PrimeComposer: Faster Progressively Combined Diffusion for Image Composition with Attention Steering
Abstract.
Image composition involves seamlessly integrating given objects into a specific visual context. Current training-free methods rely on composing attention weights from several samplers to guide the generator. However, since these weights are derived from disparate contexts, their combination leads to coherence confusion and loss of appearance information. These issues worsen with their excessive focus on background generation, even when unnecessary in this task. This not only impedes their swift implementation but also compromises foreground generation quality. Moreover, these methods introduce unwanted artifacts in the transition area. In this paper, we formulate image composition as a subject-based local editing task, solely focusing on foreground generation. At each step, the edited foreground is combined with the noisy background to maintain scene consistency. To address the remaining issues, we propose PrimeComposer, a faster training-free diffuser that composites the images by well-designed attention steering across different noise levels. This steering is predominantly achieved by our Correlation Diffuser, utilizing its self-attention layers at each step. Within these layers, the synthesized subject interacts with both the referenced object and background, capturing intricate details and coherent relationships. This prior information is encoded into the attention weights, which are then integrated into the self-attention layers of the generator to guide the synthesis process. Besides, we introduce a Region-constrained Cross-Attention to confine the impact of specific subject-related tokens to desired regions, addressing the unwanted artifacts shown in the prior method thereby further improving the coherence in the transition area. Our method exhibits the fastest inference efficiency and extensive experiments demonstrate our superiority both qualitatively and quantitatively. The code is available at https://github.com/CodeGoat24/PrimeComposer.
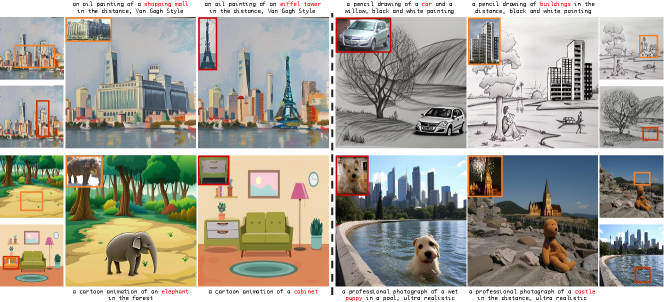
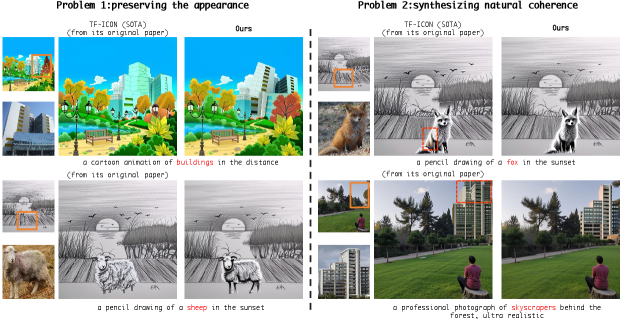
1. Introduction
Image composition entails seamlessly incorporating the given object into the specific visual context without altering the object’s appearance while ensuring natural transitions. Earlier studies employ personalized concept learning (Gal et al., 2022, 2023; Kawar et al., 2023; Kumari et al., 2023; Ruiz et al., 2023), yet they often rely on costly instance-based optimization and encounter limitations in generating concepts with specified backgrounds. Although these challenges are effectively addressed by utilizing diffusion models to explicitly incorporate additional guiding images (Song et al., 2022; Yang et al., 2023), retraining these pre-trained models on customized datasets risks compromising their rich prior knowledge. Consequently, these methods exhibit limited compositional abilities beyond their training domain, and they still demand substantial computational resources.
A recent study (Lu et al., 2023) develops a training-free framework, TF-ICON, that leverages attention weights from several samplers to composite the self-attention map of the diffuser during the composition process. Despite achieving notable success in this task, it still faces substantial challenges in preserving the appearance of complex objects (Fig. 2 (left)) and synthesizing a natural coherence (Fig. 2 (right)). The primary issue resides in its composite self-attention maps: the incorporation of attention weights from different contexts introduces potential ambiguity. To be precise, in TF-ICON, each sampler’s weights are calculated within its specific global context (Vaswani et al., 2017), and the act of forcibly combining them leads to the synthesized confusion in coherent relations and loss of appearance information. This undoubtedly hampers its ability to accurately represent the intrinsic characteristics of the given object and establish a coherent relationship. These issues are further accentuated by its overemphasis on background generation (a tailored sampler). It is essential to underscore that the background inherently necessitates no alteration, given the user’s exclusive focus on foreground area generation. This superfluous emphasis not only introduces computational overhead but also leads to compromise on the foreground synthesis. Moreover, this approach introduces the unwanted artifacts in the transition area as shown in Fig. 4, further hindering the natural coherence establishment.
In summary, while the current training-free method has mitigated the need for costly optimization and retraining, it remains incapable of capturing the nuanced appearance of objects and forging dependable coherent relations. Urgent exploration of more effective steering mechanisms for training-free composition, without compromising efficiency, is imperative.
In this paper, we novelly formulate this task as a subject-guided local editing problem, focusing solely on foreground generation due to the unnecessary alteration of the background. To achieve this, as depicted in Fig. 3, we utilize the pre-trained Latent Diffusion Model (LDM) (Rombach et al., 2022) to edit local foreground areas evolvingly based on the given object and text. Then the resultant edited area is spatially combined with a certain noised background at each step to maintain the scene. To address the remaining issues, we propose a faster training-free method, dubbed PrimeComposer, a progressively combined diffusion model that integrates the user-provided object to the background through well-designed attention steering across different noise levels. This progressive steering is primarily facilitated by our Correlation Diffuser (CD). Specifically, in each step, we combine specific noisy-level versions of the provided object and background at the pixel level while simultaneously segmenting the synthesized subject from the previous step’s results. These components are then fed into the CD’s diffusion pipeline where the synthesized subject interacts with both the referenced object and background within self-attention layers. The interrelation information, encoded as prior weights, encapsulates rich mutual correlations and object appearance features. Consequently, we infuse them into LDM’s self-attention maps (yellow and orange regions in Fig. 3 (bottom right), respectively) to meticulously steer the preservation of object appearance and ensure harmonious coherence establishment. To fortify the steering impact, we further advance the classifier-free guidance (Ho and Salimans, 2022), elaborated in Sec. 4.5. Additionally, we introduce Region-constrained Cross-Attention (RCA), replacing cross-attention layers in LDM, to confine the impact of specific subject-related tokens to predefined regions in attention maps. This helps mitigate unwanted artifacts, thereby enhancing coherence in the transition area.
Note that CD is the only sampler for steering and all the infused attention weights are computed from the accordant context. However, intuitively infusing object appearance-related weights on all layers will result in the subject overfitting, as shown in Fig. 5, thereby leading to unexpected coherence problems: style inconsistency. Therefore, we propose to control appearance infusion only in the decoder part of the U-Net since the decoder has been proven to focus on learning the appearance and textures (Zhang et al., 2023b).
Our contributions can be summarized as follows:
(1) We formulate image composition as a subject-guided local editing problem and propose a faster training-free method to seamlessly integrate given objects into the specific visual scene across various domains. (2) We develop the CD to simultaneously alleviate the challenge of preserving complex objects’ appearance and synthesizing natural coherence by well-designed attention steering. (3) We introduce RCA to confine the impact of specific subject-related tokens in attention maps, thereby effectively addressing the unwanted artifacts. (4) Our method exhibits the fastest inference efficiency and extensive experiments demonstrate our superiority both qualitatively and quantitatively.
2. Related Work
Image composition serves as a valuable tool for diverse downstream tasks, e.g., entertainment, and data augmentation (Dwibedi et al., 2017; Liu et al., 2021; Lu et al., 2023; Cai et al., 2024; Wang et al., 2024b). The practice for this task broadly falls into two categories: text-guided and image-guided composition.
Text-guided composition (Avrahami et al., 2023, 2022; Chefer et al., 2023; Feng et al., 2022; Liu et al., 2022) involves generating images based on a text prompt that specifies multiple objects. This approach allows for diverse appearances as long as the semantics align with the prompt. Despite its effectiveness, semantic errors may arise, especially with prompts involving multiple objects. These errors, including attribute leakage and missing objects, often necessitate extensive prompt engineering (Witteveen and Andrews, 2022).
Conversely, image-guided composition (Brown et al., 2022; Gafni and Wolf, 2020; Li et al., 2023; Song et al., 2022; Xue et al., 2022; Yang et al., 2023; Zhang et al., 2021; Lu et al., 2023) incorporates specific objects and scenarios from user-provided photos, potentially with the assistance of a text prompt. This approach presents greater challenges, particularly when dealing with images from different visual domains. Specifically, image-guided composition encompasses various sub-tasks (Niu et al., 2021), such as object placement (Azadi et al., 2020; Chen and Kae, 2019; Lin et al., 2018; Tripathi et al., 2019; Zhang et al., 2020b; Wang et al., 2023, 2024a), image blending (Wu et al., 2019; Zhang et al., 2020a), image harmonization (Cong et al., 2020; Cun and Pun, 2020; Jiang et al., 2021; Xue et al., 2022; Niu et al., 2024a, b), and shadow generation (Hong et al., 2022; Liu et al., 2020; Sheng et al., 2021; Zhang et al., 2019). These diverse tasks are typically tackled by distinct models and pipelines, showcasing the intricacy of image-guided composition. Recently, diffusion models have demonstrated impressive capabilities in image-guided composition by simultaneously tackling all these subtasks. While prior studies have explored personalized concept learning (Gal et al., 2022, 2023; Kawar et al., 2023; Kumari et al., 2023; Ruiz et al., 2023), they often rely on costly instance-based optimization and face limitations in generating concepts with specified backgrounds. To overcome these challenges, subsequent studies (Song et al., 2022; Yang et al., 2023) effectively incorporate additional guiding images into diffusion models through retraining pre-trained models on tailored datasets. However, this poses a risk of compromising their rich prior knowledge. Besides, these models exhibit limited compositional abilities beyond their training domain and demand substantial computational resources. A recent study (Lu et al., 2023) introduces a training-free method involving the gradual injection of composite self-attention maps through multiple samplers. Despite its remarkable success, it encounters challenges in preserving the appearance of complex objects and synthesizing natural coherence.
Diverging from the approaches mentioned earlier, we novelly formulate this task as an subject-guided local editing problem. Our progressively combined Diffusion, PrimeComposer, intricately depicts the object and achieves harmonious coherence through well-designed attention steering across a progression of noise levels.
3. Preliminary
Denoising diffusion probabilistic models (DDPMs) (Ho et al., 2020) are designed to reverse a parameterized Markovian image noising process. They start with isotropic Gaussian noise samples and gradually transform them into samples from a training distribution by iteratively removing noise. Given a data distribution , the forward noising process produces a sequence of latent by adding Gaussian noise with variance at each time step t:
(1) | ||||
When is sufficiently large, the last latent approximates an isotropic Gaussian distribution.
An important property of the forward noising process is that any step can be directly sampled from , without generating the intermediate steps:
(2) | ||||
where , , and .
To draw a new sample from the distribution , the Markovian process is reversed. Starting from a Gaussian noise sample with , a reverse sequence is generated by sampling the posteriors .
However, is unknown and depends on the unknown data distribution . To approximate this function, a deep neural network is trained to predict the mean and covariance of given as input:
(3) |
Rather than inferring directly, Ho et al. (Ho et al., 2020) propose to predict the noise that was added to to obtain according to Equation 2. Then, is derived using Bayes’ theorem:
(4) |
For more detail see (Ho et al., 2020). In this work, we leverage the pre-trained text-to-image Latent Diffusion Model (LDM) (Rombach et al., 2022), a.k.a. Stable Diffusion, which applies the noising process in the latent space.
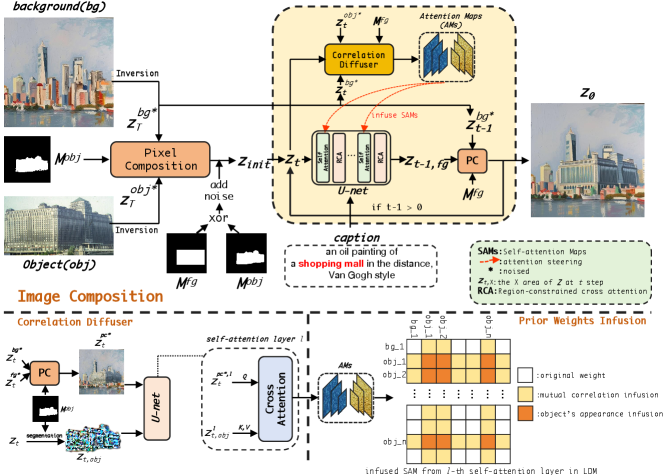
4. Method
This section begins with an overview of our method, followed by an in-depth explanation of self-attention steering based on our Correlation Diffuser (CD). Subsequently, we will explore the details of our Region-constrained Cross-Attention (RCA). Finally, we will introduce our careful extension of classifier-free guidance (CFG) during inference.
4.1. Overview
This work formulates image composition as a local object-guided editing task, utilizing LDM to depict the object and synthesize natural coherence. We aim to seamlessly synthesize the given object within specific foreground areas effectively without compromising efficiency. To achieve this, we propose PrimeComposer, a faster training-free progressively combined composer that composites the images by well-designed attention steering across different noise levels. Specifically, we leverage the prior attention weights from CD to steer the preservation of object appearance and the establishment of natural coherent relations. Its effectiveness is further enhanced through our extension of CFG. Besides, we introduce RCA to replace the cross-attention layers in LDM. RCA effectively restricts the influence of object-specific tokens to desired spatial regions, thereby mitigating unexpected artifacts around synthesized objects.
In the depicted pipeline (Fig. 3), the process begins with a background image, an object image, a caption prompt , and two binary masks and (designating the object and foreground areas, respectively). The background and object images are first inverted into latent representations and using DPM-Solver++ (Lu et al., 2022) following (Lu et al., 2023). These representations are then composited at the pixel level based on . To harness the prior knowledge of LDM for synthesizing the coherence, Gaussian noise is introduced to the transition areas (where XOR ), resulting in the initial input noise . In each step t, we discern the attention weights embodying object appearance features and coherent correlations from CD’s self-attention layers. These prior weights are then infused into LDM’s self-attention maps to guide foreground generation. Additionally, all cross-attention maps in LDM are rectified to limit the impact of object-specific tokens in predefined regions. To preserve the unchanged scene, the edited foreground areas at each step are combined with the certain noisy version of the background based on . This iterative process ensures seamless composition.
4.2. Self-Attention Steering
While the composite noise acts as the initial input, and the caption prompt contributes to inpainting the transition areas, LDM still encounters challenges in preserving the appearance of the object and synthesizing harmonious results effectively as shown in Fig. 7. To tackle this, we propose the CD to chase down the attention weights that encapsulate rich prior semantic information of the object’s features and coherent relations. Subsequently, these prior attention weights are employed to guide the synthesis process in the initial steps where is the hyperparameter.
4.2.1. Correlation diffuser
The CD is adapted from the pre-trained Stable Diffusion with tailored self-attention layers to generate prior attention maps. Specifically, at each timestep t, it takes the pixel composite image (derived from the specific noise version of the user-provided object and background) and the latent representation of the synthesized object (segmented from the previous step’s result ) as input. In each self-attention layer l, the self-attention map are computed as follows:
(5) | |||
(6) |
where , h, w denote the height and width of background, n denote the flatten pixel amount of the object and are projection matrices.
4.2.2. Prior weights infusion
The obtained prior attention map comprises two constituents: and . reflects the relations between the synthesized object and background, while contains the object appearance features. These constituents are then infused into the l-th self-attention maps in LDM, as illustrated by the yellow and orange regions in Fig. 3 (bottom right), respectively. The process can be formulated as: where is the function to produce the infused self-attentionmaps .
However, the intuitive infusion of on all layers may result in the synthesized object closely resembling the given image, i.e., subject overfitting. This, in turn, can lead to unexpected coherence problems, as depicted in Fig. 5. To address this issue, we propose a controlled approach to appearance infusion: restricting it only to the decoder part of the U-Net. This decision is grounded in the understanding that the decoder primarily focuses on learning appearance and texture (Zhang et al., 2023b), thus promoting more natural coherence in the synthesized output.
4.3. Region-constrained Cross Attention
Following (Lu et al., 2023), the caption prompt is utilized to guide the synthesis of transition areas. However, this introduces a coherence problem, as demonstrated in Fig. 4 and Fig. 7: the newly generated object isn’t consistently guaranteed to appear appropriately within the regions outlined by , thereby causing the unwanted artifacts. To address this challenge, we introduce RCA, which replaces the cross-attention layers in the U-Net to restrict the impact of object-specific tokens to regions defined by .
Specifically, the latent noisy image is projected to a query matrix q, while the text prompt’s embedding is projected to the key k and value v matrices via learned linear projections. The cross-attention maps A is computed as , where p denote the amount of tokens.
Then, certain attention maps corresponding to object-related tokens (e.g., ’white fox’ and ’lemon’ in Fig. 4) in A are rectified by applying the binary mask :
(7) |
where and represents the weight of the object-related token’s map and the value of , respectively, at the position (i, j). After that, we obtain the rectified attention maps and the output of RCA layer is defined as Softmax.
Through the use of rectified attention maps, this module effectively restricts the impact of object-related tokens to specific spatial regions on the image features. Consequently, the model can enforce the generation of objects in desired positions and shapes, addressing the coherence problem highlighted earlier.
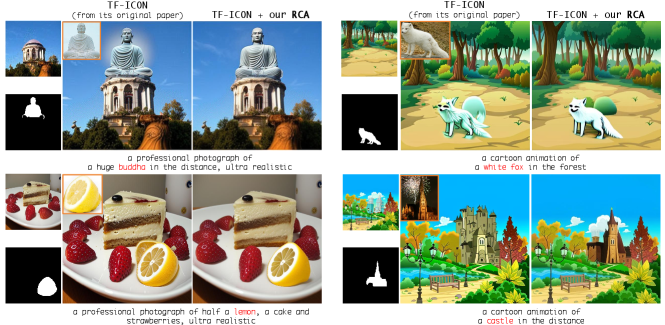
4.4. Background Preserving Combining
Inspired by the concept of blending two images by separately combining each level of their Laplacian pyramids (Burt and Adelson, 1987), our approach involves combining synthesized foreground areas and the given background across different noise levels to maintain the unchanged scene. The underlying principle is that at each step in the diffusion process, a noisy latent is projected onto a manifold of naturally noised images at a specific level. While blending two noisy images (from the same level) may result in output likely outside the manifold, the subsequent diffusion step projects the result onto the next-level manifold, thereby improving coherence (Avrahami et al., 2022).
Thus, at each step t, starting from a latent , we perform a single diffusion step. The resultant latent is segmented based on , yielding a latent denoted . In addition, we obtain a noised version of the input background using Equ. 2. The two latents are combined using the foreground mask:
(8) |
4.5. Extended Classifier-free Guidance
To reinforce the steering effect of the infused prior weights in foreground generation, CFG is extended in each sampling step to extrapolate the predicted noise along the direction specified by certain infusions:
Where , c, and f signify a null, caption prompt, and infusion condition, respectively. and represent the employed LDM and its output noise. The hyperparameter denotes the guidance scale, and the reinforcement effect becomes stronger as increases.
As shown in Fig. 7, this careful design effectively strengthens the ability to generate more harmonious images, since this leads LDM to become more adept at capturing and preserving the subtle details of the object’s appearance and coherence relations. The visualization of the saliency maps derived from our extended CFG is provided in the supplementary.
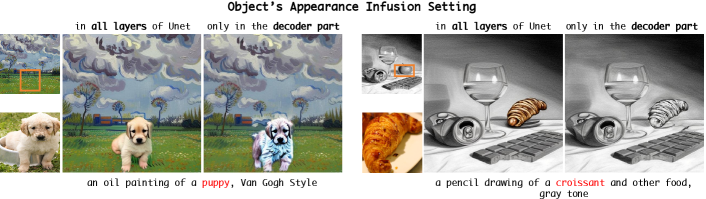
5. Experiments
5.1. Implementation Details
5.1.1. Test benchmark
We employ the only publicly released cross-domain composition benchmark (Lu et al., 2023), which contains 332 samples. Each sample consists of a background image, an object image, a foreground mask, an object mask, and a caption prompt. The background images comprise four visual domains: photorealism, pencil sketching, oil painting, and cartoon animation. We adjust all the caption prompts to mark the object-specific tokens. The details are left to supplementary.
5.1.2. Baselines
We conduct a qualitative comparison between our method and state-of-the-art baselines. The baselines includes Deep Image Blending (DIB) (Zhang et al., 2020a), Blended Diffusion (Avrahami et al., 2022), Paint by Example (Yang et al., 2023), SDEdit (Meng et al., 2022), and TF-ICON (Lu et al., 2023). For the quantitative assessment, all baselines are considered, excluding DCCF, as it is designed for harmonizing images after copy-and-paste operations.
5.1.3. Test configures
Given that most baselines are trained primarily in the photorealism domain, where objective metrics are more effective, we conducted our quantitative comparison specifically in this domain for fairness. For other domains, we relied on a user study and qualitative comparisons. We utilized the official implementation of all the baselines. Our framework employed the pre-trained Stable Diffusion with the second-order DPM-Solver++ in 20 steps. The hyperparameter for prior weights infusion was set to 0.2 and the scale of classifier-free guidance was set to 2.5 for the photorealism domain and 5 for other cross-domains.
5.1.4. Evaluation metrics
We evaluate our method using four metrics: (1) : measures background consistency based on the LPIPS metric (Zhang et al., 2018). (2) : evaluates the low-level similarity between the object region and the reference using the LPIPS metric (Zhang et al., 2018). (3) : assesses the semantic similarity between the object region and the reference in the CLIP embedding space (Zhang et al., 2020a). (4) : measures the semantic alignment between the text prompt and the resultant image (Radford et al., 2021).
Methods | ||||
---|---|---|---|---|
WACV’20 DIB(Zhang et al., 2020a) | 0.11 | 0.63 | 77.57 | 26.84 |
ICLR’22 SDEdit(Meng et al., 2022) | 0.42 | 0.66 | 77.68 | 27.98 |
CVPR’22 Blended(Avrahami et al., 2022) | 0.11 | 0.77 | 73.25 | 25.19 |
CVPR’23 Paint(Yang et al., 2023) | 0.13 | 0.73 | 80.26 | 25.92 |
ICCV’23 TF-ICON(Lu et al., 2023) | 0.10 | 0.60 | 82.86 | 28.11 |
Ours | 0.08 | 0.48 | 84.71 | 30.26 |
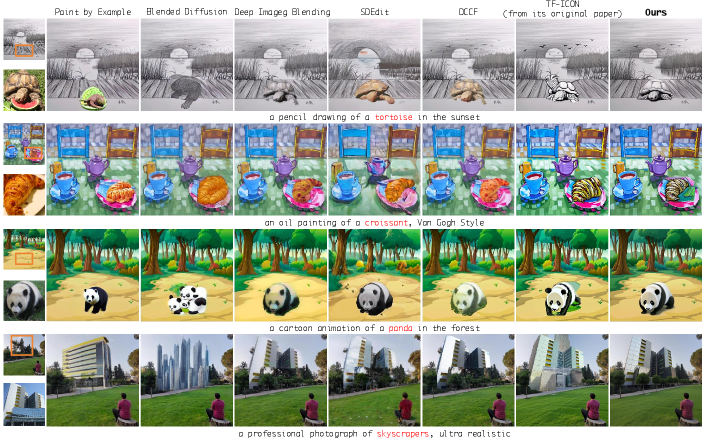
5.2. Qualitative Comparisons
The qualitative comparison results in Fig. 6 highlight the superior performance of PrimeComposer in seamlessly integrating objects across various domains while preserving their appearance and synthesizing natural coherence. Notably, some baselines, such as Paint by Example, face challenges in maintaining the appearance of the given object. Blended Diffusion, relying solely on text prompts, fails to ensure the synthesized object matches the reference image. Additionally, Deep Image Blending, DCCF and SDEdit struggle to achieve harmonious transitions. While TF-ICON performs relatively well compared to other methods, it still faces difficulties in simultaneously preserving object appearance and synthesizing natural coherence. For example, it fails to preserve the identity features of the tortoise in the first row and struggles to achieve optimal coherence around the synthesized building in the last row. Additional qualitative comparison results are left to supplementary.
5.3. Quantitative Analysis
As demonstrated in Tab. 1, our proposed PrimeComposer outperforms all competitors consistently across all metrics, highlighting the exceptional visual quality of the composite images it generates. Notably, TF-ICON also exhibits commendable generation quality. However, its composite self-attention map, derived from multiple samplers, introduces synthesis confusion. As a consequence, the realism and harmony of its resulting images are compromised. In contrast, our PrimeComposer Competently alleviates these challenges. Notably, PrimeComposer surpasses it by 1.85 and 2.15 on and , respectively. This demonstrates that our method outperforms previous approaches in preserving object appearance and achieving harmonious coherence synthesis.
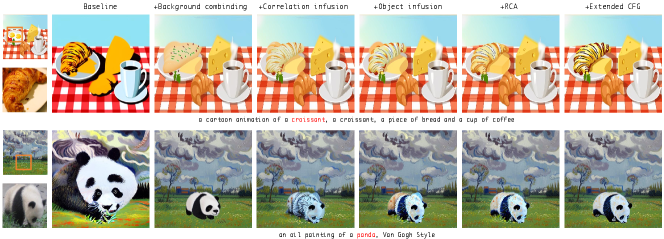
5.4. Inference Time Comparison
We conduct a comparison with the previous SOTA training-free method on an NVIDIA A100 40GB PCIe. Considering the time depends on the size of the user mask and reference image, we measure the averaged inference time per image across various domains in the test benchmark to ensure fairness. As shown in Tab. 2, PrimeComposer consistently exhibits faster inference times than TF-ICON across all domains. Remarkably, our inference time in the photorealism domain is notably lower than TF-ICON, specifically achieving a time of 14.32 seconds. This phenomenon is expected, considering that TF-ICON employs four samplers for composition, while our approach only utilizes two samplers, i.e., the pre-trained LDM and a Correlation Diffuser, during the process, resulting in a more efficient computational performance. See supplementary for additional inference speed comparisons with training baselines, which further proves the efficiency of our method.
5.5. User Study
We conduct a user study to compare image composition baselines across different domains. Specifically, we invited 30 participants through voluntary participation and assigned them the task of completing 40 ranking questions. The ranking criteria comprehensively considered factors including foreground preservation, background consistency, seamless composition, and text alignment. The results are presented in Tab. 4, where the domain information is formatted as ’foreground domain & background domain’. Notably, our method received favorable feedback from the majority of participants across various domains.
5.6. Ablation Study
we conduct ablations on key design choices in the following cases: (1) Baseline, where the composition is synthesized by LDM from T to 0 only with the caption prompt. The initial noise is the pixel composition derived from inverted codes of the given object and background; (2) Background combining is applied to maintain the unchanged scene; (3) Correlation infusion is employed to steer the natural coherent relation establishment; (4) Object infusion is employed to steer the preservation of the object’s appearance; (5) Region-constrained Cross-Attention is used to enforce the generation of objects in desired positions and shapes, addressing the coherence problem caused by the caption prompt; (6) Extended CFG, tailored to reinforce the steering impact of prior weights infusion.
Quantitative results: Tab. 3 presents quantitative ablation results, showcasing the superior performance of our complete algorithm across all metrics except . It is noteworthy that the baseline achieves the best result, as it generates compositions solely relying on the caption prompt without any additional constraints (Lu et al., 2023). These ablation results underline the effectiveness of our proposed algorithm in enhancing various aspects of the composition process.
Methods | PI in Cartoon | PI in Sketch | PI in Painting | PI in Photorealism |
---|---|---|---|---|
TF-ICON(Lu et al., 2023) | 28.98s | 29.12s | 29.75s | 30.55s |
Ours | 16.62s | 15.25s | 15.58s | 16.23s |
Methods | ||||
---|---|---|---|---|
Baseline | 0.40 | 0.56 | 74.68 | 31.32 |
+Background combining | 0.10 | 0.53 | 75.49 | 30.01 |
+Correlation infusion | 0.09 | 0.51 | 82.51 | 29.87 |
+Object infusion | 0.09 | 0.50 | 83.36 | 30.18 |
+RCA | 0.08 | 0.48 | 84.12 | 30.24 |
+Extended CFG | 0.08 | 0.48 | 84.71 | 30.26 |
Methods | P & P | P & O | P & S | P & C | Total |
---|---|---|---|---|---|
Blended(Meng et al., 2022) | 2.14 | 1.95 | 1.78 | 2.21 | 2.02 |
SDEdit(Avrahami et al., 2022) | 3.09 | 2.88 | 3.08 | 2.97 | 3.01 |
Paint(Yang et al., 2023) | 3.31 | 2.93 | 2.73 | 2.90 | 2.97 |
DCCF(Zhang et al., 2020a) | 3.76 | 3.35 | 3.52 | 3.58 | 3.55 |
TF-ICON(Lu et al., 2023) | 4.63 | 4.46 | 4.37 | 4.39 | 4.46 |
Ours | 4.87 | 5.43 | 5.52 | 4.95 | 5.19 |
Qualitative results: To further visualize the effectiveness of each design choice, we provide qualitative results shown in Fig. 7. These results directly prove the indispensable role of all design choices. More qualitative ablation results are left to supplementary.
6. Conclusion
In this paper, we formulate image composition as a subject-guided local image editing task and propose a faster training-free diffuser, PrimeComposer. Leveraging well-designed attention steering, primarily through the Correlation Diffuser, our method seamlessly integrates foreground objects into noisy backgrounds while maintaining scene consistency. The introduction of Region-constrained Cross-Attention further enhances coherence and addresses unwanted artifacts in prior methods. Our approach demonstrates the fastest inference efficiency and outperforms existing methods both qualitatively and quantitatively in extensive experiments.
7. Acknowledgments
This work was supported by National Natural Science Fund of China (62176064) and Shanghai Municipal Science and Technology Commission (22dz1204900).
References
- (1)
- Avrahami et al. (2023) Omri Avrahami, Ohad Fried, and Dani Lischinski. 2023. Blended latent diffusion. ACM Transactions on Graphics 42, 4 (2023), 1–11.
- Avrahami et al. (2022) Omri Avrahami, Dani Lischinski, and Ohad Fried. 2022. Blended diffusion for text-driven editing of natural images. In CVPR. 18208–18218.
- Azadi et al. (2020) Samaneh Azadi, Deepak Pathak, Sayna Ebrahimi, and Trevor Darrell. 2020. Compositional gan: Learning image-conditional binary composition. IJCV 128 (2020), 2570–2585.
- Brown et al. (2022) Andrew Brown, Cheng-Yang Fu, Omkar Parkhi, Tamara L Berg, and Andrea Vedaldi. 2022. End-to-end visual editing with a generatively pre-trained artist. In ECCV. Springer, 18–35.
- Burt and Adelson (1987) Peter J Burt and Edward H Adelson. 1987. The Laplacian pyramid as a compact image code. In Readings in computer vision. 671–679.
- Cai et al. (2024) Yanlu Cai, Weizhong Zhang, Yuan Wu, and Cheng Jin. 2024. PoseIRM: Enhance 3D Human Pose Estimation on Unseen Camera Settings via Invariant Risk Minimization. In CVPR. 2124–2133.
- Chefer et al. (2023) Hila Chefer, Yuval Alaluf, Yael Vinker, Lior Wolf, and Daniel Cohen-Or. 2023. Attend-and-excite: Attention-based semantic guidance for text-to-image diffusion models. ACM Transactions on Graphics 42, 4 (2023), 1–10.
- Chen and Kae (2019) Bor-Chun Chen and Andrew Kae. 2019. Toward realistic image compositing with adversarial learning. In CVPR. 8415–8424.
- Cong et al. (2020) Wenyan Cong, Jianfu Zhang, Li Niu, Liu Liu, Zhixin Ling, Weiyuan Li, and Liqing Zhang. 2020. Dovenet: Deep image harmonization via domain verification. In CVPR. 8394–8403.
- Cun and Pun (2020) Xiaodong Cun and Chi-Man Pun. 2020. Improving the harmony of the composite image by spatial-separated attention module. IEEE Transactions on Image Processing 29 (2020), 4759–4771.
- Dwibedi et al. (2017) Debidatta Dwibedi, Ishan Misra, and Martial Hebert. 2017. Cut, paste and learn: Surprisingly easy synthesis for instance detection. In ICCV. 1301–1310.
- Feng et al. (2022) Weixi Feng, Xuehai He, Tsu-Jui Fu, Varun Jampani, Arjun Akula, Pradyumna Narayana, Sugato Basu, Xin Eric Wang, and William Yang Wang. 2022. Training-free structured diffusion guidance for compositional text-to-image synthesis. arXiv preprint arXiv:2212.05032 (2022).
- Gafni and Wolf (2020) Oran Gafni and Lior Wolf. 2020. Wish you were here: Context-aware human generation. In CVPR. 7840–7849.
- Gal et al. (2022) Rinon Gal, Yuval Alaluf, Yuval Atzmon, Or Patashnik, Amit H Bermano, Gal Chechik, and Daniel Cohen-Or. 2022. An image is worth one word: Personalizing text-to-image generation using textual inversion. arXiv preprint arXiv:2208.01618 (2022).
- Gal et al. (2023) Rinon Gal, Moab Arar, Yuval Atzmon, Amit H Bermano, Gal Chechik, and Daniel Cohen-Or. 2023. Designing an encoder for fast personalization of text-to-image models. arXiv preprint arXiv:2302.12228 (2023).
- Ho et al. (2020) Jonathan Ho, Ajay Jain, and Pieter Abbeel. 2020. Denoising diffusion probabilistic models. NeurIPS 33 (2020), 6840–6851.
- Ho and Salimans (2022) Jonathan Ho and Tim Salimans. 2022. Classifier-Free Diffusion Guidance. arXiv preprint arXiv:2207.12598 (2022).
- Hong et al. (2022) Yan Hong, Li Niu, and Jianfu Zhang. 2022. Shadow generation for composite image in real-world scenes. In AAAI, Vol. 36. 914–922.
- Jiang et al. (2021) Yifan Jiang, He Zhang, Jianming Zhang, Yilin Wang, Zhe Lin, Kalyan Sunkavalli, Simon Chen, Sohrab Amirghodsi, Sarah Kong, and Zhangyang Wang. 2021. Ssh: A self-supervised framework for image harmonization. In ICCV. 4832–4841.
- Kawar et al. (2023) Bahjat Kawar, Shiran Zada, Oran Lang, Omer Tov, Huiwen Chang, Tali Dekel, Inbar Mosseri, and Michal Irani. 2023. Imagic: Text-based real image editing with diffusion models. In CVPR. 6007–6017.
- Kumari et al. (2023) Nupur Kumari, Bingliang Zhang, Richard Zhang, Eli Shechtman, and Jun-Yan Zhu. 2023. Multi-concept customization of text-to-image diffusion. In CVPR. 1931–1941.
- Li et al. (2023) Yuheng Li, Haotian Liu, Qingyang Wu, Fangzhou Mu, Jianwei Yang, Jianfeng Gao, Chunyuan Li, and Yong Jae Lee. 2023. Gligen: Open-set grounded text-to-image generation. In CVPR. 22511–22521.
- Lin et al. (2018) Chen-Hsuan Lin, Ersin Yumer, Oliver Wang, Eli Shechtman, and Simon Lucey. 2018. St-gan: Spatial transformer generative adversarial networks for image compositing. In CVPR. 9455–9464.
- Liu et al. (2020) Daquan Liu, Chengjiang Long, Hongpan Zhang, Hanning Yu, Xinzhi Dong, and Chunxia Xiao. 2020. Arshadowgan: Shadow generative adversarial network for augmented reality in single light scenes. In CVPR. 8139–8148.
- Liu et al. (2021) Liu Liu, Zhenchen Liu, Bo Zhang, Jiangtong Li, Li Niu, Qingyang Liu, and Liqing Zhang. 2021. OPA: object placement assessment dataset. arXiv preprint arXiv:2107.01889 (2021).
- Liu et al. (2022) Nan Liu, Shuang Li, Yilun Du, Antonio Torralba, and Joshua B Tenenbaum. 2022. Compositional visual generation with composable diffusion models. In ECCV. Springer, 423–439.
- Lu et al. (2022) Cheng Lu, Yuhao Zhou, Fan Bao, Jianfei Chen, Chongxuan Li, and Jun Zhu. 2022. Dpm-solver++: Fast solver for guided sampling of diffusion probabilistic models. arXiv preprint arXiv:2211.01095 (2022).
- Lu et al. (2023) Shilin Lu, Yanzhu Liu, and Adams Wai-Kin Kong. 2023. TF-ICON: Diffusion-based training-free cross-Domain image composition. In ICCV. 2294–2305.
- Meng et al. (2022) Chenlin Meng, Yutong He, Yang Song, Jiaming Song, Jiajun Wu, Jun-Yan Zhu, and Stefano Ermon. 2022. SDEdit: Guided Image Synthesis and Editing with Stochastic Differential Equations. In ICLR.
- Niu et al. (2024a) Li Niu, Junyan Cao, Yan Hong, and Liqing Zhang. 2024a. Painterly Image Harmonization by Learning from Painterly Objects. In AAAI, Vol. 38. 4343–4351.
- Niu et al. (2021) Li Niu, Wenyan Cong, Liu Liu, Yan Hong, Bo Zhang, Jing Liang, and Liqing Zhang. 2021. Making images real again: A comprehensive survey on deep image composition. arXiv preprint arXiv:2106.14490 (2021).
- Niu et al. (2024b) Li Niu, Yan Hong, Junyan Cao, and Liqing Zhang. 2024b. Progressive Painterly Image Harmonization from Low-Level Styles to High-Level Styles. In AAAI, Vol. 38. 4352–4360.
- Radford et al. (2021) Alec Radford, Jong Wook Kim, Chris Hallacy, Aditya Ramesh, Gabriel Goh, Sandhini Agarwal, Girish Sastry, Amanda Askell, Pamela Mishkin, Jack Clark, et al. 2021. Learning transferable visual models from natural language supervision. In ICML. 8748–8763.
- Rombach et al. (2022) Robin Rombach, Andreas Blattmann, Dominik Lorenz, Patrick Esser, and Björn Ommer. 2022. High-resolution image synthesis with latent diffusion models. In CVPR. 10684–10695.
- Ruiz et al. (2023) Nataniel Ruiz, Yuanzhen Li, Varun Jampani, Yael Pritch, Michael Rubinstein, and Kfir Aberman. 2023. Dreambooth: Fine tuning text-to-image diffusion models for subject-driven generation. In CVPR. 22500–22510.
- Sheng et al. (2021) Yichen Sheng, Jianming Zhang, and Bedrich Benes. 2021. SSN: Soft shadow network for image compositing. In CVPR. 4380–4390.
- Song et al. (2022) Yizhi Song, Zhifei Zhang, Zhe Lin, Scott Cohen, Brian Price, Jianming Zhang, Soo Ye Kim, and Daniel Aliaga. 2022. Objectstitch: Generative object compositing. arXiv preprint arXiv:2212.00932 (2022).
- Tripathi et al. (2019) Shashank Tripathi, Siddhartha Chandra, Amit Agrawal, Ambrish Tyagi, James M Rehg, and Visesh Chari. 2019. Learning to generate synthetic data via compositing. In CVPR. 461–470.
- Vaswani et al. (2017) Ashish Vaswani, Noam Shazeer, Niki Parmar, Jakob Uszkoreit, Llion Jones, Aidan N Gomez, Łukasz Kaiser, and Illia Polosukhin. 2017. Attention is all you need. In NeurIPS, Vol. 30.
- Wang et al. (2023) Yibin Wang, Yuchao Feng, Jie Wu, Honghui Xu, and Jianwei Zheng. 2023. CA-GAN: Object placement via coalescing attention based generative adversarial network. In ICME. 2375–2380.
- Wang et al. (2024a) Yibin Wang, Yuchao Feng, and Jianwei Zheng. 2024a. Learning Object Placement via Convolution Scoring Attention. In BMVC. 1–12.
- Wang et al. (2024b) Yibin Wang, Weizhong Zhang, Jianwei Zheng, and Cheng Jin. 2024b. High-fidelity Person-centric Subject-to-Image Synthesis. In CVPR. 7675–7684.
- Witteveen and Andrews (2022) Sam Witteveen and Martin Andrews. 2022. Investigating prompt engineering in diffusion models. arXiv preprint arXiv:2211.15462 (2022).
- Wu et al. (2019) Huikai Wu, Shuai Zheng, Junge Zhang, and Kaiqi Huang. 2019. Gp-gan: Towards realistic high-resolution image blending. In ACM MM. 2487–2495.
- Xue et al. (2022) Ben Xue, Shenghui Ran, Quan Chen, Rongfei Jia, Binqiang Zhao, and Xing Tang. 2022. Dccf: Deep comprehensible color filter learning framework for high-resolution image harmonization. In ECCV. Springer, 300–316.
- Yang et al. (2023) Binxin Yang, Shuyang Gu, Bo Zhang, Ting Zhang, Xuejin Chen, Xiaoyan Sun, Dong Chen, and Fang Wen. 2023. Paint by example: Exemplar-based image editing with diffusion models. In CVPR. 18381–18391.
- Zhang et al. (2023a) Bo Zhang, Yuxuan Duan, Jun Lan, Yan Hong, Huijia Zhu, Weiqiang Wang, and Li Niu. 2023a. ControlCom: Controllable image composition using diffusion model. arXiv preprint arXiv:2308.10040 (2023).
- Zhang et al. (2021) He Zhang, Jianming Zhang, Federico Perazzi, Zhe Lin, and Vishal M Patel. 2021. Deep image compositing. In WACV. 365–374.
- Zhang et al. (2020b) Lingzhi Zhang, Tarmily Wen, Jie Min, Jiancong Wang, David Han, and Jianbo Shi. 2020b. Learning object placement by inpainting for compositional data augmentation. In ECCV. Springer, 566–581.
- Zhang et al. (2020a) Lingzhi Zhang, Tarmily Wen, and Jianbo Shi. 2020a. Deep image blending. In WACV. 231–240.
- Zhang et al. (2018) Richard Zhang, Phillip Isola, Alexei A Efros, Eli Shechtman, and Oliver Wang. 2018. The unreasonable effectiveness of deep features as a perceptual metric. In CVPR. 586–595.
- Zhang et al. (2019) Shuyang Zhang, Runze Liang, and Miao Wang. 2019. Shadowgan: Shadow synthesis for virtual objects with conditional adversarial networks. Computational Visual Media 5 (2019), 105–115.
- Zhang et al. (2023b) Shiwen Zhang, Shuai Xiao, and Weilin Huang. 2023b. Forgedit: Text guided image editing via learning and forgetting. arXiv preprint arXiv:2309.10556 (2023).
Appendix A Algorithms
The computation pipeline of our PrimeComposer and RCA are illustrated in Algorithm 1 and Algorithm 2, respectively.
Appendix B Preprocessing the Test Benchmark
To effectively alleviate the unwanted artifacts appearing around the synthesized objects, we propose RCA to restrict the impact of object-specific tokens. To identify these tokens, we adjust the prompts by incorporating special tags, denoted as , placed before and after target words through manual annotation. This adjustment facilitates the precise marking of object-specific tokens. For instance, the original caption ’a cartoon animation of a white fox in the forest’ is adjusted to ’a cartoon animation of a white fox in the forest’. Before the composition process, we identify and record the indices of these specially tagged tokens for each input sample, ensuring targeted and effective region-constrained attention during synthesis. We will release the preprocessed benchmark to the public.
Appendix C Additional Inference Time Comparison
Given that most training baselines are primarily trained in the photorealism domain, we exclusively compare the inference speed with them within photorealism domains using an NVIDIA A100 40GB PCIe. As depicted in Table 5, our PrimeComposer demonstrates faster inference speed than all the considered baselines, underscoring our superior efficiency in this task.
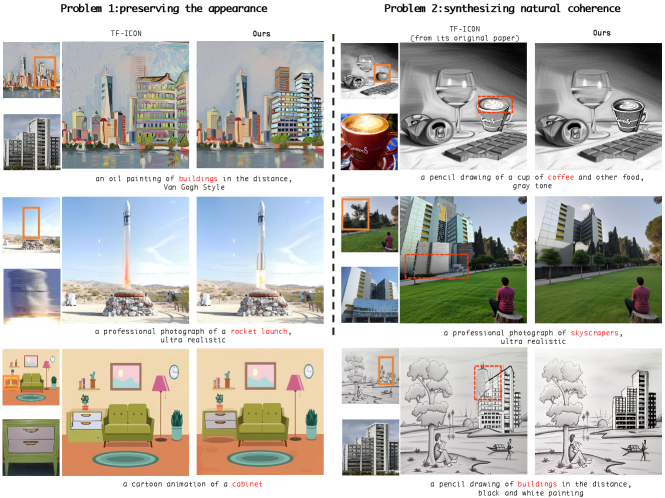
Appendix D Societal Impacts
The widespread use of PrimeComposer in image composition has some interesting effects on how we create and see pictures. One potential impact is that it might lead to misunderstandings or misrepresentations of different cultures. People could unintentionally use this tool to mix and match cultural symbols, possibly spreading stereotypes or watering down the true meaning behind these symbols. To avoid this, it’s important to educate users on cultural sensitivity. Another thing to think about is how easy it becomes to create fake pictures that look real. As more and more people use tools like PrimeComposer, it might get harder to tell if a picture is genuine or if it has been altered. This could make it challenging for people to trust what they see online and might require us to be more careful and critical when looking at pictures. The way we think about art and creativity might also change. With tools like PrimeComposer, anyone can create unique and diverse images easily. While this is exciting, it might challenge traditional ideas about who gets credit for creating something. It raises questions about who owns the rights to these images and what it means to be a creator in a world where machines assist in the creative process.
In essence, PrimeComposer opens up new possibilities for creativity, but it also brings up important issues around cultural understanding, trust in images, and the evolving nature of art and creativity in a tech-driven world. Addressing these concerns ensures that technology contributes positively to how we express ourselves and understand the world around us.
Appendix E Additional Cases of Challenges
Additional cases of challenges the current SOTA method encountered are exhibited in Fig. 8.
Appendix F Additional Qualitative Results
Appendix G Additional Ablation
Additional ablation studies are exhibited in Fig. 11.
Appendix H Visualizatin of Our Extended CFG
Classifier-free guidance is extended in our work to reinforce the steering effect of the infused prior weights in foreground generation. The extended CFG is defined as
To qualitatively assess its effectiveness, we present the averaged saliency map (SM) in Fig. 12. These visualizations showcase the extended CFG facilitates establishing coherent relations and preserving the object’s appearance. This phenomenon aligns with our design philosophy.
Appendix I Limitation
Firstly, our approach faces a common challenge in the field, which is the limited ability to freely control the object’s viewpoint. While efforts have been made to address this concern, as demonstrated in (Zhang et al., 2023a), it typically involves resource-intensive training processes. Secondly, our current methodology cannot seamlessly integrate multiple objects into the background simultaneously. This aspect poses a significant challenge in application scenarios where compositions involve more complex scenes or diverse elements. Thirdly, although our method demonstrates accelerated inference times compared to the previous approaches, we acknowledge that the current speed of reasoning may not fully meet the demands of practical applications. We recognize the need for further optimizations to enhance the efficiency of our method and make it more suitable for real-time or near-real-time applications.
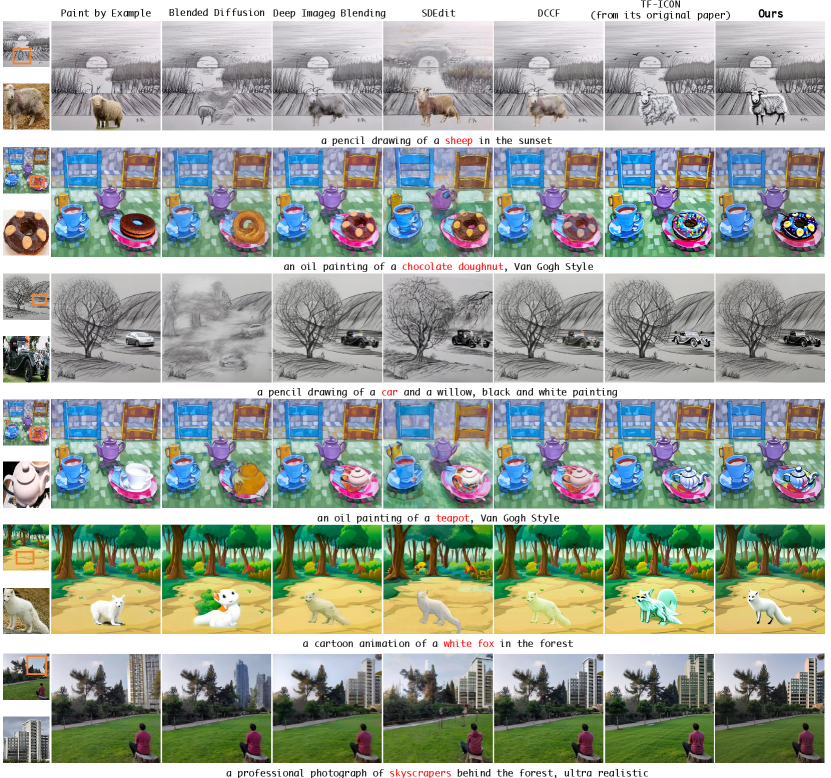
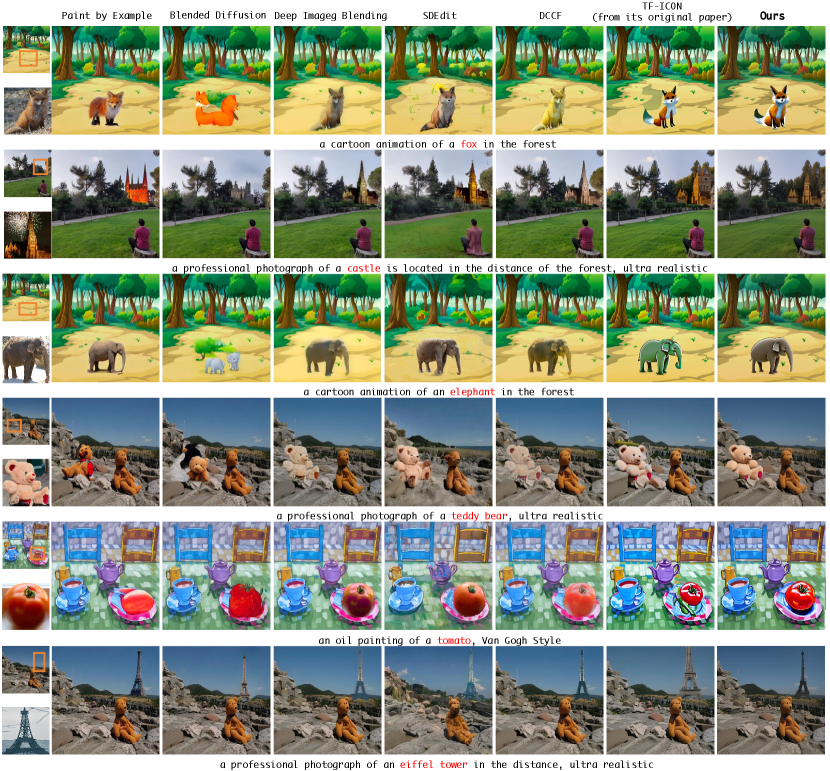
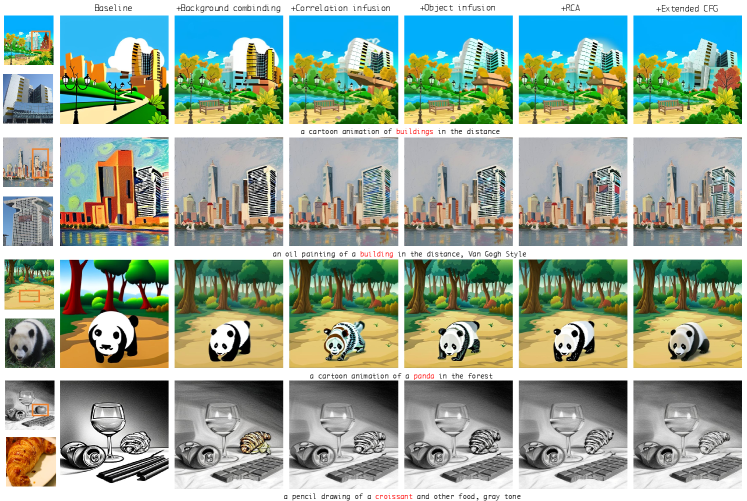
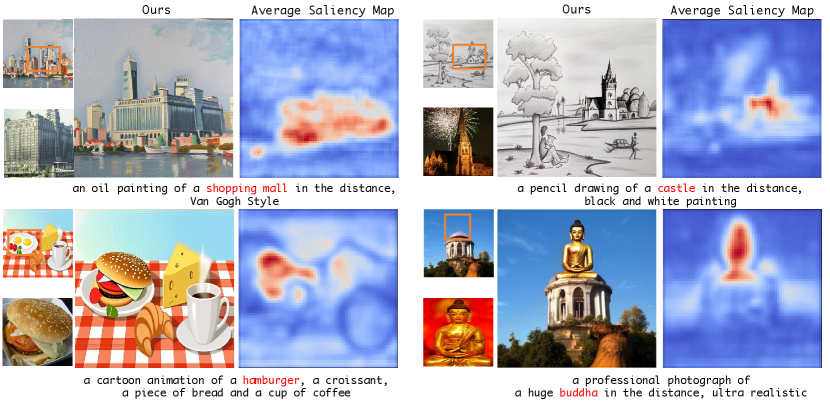