Paragraph-to-Image Generation with Information-Enriched Diffusion Model
Abstract
Text-to-image (T2I) models have recently experienced rapid development, achieving astonishing performance in terms of fidelity and textual alignment capabilities. However, given a long paragraph (up to 512 words), these generation models still struggle to achieve strong alignment and are unable to generate images depicting complex scenes. In this paper, we introduce an information-enriched diffusion model for paragraph-to-image generation task, termed ParaDiffusion, which delves into the transference of the extensive semantic comprehension capabilities of large language models to the task of image generation. At its core is using a large language model (e.g., Llama V2) to encode long-form text, followed by fine-tuning with LoRA to align the text-image feature spaces in the generation task. To facilitate the training of long-text semantic alignment, we also curated a high-quality paragraph-image pair dataset, namely ParaImage. This dataset contains a small amount of high-quality, meticulously annotated data, and a large-scale synthetic dataset with long text descriptions being generated using a vision-language model.
Experiments demonstrate that ParaDiffusion outperforms state-of-the-art models (SD XL, DeepFloyd IF) on ViLG-300 and ParaPrompts, achieving up to and human voting rate improvements for visual appeal and text faithfulness, respectively. The code and dataset will be released to foster community research on long-text alignment.
The project website is at: ParaDiffusion.
![[Uncaptioned image]](https://cdn.awesomepapers.org/papers/4cd24d50-a384-48f7-9f5d-1b208726d377/x1.png)
1 Introduction
Recently, text-to-image (T2I) generative models have emerged as focal points of scholarly attention and progress within the computer version field. Some notable works, such as Stable Diffusion [27] of Stability AI, DALL-E2 [26] of OpenAI, Imagen [30] of Google, RAPHAEL [40] of SenseTime, and Emu [4] of Meta, have achieved substantial progress, accomplishments, and influence for text-based image generation tasks. As the name implies, semantic alignment proficiency is paramount for text-guided image generation tasks, wherein the model is required to generate image content corresponding to any provided textual description. The majority of current T2I models focus on generating high-quality images based on processing relatively short textual inputs and simple descriptions. The long-text semantic alignment in the field of text-guided image generation still poses a few challenges.
Given a comprehensive paragraph, as depicted in Figure 1, extending up to words, the generation model is required to generate a detailed image that encompasses all the objects, attributes, and spatial positions mentioned within the given paragraph. For such a task, we define it as paragraph-to-image generation. The majority of current T2I models are unable to handle such intricate long-text semantic alignment, primarily due to the two constraints: 1) Data limitations. The mainstream public text-image pair dataset, LAION-5B [32], offers only simple text-image pair information, with an average textual description consisting of approximately words, which suffer from a lack of informative content. The simplicity of the image-text descriptions limits the model to learn complex and long-text semantic alignment. 2) Architecture constraints. Existing T2I models, such as Stable Diffusion and DALL-E2, employ CLIP [24] trained on LAION-5B as the text encoder, which only supports a maximum of tokens. Recently, DeepFloyd [5] and PIXART- [3] have attempted to leverage the powerful textual understanding capabilities of large language model (e.g., T5-XXL [25]) for text-image generation tasks, and demonstrated that T5-XXL outperforms CLIP in image-text alignment. Note that, these works merely extended the text embedding of text encoder from tokens to tokens without investigating the semantic alignment ability for long-text inputs. Furthermore, the T5-XXL model is trained on pure text data without prior knowledge of image-text pairs, and aligning text embeddings of the frozen T5-XXL with visual features in a brute-force manner may not be the optimal solution.
In this paper, we explore solutions of long-term text and image alignment from the perspectives of both training data and the network structure.
For the first challenge, we construct and present a high-quality, textual rich paragraph-to-image pairs dataset, namely ParaImage, where the corresponding textual descriptions can extend up to 400 words. The long-term text description encompasses the objects, attributes, and spatial locations in the image, along with the corresponding visual style. ParaImage consists primarily of two types of data: 1) ParaImage-Big with Generative Captions. We select four million high-quality images from LAION 5B and employ a powerful vision-language model (i.e., CogVLM [37]) to generate semantically rich textual descriptions. This dataset is primarily utilized to achieve alignment between long text and images, enabling the diffusion model to perceive the rich semantic information embedded in lengthy textual descriptions. 2) ParaImage-Small with Manual Captions. A few thousand high-quality images are thoughtfully selected from a dataset with million of images with some common principles in photography, then professionally annotated by skilled annotators. Considering the inherent limitations in precision associated with synthetic data and the efficiency of quality-tuning [4], it is necessary to construct a manually annotated, high-quality paragraph-image pair dataset for the final stage of quality tuning.
For the second challenge, we explore the transfer of long-text semantic understanding capabilities from the state-of-the-art large language models, i.e., Llama V2 [35], to the paragraph-to-image generation task. To harness the robust performance of LLM more effectively, different from the prior methods using frozen weight, we design an efficient training strategy to fine-tune Llama V2 concurrently with the optimization of diffusion models. This ensures that the extracted text embeddings are more compatible with the text-image pair space. Besides, the design enables the adaptation of a decoder-only LLM to text-to-image generation tasks. This, in turn, allows us to leverage the advantages of a decoder-only LLM (i.e., Llama V2), such as the powerful understanding ability from the larger training text corpus (four times that of T5). We show some generated examples by ParaDiffusion in Figure 1.
Our main contributions are summarized as follows:
-
1.
For the first time, we explore the long-form text alignment challenge for the image generation task, namely, the paragraph-to-image generation task. A comprehensive solution from both data and algorithmic aspects is provided for the challenging setting.
-
2.
We introduce a high-quality, rich-text paragraph-to-image pairs dataset, namely ParaImage, where the associated textual descriptions extend up to 400 words, meticulously documenting object identities, properties, spatial relationships, and image style, etc.
-
3.
We re-evaluate how to better transfer the semantic understanding capabilities of LLM to the text-image generation task, proposing an effective training strategy for fine-tuning LLM, e.g., Llama V2.
-
4.
Experiments demonstrate that ParaDiffusion outperforms state-of-the-art models (SD XL, DeepFloyd IF) on ViLG-300 and ParaPrompts, achieving up to and human voting rate improvements for visual appeal and text faithfulness, respectively.
2 Related Work
Text-to-Image Models. Text-to-image generation [10] require the model to generate an image corresponding to a given textual description. Recently, diffusion-based methods [27, 26, 4, 41, 7, 8, 2, 13] have demonstrated remarkable performance and found applications in various downstream tasks, including controllable generation [42, 29], controlled editing [9], and perception tasks [39, 38]. Stable Diffusion [27] enhances and accelerates the traditional DDPM [10] by conducting denoising processes in the latent space. Imagen [30] firstly incorporates large frozen language models (i.e., T5XXL [25]) as text encoders for text-to-image generation tasks, demonstrating their significant performance. DALL-E2 utilizes CLIP [24] as a text encoder and a diffusion model [31] as a decoder to address text-to-image generation tasks. Emu [4], on the other hand, introduces new insight that supervised fine-tuning with small but high-quality, visually appealing images can significantly improve the generation quality. RAPHAEL [40], ERNIE-ViLG [6], and Ediffi [1] approach the task of text-to-image generation from the perspective of an ensemble of expert denoisers, exploring potential gains in performance.
Recently, DeepFloyd [5] and PIXART- [3] further validate the superior text-image semantic alignment capabilities of large language models over CLIP, as they both employ T5 XXL as a text encoder. However, these models still solely explore short-text textual alignment tasks, restricting the text encoder to within 128 tokens. They do not delve deeply into unlocking the full potential of large language models (LLM) and lack exploration of data methodologies. In contrast to these approaches, we further investigate the gains in rich paragraph-image alignment capabilities offered by the state-of-the-art language model (i.e., Llama V2 [35]). Additionally, we provide an effective solution from a data-centric perspective.
Large Language Models. Large language models primarily consist of two mainstream architectures: the Encoder-Decoder architecture [25, 17] and the decoder-only architecture [34, 35, 22]. Recently, decoder-only models, such as ChatGPT [22], GPT-4, Llama V1 [34], and Llama V2 [35], have exerted significant influence and advancements across various domains. But for encoder-decoder architecture, as far as we know, the latest one, T5 [25], was proposed three years ago. Decoder-only architectures have achieved significant victories in both training data scale and semantic understanding performance. However, existing text-to-image models [30, 5, 3], only conduct exploration of the encoder-decoder architecture, i.e., T5 [25], as a text encoder. This choice is made under the assumption that the decoder-only architecture might not efficiently extract textual features for text-image task. In this paper, we propose a universal and efficient fine-tuning strategy to adapt any decoder-only architecture (e.g., Llama V2 [35]) to a text-guided image generation model. We present an intriguing insight: directly using frozen LLMs [30, 5] as text encoders is not an elegant solution, while LLMs are trained on pure text data, without considering whether the text embedding is suitable for the text-image feature space. Inspired by the success of instruction-tuning [36, 16] and LoRA [11], we propose a strategy for paragraph-image alignment learning with language model adaptation. This involves freezing the pretrained Large Language Model weights and introducing a certain degree of trainable parameters to learn the textual relationships between paragraphs and images.
3 Approach
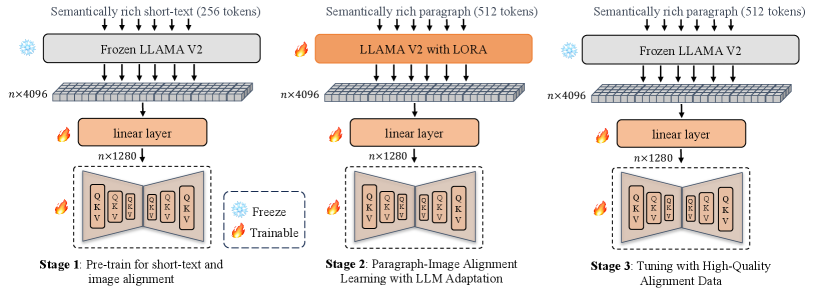
As discussed earlier, we propose a comprehensive solution at both the data and architecture levels for the paragraph-to-image generation task. Thus, this section is divided into two parts: 1) Algorithm Level (§3.1). We introduce a paragraph-to-image generation architecture with diffusion model. 2) Dataset Level (§3.2). we present a high-quality, textual rich paragraph-to-image pairs dataset, where the corresponding textual descriptions can extend up to words.
3.1 Algorithm: ParaDiffusion
Following LDM [27], our architecture consists of three components: a text encoder to encode textual descriptions, an autoencoder (AE) to encode an image into latent embeddings, and a U-Net [28] to learn the denoising process.
3.1.1 Text Paragraph Encoder
Prior works [5, 3] directly utilize frozen encoder-decoder language model (i.e., T5 [25]) for short-text to image generation tasks, restricting the text encoder to within 128 tokens. We present a insight that efficiently fine-tuning a more powerful decoder-only language model can yield stronger performance in long-text alignment (up to 512 tokens). Compared to the encoder-decoder architecture of large language models, recent decoder-only models, such as ChatGPT [22], and Llama V2 [35], have garnered more attention and success. There are some evident advantages to using the decoder-only language models as text encoders: 1) Models like Llama and the GPT series, based on the decoder-only architecture, exhibit stronger semantic understanding and generalization capabilities. 2) The training corpora are also nearly four times larger than that of the encoder-decoder language model, i.e., T5 [25]. However, typically, decoder-only architectures are not adept at feature extraction and mapping tasks. Therefore, we propose Paragraph-Image Alignment Learning with Language Model Adaptation to address this issue, adapting the decoder-only model, i.e., Llama V2 [35] for text-to-image generation tasks.
3.1.2 Paragraph-Image Alignment Learning with Language Model Adaptation
Given a text description , the standard Text to Image Diffusion Models is probabilistic model designed to model conditional distributions of the form , where a conditional denoising autoencoder is used to learn the reverse process of a fixed Markov Chain of length . The corresponding objective can be simplified to:
(1) |
where denotes the text encoder. refers to a AE [27] for mapping image to latent feature. Typically, during training, we optimize only the Unet of the diffusion model , while freezing the . This is because we consider the text embedding from , i.e., CLIP, to be well-suited for text-image alignment tasks. However, when using an LLM as the text encoder, it is essential to consider whether a frozen LLM is appropriate for this context.
Inspired by the success of instruction-tuning [36, 16] and LoRA [11], we propose a strategy for paragraph-image alignment learning with language model adaptation. This involves freezing the pretrained Large Language Model weights and introducing a certain degree of trainable parameters to learn the textual relationships between paragraphs and images. The objective is revised as follows:
(2) |
where both and can be jointly optimized for learning better text representation. Compared to direct fine-tuning, this strategy offers two advantages: 1) During the training of paragraph-image alignment, it preserves the powerful semantic understanding capabilities of the LLM, preventing knowledge overfitting to simple text-image semantics. 2) Storage and compute efficient. Requires only limited computational resources and incurs no additional inference costs.
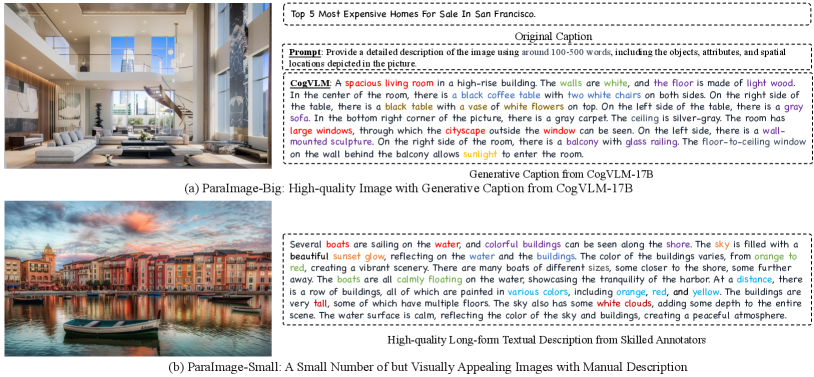
3.1.3 Training Strategy
As shown in Figure 2, ParaDiffusion adopts a three-stage training approach. Similar to the prior works [4, 30], stage 1 is employed to acquire general text-image semantic alignment knowledge. Stage 2 is introduced to simultaneously fine-tune the LLM and the diffusion model for paragraph-image alignment. In Stage 3, a high-quality small dataset, consisting of carefully selected images, is used to further enhance the model performance.
Stage 1: Pre-train for Short-text and Image Alignment In this stage, we gathered a dataset of 300 million examples to train the Unet of diffusion model with 1.3 billion parameters. Among these, one billion examples were selected from the 5 billion images of the LAION dataset [32]. Additionally, we constructed an internal dataset containing over 200 million examples. Similar to SDXL [23], the model was trained with progressively increasing resolutions. It started with training at a resolution of 256 and was later adjusted to a resolution of 512. The entire pre-training process took 5 days on 56 A100 GPUs.
Stage 2: Paragraph-Image Alignment Learning with LLM Adaptation. To perform paragraph-image alignment learning (§ 3.1.2), we construct a large scale dataset with several million paragraph-image pairs, namely ParaImage-Big, where the long-form text is generated by CogVLM [37], as illustrated in Figure 3(a). The entire paragraph-image alignment learning process took 2 days on 56 A100 GPUs.
Stage 3: High-Quality Alignment Data Finally, we created an extremely small but high-quality dataset, namely ParaImage-Small, to further enhance the performance of model with a small learning rate. This dataset consists of 3 manually selected images, each accompanied by human-annotated long-text descriptions. These descriptions provide detailed information about the objects in the images, including their attributes and spatial relationships.
3.2 Dataset: ParaImage
3.2.1 Image with Generative Captions
Inspired by the success of current large vision-language models [37, 43, 19], we propose to leverage the SOTA model, i.e., CogVLM for automatically generating extensive long-form textual annotations for images. Firstly, we gathered approximately 3.3 million high-quality images from the LAION-Aesthetics [15] and SAM datasets [14]. For the LAION-Aesthetics, we downloaded around million images with aesthetics scores above and then filtered them to a set of million images with a minimum short edge resolution of 512 pixels. For SAM dataset, we obtained 2 million images and further filtered out those with mosaic elements, resulting in a final dataset of around 100k images. Then, we prompted CogVLM to generate corresponding long-form textual descriptions for the images, as illustrated in Figure 3(a). Figure 4 and Table 1 present a detailed statistical comparison, and it is evident that in terms of textual descriptions, the captions of the proposed dataset are richer and longer in semantic content. of captions from LAION have a text length of fewer than 25 words, whereas over of captions from ParaImage-Big exceed words, with a few extending to over 200 words.
3.2.2 ParaImage-Small: Image with Manual Captions
The generated captions from CogVLM cannot be guaranteed to be accurate; therefore, it is essential to create a small but high-quality dataset with manual annotations (i.e., ParaImage-Small). This dataset consists of k, manually selected by annotators from exquisite images of LAION-Aesthetics, adhering to common principles in photography. Then, the images were annotated with corresponding long-text descriptions, detailing the objects in the images, the attributes of these objects, and their spatial relationships. The details for Paragraph Description Annotation Rule are as follows:
-
1.
The description should be no less than words and no more than words.
-
2.
The first sentence of the description should commence with a portrayal of the main subject of the image, e.g., A close-up photo of a beautiful woman.
-
3.
The description should be objective and avoid subjective emotions and speculations, such as (‘On a cloudy day, it might rain in the future’).
-
4.
The description should cover details about the objects’ number, color, appearance, and location.
-
5.
The description should follow a logical sequence, such as from left to right or from the center outward.
-
6.
Avoid using prefixes like ‘In the picture is,’ ‘This image depicts’, or ‘Here is.’
Dataset | Image | Caption | ||
---|---|---|---|---|
Number | Short Side | Ave. Words | Ave. Nouns | |
LAION [32] | 2.3b | 537.2 | 11.8 | 6.4 |
LAION-Aesthetics [15] | 625k | 493.6 | 11.3 | 6.8 |
ParaImage-Big | 3.3m | 771.3 | 132.9 | 46.8 |
ParaImage-Small | 3.1k | 1326.2 | 70.6 | 34.2 |
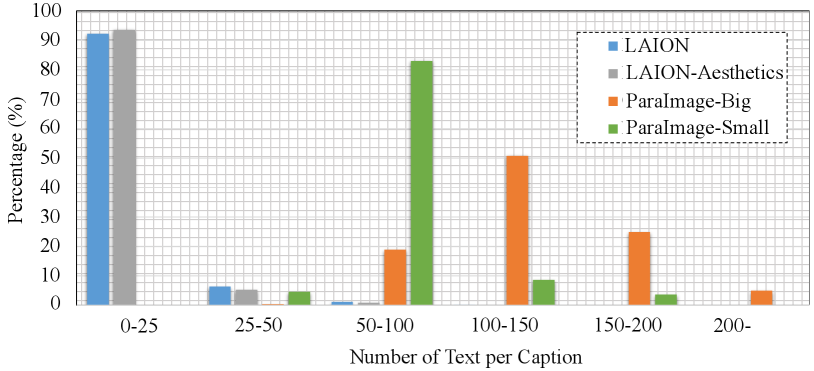
Details regarding the selection principles of images are provided in the supplementary materials. Based on the images selection annotation rules, we invited and trained annotators to score the images from to based on aesthetics and content, with being the highest score. There are two rounds of quality inspection, each round involving staff members for review. Finally, we selected images with the highest score, resulting in around images. With these images, we hired annotators to provide a detailed description for each image following the annotation guidelines above. Then two rounds of quality audits were conducted afterwards, with two people in each round. For the images that does not meet the evaluation criteria, we would conduct revisions. The entire labeling process took two weeks, with one week for selecting pictures and the other week to provide detailed text descriptions.
4 Experiments
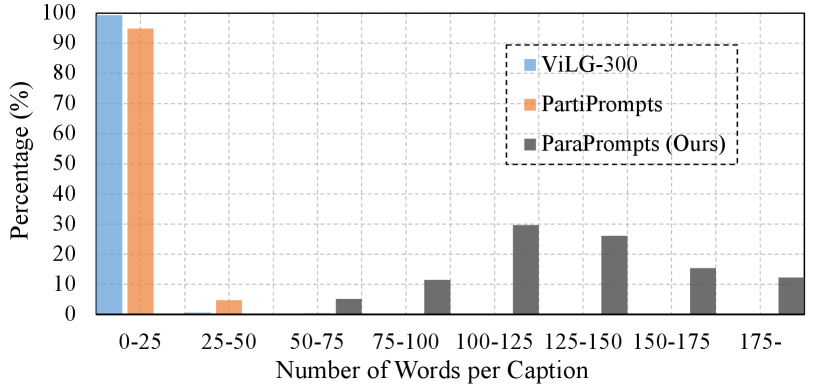
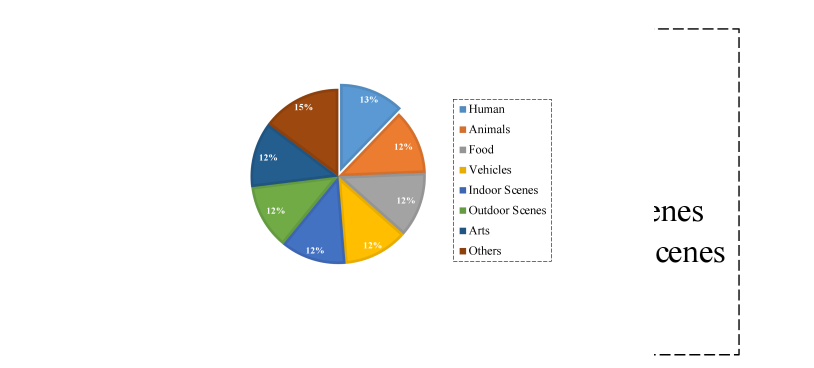
4.1 Implementation Details
Algorithm. Different from the prior works [27, 26, 4], for the first time, we used a powerful decoder-only large language model (i.e., Llama V2 [35]) as the text encoder. To learn the long-term paragraph-image alignment, we adjusted the length of extracted text tokens to tokens, enabling precise alignment for very complex and semantically rich prompts. For the diffusion model, we follow SDXL [23] and use a Unet of B parameters to learn the latent Gaussian noise. Compared to previous work, our training cost is also significantly lower, with the entire model training process only requiring V100 GPUs for days. Following LoRA [11], we apply LoRA to each layer of Llama V2 [35]. At different stages, we employ varying learning rate strategies. For Stage 1, the learning rate is set to with cosine annealing decay for a single cycle. For Stage 2, the learning rate is , and for Stage 3, it is .
Datasets and Metrics. We use Zero-shot FID-30K on MS-COCO [18] and human evaluation on ViLG-300[6] to assess our algorithm. Additionally, considering that the current test prompts focus on short text-to-image generation, ignoring the evaluation for paragraph-to-image generation, we introduced a new evaluation set of prompts called ParaPrompts, including long-text descriptions. Figure 5 illustrates the distribution comparison of prompt lengths between ParaPrompts and the previous test set. It is evident that previous prompts testing was mostly concentrated on text alignments within the range of 0-25 words, while our prompts extend to long-text alignments of words or more. Additionally, we present a new insight that longer text descriptions are more challenging, both in terms of visual appeal and text faithfulness. Relevant discussions and comparisons are provided in the supplementary materials. Figure 6 presents the distribution of prompt content, where we categorized prompts into eight scene categories to achieve a more comprehensive evaluation.
4.2 Performance Comparisons and Analysis
4.2.1 Fidelity Assessment on COCO Dataset
Zero-shot FID-30K on MS-COCO [18] is a universal evaluation method for text-image generation tasks. Table 2 presents relevant comparisons between ParaDiffusion and other existing works. Our ParaDiffusion achieved an FID score of , demonstrating similar performance to SD XL [23] and PIXART- [3]. In comparison, RAPHAEL [40] and DeepFloyd-IF [5] achieved better scores, while utilizing larger models with more parameters. Furthermore, we would like to argue that FID may not be an appropriate metric for image quality evaluation, where a higher score does not necessarily indicate better-generated images. Many studies [3, 5, 23], have demonstrated that, instead, the evaluation by human users is a more authoritative measure.
Method | Text Encoder | #Params | FID-30K | Venue/Date |
---|---|---|---|---|
DALL-E | - | 12.0B | 27.50 | Blog, Jan. 2021 |
GLIDE [21] | - | 5.0B | 12.24 | ICML’22 |
DALL-E2 [26] | CLIP [24] | 6.5B | 10.39 | arXiv, April 2022 |
PIXART- [3] | T5-XXL [25] | 0.6B | 10.65 | arXiv, Oct. 2023 |
SD XL [23] | CLIP [24] | 2.6B | - | arXiv, Jul. 2023 |
GigaGAN [12] | CLIP [24] | 0.9B | 9.09 | CVPR’23 |
SD [27] | CLIP [24] | 0.9B | 8.32 | CVPR’22 |
Imagen [30] | T5-XXL [25] | 3.0B | 7.27 | NeurIPS’22 |
ERNIE-ViLG 2.0 | CLIP [24] | 22B | 6.75 | CVPR’23 |
DeepFloyd-IF [5] | T5-XL [25] | 4.3B | 6.66 | Product, May 2023 |
RAPHAEL [40] | CLIP [24] | 3.0B | 6.61 | arXiv, May 2023 |
ParaDiffusion | Llama V2 [35] | 1.3B | 9.64 | - |
4.2.2 ViLG-300 [6]
Following the prior works [3, 6], we also conducted a User Study evaluation from the perspectives of visual appeal and text faithfulness on 300 prompts from ViLG-300 [6]. We only chose the recent models with sota performance for comparative evaluation, as involving human evaluators can be time-consuming. Figure 7 presents the related rating proportions from expert evaluators.
Visual Appeal. Our model significantly outperforms DeepFloyd-IF and SD XL-Refiner in terms of visual appeal, while both of these are considered among the best models of the past year. In comparison to PIXART-[3], our model has achieved competitive performance. We want to emphasize that our model did not specifically focus on Visual Appeal. Additionally, PIXART-[3] and our work are concurrent efforts. Therefore, we believe the performance is acceptable.
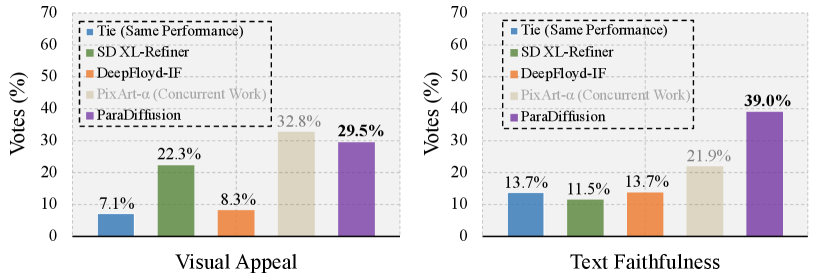
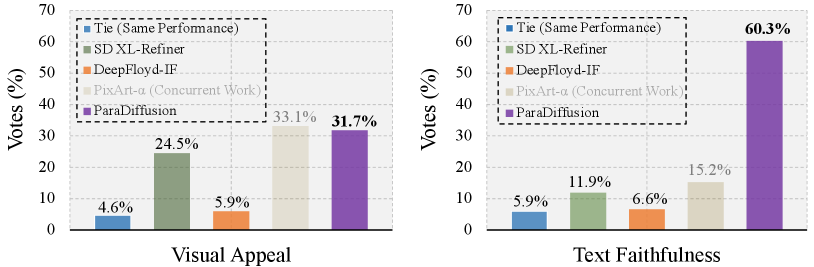
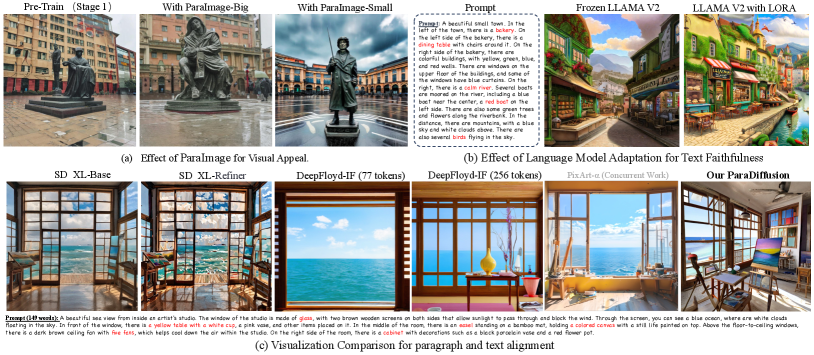
Text Faithfulness. Figure 7 (right) shows that our model achieved outstanding performance in Text Faithfulness among the four models, with a voting percentage of . ‘Tie (Same Performance)’ received a very high voting percentage. This is because ViLG-300 includes many simple prompts, leading to consistently good results and making it challenging to differentiate among them. We provide additional cases in the supplementary material to further analyze this situation.
4.2.3 ParaPrompts-400
Figure 8 presents the results on ParaPrompts-400.
Visual Appeal. Similar to ViLG-300 [6], our ParaDiffusion achieved outstanding results in terms of visual appeal on the ParaPrompts dataset, surpassing SD XL and DeepFloyd-IF and approaching PIXART- [3]. Compared to the performance on the ViLG-300 [6] dataset, there is a decrease in the voting percentage for the ‘Tie’ category. This indicates that in more challenging long-text scenarios, the performance differences among different models become more pronounced.
Text Faithfulness. As for paragraph-guided image generation setting, ParaDiffusion demonstrates a more pronounced advantage in text faithfulness, reaching , while other models achieve only around . This indicates that ParaDiffusion significantly outperforms other models in aligning long-text aspects such as object, object attributes, and object positional relationships. In the supplementary material, we provide additional visualizations and analyses to support this claim.
Methods | # Trainable Params | Win (%) | Tie (%) | Lose (%) |
---|---|---|---|---|
w/o LoRA (Base) | - | - | - | - |
w LoRA | 4.2M (0.06%) | 50.6 (+5.5) | 4.3 | 45.1 |
w LoRA | 16.7M (0.25%) | 51.1 (+5.8) | 3.6 | 45.3 |
w LoRA | 33.6M (0.51%) | 53.4 (+4.7) | 2.1 | 48.7 |
w LoRA | 67.1M (1.01%) | 51.9 (+6.6) | 2.8 | 45.3 |
Dataset | Visual Appeal | Text Faithfulness | ||
---|---|---|---|---|
Win (%) | Lose (%) | Win (%) | Lose (%) | |
w/o ParaImage (Pre-Train, Stage-1) | - | - | - | - |
w ParaImage-B (Stage-2 Stage-1) | 82.1 | 17.9 | 76.9 | 32.1 |
w ParaImage-S (Stage-3 Stage-2) | 86.2 | 13.8 | 53.1 | 46.9 |
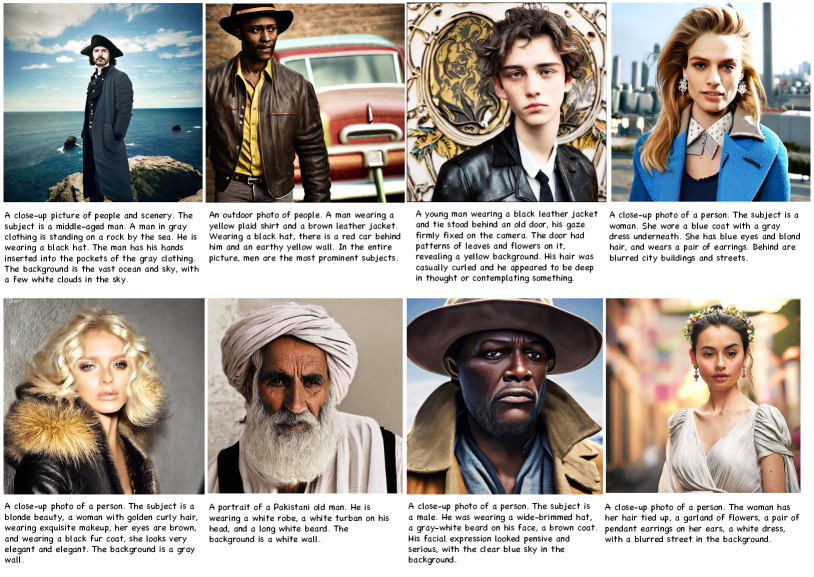
4.3 Ablation Study
4.3.1 Effect of Language Model Adaptation
Table 3 presents the ablation study for LLM Adaptation. ‘Base’ denotes directly performing paragraph-image alignment learning (Stage-2) with ParaImage-Big without fine-tuning Llama V2 using LoRA. Compared to the base model, our LLM adaptation with LoRA demonstrates an improvement of nearly in human voting rates. Another insight reveals that during the process of increasing trainable parameters from million to million, the performance appears to gain consistently. Therefore, in our final configuration, we randomly select 16.7 million trainable parameters as the ultimate setting. Figure 9 (b) presents the visual comparisons for the performance from LLM adaptation.
4.3.2 Effect of ParaImage
Table 4 presents the ablation study for the proposed dataset, ParaImage. ParaImage-Big brings significant improvements, with approximately a increase in human voting rates for both visual appeal and text faithfulness simultaneously. The smaller-scale, and high-quality set of 3k images in ParaImage-Small further enhances aesthetic aspects, achieving a remarkable around increase in human preference rates compared to that of Stage 2. Figure 9 (a) presents a visual comparison, vividly illustrating the gains in visual appeal. In this case, the prompt is ‘An exquisite sculpture in the center of a square on a rainy day.’
5 Conclusion
In this paper, we firstly present the challenges of long-text alignment in the task of text-guided image generation. Additionally, we propose an effective solution that addresses both the data and algorithmic aspects. In terms of algorithms, we introduce an information-enriched diffusion model, which explores the transfer of the long-text semantic understanding capabilities from large language models to the image generation task. For data, we construct and present a high-quality, textual rich paragraph-to-image pairs dataset, where the corresponding textual descriptions can extend up to words. The experiments demonstrate that our ParaDiffusion achieves outstanding performance in both visual appeal and text faithfulness aspects.
6 Appendix
6.1 More Visualizations
Table 10 and Table 11 provides more visualizations of ParaDiffusion for human-centric and scenery-centric domains, respectively. The visualization reveals that our ParaDiffusion can generate intricate and realistic composite images of individuals as well as picturesque scenes. Moreover, it is noteworthy that images generated through paragraph-image generation exhibit a compelling narrative quality, enriched with profound semantics. The generated images consistently feature intricate object details and demonstrate effective multi-object control.
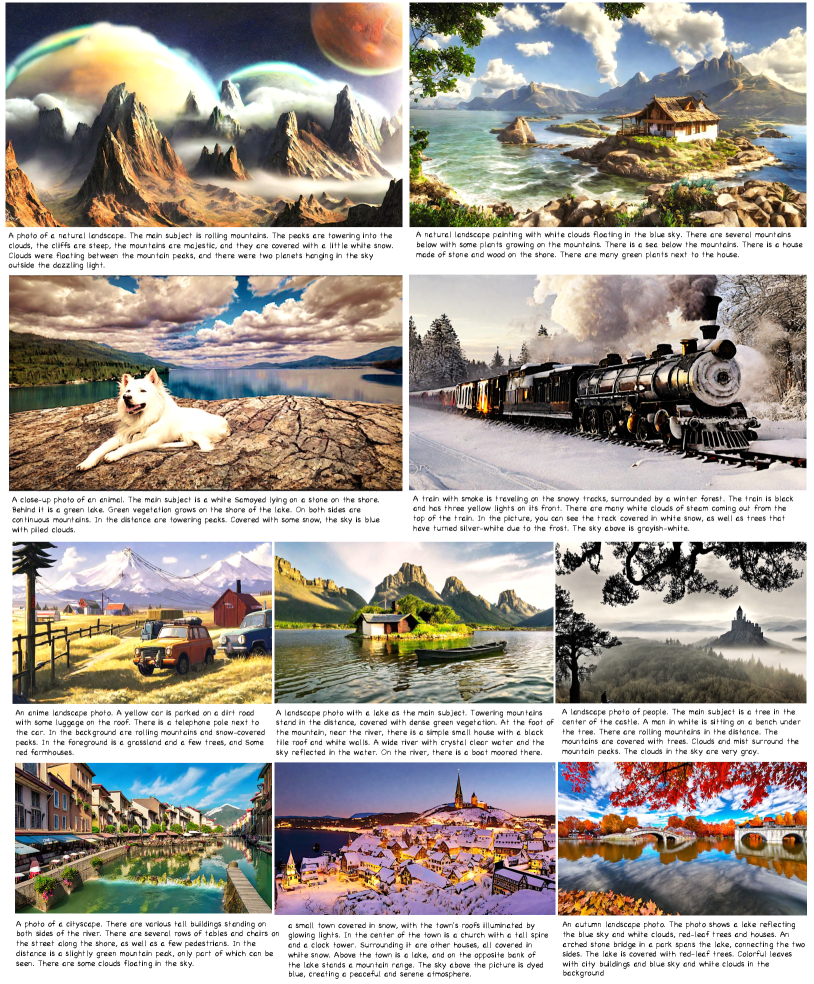
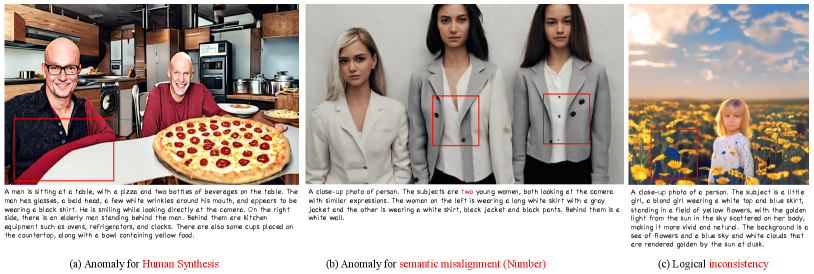
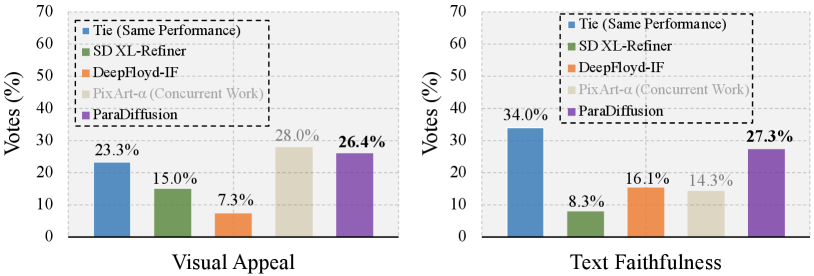
6.2 Limitations
Despite ParaDiffusion achieving excellent performance in long-text alignment and Visual Appeal, there are still some areas for improvement, such as inference speed. ParaDiffusion has not been optimized for speed, and implementing effective strategies, such as ODE solvers [20] or consistency models [33], could lead to further enhancement and optimization in inference speed. In addition, while ParaDiffusion exhibits the capability to produce images of high realism, the presence of undesirable instances persists, as depicted in Figure 12. Two predominant strategies prove effective in addressing these challenges: Firstly, at the data level, augmenting the dataset with additional high-quality images enhances diversity, contributing to further model refinement. Secondly, at the algorithmic level, the incorporation of additional constraints, such as geometric and semantic constraints, serves to imbue synthesized images with greater logical and semantic coherence.
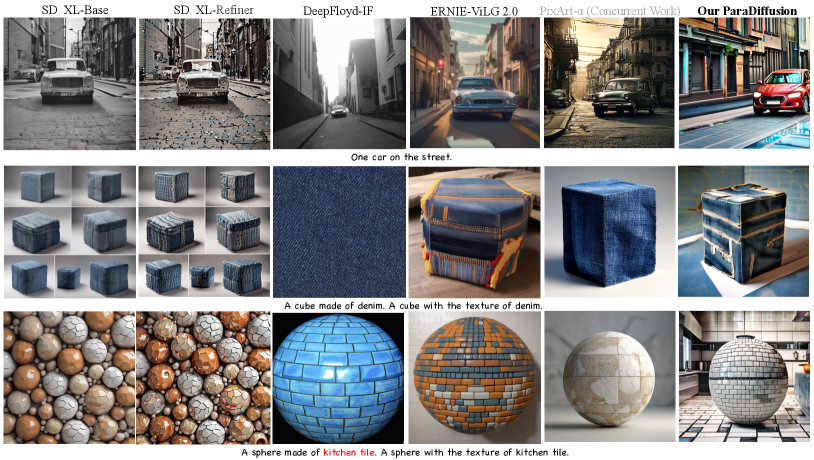
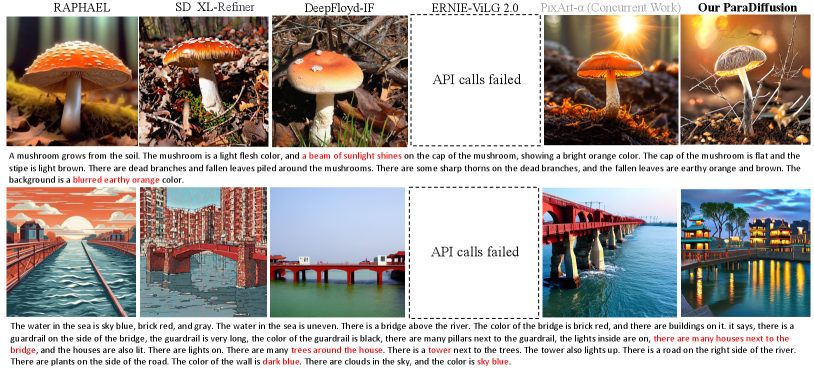
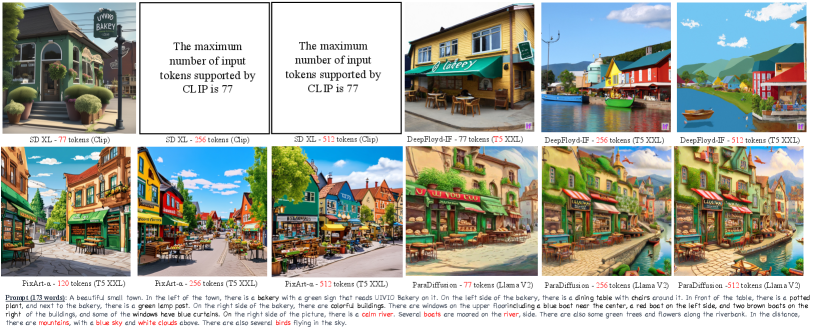
6.3 Experiments on 1,600 prompts of PartiPrompts
We also provides the related experiment results on PartiPrompts-1600, as shown in Figure 13. It can be observed that our model also achieved outstanding performance in Text Faithfulness, with a human voting rate, significantly outperforming previous models such as SD XL and DeepFloyd-IF. Additionally, our model demonstrated a competitive advantage in visual appeal, surpassing SD XL and DeepFloyd-IF, and approaching the performance of contemporaneous work PIXART-. A notable observation in ParaPrompts is the high proportion of ’Tie’ votes in human evaluations, especially for Text Faithfulness, with a voting rate of up to . This is attributed to the presence of numerous simple or abstract prompts in ParaPrompts-1600, making it challenging to provide precise voting results, such as for prompts like ‘happiness’ or ‘emotion.’
6.4 Visualization Comparison on ViLG-300 and ParaPrompts-400
To offer a more intuitive comparison, we provide visualizations comparing our model with prior works on ViLG-300 and ParaPrompts-400 datasets, as depicted in Figure 14 and Figure 15. From the perspective of visual appeal, the synthesized images produced by our ParaDiffusion align well with human aesthetics. They exhibit qualities reminiscent of photographic images in terms of lighting, contrast, scenes, and photographic composition. Concerning the alignment of long-form text, our ParaDiffusion demonstrates outstanding advantages, as illustrated in Figure 15. Previous works often struggle to precisely align each object and attribute in lengthy textual descriptions, as seen in the second row of Figure 15. Existing models frequently miss generating elements like ‘towers’ and ‘houses’, and their relative spatial relationships are flawed. In contrast, our model excels in accurately aligning the textual description with the content in the image.
6.5 More Details for ParaImage-Small
As stated in the main text, we selected 3,000 exquisite images from a pool of 650,000 images curated by LAION-Aesthetics [15], adhering to common photographic principles. The detailed Aesthetic Image Selection Rule is outlined as follows:
-
1.
The selected images will be used to annotate long-form descriptions (128-512 words, 4-10 sentences). Please assess whether the chosen images contain sufficient information (number and attributes of objects, image style) to support such lengthy textual descriptions.
-
2.
The images should not include trademarks, any text added in post-production, and should be free of any mosaic effects.
-
3.
Spatial Relationships between Multiple Objects: For images with multiple objects, there should be sufficient spatial hierarchy or positional relationships between these objects. For example, in a landscape photograph, the spatial distribution of mountains, lakes, and trees should create an interesting composition. There should be clear left-right relationships between multiple people.
-
4.
Interaction between Multiple Objects: For images with multiple objects, choose scenes that showcase the interaction between the objects. This can include dialogue between characters, interactions between animals, or other interesting associations between objects.
-
5.
Attribute of Single Object: All key details of the main subject should be clearly visible, and the subject’s attribute information should include at least three distinct aspects, including color, shape, and size. For example, in wildlife photography, the feather color, morphology, and size of an animal should be clearly visible.
-
6.
Colors, Shapes, and Sizes of Objects: Various objects in the image should showcase diversity in colors, shapes, and sizes. This contributes to creating visually engaging scenes.
-
7.
Clarity of the Story: The selected images should clearly convey a story or emotion. Annotators should pay attention to whether the image presents a clear and engaging narrative. For example, a couple walking on the street, a family portrait, or animals engaging in a conflict.
-
8.
Variety of Object Categories: A diverse set of object categories enriches the content of the image. Ensure that the image encompasses various object categories to showcase diversity. For instance, on a city street, include people, cyclists, buses, and unique architectural structures simultaneously.
Following the aforementioned rules, we instructed the annotators to rate the images on a scale of 1-5, with 5 being the highest score. Subsequently, we selected images with a score of 5 as the data source for ParaImage-Small, resulting in approximately 3k images.
6.6 Risk of Conflict between Visual Appeal and Text Faithfulness
We also explored the potential of existing architectures (e.g., SD XL, DeepFloyd-IF) for long-text alignment of text-image image generation, as shown in Figure 16. Firstly, all methods that utilize CLIP as a text encoder, such as SDXL, face limitations in supporting paragraph-image tasks due to the maximum number of input tokens supported by CLIP being . Secondly, we investigated the performance of methods using T5 XXL as a text encoder, e.g., DeepFloyd-IF [5] and PIXART- [3]. We directly adjusted the tokens limitation of these methods to accommodate longer lengths, enabling support for image generation settings involving the alignment of long-form text. With an increase in the number of tokens, the visual appeal quality of DeepFloyd-IF experiences a significant decline, becoming more cartoonish around tokens. Furthermore, its semantic alignment is unsatisfactory, with many generated objects missing, such as the table. Similarly, PIXART- fails to achieve satisfactory semantic alignment even with the maximum token limit increase, and its visual appeal also experiences a certain degree of decline. In contrast, our ParaDiffusion exhibits a more stable performance, achieving good semantic alignment with tokens and showing minimal decline in visual appeal as the token count increases.
References
- Balaji et al. [2022] Yogesh Balaji, Seungjun Nah, Xun Huang, Arash Vahdat, Jiaming Song, Karsten Kreis, Miika Aittala, Timo Aila, Samuli Laine, Bryan Catanzaro, et al. ediffi: Text-to-image diffusion models with an ensemble of expert denoisers. arXiv preprint arXiv:2211.01324, 2022.
- Chang et al. [2023] Huiwen Chang, Han Zhang, Jarred Barber, AJ Maschinot, Jose Lezama, Lu Jiang, Ming-Hsuan Yang, Kevin Murphy, William T Freeman, Michael Rubinstein, et al. Muse: Text-to-image generation via masked generative transformers. arXiv preprint arXiv:2301.00704, 2023.
- Chen et al. [2023] Junsong Chen, Jincheng Yu, Chongjian Ge, Lewei Yao, Enze Xie, Yue Wu, Zhongdao Wang, James Kwok, Ping Luo, Huchuan Lu, et al. Pixart-: Fast training of diffusion transformer for photorealistic text-to-image synthesis. arXiv preprint arXiv:2310.00426, 2023.
- Dai et al. [2023] Xiaoliang Dai, Ji Hou, Chih-Yao Ma, Sam Tsai, Jialiang Wang, Rui Wang, Peizhao Zhang, Simon Vandenhende, Xiaofang Wang, Abhimanyu Dubey, et al. Emu: Enhancing image generation models using photogenic needles in a haystack. arXiv preprint arXiv:2309.15807, 2023.
- Deepfloyd. [2023] Deepfloyd. Deepfloyd. URL https://www.deepfloyd.ai/., 2023.
- Feng et al. [2023] Zhida Feng, Zhenyu Zhang, Xintong Yu, Yewei Fang, Lanxin Li, Xuyi Chen, Yuxiang Lu, Jiaxiang Liu, Weichong Yin, Shikun Feng, et al. Ernie-vilg 2.0: Improving text-to-image diffusion model with knowledge-enhanced mixture-of-denoising-experts. In Proceedings of the IEEE/CVF Conference on Computer Vision and Pattern Recognition, pages 10135–10145, 2023.
- Gu et al. [2023] Yuchao Gu, Xintao Wang, Jay Zhangjie Wu, Yujun Shi, Yunpeng Chen, Zihan Fan, Wuyou Xiao, Rui Zhao, Shuning Chang, Weijia Wu, et al. Mix-of-show: Decentralized low-rank adaptation for multi-concept customization of diffusion models. arXiv preprint arXiv:2305.18292, 2023.
- He et al. [2023] Yefei He, Luping Liu, Jing Liu, Weijia Wu, Hong Zhou, and Bohan Zhuang. Ptqd: Accurate post-training quantization for diffusion models. arXiv preprint arXiv:2305.10657, 2023.
- Hertz et al. [2022] Amir Hertz, Ron Mokady, Jay Tenenbaum, Kfir Aberman, Yael Pritch, and Daniel Cohen-Or. Prompt-to-prompt image editing with cross attention control. arXiv preprint arXiv:2208.01626, 2022.
- Ho et al. [2020] Jonathan Ho, Ajay Jain, and Pieter Abbeel. Denoising diffusion probabilistic models. Advances in neural information processing systems, 33:6840–6851, 2020.
- Hu et al. [2021] Edward J Hu, Yelong Shen, Phillip Wallis, Zeyuan Allen-Zhu, Yuanzhi Li, Shean Wang, Lu Wang, and Weizhu Chen. Lora: Low-rank adaptation of large language models. arXiv preprint arXiv:2106.09685, 2021.
- Kang et al. [2023] Minguk Kang, Jun-Yan Zhu, Richard Zhang, Jaesik Park, Eli Shechtman, Sylvain Paris, and Taesung Park. Scaling up gans for text-to-image synthesis. In Proceedings of the IEEE/CVF Conference on Computer Vision and Pattern Recognition, pages 10124–10134, 2023.
- Kawar et al. [2023] Bahjat Kawar, Shiran Zada, Oran Lang, Omer Tov, Huiwen Chang, Tali Dekel, Inbar Mosseri, and Michal Irani. Imagic: Text-based real image editing with diffusion models. In Proceedings of the IEEE/CVF Conference on Computer Vision and Pattern Recognition, pages 6007–6017, 2023.
- Kirillov et al. [2023] Alexander Kirillov, Eric Mintun, Nikhila Ravi, Hanzi Mao, Chloe Rolland, Laura Gustafson, Tete Xiao, Spencer Whitehead, Alexander C Berg, Wan-Yen Lo, et al. Segment anything. arXiv preprint arXiv:2304.02643, 2023.
- laion [2022] laion. https://laion.ai/blog/laion-aesthetics/. blog, 2022.
- Lester et al. [2021] Brian Lester, Rami Al-Rfou, and Noah Constant. The power of scale for parameter-efficient prompt tuning. arXiv preprint arXiv:2104.08691, 2021.
- Lewis et al. [2019] Mike Lewis, Yinhan Liu, Naman Goyal, Marjan Ghazvininejad, Abdelrahman Mohamed, Omer Levy, Ves Stoyanov, and Luke Zettlemoyer. Bart: Denoising sequence-to-sequence pre-training for natural language generation, translation, and comprehension. arXiv preprint arXiv:1910.13461, 2019.
- Lin et al. [2014] Tsung-Yi Lin, Michael Maire, Serge Belongie, James Hays, Pietro Perona, Deva Ramanan, Piotr Dollár, and C Lawrence Zitnick. Microsoft coco: Common objects in context. In Computer Vision–ECCV 2014: 13th European Conference, Zurich, Switzerland, September 6-12, 2014, Proceedings, Part V 13, pages 740–755. Springer, 2014.
- Liu et al. [2023] Haotian Liu, Chunyuan Li, Qingyang Wu, and Yong Jae Lee. Visual instruction tuning. arXiv preprint arXiv:2304.08485, 2023.
- Lu et al. [2022] Cheng Lu, Yuhao Zhou, Fan Bao, Jianfei Chen, Chongxuan Li, and Jun Zhu. Dpm-solver: A fast ode solver for diffusion probabilistic model sampling in around 10 steps. Advances in Neural Information Processing Systems, 35:5775–5787, 2022.
- Nichol et al. [2021] Alex Nichol, Prafulla Dhariwal, Aditya Ramesh, Pranav Shyam, Pamela Mishkin, Bob McGrew, Ilya Sutskever, and Mark Chen. Glide: Towards photorealistic image generation and editing with text-guided diffusion models. arXiv preprint arXiv:2112.10741, 2021.
- Ouyang et al. [2022] Long Ouyang, Jeffrey Wu, Xu Jiang, Diogo Almeida, Carroll Wainwright, Pamela Mishkin, Chong Zhang, Sandhini Agarwal, Katarina Slama, Alex Ray, et al. Training language models to follow instructions with human feedback. Advances in Neural Information Processing Systems, 35:27730–27744, 2022.
- Podell et al. [2023] Dustin Podell, Zion English, Kyle Lacey, Andreas Blattmann, Tim Dockhorn, Jonas Müller, Joe Penna, and Robin Rombach. Sdxl: Improving latent diffusion models for high-resolution image synthesis. arXiv preprint arXiv:2307.01952, 2023.
- Radford et al. [2021] Alec Radford, Jong Wook Kim, Chris Hallacy, Aditya Ramesh, Gabriel Goh, Sandhini Agarwal, Girish Sastry, Amanda Askell, Pamela Mishkin, Jack Clark, et al. Learning transferable visual models from natural language supervision. In International conference on machine learning, pages 8748–8763. PMLR, 2021.
- Raffel et al. [2020] Colin Raffel, Noam Shazeer, Adam Roberts, Katherine Lee, Sharan Narang, Michael Matena, Yanqi Zhou, Wei Li, and Peter J Liu. Exploring the limits of transfer learning with a unified text-to-text transformer. The Journal of Machine Learning Research, 21(1):5485–5551, 2020.
- Ramesh et al. [2022] Aditya Ramesh, Prafulla Dhariwal, Alex Nichol, Casey Chu, and Mark Chen. Hierarchical text-conditional image generation with clip latents. arXiv preprint arXiv:2204.06125, 2022.
- Rombach et al. [2022] Robin Rombach, Andreas Blattmann, Dominik Lorenz, Patrick Esser, and Björn Ommer. High-resolution image synthesis with latent diffusion models. In Proceedings of the IEEE/CVF Conference on Computer Vision and Pattern Recognition, pages 10684–10695, 2022.
- Ronneberger et al. [2015] Olaf Ronneberger, Philipp Fischer, and Thomas Brox. U-net: Convolutional networks for biomedical image segmentation. In Medical Image Computing and Computer-Assisted Intervention–MICCAI 2015: 18th International Conference, Munich, Germany, October 5-9, 2015, Proceedings, Part III 18, pages 234–241. Springer, 2015.
- Ruiz et al. [2023] Nataniel Ruiz, Yuanzhen Li, Varun Jampani, Yael Pritch, Michael Rubinstein, and Kfir Aberman. Dreambooth: Fine tuning text-to-image diffusion models for subject-driven generation. In Proceedings of the IEEE/CVF Conference on Computer Vision and Pattern Recognition, pages 22500–22510, 2023.
- Saharia et al. [2022a] Chitwan Saharia, William Chan, Saurabh Saxena, Lala Li, Jay Whang, Emily L Denton, Kamyar Ghasemipour, Raphael Gontijo Lopes, Burcu Karagol Ayan, Tim Salimans, et al. Photorealistic text-to-image diffusion models with deep language understanding. Advances in Neural Information Processing Systems, 35:36479–36494, 2022a.
- Saharia et al. [2022b] Chitwan Saharia, Jonathan Ho, William Chan, Tim Salimans, David J Fleet, and Mohammad Norouzi. Image super-resolution via iterative refinement. IEEE Transactions on Pattern Analysis and Machine Intelligence, 45(4):4713–4726, 2022b.
- Schuhmann et al. [2022] Christoph Schuhmann, Romain Beaumont, Richard Vencu, Cade Gordon, Ross Wightman, Mehdi Cherti, Theo Coombes, Aarush Katta, Clayton Mullis, Mitchell Wortsman, et al. Laion-5b: An open large-scale dataset for training next generation image-text models. arXiv preprint arXiv:2210.08402, 2022.
- Song et al. [2023] Yang Song, Prafulla Dhariwal, Mark Chen, and Ilya Sutskever. Consistency models. 2023.
- Touvron et al. [2023a] Hugo Touvron, Thibaut Lavril, Gautier Izacard, Xavier Martinet, Marie-Anne Lachaux, Timothée Lacroix, Baptiste Rozière, Naman Goyal, Eric Hambro, Faisal Azhar, et al. Llama: Open and efficient foundation language models. arXiv preprint arXiv:2302.13971, 2023a.
- Touvron et al. [2023b] Hugo Touvron, Louis Martin, Kevin Stone, Peter Albert, Amjad Almahairi, Yasmine Babaei, Nikolay Bashlykov, Soumya Batra, Prajjwal Bhargava, Shruti Bhosale, et al. Llama 2: Open foundation and fine-tuned chat models. arXiv preprint arXiv:2307.09288, 2023b.
- Wang et al. [2022] Yizhong Wang, Yeganeh Kordi, Swaroop Mishra, Alisa Liu, Noah A Smith, Daniel Khashabi, and Hannaneh Hajishirzi. Self-instruct: Aligning language model with self generated instructions. arXiv preprint arXiv:2212.10560, 2022.
- WeihanWang et al. [2023] WeihanWang, Wenmeng Yu Qingsong Lv, Yan Wang Wenyi Hong, Ji Qi, Zhuoyi Yang Junhui Ji, Xixuan Song Lei Zhao, Xu Bin Jiazheng Xu, Yuxiao Dong Juanzi Li, and Jie Tang Ming Dingz. Cogvlm: Visual expert for large language models. arXiv preprint arXiv:5148899, 2023.
- Wu et al. [2023a] Weijia Wu, Yuzhong Zhao, Hao Chen, Yuchao Gu, Rui Zhao, Yefei He, Hong Zhou, Mike Zheng Shou, and Chunhua Shen. Datasetdm: Synthesizing data with perception annotations using diffusion models. arXiv preprint arXiv:2308.06160, 2023a.
- Wu et al. [2023b] Weijia Wu, Yuzhong Zhao, Mike Zheng Shou, Hong Zhou, and Chunhua Shen. Diffumask: Synthesizing images with pixel-level annotations for semantic segmentation using diffusion models. arXiv preprint arXiv:2303.11681, 2023b.
- Xue et al. [2023] Zeyue Xue, Guanglu Song, Qiushan Guo, Boxiao Liu, Zhuofan Zong, Yu Liu, and Ping Luo. Raphael: Text-to-image generation via large mixture of diffusion paths. arXiv preprint arXiv:2305.18295, 2023.
- Yu et al. [2022] Jiahui Yu, Yuanzhong Xu, Jing Yu Koh, Thang Luong, Gunjan Baid, Zirui Wang, Vijay Vasudevan, Alexander Ku, Yinfei Yang, Burcu Karagol Ayan, et al. Scaling autoregressive models for content-rich text-to-image generation. arXiv preprint arXiv:2206.10789, 2022.
- Zhang et al. [2023] Lvmin Zhang, Anyi Rao, and Maneesh Agrawala. Adding conditional control to text-to-image diffusion models. In Proceedings of the IEEE/CVF International Conference on Computer Vision, pages 3836–3847, 2023.
- Zhu et al. [2023] Deyao Zhu, Jun Chen, Xiaoqian Shen, Xiang Li, and Mohamed Elhoseiny. Minigpt-4: Enhancing vision-language understanding with advanced large language models. arXiv preprint arXiv:2304.10592, 2023.