Relaxation dynamics of SIR-flocks with random epidemic states
Abstract.
We study the collective dynamics of a multi-particle system with three epidemic states as an internal state. For the collective modeling of active particle system, we adopt modeling spirits from the swarmalator model and the SIR epidemic model for the temporal evolution of particles’ position and internal states. Under suitable assumptions on system parameters and non-collision property of initial spatial configuration, we show that the proposed model does not admit finite-time collisions so that the standard Cauchy-Lipschitz theory can be applied for the global well-posedness. For the relaxation dynamics, we provide several sufficient frameworks leading to the relaxation dynamics of the proposed model. The proposed sufficient frameworks are formulated in terms of system parameters and initial configuration. Under such sufficient frameworks, we show that the state configuration relaxes to the fixed constant configuration via the exponentially perturbed gradient system and explicit dynamics of the SIR model. We present explicit lower and upper bounds for the minimal and maximal relative distances.
Key words and phrases:
SIR model, swarmalator, epidemic2020 Mathematics Subject Classification:
70G60, 34D06, 70F10
1. Introduction
The purpose of this paper is to continue the studies begun in [19, 20] on the collective dynamcis modeling of active particles with internal states. Collective behaviors of complex systems are ubiquitous in nature, e.g., crowd dynamics [2], aggregation of bacteria [8, 9, 10, 26], flocking of birds [5, 6, 12, 13, 15, 22, 23, 24, 29, 30, 34, 37], synchronization of fireflies [11, 38] and swarming of fish [16], etc. See survey articles [1, 3, 7, 17, 21, 33, 36]. In recent years, thanks to the emerging applications to the decentralized control of multi-particle systems, collective behaviors have received a lot of attention from diverse scientific disciplines such as applied mathematics, biology, control theory and statistical physics etc. In this work, we are interested in the first-order modeling of active particles with random epidemic states (susceptible(), infected() and recovered()) as an internal state, i.e., we assume that particle can take aforementioned three epidemic states with certain probabilities denoted by and whose precise meaning will be clarified in a minute. Thus, the state of particle is represented by position vector and probability vector for epidemic states , respectively. In what follows, we briefly describe how to model the dynamics of position vectors and epidemic vectors via continuous dynamical systems. Let be the size of system, i.e., the total number of particles in a given ensemble .
First, we use the position dynamics of the swarmalator model [31, 32] which describes the attractive and repulsive forces:
(1.1) |
where are nonnegative coupling strengths, and represent attractive and repulsive weights whose explicit functional forms will be discussed in Section 3, and we assume that positive system parameters satisfy the relation:
(1.2) |
so that repulsive force is dominant in a small relative distance regime. Here denotes the standard -norm in .
Next, we use the modeling spirit of the SIR model for the dynamics of epidemic state . We introduce convex set consisting of all admissible state vectors:
(1.3) |
and we set
and
Then, we assume that the dynamics of is governed by the following coupled system:
(1.4) |
Finally, we couple two systems (1.1) and (1.4) via nonnegative system functions and by imposing suitable functional dependences between position variable and internal variable :
(1.5) |
Moreover, we also assume that there exist positive constants and such that
Finally, we combine all three ingredients (1.1), (1.4) and (1.5) together to write down the dynamical system for with suitable initial data:
(1.6) |
Throughout the paper, we call the coupled system (1.6) as the “SIR-flock model” for simplicity.
The goal of this work is to provide sufficient frameworks leading to the emergent dynamics of the SIR-flock model (1.6). Now, we briefly discuss four main results.
First, we show that if spatial configuration is noncollisional initially, there will be no finite-time collisions so that system (1.6) is globally well-posed by the Cauchy-Lipschitz theory (see Theorem 2.2).
Second, we present a sufficient conditions for the relaxation of epidemic state toward a constant epidemic state. More specifically, our sufficient framework for the relaxation of is expressed in terms of network topology and a recovering vector :
(1.7) |
where is a positive constant and is a nonnegative constant. Under this framework (1.7), we show that the epidemic state relaxes to a constant state (see Theorem 4.1): there exist a constant state and such that
Third, we show that if initial configuration and system parameters satisfy suitable conditions, then minimal and maximal relative distances are positive uniformly in time, i.e., there exist positive constants and such that
see Theorem 5.1.
Fourth, we show that the spatial configuration relaxes to a constant configuration asymptotically under a suitable condition on the initial configuration and system parameters (see Theorem 6.1).
The rest of this paper is organized as follows. In Section 2, we briefly review basic properties of the SIR epidemic model and the swarmalator model on the asymptotic relaxation of state variables and present a global well-posedness by verifying nonexistence of finite-time collisions. In Section 3, we discuss the modeling spirit of system parameters and coupling functions. In Section 4, we study the relaxation of epidemic states toward a constant state and in particular, we provide a sufficient condition leading to asymptotic removal of infected particles. In Section 5, we study the existence of positive lower bound and upper bound for the minimal and maximal relative distances, respectively. In Section 6, we study a relaxation of spatial configuration toward a fixed spatial configuration using the perturbed gradient flow theory. In Section 7, we provide several numerical examples and compare them with analytical results obtained in previous sections. Finally, Section 8 is devoted to a brief summary of our main results and some remaining issues for a future work.
2. Preliminaries
In this section, we briefly review basic properties on two related models “the SIR epidemic model” and “the swarmalator model” which correspond to the subsystems of system (1.6).
2.1. The SIR epidemic model
In this subsection, we briefly discuss the SIR model [4, 35] which is a prototype model for the spread of disease or virus. Kermack and McKendrick’s work in 1927 motivated a large number of modelings in epidemics [25]. In particular, there are some of the works for diffusive SIR models on stability and numerical methods [18, 14, 28]. First, we introduce the following three observables:
Then, we assume that the state is governed by the Cauchy problem to the coupled system of ODEs:
(2.1) |
where and are positive constants. This model was designed to explain the spreading of epidemic diseses. We can express the SIR model via the following pictorial diagram.
Next, we list basic properties of the SIR model in the following lemma.
Proposition 2.1.
Proof.
(ii) We integrate the first two equations in (2.1) to obtain
(2.3) |
The nonnegativity of follows from the third equation and the nonnegativity of :
On the other hand, it follows from (2.2) and (2.3) that is bounded by :
Since and is bounded above by 1, there exists an asymptotic constant state such that
(2.4) |
(iii) By , one has
∎
2.2. The swarmalator model
Let and be the position and phase of the -th swarmalator, respectively. Then, its dynamics is governed by the Cauchy problem to the Kuramoto-type swarmalator model:
(2.5) |
Here, constants and are the natural velocity and frequency of the -th particle, respectively, denotes the standard Euclidean -norm in . System parameters and interaction functions and satisfy
(2.6) |
If we simply set , system becomes the Kuramoto model [27]:
Due to the singular terms in the swarmalator model , it is important to make sure that there is no finite-time collision which can be quantified in the following proposition.
First, we set several parameters:
(2.7) |
Next, we state two results on the positivity of minimal and maximal distance between particles.
Proposition 2.2.
Proposition 2.3.
Proof.
For a proof, we refer to [20]. ∎
Consider the following ansatz for and :
In this case, system (2.5) becomes
(2.10) |
By Proposition 2.1 and convergence result of the perturbed gradient system, system (2.10) exhibits phase synchronization.
Theorem 2.1.
Proof.
For a proof, we refer to Section 4.2 of [20]. ∎
2.3. A global well-posedness
In this subsection, we discuss a global well-posedness of system (1.6). Since the R.H.S. of contains the term in the denominators, as long as we can rule out the possibility of finite-time collisions, we obtain a global well-posedness using the standard Cauchy-Lipschitz theory. In the sequel, we show that system (1.6) does not admit a finite-time collision, as long as there is no collisions initially. This will be done using a contradiction argument and Gronwall’s inequality.
Suppose that initial spatial configuration satisfy
Then, by the continuity of solution, there will be no collisions between particles at least small time interval with . Then, using the Cauchy-Lipschitz theory, we can show that system (1.6) has a local smooth solution in the time-interval . Now, in order to show a global well-posedness, it suffices to show that there will be no finite-time collisions. Suppose there is a finite-time collision and let be the first collision time. We take one of the particles that make a collision and fix it by . Then, we define a set containing all the particles involved in the collision at time by . Now, we set the following handy notation:
Then, it is easy to see that
(2.11) |
By direct calculations, one has
(2.12) |
In the following lemma, we provide estimates for .
Lemma 2.1.
The term with satisfies
where is the cardinality of the set and .
Proof.
We provide estimate for () as follows:
(i) (Estimate of ): Note that
Therefore, one has
(2.13) |
Similarly, one has
(2.14) |
We combine (2.13) and (2.14) to obtain
(ii) (Estimate of ): Since , one has
where we used the Cauchy-Schwarz inequality to see
Similarly, one has
∎
It follows from (2.12) and Lemma 2.1 that
(2.15) |
where are positive constants defined as follows.
On the other hand, since in (1.2), there exists a small positive constant such that for ,
(2.16) |
Therefore, we combine (2.15) and (2.16) to find
Moreover, there exists a positive constant such that for ,
Thus, we have
i.e.,
We integrate the above differential inequality from to to get
On the other hand, it follows from (2.11) that the left-hand side is zero, but the right-hand side is strictly positive, which is contradictory. Therefore, there are no finite-time collisions between particles from the well-prepared initial configuration. Finally, we can summarize the previous argument as follows.
Theorem 2.2.
(A global well-posedness) Suppose that initial spatial configuration is noncollisional in the sense that
Then, there exists a unique global solution to system (1.6) in any finite-time interval.
3. Modeling of system functions
In this section, we discuss meaning of the system parameters and system functions appearing in the SIR-flock (1.6):
3.1. Interaction topology and recovering vector
For the modeling purpose, we assume that the nonnegative value depends on the relative distance between the -th particle and the -th particle. To be definiteness, we set
(3.1) |
where is a positive constant and is a nonnegative constant. Since the -th particle can not be affected by itself, we assume the diagonal entries of are zero:
Now we discuss the natural recovering vector . Suppose that every particles have own immune system, the disease will disappear automatically. Thus, we assume
If the immune system of the -th particle is well-functioning, then has a large value. In contrast, if the immune system of the -th particle is not well-functioning, then will have a small value. In this work, we assume that the natural recovering vector is a positive constant (see the following figure in the sequel).
3.2. Coupling weight functions
Recall that the dynamics of is governed by the following system:
(3.2) |
Now, the matter of question is how to model and in terms of s.
If two state vectors and are similar, then particles and will attract each others, whereas if two state vectors and are dissimilar, then and will repel each other. For definiteness, we set
(3.3) |
where and are positive constants. Here and are positive constants presenting social distancing. These social distancing constants play an important role in preventing collisions between particles. Note that the ansatz (3.3) satisfies symmetry:
(3.4) |
Next, we will impose conditions on and later in (3.2). To illustrate the functional relations in the right-hand side of (3.3), we consider an ensemble which is partitioned into two sub-ensembles:
Recall that and are probabilities that -th particle are in infected state and are not in infected state, respectively.
Consider the inner product-like function as follows:
(3.5) |
Note that becomes larger when and . If and are similar, then becomes larger, and one has
(3.6) |
Now, we set
(3.7) |
Then, and satisfy the following monotonicity properties:
-
(1)
If and become similar, increases and decreases.
-
(2)
If and become dissimilar, decreases and increases.
The relations (3.5) and (3.7) yield (3.3). On the other hand, it follows from (3.3) and (3.6) that the coupling weight functions and admit positive lower bound and upper bounds:
(3.8) |
Proposition 3.1.
3.3. Symptom expression vector
Next, we introduce the symptom expression vector motivated by the ongoing pandemic COVID-19. In April 2020, the daily COVID-19 confirmed number per day of Korea was less than 20. Most of confirmed people were isolated, however patients with no symptoms of COVID-19 were still spreading the virus. That is why the daily confirmed number per day does not goes to 0 directly. So we need to consider the ratio of symptom expressions. Some people show symptoms well when they got the virus, in contrast some people does not show any symptoms although they got the virus. So even for the same inputs, different degree of output can emerge. Thus, we define the symptom expression vector that are related to previous phenomena. We define define each component to following the following properties:
-
•
If the -th particle shows the symptom well, then we put large value to .
-
•
If the -th particle does not show the symptom well, then we put small value to .
We may use the symptom expression vector to express the condition of being suspected as a patient. In this paper, we assume that the symptom expression vector is a time-independent constant vector. We assume that the condition of being suspected as a patient only depends on the product , and we also define some fixed threshold constant to make decision. If , then the person will be confirmed at time .
4. Relaxation of epidemic states
In this section, we study the relaxation dynamics of the SIR-flock model (1.6). First, we show that is invariant along (1.6).
Lemma 4.1.
Proof.
We basically use the same arguments as in Proposition 2.1.
Now, we need to show the positivity of and .
(Positivity of ): Due to (4.2), it suffices to check the positivity of and . Now, we integrate to find
Thus, one has
(Positivity of ): It follows from (2.1)2 that
This yields
Now, we introduce a time-varying minimal index such that
Since each is analytic, there exists the refinement of time-interval
such that the index of is not changed on each interval , and
Therefore, we have
This yields
Therefore, one has
(Positivity of ): Since and , one has
Therefore, we combine all the estimates for and to get
Now, we discuss the asymptotic convergence of the probability vector toward a constant probability vector in (1.4).
Theorem 4.1.
Let be a solution of system (1.6). Then, the following assertions hold.
-
(1)
There exists a constant state with such that
-
(2)
If the recovering value satisfies
(4.3) then there exists a positive constant such that
Proof.
(i) Since and are non-negative,
which means is non-increasing. Since is bounded below by zero, there are some constants that converges to , as goes by monotone convergence theorem. Similarly,
and is non-decreasing and bounded above, there are some constants that converges to , as goes . Moreover, as converges,
(ii) Recall the equation for :
Now, we use the method of integrating factor to derive Gronwall’s inequality for :
Thus, we have
This implies
∎
Next, under a more relaxed condition compared to (4.3), we improve the second result of Theorem 4.1 as follows.
Corollary 4.1.
Let be the solution of the system (1.6). If satisfies following condition:
(4.4) |
then decays to zero exponentially fast.
Proof.
First, we write the dynamics of s in matrix form:
where and are given as follows.
Since each of is decreasing,
This implies that for every ,
where is a partial order compoentwise, and
Therfore, we obtain
(4.5) |
We multiply the both sides of (4.5) by to yield
where is given as follows.
If
then is negative, so decays to zero exponentially fast. ∎
5. Quantitative estimates for relative distances
In this section, we provide explicit quantitative estimates on relative distances between particles. For a given spatial configuration , we consider the set of all relative distances for , and rearrange them in an increasing order:
In what follows, we derive a uniform lower-bound for and a uniform upper-bound for so that we can control the singular terms in (1.6) uniformly in time. First, we recall notation:
(5.1) |
Theorem 5.1.
Let be a solution of system (1.6) with the initial data . Then, the following assertions hold.
-
(1)
(Existence of a positive lower bound to ): If initial data satisfy
there is a positive constant such that
-
(2)
(Existence of a positive upper bound to ): If initial data and system functions satisfy
there exists a positive constant such that
Proof.
We leave its proof in the next two subsections. ∎
5.1. A positive lower bound for minimal relative distance
In this subsection, we show that has a positive lower bound which is independent of . Since the particles do not collide in finite-time interval, there exists an analytic solution in finite time (see Theorem 2.2). Therefore, each distance and ordered distance are Lipschitz continuous. In addition, according to the analyticity, for given , there exist a sequence of times :
such that we can decompose the whole time interval into the union of subintervals
to make sure that is not changed in each subinterval. To demonstrate the existence of positive uniform lower bound, we first provide three lemmas. First, we show a positive lower bound of maximal distance :
(5.2) |
Next, we provide a series of lemmas to estimate maximal and minimal relative distances. First, we derive a differential inequality for .
Proof.
For given , we choose and such that
Then, by direct calculation, we obtain
(5.3) |
for . We now estimate () one by one.
(Estimate of ): We use
to get
(5.4) |
(Estimate of ) : Since is the maximal distance,
Similarly, we have
Therefore, one has
(5.5) |
To derive a positive lower bound for , we first verify that the maximal distance is bounded away from zero uniformly in time, and then we show that this positive lower bound propagates to the lower graded relative distance when we reach to the minimal relative distance (the first assertion in Theorem 5.1) via the following two lemmas.
Now we derive a positive lower bound for the maximal distance .
Lemma 5.2.
(maximal relative distance) Suppose that initial spatial configuration is non-collisional:
and let be a solution to system (1.6). Then, one has
Proof.
We use an induction argument on the time intervals .
(Initial step): we claim that
Suppose not, since , there exist and such that
Therefore, for ,
For all , we get
By Lemma 4.1, one has
This implies
which is contradictory to
(Inductive step) : Suppose that for some ,
Now we consider on . Due to the continuity of and induction hypothesis, one has
Therefore, we can use the same criteria above to get
Thus, one has the desired estimate. ∎
In the following lemma, we show the backward propagation of a positive lower bound for the ordered relative distance.
Lemma 5.3.
(Backward propagation of lower bounds) Suppose that initial spatial configuration is non-collisional:
and let be a solution to system (1.6), and let be a fixed constant. If there exist a positive constant such that
then there exists a positive constant such that
Proof.
We refer to the proof of Lemma 3.4 in [20]. ∎
Now, we are ready to provide a positive lower bound for the minimal relative distance .
Proof of the first assertion in Theorem 5.1: Suppose initial spatial configuration is noncollisional:
and let be a solution to system (1.6). By Lemma 5.2, there exists such that
For , we apply Lemma 5.3 to show that
Again, we apply Lemma 5.3 inductively until we reach to derive the desired a positive lower bound for . ∎
5.2. A positive upper bound for maximal relative distance
In this subsection, we derive an existence of a positive upper bound for the maximal relative distance. First, we study the estimate for a differential inequality.
Lemma 5.4.
Let be a differentiable function satisfying the following differential inequality:
where constants and satisfy
Then, there exists a positive constant such that
Proof.
We use phase line analysis for . For this, we define
Then, it is easy to see that
Therefore, is uniformly bounded by . ∎
Now, we are ready to provide a proof for the second assertion in Theorem 5.1.
Proof of the second assertion in Theorem 5.1: Suppose initial data and system parameters satisfy
and let be a solution to system (1.6). Then, for , we choose and such that
By Lemma 5.2, one has
(5.7) |
In what follows, we estimate () one by one.
(Estimate of ): We use
to get
(5.8) |
(Estimate of ): We use to see
Now, we define the angle between and by . Since
one has
Thus, one has
Now, we define the function
Then, we have
Since
we have
(5.9) |
(Estimate of ): Similar to , we have
(5.10) |
Finally, in (LABEL:E-5), we combine all the estimates (5.8), (5.9) and (5.10) to find
or equivalently
Now, we apply Lemma 5.4 with parameters
to derive the desired estimate.
Remark 5.1.
Note that for , nonexistence of finite-time collisions and uniform lower bound of diameter are always warranted, however conditions on parameter is needed to guarantee uniform upper bound of diameter.
6. Relaxation of spatial configuration
In this section, we study the relaxation of spatial configuration toward a constant configuration.
Consider a perturbed gradient system with decaying forcing :
(6.1) |
where is a one-body potential.
Proposition 6.1.
Suppose that external forcing and potential satisfy the following conditions:
-
(1)
There exist positive constants and such that
-
(2)
There exists a compact set such that is contained in for all ,
-
(3)
V is analytic in and the map is uniformly continuous in time,
and let be a solution of (6.1). Then, there exists such that
Proof.
We refer to [20] for a proof. ∎
Now we are ready to prove the convergence of spatial configuration.
Theorem 6.1.
Proof.
We consider three cases below.
Case A ( and ): We set the potential function and the function by
Note that is in when each of is dimensional. First of all, we claim that the analyticity of the potential function defined in . we can restrict the solution space because of the existence of uniform lower bound of distance. We may exclude the hyperplanes for all . So we write the restricted space as a union of open connected domain
By the continuity of the solution, are invariant sets for . Without loss of generality, we may assume and thus . That is is an open domain and is analytic on .
Now, we will show that there exists positive constants and such that
Note that we have verified that there exists a positive constant such that
It’s clear that for all ,
In addition, one has
This means and decay in exponential order. Since we have uniform upper and lower bounds of , there exist positive constants and such that
Lastly, upper and lower bounds guarantee the boundedness of and . Similarly, is bounded too. Thus we get uniform continuity of in time. Thus, every hypothesis in Proposition 6.1 is satisfied to get that converges.
Case B : By setting
we can prove the desired estimate similarly as in Case A.
Case C : Similar to Case B, we can show the desired estimate with
∎
Next, we consider a two-particle system with the following initial SIR state:
and we set
Consider a system for spatial position:
Note that since , the center of the two particles is constant:
Now, we set
and derive the equation for :
As long as each coordinate of is positive,
Thus, one has
so the difference has upper and lower bounds that are uniform in time.
It follows from Lemma 5.2 that for ,
and . Therefore, has both upper and lower bounds and by the same reason in Theorem 6.1, there exists such that converges, as tends to infinity.
Now, we consider an equilibrium :
Thus we have
This yields
Since , we have
In addition, we can weaken the condition that guarantees the exponential decay of .
Theorem 6.2.
Suppose system parameters satisfy
and let be a solution to system (2.1). Then, there exist positive constants and such that
where is a positive constant defined by
Proof.
Note that
Thus, for every , there exists positive constant with respect to such that
By the given condition, there exists a positive constant such that
For ,
This yields
∎
Remarks.
Since
we get
The Jacobian matrix of system at is
Next, we consider two cases for .
Case A: Consider the case
In this case, all eigenvalues are real and eigenvalues for and are negative. This means that and decay to exponentially fast.
Case B: Consider the case
The right-hand side is less or equal to
This yields
Note that the left-hand side denotes the number of total infections. Thus, we can say that decays to zero exponentially fast, or the total number of total infection is bounded.
7. Numerical Simulations
In this section, we provide several numerical examples to confirm analytical convergence results that we have shown in previous sections. At first, we set initial condition by for uninfected 16 people and for infected 4 people. Initial location is set by random seed in 3 by 3 plane. We set
and we use the fourth order Runge-Kutta method for all simulations.
(Convergence of ): In Figure 1 and Figure 2, we used
here. In Theorem 4.1, we have shown that , , and converges. To authenticate this assertion by numerical method, we observed convergence of , , and .
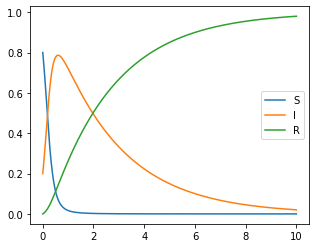
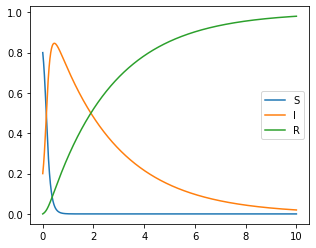
Moreover, by observing , , and that converges to zero, we obtain that and converge.
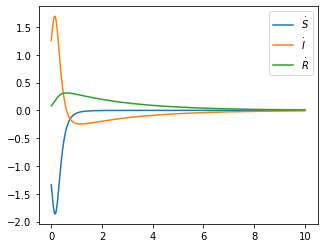
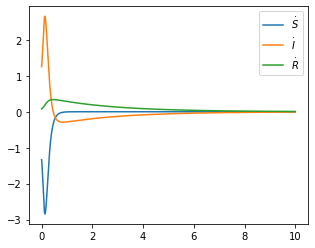
(Exponential decay of ): For arbitrary , we showed that decays to zero exponentially fast when as in Theorem 4.1. In Figure 3 and Figure 4, we set
and observed two simulations based on two set of system parameters:
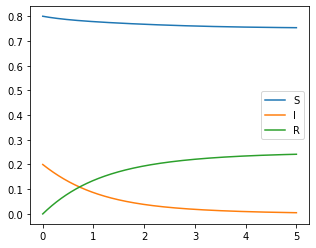
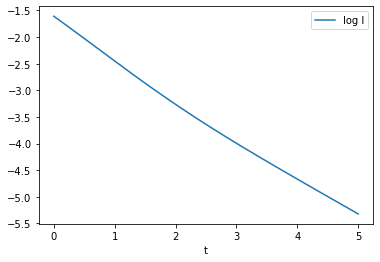
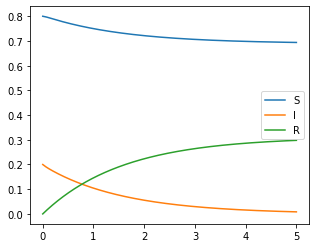
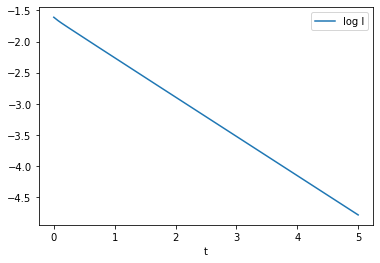
(Effect of social distancing): Since and are coefficients of attracting and repulsion forces, respectively, larger ratio represents for more intensive social distancing. We observe that the maximal value of decreases, as increases. We set and , and changed values of and with . We plot graph for the cases of and .
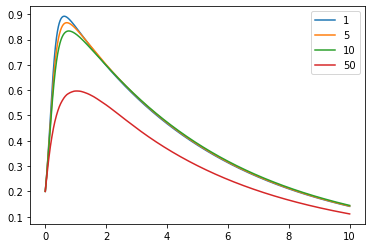
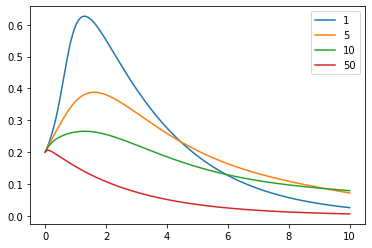
(Behavior of the particles): We observed that infectious particles aggregated and they were isolated from non-infectious factors. It implies that this model can effectively reduce the number of infectious particles by adjusting the coefficients. We illustrated the behavior of the particles in
and , . The purple ones represents for non-infectious particles. Moreover, we compared with the model that has no attracting and no repulsing term by setting . It is illustrated in the first graph in Figure 6 , red one is for , and blue one is for . We could decrease the average of .
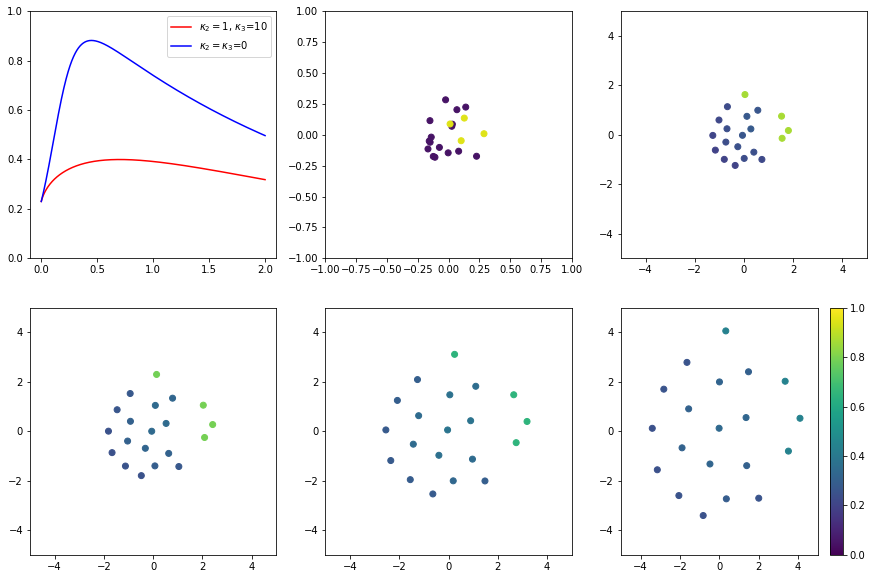
We set
and repeated the process.
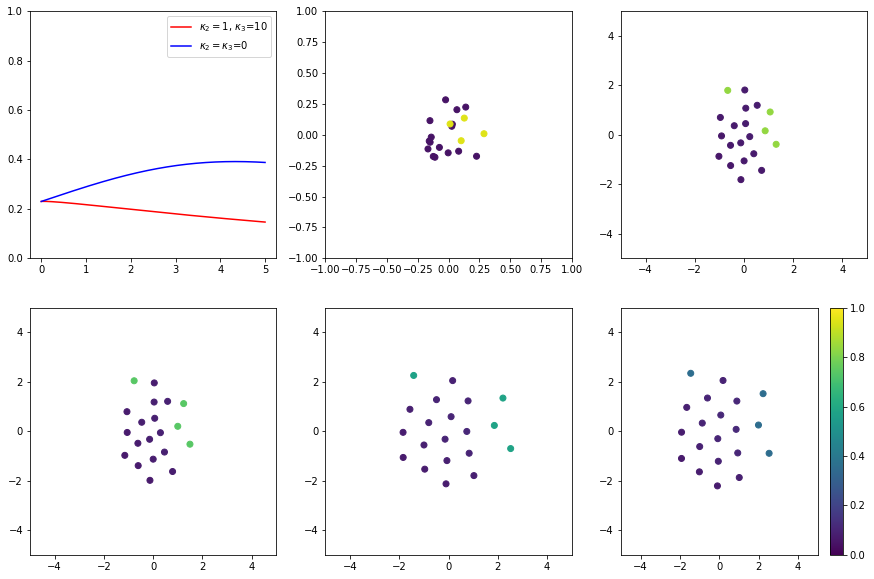
8. conclusion
In this paper, we have studied the emergent behaviors of a flock with SIR internal states, and have presented a new particle model with an aggregate property and epidemic internal forces by combining the SIR model and the swarmalator model. We considered that each particle has a state vector which is a kind of internal state following the SIR dynamics. From this argument, we could obtain the SIR-aggregation model by imposing the repulsive/attractive force between two particles depending on the distance between two particles and the internal states. We imposed strong repulsive force if one of them has a high probability of infection to model the social distancing, in contrast, we imposed weak repulsive force if both of them has low probabilities of infection. From this modeling, we modeled the social distancing of particles. We expect that we can do numeric experiments to find the efficiency of social distance for given strategies. We also provided the theoretical result of the SIR model, for example, nonexistence of finite-time collisions, positive lower bound for minimal relative distance, and a uniform upper bound for spatial diameter. From the numerical simulations, we could check that the isolation of the infected particle is an effective way to reduce the number of infected particles. Since we only considered that the recovered particles were never infected again, we have monotonicity on the number of susceptible/recovered particles. From those properties, we could prove the emergent dynamics. In our proposed model, we did not consider the reinfection which can happen in reality. Thus, we will leave this issue for a future work.
References
- [1] J. A. Acebron, L. L. Bonilla, C. J. P. Pérez Vicente, F. Ritort and R. Spigler, The Kuramoto model: A simple paradigm for synchronization phenomena, Rev. Mod. Phys. 77 (2005) 137–185.
- [2] G. Ajmone Marsan, N. Bellomo and L. Gibelli, Towards a systems approach to behavioral social dynamics, Math. Models Methods Appl. Sci. 26 (2016) 1051–1093.
- [3] G Albi, N. Bellomo, L. Fermo, S.-Y. Ha, J. Kim, L. Pareschi, D. Poyato and J. Soler, Vehicular traffic, crowds, and swarms: From kinetic theory and multiscale methods to applications and research perspectives, Math. Models Methods Appl. Sci. 29 (2019) 1901–2005.
- [4] K. M. Ariful Kabir, K. Kuga and J. Tanimoto, Analysis of SIR epidemic model with information spreading of awareness, Chaos, Solitons and Fractals, 119 (2019), 118-125.
- [5] M. Ballerini, N. Cabibbo, R. Candelier, A. Cavagna, E. Cisbani, I. Giardina, V. Lecomte, A. Orlandi, G. Parisi, A. Procaccini, M. Viale, and V. Zdravkovic, Interaction ruling animal collective behavior depends on topological rather than metric distance: Evidence from a field study, Proc. Natl. Acad. Sci. USA 105 (2008) 1232–1237.
- [6] N. Bellomo and S.-Y. Ha, A quest toward a mathematical theory of the dynamics of swarms, Math. Models Methods Appl. Sci. 27 (2017) 745–770.
- [7] N. Bellomo, S.-Y. Ha and N. Outada, Towards a mathematical theory of behavioral swarms, To appear in ESIAM: Control, Optimization and Calculus of Variations.
- [8] A. L. Bertozzi and J. Brandman, Finite-time blow-up of -weak solutions of an aggregation equation, Commun. Math. Sci. 8 (2010) 45–65.
- [9] A. Bertozzi, J. A. Carrillo and T. Laurent, Blow-up in multidimensional aggregation equations with mildly singular interaction kernels, Nonlinearity 22 (2009), 683–710.
- [10] A. L. Bertozzi, T. Laurent and J. Rosado, theory for the multidimensional aggregation equation, Commun. Pure Appl. Math. 64 (2011) 45–83.
- [11] J. Buck and E. Buck, Biology of sychronous flashing of fireflies, Nature 211 (1966) 562.
- [12] J. A. Carrillo, Y.-P. Choi, P. B. Mucha and Jan Peszek, Sharp conditions to avoid collisions in singular Cucker–Smale interactions, Nonlinear Analysis: Real World Applications 37 (2017) 317–328.
- [13] A. Cavagna, L. D. Castello, I. Giardina, T. Grigera, A. Jelic, S. Melillo, T. Mora, L. Parisi, E. Silvestri, M. Viale and A. M. Walczak, Flocking and turning: a new model for self-organized collective motion, J. Stat. Phys. 158 (2015) 601–627.
- [14] S. Chinviriyasit and W. Chinviriyasit, Numerical modelling of an SIR epidemic model with diffusion, Applied Mathematics and Computation, 216 (2010), 395-409.
- [15] F. Cucker and S. Smale, Emergent behavior in flocks, IEEE Trans. Automat. Control 52 (2007) 852–862.
- [16] P. Degond and S. Motsch, Large-scale dynamics of the Persistent Turing Walker model of fish behavior, J. Stat. Phys. 131 (2008) 989–1022.
- [17] R. Duan, M. Fornasier and G. Toscani, A kinetic flocking model with diffusion, Comm. Math. Phys. 300 (2010) 95–145.
- [18] C. Gai, D. Iron and T. Kolokolnikov, Localized outbreaks in an S-I-R model with diffusion, J. of Math. Bio. 80 (2020) 1389–1411.
- [19] S.-Y. Ha, J. Jung, J. Kim, J. Park and X. Zhang, A mean-field limit of the particle swarmalator model, To appear in Kinetic and Related Models.
- [20] S.-Y. Ha, J. Jung, J. Kim, J. Park and X. Zhang, Emergent behaviors of the swarmalator model for position-phase aggregation, Math. Models Methods Appl. Sci. 29 (2019) 2225–2269.
- [21] S.-Y. Ha, D. Ko, J. Park and X. Zhang, Collective synchronization of classical and quantum oscillators, EMS Surveys in Mathematical Sciences 3 (2016) 209–267.
- [22] S.-Y. Ha and J.-G. Liu, A simple proof of Cucker-Smale flocking dynamics and mean–field limit, Commun. Math. Sci. 7 (2009) 297–325.
- [23] S.-Y. Ha and T. Ruggeri, Emergent dynamics of a thermodynamically consistent particle model, Arch. Ration. Mech. Anal. 223 (2017) 1397–1425.
- [24] S.-Y. Ha and E. Tadmor, From particle to kinetic and hydrodynamic description of flocking, Kinet. Relat. Models 1 (2008) 415–435.
- [25] W. O. Kermack and A. G. McKendrick, A contribution to the mathematical theory of epidemics, Proc. Roy. Soc. Lond. A 115 (1927), 700–721.
- [26] T. Kolokolnikov, J. A. Carrillo, A. Bertozzi, R. Fetecau, M. Lewis, Emergent behaviour in multi-particle systems with non-local interactions, Phys. D 260 (2013), 1–4.
- [27] Y. Kuramoto, International symposium on mathematical problems in mathematical physics, Lecture Notes in Theoretical Physics 30 420 (1975).
- [28] Z. Liu, Z. Shen, H. Wang and Z. Jin, Analysis of a local diffusive SIR model with seasonality and nonlocal incidence of infection, SIAM J. Appl. Math., 79 (2019), 2218-2241.
- [29] S. Motsch and E. Tadmor, Heterophilious dynamics enhances consensus, SIAM Rev. 56 (2014) 577–621.
- [30] S. Motsch and E. Tadmor, A new model for self-organized dynamics and its flocking behavior, J. Stat. Phys. 144 (2011) 923–947.
- [31] K. P. O’Keeffe, J. H. Evers and T. Kolokolnikov, Ring states in swarmalator systems, Phys. Rev. E 98 (2018) 022203.
- [32] K. P. O’Keeffe, H. Hong and S. H. Strogatz, Oscillators that sync and swarm, Nature Communications 8 (2017) 1504.
- [33] A. Pikovsky, M. Rosenblum and J. Kurths, Synchronization: A universal concept in nonlinear sciences, Cambridge University Press, Cambridge, 2001.
- [34] J. Toner and Y. Tu, Flocks, herds, and Schools: A quantitative theory of flocking, Phys. Rev. E 58 (1988) 4828–4858.
- [35] C. Vargas-De-Le’on, On the global stability of SIS , SIR and SIRS epidemic models with standard incidence, Chaos, Solitons and Fractals, 44 (2011), 1106-1110.
- [36] T. Vicsek and A. Zefeiris, Collective motion, Phys. Rep. 517 (2012) 71–140.
- [37] T. Vicsek, Czirók, E. Ben-Jacob, I. Cohen and O. Schochet, Novel type of phase transition in a system of self-driven particles, Phys. Rev. Lett. 75 (1995) 1226–1229.
- [38] A. T. Winfree, Biological rhythms and the behavior of populations of coupled oscillators, J. Theor. Biol. 16 (1967) 15–42.