QueryExplorer: An Interactive Query Generation Assistant for Search and Exploration
Abstract
Formulating effective search queries remains a challenging task, particularly when users lack expertise in a specific domain or are not proficient in the language of the content. Providing example documents of interest might be easier for a user. However, such query-by-example scenarios are prone to concept drift, and the retrieval effectiveness is highly sensitive to the query generation method, without a clear way to incorporate user feedback. To enable exploration and to support Human-In-The-Loop experiments we propose QueryExplorer111https://github.com/emory-irlab/query-explorer – an interactive query generation, reformulation, and retrieval interface with support for HuggingFace generation models and PyTerrier’s retrieval pipelines and datasets, and extensive logging of human feedback. To allow users to create and modify effective queries, our demo222Demonstration Video of QueryExplorer supports complementary approaches of using LLMs interactively, assisting the user with edits and feedback at multiple stages of the query formulation process. With support for recording fine-grained interactions and user annotations, QueryExplorer can serve as a valuable experimental and research platform for annotation, qualitative evaluation, and conducting Human-in-the-Loop (HITL) experiments for complex search tasks where users struggle to formulate queries.
QueryExplorer: An Interactive Query Generation Assistant for Search and Exploration
Kaustubh D. Dhole Department of Computer Science Emory University, Atlanta [email protected] Shivam Bajaj Cox Communications Atlanta [email protected] Ramraj Chandradevan Department of Computer Science Emory University, Atlanta [email protected] Eugene Agichtein Department of Computer Science Emory University, Atlanta [email protected]
1 Introduction
Being able to retrieve documents in multiple languages is becoming critical as the Internet increasingly provides access to information across a wide range of languages and domains. However, creating effective search queries for cross-language and multi-language retrieval can be a daunting task for users. First, users may be unfamiliar with the language of the documents with the information they need, or may even be unaware of this information, making it hard to craft effective queries. Second, most people are not familiar with the vocabulary and jargon used in other areas or fields, which can hinder their ability to formulate good search queries. Consider a scenario where a user is tasked with identifying documents pertinent to legal disputes. They may lack familiarity with the specialized terminology, yet possess examples of specific documents in question.
“Query-by-example” (QBE) is one solution to such a challenge. It allows users to explore document collections by specifying an example document (rather than an explicit query) of what they are searching for. Although considerable advancements have been made in the domain of query-by-example, in recently using neural IR techniques Sarwar and Allan (2020); Zloof (1975); Alaofi et al. (2023); Zhang et al. (2012), there is a lack of effective and easily configurable search interface tools for exploring and annotating query-by-example experiments, especially in the interactive setting.
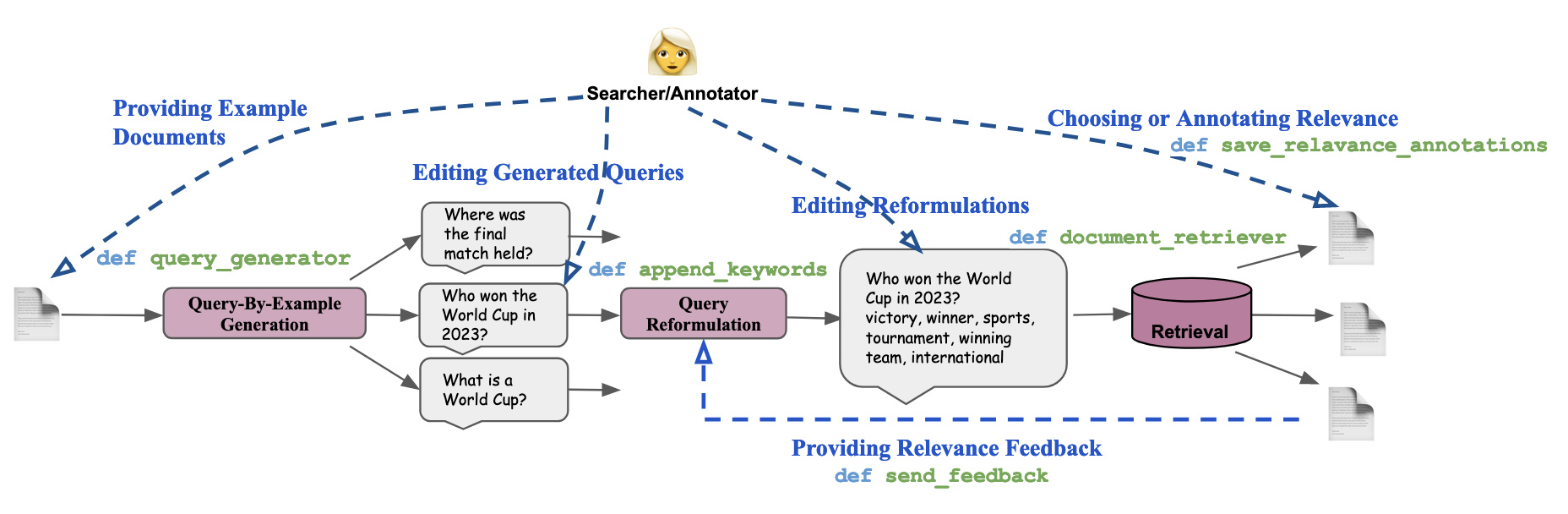
While a good QBE interface could be valuable, a tool that can facilitate generating queries with a Human-In-The-Loop (HITL) setting can result in an even more effective search. Extensive prior work has shown that automatically generated queries can be improved with the searcher’s inputs Jiang et al. (2014), via providing in-domain and world-knowledge Cho et al. (2022); Mackie et al. (2023), as well as from relevance or pseudo-relevance feedback Abdul-Jaleel et al. (2004); Li et al. (2018); Zheng et al. (2020); Wang et al. (2023b) through search results and this process can iterate several times till the searcher is satisfied with the presented search results. Researchers studying searcher behavior and gathering associated annotated data may need iterating over different query generators, multiple prompting and training strategies, several retrieval pipelines, recording various ways to incorporate implicit feedback, and a rigorous number of hyperparameters. For instance, a search engine analyst might be interested in how searchers edit queries.
To investigate these much-needed capabilities, we propose a configurable, interactive search interface tool, QueryExplorer, which supports automatic and interactive query generation and reformulation for mono-lingual and multi-lingual interactive search. QueryExplorer can both facilitate and record the query generation process and interactions of a searcher – from query formulation in a pure QBE setting to tracking the resulting query’s impact on the retrieved search results and the user’s exploration. To our knowledge, QueryExplorer is the first such interactive query exploration interface to be shared with the research community.
Specifically, QueryExplorer demonstrates the following novel capabilities:
-
•
A simple document search functionality with natural support for multi-lingual and cross-lingual search, making it easy for searchers to navigate and analyze search results.
-
•
Support for both automated query generation and reformulation, and human-in-the-loop capabilities such as propagating the human input back to query reformulation, allowing searchers to collaborate with automated query generation models.
- •
-
•
Extensive instrumentation support for query generation and reformulation experiments, including the ability to record query edits, reformulations, all user interactions, and relevance judgments making it useful for collecting and annotating end-to-end datasets.
In summary, we believe QueryExplorer could provide valuable tools for i) performing qualitative analysis over information retrieval experiments and datasets, ii) investigating interactive retrieval feedback and performing Human-In-The-Loop (HITL) studies, and iii) gathering user annotations and understanding searcher behavior. Our system was built initially Dhole et al. (2023b) for the BETTER search tasks333IARPA Better Research Program Mckinnon and Rubino (2022); Soboroff (2023) and was later generalized and expanded to support end-to-end query generation and reformulation experiments. We share the Python code, a Google Colab notebook as well as the video demonstration here444https://github.com/emory-irlab/query-explorer.
2 Related Work
There have been many ranking and retrieval tools with annotation support released previously Lin et al. (2021); Macdonald et al. (2021); Scells and Potthast (2023); Akiki et al. (2023); Ng et al. (2023); Giachelle et al. (2022), but all of them have focused on the ad-hoc search setting by assuming a readily available query without the need for generation, reformulation or feedback. Spacerini Akiki et al. (2023) leveraged the Pyserini Lin et al. (2021) toolkit and the Hugging Face library to facilitate the creation and hosting of search systems for ad-hoc search. SimplyRetrieve Ng et al. (2023), FastRag Izsak et al. (2023) and RaLLe Hoshi et al. (2023) focus on retrieval augmented generation.
Recent advancements in transformer models and their availability via open-source ecosystems like HuggingFace Wolf et al. (2020) and LangChain Chase (2022) have facilitated the seamless integration of multiple models Dhole (2024). However, despite the accessibility of these tools for researchers and annotators, the integration of the query generator pipeline into search engines remains underdeveloped. Furthermore, the expansion of these tools into multilingual search capabilities has been limited.
Besides, success in few-shot prompting Srivastava et al. (2023); Brown et al. (2020a); Liu et al. (2023); Brown et al. (2020b) has led large language models to play a key role in reducing the information burden on users by especially assisting them with writing tasks namely essay writing, summarisation, transcript and dialog generation, etc. This success has also been transferred to tasks related to query generation Jeong et al. (2021); Nogueira et al. (2019). While large language model applications are prevalent and numerous studies have been conducted for search interfaces Liu et al. (2022, 2021a, 2021b); Xu et al. (2009), there has been little impetus to combine search interfaces with large language model-based query generation.
QueryExplorer distinguishes itself by offering a more comprehensive integration of various search frameworks, including query generators, reformulators, and multilingual models. Unlike previous approaches, our tool addresses query generation by assuming the ‘query-by-example’ setting, which operates without an explicit query. The query generator component overcomes this challenge by generating a suggested query and refining it through iterative human interaction and feedback.
We now briefly describe the different components of QueryExplorer.
3 QueryExplorer
The QueryExplorer Interface is made up of 2 tabs – The Query Generation tab and the Settings tab. Both of them are described below. The Query Generation tab is displayed to end users or searchers and annotators and the Settings tab is reserved for researchers555We use the term searchers and researchers to differentiate between the higher level goals of the two tabs but both could encompass analysts/annotators/testers, etc. looking to gather data for query generation and IR studies by allowing them to investigate different settings. The complete interface is built using HuggingFace’s Gradio platform. Gradio Abid et al. (2019) is an open-source Python package to quickly create easy-to-use, configurable UI components and has been popularly used for machine learning models.
3.1 Searcher’s Tab: Query Generation
This Query Generation tab serves as a simple interface for searchers and annotators which permits end-to-end query generation (QG) – i) Ad-hoc QG: users can write search queries by themselves ii) Query-by-Example: users can generate queries through prompting a HuggingFace Wolf et al. (2020) model and select appropriate ones, query reformulation – through a HuggingFace model to generate useful keywords, and document or passage retrieval – through a PyTerrier Macdonald et al. (2021) retrieval pipeline over multiple retrieval datasets supported through IRDatasets MacAvaney et al. (2021). We display the top-k relevant documents and their source language translations if applicable.
Each of the generated queries can be used by itself or in combination to retrieve documents. Users can further edit the queries, as well as receive assistance from the output of a query reformulator. Users can further interact with the retrieved documents or passages, and provide relevance annotations for each of the documents.
We now describe the default models provided for each of the above settings for the demonstration. Each of these can be easily substituted with the researcher’s choices by minor modifications to the configuration settings or code.
Functions | Actions (Searchers and Annotators) | Configurable Settings (Researchers) |
---|---|---|
Provide Example Documents | Choice of Domain, Example Documents | |
query_generator | Edit Generated Queries | 0-shot/Few-shot QG, Prompt, Exemplars, HF model |
append_keywords | Edit Reformulations to Create Better Query | 0-shot/Few-shot QG, Prompt, Exemplars, HF model |
document_retriever | Annotate Relevance of Document to Query | Retrieval PyTerrier Pipeline, Index, Documents, |
send_feedback | Select Documents for Providing Relevance Feedback | 0-shot/Few-shot QG, Prompt, Exemplars, Number of Documents |
3.1.1 Query-By-Example Generation (QBE)
We use the flan-t5-xxl model Chung et al. (2022), which is the instruction tuned Peng et al. (2023) version of the text-to-text transformer T5 Raffel et al. (2020). It has been fine-tuned on a large number of tasks making it convenient Aribandi et al. (2022) for learning new tasks. The default version shown to the user is a few-shot wrapper over flan-t5-xxl – that takes in the user’s example document or passage and prepends an instruction Generate a query given the following document along with 3 document-query pairs from MSMarco as exemplars to it.
3.1.2 Query Reformulation (QR)
We use a zero-shot approach to generate keywords for the given query. flan-t5-xxl is passed the instruction Improve the search effectiveness by suggesting expansion terms for the query Wang et al. (2023a) along with the original query as input. Zero-shot query reformulation Dhole and Agichtein (2024); Yang et al. (2023); Wang et al. (2023a) has been recently popular to expand queries to increase their retrieval effectiveness through zero-shot prompting of large language models. A user-facing interface can provide opportunities to mitigate bad reformulations Weller et al. (2023) (Refer Appendix Listing 1).
3.1.3 Retrieving Documents
For retrieval, we employ PyTerrier retrievers as default. The architecture of PyTerrier is inherently designed to support operations over retrievers and rerankers to build end-to-end retrieval pipelines and has been a popular choice of retrieval engine among information retrieval researchers. In QueryExplorer, researchers can add their own custom PyTerrier pipelines too in the below dictionary (Refer Appendix Listing 2). This would also be reflected in the dropdown in the Settings tab.
3.1.4 Incorporating Relevance Feedback (RF)
We provide searchers the ability to improve the current query by utilizing a retrieved document of their choice. We use a zero-shot approach to incorporate the user-selected documents in the style of Wang et al. (2023a); Dhole and Agichtein (2024). The user-selected documents and the query are prompted to regenerate keywords through an instruction Based on the given context information , generate keywords for the following query where is a user-selected document (Refer Appendix Listing 3).
3.2 PyTerrier and HuggingFace support
In an effort to expedite the process of prototyping diverse experiments for IR researchers, QueryExplorer incorporates PyTerrier Macdonald et al. (2021) support. This integration enables the utilization of retrieval pipelines created through PyTerrier within the QueryExplorer interface, enhancing its functionality for both annotation and qualitative analysis across different retrieval and reranking algorithms. During the demonstration, we showcase the interface’s capability to display search results using the BM25 pipeline, highlighting the flexibility to substitute this with other custom pipelines as needed. This feature essentially adds a layer of qualitative analysis to the PyTerrier retrieval pipelines. Furthermore, to broaden the utility of PyTerrier in handling IR datasets, QueryExplorer has been designed to facilitate the indexing of documents from these datasets, thereby enabling qualitative experiments across multiple benchmarks and datasets.
Recognizing the widespread adoption of HuggingFace’s Wolf et al. (2020) models within the research community, QueryExplorer leverages these models for query generation, reformulation, and incorporating feedback. This allows for the comprehensive evaluation of search functionalities across a diverse range of large language models.
While we designed with PyTerrier and HuggingFace ecosystems in mind due to their popularity, datasets and models using other packages can also exploit the QueryExplorer interface over their systems.
3.3 Relevance Annotations
We allow each document to be annotated for relevance to help researchers gather relevance annotations through a slider component. Annotations are immediately saved in a separate JSON file.
3.4 Researcher’s Tab: Settings
The Settings tab is designed for researchers (or specialists) who intend to gather data or study the performance of the interaction by allowing them to vary the various components in the query generation pipeline – like the choice of retriever, the dataset to retrieve from, or the instruction and few-shot examples for the query generator and reformulator. The various dimensions along which the researcher can vary the settings from the interface and the corresponding searcher’s actions are described in Table 1.
3.4.1 Interaction logging
The researcher can look at the continuously recorded annotations consisting of – generated queries, post-reformulation queries, query-document relevance annotations, and feedback information – all with metadata of session and timestamps. These can be viewed directly in the tab as well as be utilized for subsequent analysis.
QueryExplorer by default stores the recordings in three JSON formatted files:
-
•
Query Logs: where different versions of the queries along with the source of change (whether through a model or the user or through a reformulator, etc.) and additional metadata like timestamps and user session information are stored.
-
•
Predicted Search Results: where the user’s search queries and corresponding retrieved documents are stores
-
•
Document-Query Relevance Annotations: where an annotator’s document-query annotated pairs are stored
Documenting detailed annotations such as changes in queries and the evolution of queries over time can provide several benefits for researchers. This includes the ability to detect users who may not be attentive or who might be using automated bots. Additionally, observing the patterns and behaviors of users during their search activities can offer valuable insights. Furthermore, assessing the effort involved in formulating queries and perusing documents, as indicated by the time spent on these tasks, can also be advantageous for research purposes.
4 Conclusion
Interactive query generation and reformulation is of significant interest for many search and exploration tasks like (document-query) pairs augmentation Alaofi et al. (2023); Dhole et al. (2023a), document expansion Nogueira et al. (2019); Gospodinov et al. (2023) and keyword expansion Dhole and Agichtein (2024); Carpineto and Romano (2012); Jagerman et al. (2023); Wang et al. (2023a). QueryExplorer acts as a resource to permit qualitative evaluation of query generation and retrieval in conjunction. Such a combined interface is crucial as it permits immediate retrieval feedback from the user to be incorporated into the search process.
This paper demonstrates the novel capabilities of QueryExplorer to assist researchers with investigating the construction, feedback, and evaluation of the interactive query generation process. Furthermore, QueryExplorer provides extensive fine-grained instrumentation to record the end-to-end generation process from query formulation to retrieval feedback to enable the construction of search interaction and feedback datasets. Researchers can also quickly perform qualitative analysis by loading up QueryExplorer’s lightweight search interface through Colab and gather data quickly. QueryExplorer’s interface also provides an avenue to perform Human-In-The-Loop (HITL) studies. Apart from qualitative studies, we believe QueryExplorer could be effective in performing more sophisticated information retrieval experiments as well as serve as a tool to incorporate retrieval feedback and conduct Human-In-The-Loop studies.
5 Ethical Statement
QueryExplorer serves as a comprehensive tool, capturing the entire pipeline for purposes of analysis, annotation, and logging. The components within QueryExplorer, including query generators, reformulators, and PRF, can be replaced with even larger LM alternatives. However, these substitutes might lead to the generation of biased or toxic keywords and reformulations. Therefore, it is crucial to consider QueryExplorer within the broader sociotechnical framework Dhole (2023) implement appropriate filters, and conduct thorough testing before any deployment.
6 Acknowledgements
This work was supported in part by IARPA BETTER (#2019-19051600005). The views and conclusions contained in this work are those of the authors and should not be interpreted as necessarily representing the official policies, either expressed or implied, or endorsements of ODNI, IARPA, or the U.S. Government. The U.S. Government is authorized to reproduce and distribute reprints for governmental purposes notwithstanding any copyright annotation therein.
References
- Abdul-Jaleel et al. (2004) Nasreen Abdul-Jaleel, James Allan, W Bruce Croft, Fernando Diaz, Leah Larkey, Xiaoyan Li, Mark D Smucker, and Courtney Wade. 2004. Umass at trec 2004: Novelty and hard. Computer Science Department Faculty Publication Series, page 189.
- Abid et al. (2019) Abubakar Abid, Ali Abdalla, Ali Abid, Dawood Khan, Abdulrahman Alfozan, and James Y. Zou. 2019. Gradio: Hassle-free sharing and testing of ml models in the wild. ArXiv, abs/1906.02569.
- Akiki et al. (2023) Christopher Akiki, Odunayo Ogundepo, Aleksandra Piktus, Xinyu Zhang, Akintunde Oladipo, Jimmy Lin, and Martin Potthast. 2023. Spacerini: Plug-and-play search engines with pyserini and hugging face. arXiv preprint arXiv:2302.14534.
- Alaofi et al. (2023) Marwah Alaofi, Luke Gallagher, Mark Sanderson, Falk Scholer, and Paul Thomas. 2023. Can generative llms create query variants for test collections? an exploratory study. In Proceedings of the 46th International ACM SIGIR Conference on Research and Development in Information Retrieval, SIGIR ’23, page 1869–1873, New York, NY, USA. Association for Computing Machinery.
- Aribandi et al. (2022) Vamsi Aribandi, Yi Tay, Tal Schuster, Jinfeng Rao, Huaixiu Steven Zheng, Sanket Vaibhav Mehta, Honglei Zhuang, Vinh Q. Tran, Dara Bahri, Jianmo Ni, Jai Gupta, Kai Hui, Sebastian Ruder, and Donald Metzler. 2022. Ext5: Towards extreme multi-task scaling for transfer learning. In International Conference on Learning Representations.
- Brown et al. (2020a) Tom Brown, Benjamin Mann, Nick Ryder, Melanie Subbiah, Jared D Kaplan, Prafulla Dhariwal, Arvind Neelakantan, Pranav Shyam, Girish Sastry, Amanda Askell, et al. 2020a. Language models are few-shot learners. Advances in neural information processing systems, 33:1877–1901.
- Brown et al. (2020b) Tom Brown, Benjamin Mann, Nick Ryder, Melanie Subbiah, Jared D Kaplan, Prafulla Dhariwal, Arvind Neelakantan, Pranav Shyam, Girish Sastry, Amanda Askell, et al. 2020b. Language models are few-shot learners. volume 33, pages 1877–1901.
- Carpineto and Romano (2012) Claudio Carpineto and Giovanni Romano. 2012. A survey of automatic query expansion in information retrieval. Acm Computing Surveys (CSUR), 44(1):1–50.
- Chase (2022) Harrison Chase. 2022. Langchain.
- Cho et al. (2022) Sukmin Cho, Soyeong Jeong, Wonsuk Yang, and Jong Park. 2022. Query generation with external knowledge for dense retrieval. In Proceedings of Deep Learning Inside Out (DeeLIO 2022): The 3rd Workshop on Knowledge Extraction and Integration for Deep Learning Architectures, pages 22–32, Dublin, Ireland and Online. Association for Computational Linguistics.
- Chung et al. (2022) Hyung Won Chung, Le Hou, Shayne Longpre, Barret Zoph, Yi Tay, William Fedus, Eric Li, Xuezhi Wang, Mostafa Dehghani, Siddhartha Brahma, et al. 2022. Scaling instruction-finetuned language models. arXiv preprint arXiv:2210.11416.
- Dhole (2023) Kaustubh Dhole. 2023. Large language models as SocioTechnical systems. In Proceedings of the Big Picture Workshop, pages 66–79, Singapore. Association for Computational Linguistics.
- Dhole (2024) Kaustubh Dhole. 2024. Kaucus-knowledgeable user simulators for training large language models. In Proceedings of the 1st Workshop on Simulating Conversational Intelligence in Chat (SCI-CHAT 2024), pages 53–65.
- Dhole et al. (2023a) Kaustubh Dhole, Varun Gangal, Sebastian Gehrmann, Aadesh Gupta, Zhenhao Li, Saad Mahamood, Abinaya Mahadiran, Simon Mille, Ashish Shrivastava, Samson Tan, et al. 2023a. Nl-augmenter: A framework for task-sensitive natural language augmentation. Northern European Journal of Language Technology, 9(1).
- Dhole and Agichtein (2024) Kaustubh D. Dhole and Eugene Agichtein. 2024. Genqrensemble: Zero-shot llm ensemble prompting for generative query reformulation. In Advances in Information Retrieval, pages 326–335, Cham. Springer Nature Switzerland.
- Dhole et al. (2023b) Kaustubh D. Dhole, Ramraj Chandradevan, and Eugene Agichtein. 2023b. An interactive query generation assistant using llm-based prompt modification and user feedback.
- Giachelle et al. (2022) Fabio Giachelle, Ornella Irrera, and Gianmaria Silvello. 2022. Doctag: A customizable annotation tool for ground truth creation. In European Conference on Information Retrieval, pages 288–293. Springer.
- Gospodinov et al. (2023) Mitko Gospodinov, Sean MacAvaney, and Craig Macdonald. 2023. Doc2query–: When less is more. In European Conference on Information Retrieval, pages 414–422.
- Hoshi et al. (2023) Yasuto Hoshi, Daisuke Miyashita, Youyang Ng, Kento Tatsuno, Yasuhiro Morioka, Osamu Torii, and Jun Deguchi. 2023. Ralle: A framework for developing and evaluating retrieval-augmented large language models.
- Izsak et al. (2023) Peter Izsak, Moshe Berchansky, Daniel Fleischer, and Ronen Laperdon. 2023. fastrag: Efficient retrieval augmentation and generation framework.
- Jagerman et al. (2023) Rolf Jagerman, Honglei Zhuang, Zhen Qin, Xuanhui Wang, and Michael Bendersky. 2023. Query expansion by prompting large language models. arXiv preprint arXiv:2305.03653.
- Jeong et al. (2021) Soyeong Jeong, Jinheon Baek, ChaeHun Park, and Jong Park. 2021. Unsupervised document expansion for information retrieval with stochastic text generation. In Proceedings of the Second Workshop on Scholarly Document Processing, pages 7–17, Online. Association for Computational Linguistics.
- Jiang et al. (2014) Jyun-Yu Jiang, Yen-Yu Ke, Pao-Yu Chien, and Pu-Jen Cheng. 2014. Learning user reformulation behavior for query auto-completion. In Proceedings of the 37th international ACM SIGIR conference on Research & development in information retrieval, pages 445–454.
- Li et al. (2018) Canjia Li, Yingfei Sun, Ben He, Le Wang, Kai Hui, Andrew Yates, Le Sun, and Jungang Xu. 2018. NPRF: A neural pseudo relevance feedback framework for ad-hoc information retrieval. In Proceedings of the 2018 Conference on Empirical Methods in Natural Language Processing, pages 4482–4491, Brussels, Belgium. Association for Computational Linguistics.
- Lin et al. (2021) Jimmy Lin, Xueguang Ma, Sheng-Chieh Lin, Jheng-Hong Yang, Ronak Pradeep, and Rodrigo Nogueira. 2021. Pyserini: A python toolkit for reproducible information retrieval research with sparse and dense representations. In Proceedings of the 44th International ACM SIGIR Conference on Research and Development in Information Retrieval, SIGIR ’21, page 2356–2362, New York, NY, USA. Association for Computing Machinery.
- Liu et al. (2021a) Chang Liu, Ying-Hsang Liu, Jingjing Liu, and Ralf Bierig. 2021a. Search interface design and evaluation. Found. Trends Inf. Retr., 15(3–4):243–416.
- Liu et al. (2021b) Chang Liu, Ying-Hsang Liu, Jingjing Liu, and Ralf Bierig. 2021b. Search interface design and evaluation. 15(3–4):243–416.
- Liu et al. (2023) Pengfei Liu, Weizhe Yuan, Jinlan Fu, Zhengbao Jiang, Hiroaki Hayashi, and Graham Neubig. 2023. Pre-train, prompt, and predict: A systematic survey of prompting methods in natural language processing. ACM Comput. Surv., 55(9).
- Liu et al. (2022) Ying-Hsang Liu, Paul Thomas, Tom Gedeon, and Nicolay Rusnachenko. 2022. Search interfaces for biomedical searching: How do gaze, user perception, search behaviour and search performance relate? In Proceedings of the 2022 Conference on Human Information Interaction and Retrieval, CHIIR ’22, page 78–89, New York, NY, USA. Association for Computing Machinery.
- MacAvaney et al. (2021) Sean MacAvaney, Andrew Yates, Sergey Feldman, Doug Downey, Arman Cohan, and Nazli Goharian. 2021. Simplified data wrangling with ir_datasets. In Proceedings of the 44th International ACM SIGIR Conference on Research and Development in Information Retrieval, pages 2429–2436.
- Macdonald et al. (2021) Craig Macdonald, Nicola Tonellotto, Sean MacAvaney, and Iadh Ounis. 2021. Pyterrier: Declarative experimentation in python from bm25 to dense retrieval. In Proceedings of the 30th acm international conference on information & knowledge management, pages 4526–4533.
- Mackie et al. (2023) Iain Mackie, Shubham Chatterjee, and Jeffrey Dalton. 2023. Generative relevance feedback with large language models. arXiv preprint arXiv:2304.13157.
- Mckinnon and Rubino (2022) Timothy Mckinnon and Carl Rubino. 2022. The IARPA BETTER program abstract task four new semantically annotated corpora from IARPA’s BETTER program. In Proceedings of the Thirteenth Language Resources and Evaluation Conference, pages 3595–3600, Marseille, France. European Language Resources Association.
- Ng et al. (2023) Youyang Ng, Daisuke Miyashita, Yasuto Hoshi, Yasuhiro Morioka, Osamu Torii, Tomoya Kodama, and Jun Deguchi. 2023. Simplyretrieve: A private and lightweight retrieval-centric generative ai tool. arXiv preprint arXiv:2308.03983.
- Nogueira et al. (2019) Rodrigo Nogueira, Wei Yang, Jimmy Lin, and Kyunghyun Cho. 2019. Document expansion by query prediction. arXiv preprint arXiv:1904.08375.
- Peng et al. (2023) Baolin Peng, Chunyuan Li, Pengcheng He, Michel Galley, and Jianfeng Gao. 2023. Instruction tuning with gpt-4. arXiv preprint arXiv:2304.03277.
- Raffel et al. (2020) Colin Raffel, Noam Shazeer, Adam Roberts, Katherine Lee, Sharan Narang, Michael Matena, Yanqi Zhou, Wei Li, and Peter J Liu. 2020. Exploring the limits of transfer learning with a unified text-to-text transformer. The Journal of Machine Learning Research, 21(1):5485–5551.
- Sarwar and Allan (2020) Sheikh Muhammad Sarwar and James Allan. 2020. Query by example for cross-lingual event retrieval. SIGIR ’20, page 1601–1604, New York, NY, USA. Association for Computing Machinery.
- Scells and Potthast (2023) Harrisen Scells and Martin Potthast. 2023. Pybool_ir: A toolkit for domain-specific search experiments. In Proceedings of the 46th International ACM SIGIR Conference on Research and Development in Information Retrieval, SIGIR ’23, page 3190–3194, New York, NY, USA. Association for Computing Machinery.
- Soboroff (2023) Ian Soboroff. 2023. The better cross-language datasets. In Proceedings of the 46th International ACM SIGIR Conference on Research and Development in Information Retrieval, SIGIR ’23, page 3047–3053, New York, NY, USA. Association for Computing Machinery.
- Srivastava et al. (2023) Aarohi Srivastava, Abhinav Rastogi, Abhishek Rao, Abu Awal Md Shoeb, Abubakar Abid, Adam Fisch, Adam R Brown, Adam Santoro, Aditya Gupta, Adrià Garriga-Alonso, et al. 2023. Beyond the imitation game: Quantifying and extrapolating the capabilities of language models. Transactions on Machine Learning Research.
- Wang et al. (2023a) Xiao Wang, Sean MacAvaney, Craig Macdonald, and Iadh Ounis. 2023a. Generative query reformulation for effective adhoc search. In The First Workshop on Generative Information Retrieval, SIGIR 2023.
- Wang et al. (2023b) Xiao Wang, Craig Macdonald, Nicola Tonellotto, and Iadh Ounis. 2023b. Colbert-prf: Semantic pseudo-relevance feedback for dense passage and document retrieval. ACM Transactions on the Web, 17(1):1–39.
- Weller et al. (2023) Orion Weller, Kyle Lo, David Wadden, Dawn Lawrie, Benjamin Van Durme, Arman Cohan, and Luca Soldaini. 2023. When do generative query and document expansions fail? a comprehensive study across methods, retrievers, and datasets. arXiv preprint arXiv:2309.08541.
- Wolf et al. (2020) Thomas Wolf, Lysandre Debut, Victor Sanh, Julien Chaumond, Clement Delangue, Anthony Moi, Pierric Cistac, Tim Rault, Rémi Louf, Morgan Funtowicz, et al. 2020. Transformers: State-of-the-art natural language processing. In Proceedings of the 2020 conference on empirical methods in natural language processing: system demonstrations, pages 38–45.
- Xu et al. (2009) Songhua Xu, Tao Jin, and Francis C. M. Lau. 2009. A new visual search interface for web browsing. WSDM ’09, page 152–161, New York, NY, USA. Association for Computing Machinery.
- Yang et al. (2023) Dayu Yang, Yue Zhang, and Hui Fang. 2023. Zero-shot query reformulation for conversational search. In Proceedings of the 2023 ACM SIGIR International Conference on Theory of Information Retrieval, ICTIR ’23, page 257–263, New York, NY, USA. Association for Computing Machinery.
- Zhang et al. (2012) Guanyuan Zhang, Kai Lu, and Bin Wang. 2012. Query reformulation based on user habits for query-by-humming systems. In Information Retrieval Technology: 8th Asia Information Retrieval Societies Conference, AIRS 2012, Tianjin, China, December 17-19, 2012. Proceedings 8, pages 386–395. Springer.
- Zheng et al. (2020) Zhi Zheng, Kai Hui, Ben He, Xianpei Han, Le Sun, and Andrew Yates. 2020. BERT-QE: Contextualized Query Expansion for Document Re-ranking. In Findings of the Association for Computational Linguistics: EMNLP 2020, pages 4718–4728, Online. Association for Computational Linguistics.
- Zloof (1975) Moshé M. Zloof. 1975. Query by example. AFIPS ’75, page 431–438, New York, NY, USA. Association for Computing Machinery.