et al. [2017] Carol Yim-lui Cheung, Charumathi Sabanayagam, Antony Kwan-pui Law, Neelam Kumari, Daniel Shu-wei Ting, Gavin Tan, Paul Mitchell, Ching Yu Cheng, and Tien Yin Wong. Retinal vascular geometry and 6 year incidence and progression of diabetic retinopathy. Diabetologia, 60:1770–1781, 2017.
- Dasgupta and Singh [2017] Avijit Dasgupta and Sonam Singh. A fully convolutional neural network based structured prediction approach towards the retinal vessel segmentation. In 2017 IEEE 14th international symposium on biomedical imaging (ISBI 2017), pages 248–251. IEEE, 2017.
- Dash and Bhoi [2017] Jyotiprava Dash and Nilamani Bhoi. A thresholding based technique to extract retinal blood vessels from fundus images. Future Computing and Informatics Journal, 2(2):103–109, 2017.
- Dash and Senapati [2020] Sonali Dash and Manas Ranjan Senapati. Enhancing detection of retinal blood vessels by combined approach of dwt, tyler coye and gamma correction. Biomedical Signal Processing and Control, 57:101740, 2020.
- Dashtbozorg et al. [2013] Behdad Dashtbozorg, Ana Maria Mendonça, and Aurélio Campilho. An automatic graph-based approach for artery/vein classification in retinal images. IEEE Transactions on Image Processing, 23(3):1073–1083, 2013.
- Donahue et al. [2015] Jeffrey Donahue, Lisa Anne Hendricks, Sergio Guadarrama, Marcus Rohrbach, Subhashini Venugopalan, Kate Saenko, and Trevor Darrell. Long-term recurrent convolutional networks for visual recognition and description. In Proceedings of the IEEE conference on computer vision and pattern recognition, pages 2625–2634, 2015.
- Dosovitskiy et al. [2020] Alexey Dosovitskiy, Lucas Beyer, Alexander Kolesnikov, Dirk Weissenborn, Xiaohua Zhai, Thomas Unterthiner, Mostafa Dehghani, Matthias Minderer, Georg Heigold, Sylvain Gelly, et al. An image is worth 16x16 words: Transformers for image recognition at scale. arXiv preprint arXiv:2010.11929, 2020.
- Estrada et al. [2015] Rolando Estrada, Michael J Allingham, Priyatham S Mettu, Scott W Cousins, Carlo Tomasi, and Sina Farsiu. Retinal artery-vein classification via topology estimation. IEEE transactions on medical imaging, 34(12):2518–2534, 2015.
- Fan and Mo [2016] Zhun Fan and Jia-Jie Mo. Automated blood vessel segmentation based on de-noising auto-encoder and neural network. In 2016 International conference on machine learning and cybernetics (ICMLC), volume 2, pages 849–856. IEEE, 2016.
- Farnell et al. [2008] Damian JJ Farnell, Fraser N Hatfield, Paul Knox, Michael Reakes, Stan Spencer, David Parry, and Simon P Harding. Enhancement of blood vessels in digital fundus photographs via the application of multiscale line operators. Journal of the Franklin institute, 345(7):748–765, 2008.
- Feichtenhofer [2020] Christoph Feichtenhofer. X3d: Expanding architectures for efficient video recognition. In Proceedings of the IEEE/CVF conference on computer vision and pattern recognition, pages 203–213, 2020.
- Fraz et al. [2012] Muhammad Moazam Fraz, Paolo Remagnino, Andreas Hoppe, Bunyarit Uyyanonvara, Alicja R Rudnicka, Christopher G Owen, and Sarah A Barman. An ensemble classification-based approach applied to retinal blood vessel segmentation. IEEE Transactions on Biomedical Engineering, 59(9):2538–2548, 2012.
- Gegundez-Arias et al. [2021] Manuel E Gegundez-Arias, Diego Marin-Santos, Isaac Perez-Borrero, and Manuel J Vasallo-Vazquez. A new deep learning method for blood vessel segmentation in retinal images based on convolutional kernels and modified u-net model. Computer Methods and Programs in Biomedicine, 205:106081, 2021.
- Gkioxari and Malik [2015] Georgia Gkioxari and Jitendra Malik. Finding action tubes. In Proceedings of the IEEE conference on computer vision and pattern recognition, pages 759–768, 2015.
- Hashemzadeh and Azar [2019] Mahdi Hashemzadeh and Baharak Adlpour Azar. Retinal blood vessel extraction employing effective image features and combination of supervised and unsupervised machine learning methods. Artificial intelligence in medicine, 95:1–15, 2019.
- Hatamizadeh et al. [2022] Ali Hatamizadeh, Hamid Hosseini, Niraj Patel, Jinseo Choi, Cameron C Pole, Cory M Hoeferlin, Steven D Schwartz, and Demetri Terzopoulos. Ravir: A dataset and methodology for the semantic segmentation and quantitative analysis of retinal arteries and veins in infrared reflectance imaging. IEEE Journal of Biomedical and Health Informatics, 26(7):3272–3283, 2022.
- He et al. [2015] Kaiming He, Xiangyu Zhang, Shaoqing Ren, and Jian Sun. Deep residual learning for image recognition, 2015.
- Hu et al. [2013] Qiao Hu, Michael D Abràmoff, and Mona K Garvin. Automated separation of binary overlapping trees in low-contrast color retinal images. In Medical Image Computing and Computer-Assisted Intervention–MICCAI 2013: 16th International Conference, Nagoya, Japan, September 22-26, 2013, Proceedings, Part II 16, pages 436–443. Springer, 2013.
- Iqbal [2021] Usama Iqbal. Smartphone fundus photography: a narrative review. International Journal of Retina and Vitreous, 7(1):44, 2021.
- Jiang et al. [2018] Zhexin Jiang, Hao Zhang, Yi Wang, and Seok-Bum Ko. Retinal blood vessel segmentation using fully convolutional network with transfer learning. Computerized Medical Imaging and Graphics, 68:1–15, 2018.
- Jin et al. [2022] Kai Jin, Xingru Huang, Jingxing Zhou, Yunxiang Li, Yan Yan, Yibao Sun, Qianni Zhang, Yaqi Wang, and Juan Ye. Fives: A fundus image dataset for artificial intelligence based vessel segmentation. Scientific Data, 9(1):475, 2022.
- Khalaf et al. [2016] Aya F Khalaf, Inas A Yassine, and Ahmed S Fahmy. Convolutional neural networks for deep feature learning in retinal vessel segmentation. In 2016 IEEE international conference on image processing (ICIP), pages 385–388. IEEE, 2016.
- Khan et al. [2022] Tariq M Khan, Mohammad AU Khan, Naveed Ur Rehman, Khuram Naveed, Imran Uddin Afridi, Syed Saud Naqvi, and Imran Raazak. Width-wise vessel bifurcation for improved retinal vessel segmentation. Biomedical Signal Processing and Control, 71:103169, 2022.
- Klein et al. [2011] Ronald Klein, Chiu-Fang Chou, Barbara EK Klein, Xinzhi Zhang, Stacy M Meuer, and Jinan B Saaddine. Prevalence of age-related macular degeneration in the us population. Archives of ophthalmology, 129(1):75–80, 2011.
- Köhler et al. [2013] Thomas Köhler, Attila Budai, Martin F Kraus, Jan Odstrčilik, Georg Michelson, and Joachim Hornegger. Automatic no-reference quality assessment for retinal fundus images using vessel segmentation. In Proceedings of the 26th IEEE international symposium on computer-based medical systems, pages 95–100. IEEE, 2013.
- Kumar et al. [2012] KP Sampath Kumar, Debjit Bhowmik, G Harish, S Duraivel, and B Pragathi Kumar. Diabetic retinopathy-symptoms, causes, risk factors and treatment. The Pharma Innovation, 1(8), 2012.
- Lahiri et al. [2020] Avisek Lahiri, Vineet Jain, Arnab Mondal, and Prabir Kumar Biswas. Retinal vessel segmentation under extreme low annotation: A gan based semi-supervised approach. In 2020 IEEE international conference on image processing (ICIP), pages 418–422. IEEE, 2020.
- Li et al. [2019] Di Li, Dhimas Arief Dharmawan, Boon Poh Ng, and Susanto Rahardja. Residual u-net for retinal vessel segmentation. In 2019 IEEE International Conference on Image Processing (ICIP), pages 1425–1429. IEEE, 2019.
- Li et al. [2020] Wei Li, Mingquan Zhang, and Dali Chen. Fundus retinal blood vessel segmentation based on active learning. In 2020 International conference on computer information and big data applications (CIBDA), pages 264–268. IEEE, 2020.
- Liskowski and Krawiec [2016] Paweł Liskowski and Krzysztof Krawiec. Segmenting retinal blood vessels with deep neural networks. IEEE transactions on medical imaging, 35(11):2369–2380, 2016.
- Liu et al. [2019] Bo Liu, Lin Gu, and Feng Lu. Unsupervised ensemble strategy for retinal vessel segmentation. In Medical Image Computing and Computer Assisted Intervention–MICCAI 2019: 22nd International Conference, Shenzhen, China, October 13–17, 2019, Proceedings, Part I 22, pages 111–119. Springer, 2019.
- Liu et al. [2021] Ze Liu, Yutong Lin, Yue Cao, Han Hu, Yixuan Wei, Zheng Zhang, Stephen Lin, and Baining Guo. Swin transformer: Hierarchical vision transformer using shifted windows. arXiv preprint arXiv:2103.14030, 2021.
- Long et al. [2015] Jonathan Long, Evan Shelhamer, and Trevor Darrell. Fully convolutional networks for semantic segmentation. In Proceedings of the IEEE conference on computer vision and pattern recognition, pages 3431–3440, 2015.
- Ma et al. [2019] Wenao Ma, Shuang Yu, Kai Ma, Jiexiang Wang, Xinghao Ding, and Yefeng Zheng. Multi-task neural networks with spatial activation for retinal vessel segmentation and artery/vein classification. In Medical Image Computing and Computer Assisted Intervention–MICCAI 2019: 22nd International Conference, Shenzhen, China, October 13–17, 2019, Proceedings, Part I 22, pages 769–778. Springer, 2019.
- Ma et al. [2021] Yuhui Ma, Huaying Hao, Jianyang Xie, Huazhu Fu, Jiong Zhang, Jianlong Yang, Zhen Wang, Jiang Liu, Yalin Zheng, and Yitian Zhao. Rose: A retinal oct-angiography vessel segmentation dataset and new model. IEEE Transactions on Medical Imaging, 40(3):928–939, 2021. doi: 10.1109/TMI.2020.3042802.
- Maji et al. [2016] Debapriya Maji, Anirban Santara, Pabitra Mitra, and Debdoot Sheet. Ensemble of deep convolutional neural networks for learning to detect retinal vessels in fundus images. arXiv preprint arXiv:1603.04833, 2016.
- Mendonça et al. [2013] Ana Maria Mendonça, António Sousa, Luís Mendonça, and Aurélio Campilho. Automatic localization of the optic disc by combining vascular and intensity information. Computerized medical imaging and graphics, 37(5-6):409–417, 2013.
- Mookiah et al. [2021] Muthu Rama Krishnan Mookiah, Stephen Hogg, Tom J MacGillivray, Vijayaraghavan Prathiba, Rajendra Pradeepa, Viswanathan Mohan, Ranjit Mohan Anjana, Alexander S Doney, Colin NA Palmer, and Emanuele Trucco. A review of machine learning methods for retinal blood vessel segmentation and artery/vein classification. Medical Image Analysis, 68:101905, 2021.
- Moreno-Ajona et al. [2020] David Moreno-Ajona, James Alexander McHugh, and Jan Hoffmann. An update on imaging in idiopathic intracranial hypertension. Frontiers in Neurology, 11:453, 2020.
- Morgan et al. [2016] William H Morgan, Martin L Hazelton, and Dao-Yi Yu. Retinal venous pulsation: Expanding our understanding and use of this enigmatic phenomenon. Progress in retinal and eye research, 55:82–107, 2016.
- Naseer et al. [2021] Muhammad Muzammal Naseer, Kanchana Ranasinghe, Salman H Khan, Munawar Hayat, Fahad Shahbaz Khan, and Ming-Hsuan Yang. Intriguing properties of vision transformers. Advances in Neural Information Processing Systems, 34:23296–23308, 2021.
- Neimark et al. [2021] Daniel Neimark, Omri Bar, Maya Zohar, and Dotan Asselmann. Video transformer network. arXiv preprint arXiv:2102.00719, 2021.
- Niemeijer et al. [2004] Meindert Niemeijer, Joes Staal, Bram Van Ginneken, Marco Loog, and Michael D Abramoff. Comparative study of retinal vessel segmentation methods on a new publicly available database. In Medical imaging 2004: image processing, volume 5370, pages 648–656. SPIE, 2004.
- Niemeijer et al. [2011] Meindert Niemeijer, Xiayu Xu, Alina V Dumitrescu, Priya Gupta, Bram Van Ginneken, James C Folk, and Michael D Abramoff. Automated measurement of the arteriolar-to-venular width ratio in digital color fundus photographs. IEEE Transactions on medical imaging, 30(11):1941–1950, 2011.
- Oliveira et al. [2018] Américo Oliveira, Sergio Pereira, and Carlos A Silva. Retinal vessel segmentation based on fully convolutional neural networks. Expert Systems with Applications, 112:229–242, 2018.
- Prentašić et al. [2013] Pavle Prentašić, Sven Lončarić, Zoran Vatavuk, Goran Benčić, Marko Subašić, Tomislav Petković, Lana Dujmović, Maja Malenica-Ravlić, Nikolina Budimlija, and Rašeljka Tadić. Diabetic retinopathy image database (dridb): a new database for diabetic retinopathy screening programs research. In 2013 8th International Symposium on Image and Signal Processing and Analysis (ISPA), pages 711–716. IEEE, 2013.
- Qureshi et al. [2013] Touseef Ahmad Qureshi, Maged Habib, Andrew Hunter, and Bashir Al-Diri. A manually-labeled, artery/vein classified benchmark for the drive dataset. In Proceedings of the 26th IEEE international symposium on computer-based medical systems, pages 485–488. IEEE, 2013.
- Ramos-Soto et al. [2021] Oscar Ramos-Soto, Erick Rodríguez-Esparza, Sandra E Balderas-Mata, Diego Oliva, Aboul Ella Hassanien, Ratheesh K Meleppat, and Robert J Zawadzki. An efficient retinal blood vessel segmentation in eye fundus images by using optimized top-hat and homomorphic filtering. Computer Methods and Programs in Biomedicine, 201:105949, 2021.
- Ren et al. [2015] Shaoqing Ren, Kaiming He, Ross Girshick, and Jian Sun. Faster r-cnn: Towards real-time object detection with region proposal networks. Advances in neural information processing systems, 28, 2015.
- Ronneberger et al. [2015] Olaf Ronneberger, Philipp Fischer, and Thomas Brox. U-net: Convolutional networks for biomedical image segmentation. In Medical Image Computing and Computer-Assisted Intervention–MICCAI 2015: 18th International Conference, Munich, Germany, October 5-9, 2015, Proceedings, Part III 18, pages 234–241. Springer, 2015.
- Sathananthavathi and Indumathi [2021] Vallikutti Sathananthavathi and G Indumathi. Encoder enhanced atrous (eea) unet architecture for retinal blood vessel segmentation. Cognitive Systems Research, 67:84–95, 2021.
- Schneider et al. [2012] Caroline A Schneider, Wayne S Rasband, and Kevin W Eliceiri. Nih image to imagej: 25 years of image analysis. Nature methods, 9(7):671–675, 2012.
- Shelhamer et al. [2017] Evan Shelhamer, Jonathan Long, and Trevor Darrell. Fully convolutional networks for semantic segmentation. IEEE transactions on pattern analysis and machine intelligence, 39(4):640–651, 2017.
- Sheng et al. [2023] Hongwei Sheng, Xin Yu, Feiyu Wang, MD Khan, Hexuan Weng, Sahar Shariflou, and S Mojtaba Golzan. Autonomous stabilization of retinal videos for streamlining assessment of spontaneous venous pulsations. arXiv preprint arXiv:2305.06043, 2023.
- Shin et al. [2019] Seung Yeon Shin, Soochahn Lee, Il Dong Yun, and Kyoung Mu Lee. Deep vessel segmentation by learning graphical connectivity. Medical image analysis, 58:101556, 2019.
- Soares et al. [2006] João VB Soares, Jorge JG Leandro, Roberto M Cesar, Herbert F Jelinek, and Michael J Cree. Retinal vessel segmentation using the 2-d gabor wavelet and supervised classification. IEEE Transactions on medical Imaging, 25(9):1214–1222, 2006.
- Son et al. [2019] Jaemin Son, Sang Jun Park, and Kyu-Hwan Jung. Towards accurate segmentation of retinal vessels and the optic disc in fundoscopic images with generative adversarial networks. Journal of digital imaging, 32(3):499–512, 2019.
- Soomro et al. [2019] Toufique Ahmed Soomro, Ahmed J Afifi, Junbin Gao, Olaf Hellwich, Lihong Zheng, and Manoranjan Paul. Strided fully convolutional neural network for boosting the sensitivity of retinal blood vessels segmentation. Expert Systems with Applications, 134:36–52, 2019.
- Staal et al. [2004] Joes Staal, Michael D Abràmoff, Meindert Niemeijer, Max A Viergever, and Bram Van Ginneken. Ridge-based vessel segmentation in color images of the retina. IEEE transactions on medical imaging, 23(4):501–509, 2004.
- Strudel et al. [2021] Robin Strudel, Ricardo Garcia, Ivan Laptev, and Cordelia Schmid. Segmenter: Transformer for semantic segmentation. In Proceedings of the IEEE/CVF International Conference on Computer Vision, pages 7262–7272, 2021.
- Sule and Viriri [2020] Olubunmi Sule and Serestina Viriri. Enhanced convolutional neural networks for segmentation of retinal blood vessel image. In 2020 Conference on Information Communications Technology and Society (ICTAS), pages 1–6. IEEE, 2020.
- Tang et al. [2019] Peng Tang, Qiaokang Liang, Xintong Yan, Dan Zhang, Gianmarc Coppola, and Wei Sun. Multi-proportion channel ensemble model for retinal vessel segmentation. Computers in biology and medicine, 111:103352, 2019.
- Tchinda et al. [2021] Beaudelaire Saha Tchinda, Daniel Tchiotsop, Michel Noubom, Valerie Louis-Dorr, and Didier Wolf. Retinal blood vessels segmentation using classical edge detection filters and the neural network. Informatics in Medicine Unlocked, 23:100521, 2021.
- Wang et al. [2016] Limin Wang, Yuanjun Xiong, Zhe Wang, Yu Qiao, Dahua Lin, Xiaoou Tang, and Luc Van Gool. Temporal segment networks: Towards good practices for deep action recognition. In European conference on computer vision, pages 20–36. Springer, 2016.
- Wei et al. [2021] Jiahong Wei, Guijie Zhu, Zhun Fan, Jinchao Liu, Yibiao Rong, Jiajie Mo, Wenji Li, and Xinjian Chen. Genetic u-net: automatically designed deep networks for retinal vessel segmentation using a genetic algorithm. IEEE Transactions on Medical Imaging, 41(2):292–307, 2021.
- Wintergerst et al. [2020] Maximilian WM Wintergerst, Divyansh K Mishra, Laura Hartmann, Payal Shah, Vinaya K Konana, Pradeep Sagar, Moritz Berger, Kaushik Murali, Frank G Holz, Mahesh P Shanmugam, et al. Diabetic retinopathy screening using smartphone-based fundus imaging in india. Ophthalmology, 127(11):1529–1538, 2020.
- Wong et al. [2006] Tien Yin Wong, FM Amirul Islam, Ronald Klein, Barbara EK Klein, Mary Frances Cotch, Cecilia Castro, A Richey Sharrett, and Eyal Shahar. Retinal vascular caliber, cardiovascular risk factors, and inflammation: the multi-ethnic study of atherosclerosis (mesa). Investigative ophthalmology & visual science, 47(6):2341–2350, 2006.
- Wu et al. [2016] Aaron Wu, Ziyue Xu, Mingchen Gao, Mario Buty, and Daniel J Mollura. Deep vessel tracking: A generalized probabilistic approach via deep learning. In 2016 IEEE 13th International Symposium on Biomedical Imaging (ISBI), pages 1363–1367. IEEE, 2016.
- Xu et al. [2022] Rui Xu, Jiaxin Zhao, Xinchen Ye, Pengcheng Wu, Zhihui Wang, Haojie Li, and Yen-Wei Chen. Local-region and cross-dataset contrastive learning for retinal vessel segmentation. In Medical Image Computing and Computer Assisted Intervention–MICCAI 2022: 25th International Conference, Singapore, September 18–22, 2022, Proceedings, Part II, pages 571–581. Springer, 2022.
- Yu et al. [2014] Dao-Yi Yu, K Yu Paula, Stephen J Cringle, Min H Kang, and Er-Ning Su. Functional and morphological characteristics of the retinal and choroidal vasculature. Progress in Retinal and Eye Research, 40:53–93, 2014.
- Zhang et al. [2016] Jiong Zhang, Behdad Dashtbozorg, Erik Bekkers, Josien PW Pluim, Remco Duits, and Bart M ter Haar Romeny. Robust retinal vessel segmentation via locally adaptive derivative frames in orientation scores. IEEE transactions on medical imaging, 35(12):2631–2644, 2016.
- Zhang et al. [2020] Mo Zhang, Fei Yu, Jie Zhao, Li Zhang, and Quanzheng Li. Befd: Boundary enhancement and feature denoising for vessel segmentation. In Medical Image Computing and Computer Assisted Intervention–MICCAI 2020: 23rd International Conference, Lima, Peru, October 4–8, 2020, Proceedings, Part V 23, pages 775–785. Springer, 2020.
- Zhang and Chung [2018] Yishuo Zhang and Albert CS Chung. Deep supervision with additional labels for retinal vessel segmentation task. In Medical Image Computing and Computer Assisted Intervention–MICCAI 2018: 21st International Conference, Granada, Spain, September 16-20, 2018, Proceedings, Part II 11, pages 83–91. Springer, 2018.
- Zhao et al. [2019] Yitian Zhao, Jianyang Xie, Huaizhong Zhang, Yalin Zheng, Yifan Zhao, Hong Qi, Yangchun Zhao, Pan Su, Jiang Liu, and Yonghuai Liu. Retinal vascular network topology reconstruction and artery/vein classification via dominant set clustering. IEEE transactions on medical imaging, 39(2):341–356, 2019.
- Zhou et al. [2020] Chao Zhou, Xiaogang Zhang, and Hua Chen. A new robust method for blood vessel segmentation in retinal fundus images based on weighted line detector and hidden markov model. Computer methods and programs in biomedicine, 187:105231, 2020.
- Zhou et al. [2017] Lei Zhou, Qi Yu, Xun Xu, Yun Gu, and Jie Yang. Improving dense conditional random field for retinal vessel segmentation by discriminative feature learning and thin-vessel enhancement. Computer methods and programs in biomedicine, 148:13–25, 2017.