OpenMatch: An Open Source Library for Neu-IR Research
Abstract.
OpenMatch is a Python-based library that serves for Neural Information Retrieval (Neu-IR) research. It provides self-contained neural and traditional IR modules, making it easy to build customized and higher-capacity IR systems. In order to develop the advantages of Neu-IR models for users, OpenMatch provides implementations of recent neural IR models, complicated experiment instructions, and advanced few-shot training methods. OpenMatch reproduces corresponding ranking results of previous work on widely-used IR benchmarks, liberating users from surplus labor in baseline reimplementation. Our OpenMatch-based solutions conduct top-ranked empirical results on various ranking tasks, such as ad hoc retrieval and conversational retrieval, illustrating the convenience of OpenMatch to facilitate building an effective IR system. The library, experimental methodologies and results of OpenMatch are all publicly available at https://github.com/thunlp/OpenMatch.
1. Introduction
With the rapid development of deep neural networks, Information Retrieval (IR) shows better performance and benefits lots of applications, such as open-domain question answering (Chen et al., 2017) and fact verification (Thorne et al., 2018). Being neural has become a new tendency for the IR community, which helps to overcome the vocabulary mismatch problem that comes from sparse retrieval models. Neu-IR models show their effectiveness in implementing both retrieval and reranking modules to build IR systems.
Toolkit | Retriever | Neural | Few-Shot | |
Sparse | Dense | Reranker | Training | |
Anserini (Yang et al., 2017) | ✔ | |||
MatchZoo (Guo et al., 2019) | ✔ | |||
OpenNIR (MacAvaney, 2020) | ✔ | ✔ | ||
Capreolus | ✔ | ✔ | ||
Pyserini (Lin et al., 2021) | ✔ | ✔ | ||
OpenMatch | ✔ | ✔ | ✔ | ✔ |
Recent research comes up with lots of IR models and has to compare with lots of baseline models, such as Neu-IR models and sparse retrieval methods. However, lots of baseline models have no standard implementations and experimental details, which forces some work to borrow empirical results from related work and consumes lots of efforts from researchers to reproduce the results of these methods, even for some feature-based learning-to-rank methods (Dai et al., 2018; Dai and Callan, 2019), becoming an obstacle for some IR researchers.
This paper proposes OpenMatch, an open-source library designed to support Neu-IR research. As shown in Table 1, with well-defined user interfaces, the OpenMatch library provides various modules to implement different ranking models, such as sparse retrievers (Lavrenko and Croft, 2017; Robertson and Zaragoza, 2009), dense retrievers (Karpukhin et al., 2020; Xiong et al., 2020) and neural rerankers (Xiong et al., 2017; Dai et al., 2018; Qiao et al., 2019), making it easy to build a tailored retrieval pipeline by choosing neural/non-neural ranking models and combining ranking features from different models. OpenMatch is built on Python and Pytorch to maintain well system encapsulation and model extensibility. Our OpenMatch library incorporates some few-shot training modules, which implement some advanced Neu-IR training strategies targeted at dealing with the data scarcity problem in lots of ranking scenarios (Hawking, 2004; Chirita et al., 2005; Arora et al., 2018; Roberts et al., 2020). The few-shot training strategies consist of two kinds of methods: leveraging domain knowledge in ranking (Liu et al., 2018); synthesizing and reweighting large-scale weak supervision signals to train Neu-IR models (Zhang et al., 2020; Sun et al., 2020).
OpenMatch also provides experimental results of various baselines on ranking benchmarks, which are widely used in different ranking scenarios, such as ad hoc retrieval (Qiao et al., 2019; Dai and Callan, 2019), evidence retrieval in question answering (Chen et al., 2017; Thorne et al., 2018) and conversational search (Qu et al., 2020; Dalton et al., 2020a). OpenMatch implements several widely-used Neu-IR models and reproduces the ranking results of corresponding work. We submit the reimplemented results to document and passage ranking tasks on the MS MARCO leaderboard111Team name: THU-MSR https://microsoft.github.io/msmarco/ to further guarantee the reproducibility with the hidden test. Besides, our OpenMatch based solutions rank first222Team name: CMT https://ir.nist.gov/covidSubmit/archive.html of the automatic group in TREC COVID Round 2, demonstrating its ability to build an effective IR pipeline system for a new ranking problem that has few training data.
2. System Overview
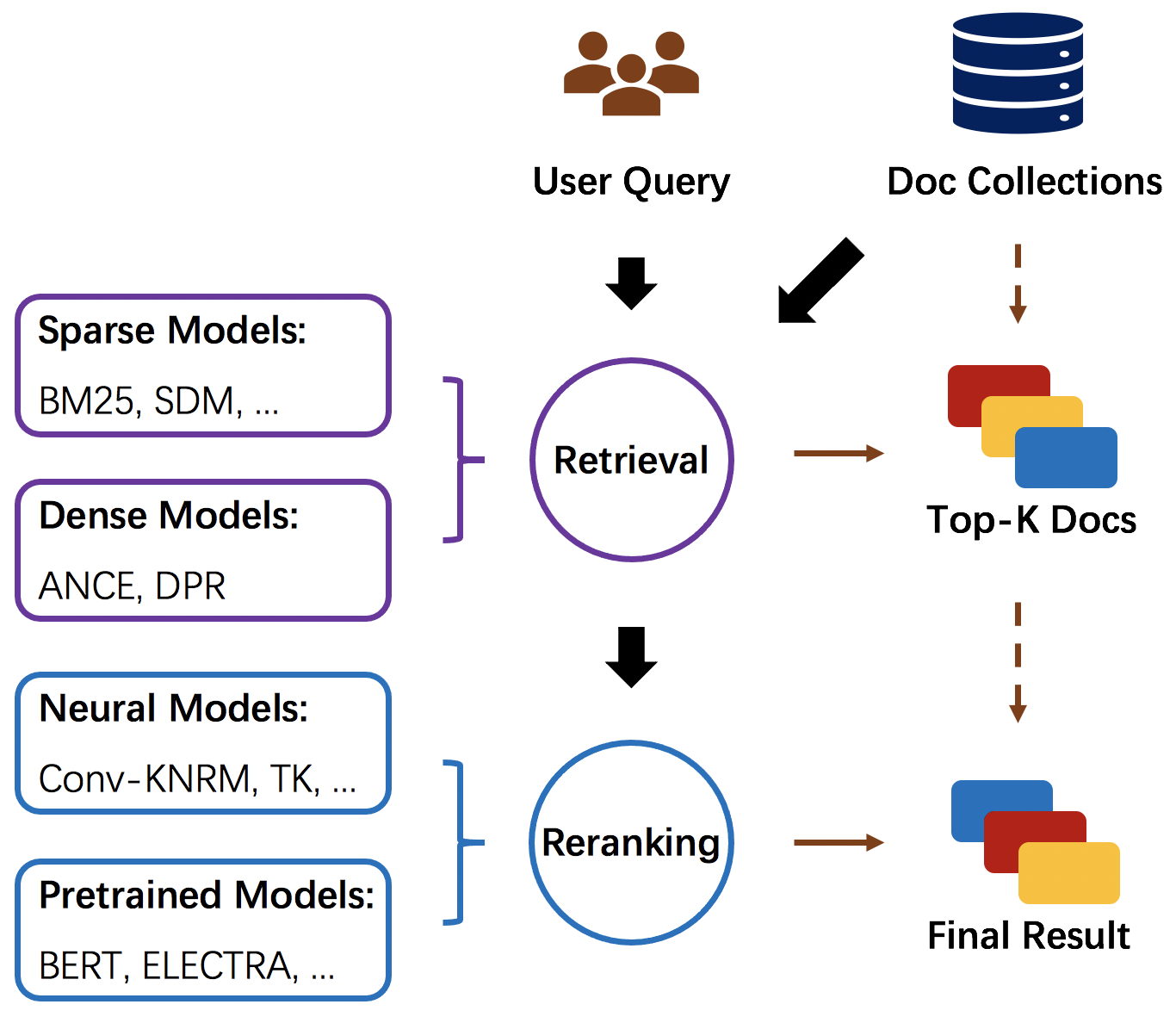
OpenMatch provides several pre-defined modules and helps users build an effective IR system with retrieval and reranking steps, as shown in Figure 1. In the retrieval stage, we first inherit Anserini (Yang et al., 2017) and ANCE (Xiong et al., 2020) to build the document index for sparse retrieval and dense retrieval to efficiently search candidate documents from a large-scale document collection. Then, for the reranking stage, OpenMatch provides various modules to extract ranking features with sparse retrieval models, dense retrieval models, Neu-IR models, and pretrained IR models. The neural modules in OpenMatch are defined to conveniently implement different Neu-IR models. Moreover, as shown in Figure 2, OpenMatch incorporates several advanced training methods to further broaden the advance of Neu-IR models in few-shot ranking scenarios. These few-shot training methods mainly focus on alleviating data scarcity in ranking scenarios by leveraging domain knowledge and data augmentation. Besides, our library also provides some modules for data preprocessing, ranking feature ensemble and automatic evaluation.
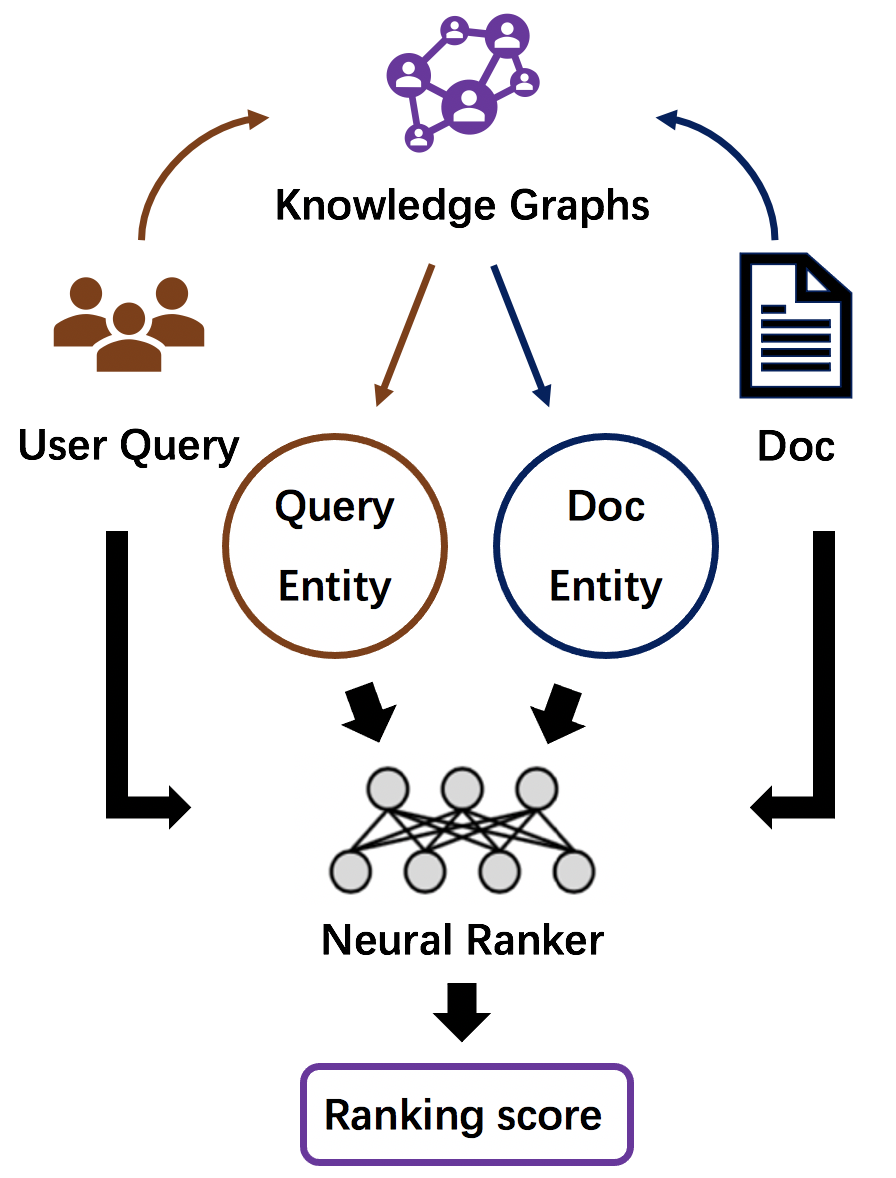
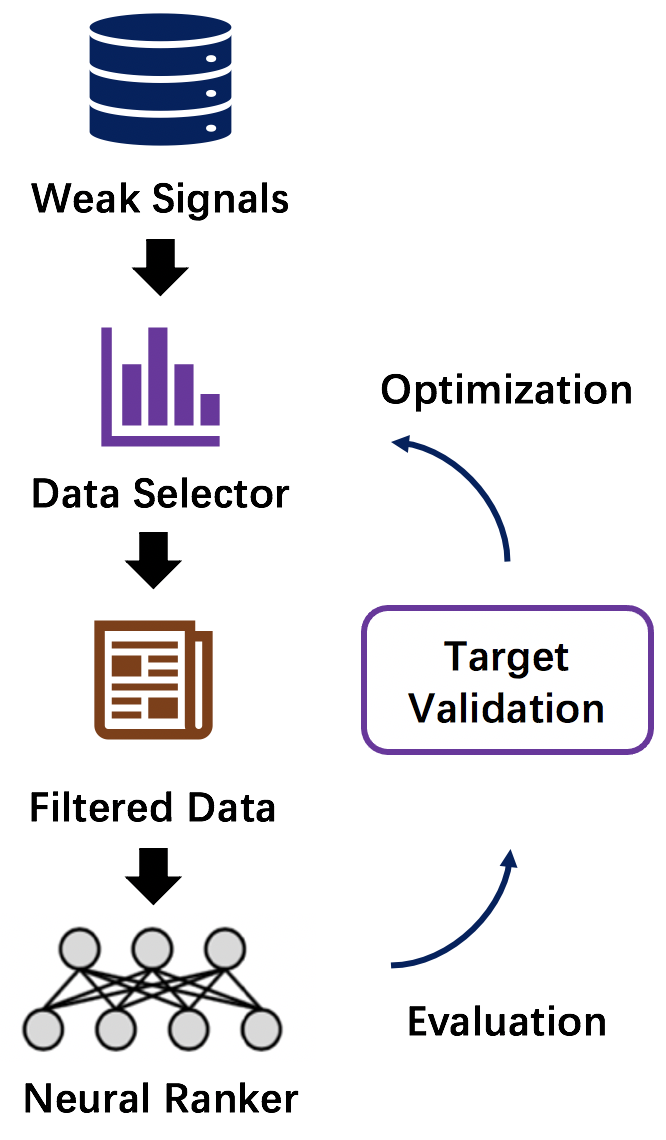
3. OpenMatch Library
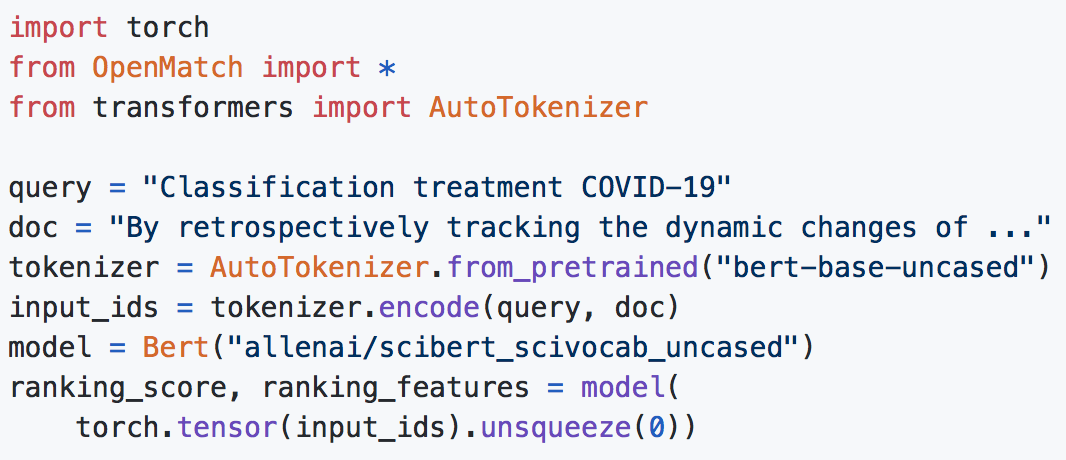
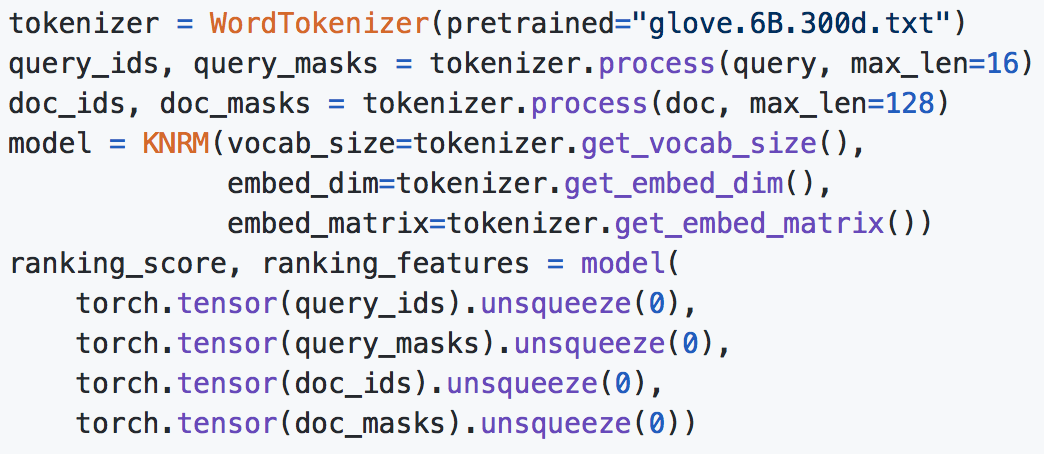
OpenMatch is built on PyTorch and provides several extensible modules in the whole IR pipeline for data processing, Neu-IR model implementation, few-shot training, model inference, and ranking performance evaluation.
3.1. Data Processing
This part presents the data processing modules in OpenMatch that can be used to deal with various data formats for ranking and develop customized classes for individual data processing, including tokenizer, dataset, and dataloader.
Tokenizer. OpenMatch follows HuggingFace’s Transformers (Wolf et al., 2019) to design the Tokenizer class for Neu-IR models, which makes it easy-to-use and extensible. The Tokenizer can be initialized from raw vocabularies and word embeddings. It maintains a token-to-id map to convert raw texts into token ids for Neu-IR models and provides two functions, stopword removal and word stemming, to process words, which are widely used in IR models. For pretrained language models, our Tokenizer module inherits the same tokenizer implementation from HuggingFace’s Transformers library.
Dataset. The Dataset class in OpenMatch is inherited from the Dataset class of PyTorch. It provides several functions to process the raw data with various formats for pair-wise training, point-wise training, and model inference. The collation function of OpenMatch further supplies an API to stack data instances into mini-batches for the Dataloader module.
Dataloader. OpenMatch also inherits the Dataloader class of PyTorch to generate batched data for Neu-IR models. It is convenient to set batch size, shuffle flags, and the number of workers in loading data. With the Dataloader module of PyTorch, OpenMatch can be easily extended to model inference process, single-GPU training process and multi-GPUs training process.
3.2. Neural Modules
This part presents neural modules in OpenMatch, including Embedder, Attention, Encoder, and Matcher. All these flexible and extensible modules help to implement different Neu-IR models.
Embedder. The Embedder class mainly focuses on mapping tokens to dense representations. It feeds the token ids as inputs and returns a sequence of token embeddings for the following neural modules. The Embedder module can choose different Tokenizer and the token representations can be initialized with randomly initialized word embeddings, word2vec-based word embeddings, or subword embeddings from deep pretrained language models.
Attention. The Attention mechanism has been widely used in various deep learning tasks and is regarded as a crucial component in Neu-IR models. OpenMatch provides two kinds of attention modules in its library, including scaled dot-product attention and multi-head self-attention (Vaswani et al., 2017).
Encoder. The Encoder class provides various modules in deep learning to encode queries and documents, which consists of the feed-forward encoder, the CNN-based encoder, and multi-head self-attention based encoder. These Encoder modules further encode word representations to get their contextual representations. Users can set the dimension size, kernel size, and the number of the convolution kernel, the number of self-attention heads, and many other parameters in Encoder modules.
Matcher. The Matcher class is designed to measure the similarity between queries and documents by conducting interactions between them, which are used in previous Neu-IR models (MacAvaney et al., 2019; Dai et al., 2018; Xiong et al., 2017; Hofstätter et al., 2020). Following widely-used IR models (Xiong et al., 2017; Dai et al., 2018), OpenMatch implements a KernelMatcher module that employs RBF kernels (Xiong et al., 2017) to establish interactions between queries and documents and estimate their similarities with the term-term based soft matching.
3.3. Few-Shot Training
In reality, many ranking scenarios are few-shot and lack training data, especially for some specific domains, such as biomedical and legal search. Thus, broadening the benefits of deep neural networks to these few-shot scenarios is a standing challenge in information retrieval (Roberts et al., 2020; Yang et al., 2019). OpenMatch consists of two kinds of methods for few-shot IR tasks to alleviate data scarcity: using domain knowledge graphs and training Neu-IR models with large-scale selected weak supervision data. These methods incorporate human knowledge and massive relevance labels to better learn the semantics of queries and documents, which significantly alleviate the data dependency in few-shot ranking scenarios (Zhang et al., 2020; Sun et al., 2020).
Domain Knowledge Enhancement. We follow the previous knowledge guided Neu-IR model EDRM (Liu et al., 2018) and aim to incorporate the semantics from domain knowledge graphs to improve ranking performance in few-shot IR scenarios. EDRM comes up with enriched entity representations with the learned entity representations (Bordes et al., 2013), entity types and entity descriptions. OpenMatch leverages these technologies to enhance the semantic representations of queries and documents, and conduct better interactions between queries and documents for ranking.
Data Augmentation. Recent work (Zhang et al., 2020; Sun et al., 2020) provides several ways to augment training data, such as using anchor-document to approximate query-document relevance (Zhang et al., 2020) and generating queries to synthesize relevance labels (Sun et al., 2020). To further boost model performance, they propose different strategies to select or reweight weak supervision data according to the performance of Neu-IR models on the target data. OpenMatch borrows these methods to guarantee the advance of Neu-IR models in few-shot scenarios.
3.4. Training Evaluation
OpenMatch also provides the training, inference, and evaluation scripts for Neu-IR models. The detailed experimental instructions and results are all provided in OpenMatch, facilitating users and researchers to quickly start and easily reproduce the ranking results of different IR systems with OpenMatch.
Training. OpenMatch provides a common training framework for Neu-IR models. We define two widely-used learning-to-rank functions for users to optimize Neu-IR models, including point-wise training and pair-wise training.
Evaluation. We inherit the TREC evaluation tool, pytrec_eval333https://github.com/cvangysel/pytrec_eval, to provide various standard metrics, such as NDCG and MAP. Besides, we also implement another widely-used evaluation metric MRR in OpenMatch. All above evaluation metrics are encapsulated in OpenMatch’s Metric class. Users can evaluate their IR models with only one line of python command.
4. Model Hub
This section presents the model hub of OpenMatch. These models can be divided into retrieval models and reranking models according to their functions. As shown in Table 2, OpenMatch provides the implementation and detailed instructions of widely-used Neu-IR models for users. With well-defined model library, OpenMatch helps users quickly establish a Neu-IR model in their experiments, as shown in Figure 3.
Components | Models |
---|---|
Sparse Retriever | Boolean AND/OR (Salton et al., 1983), LM (Ponte and Croft, 1998), BM25 (Robertson et al., 1995), SDM (Metzler and Croft, 2005), TF-IDF (Salton and Buckley, 1988), Cosine Similarity, Coordinate match, Dirichlet LM … |
Dense Retriever | DPR (Karpukhin et al., 2020), ANCE (Xiong et al., 2020), ConvDR (Yu et al., 2021) |
Neural Ranker | K-NRM (Xiong et al., 2017), Conv-KNRM (Dai et al., 2018), TK (Hofstätter et al., 2020), BERT (Devlin et al., 2018), RoBERTa (Liu et al., 2019), ELECTRA (Clark et al., 2020) … |
LeToR | Coordinate Ascent (Metzler and Croft, 2007), RankNet (Burges et al., 2005), LambdaMART (Wu et al., 2010), Random Forests (Cutler et al., 2012) … |
Retrieval. Given the user query and a large-scale document collection, retrieval models are used to select a subset from the document collection according to the given query. OpenMatch consists of two kinds of retrieval models – sparse models and dense models. The sparse models usually use discrete bag-of-word matching, such as BM25 (Robertson et al., 1995) and SDM (Metzler and Croft, 2007). Lots of previous work incorporates different sparse retrieval features with learning-to-rank methods to implement a strong baseline (Xiong et al., 2017; Dai et al., 2018; Dai and Callan, 2019). In OpenMatch, we provide twenty sparse retriever methods to reproduce these baselines.
Recently, dense retrievers provide an opportunity to conduct the semantic matching supported by pretrained language models, such as DPR (Karpukhin et al., 2020) and ANCE (Xiong et al., 2020). Moreover, to satisfy more complicated information needs with interactions between retrieval systems and users, OpenMatch also incorporates the conversational dense retriever (ConvDR) (Yu et al., 2021), which inherits the document dense representation learned in ad hoc search and map conversational queries in the embedding space for retrieval. OpenMatch also provides APIs to calculate ranking scores with them.
Reranking. Reranking models in OpenMatch consist of Neu-IR models, pretrained IR models and learning-to-rank models, and aim to provide more accurate ranking results. OpenMatch implements several typical and previously state-of-the-art Neu-IR models with its neural modules. For example, K-NRM (Xiong et al., 2017) can be built with Embedder and KernelMatcher modules. Conv-KNRM (Dai et al., 2018) encodes queries and documents as n-grams representations with convolution layers and can be implemented with Embedder, Conv1DEncoder, and KernelMatcher modules. TK (Hofstätter et al., 2020) uses multi-head attention layers as the encoder and can be implemented with Embedder, TransformerEncoder, and KernelMatcher modules. For pretrained Neu-IR models, we also inherit the pretrained language models from Huggingface’s Transformers444https://github.com/huggingface/transformers to implement reranking models. Several pretrained models can be used, such as BERT (Devlin et al., 2018), ELECTRA (Clark et al., 2020), and RoBERTa (Liu et al., 2019). OpenMatch brings these latest pretrained language models from Huggingface’s Transformers to Neu-IR models under the unified training framework. Besides, OpenMatch adopts RankLib555https://sourceforge.net/p/lemur/wiki/RankLib/ to provide various learning-to-rank methods, such as RankNet (Burges et al., 2005), Coordinate Ascent (Metzler and Croft, 2007), LambdaMART (Wu et al., 2010), and Random Forests (Cutler et al., 2012). These learning-to-rank methods are usually utilized in previous work (Dai and Callan, 2019; Zhang et al., 2020; Sun et al., 2020) and aim to combine the ranking features from different IR models of both retrieval and reranking for further improvement.
Ranking Results. We also use our OpenMatch library to reimplement different Neu-IR models and conduct ranking results on several benchmarks, as shown in Table 3. Six datasets, ClueWeb09 (Callan et al., 2009), Robust04 (Kwok et al., 2004), TREC COVID (Wang et al., 2020), MS MARCO (Nguyen et al., 2016), TREC CAsT-19 (Dalton et al., 2020b) and TREC CAsT-20 (Dalton et al., 2020a), are used in our experiments. With OpenMatch, we reproduce corresponding results of previous work on different ranking benchmarks.
Models | Benchmarks | |||
Ad hoc | TREC COVID | MS MARCO | CAsT | |
RankSVM (Dai and Callan, 2019) | ✔ | ✔ | ||
Coor-Ascent (Dai and Callan, 2019) | ✔ | ✔ | ||
K-NRM (Xiong et al., 2017) | ✔ | |||
ConvKNRM (Dai et al., 2018; Qiao et al., 2019) | ✔ | ✔ | ||
EDRM (Liu et al., 2018) | ✔ | |||
ReInfoSelect (Zhang et al., 2020) | ✔ | ✔ | ||
MetaAdaptRank (Sun et al., 2020) | ✔ | ✔ | ||
BM25 w. BERT (Qiao et al., 2019; Dai and Callan, 2019; Yu et al., 2020) | ✔ | ✔ | ✔ | ✔ |
ANCE w. BERT (Xiong et al., 2020; Yu et al., 2021) | ✔ | ✔ |
5. Related Work
Information Retrieval (IR) systems usually employ two-step pipelines to search related documents for user queries, which consist of retrieval and reranking. The IR community has a long history of building open-source toolkits for researchers. Anserini (Yang et al., 2017) is built on Lucene and aims to provide classical sparse retrieval methods that efficiently calculate query-document relevance according to the discrete term matching, such as BM25 (Robertson et al., 1995) and SDM (Metzler and Croft, 2005). Pyserini (Lin et al., 2021) also focuses on providing effective first-stage retrieval with spare retrieval and dense retrieval. MatchZoo (Guo et al., 2019) mainly focuses on conducting various neural text matching models beyond IR tasks (Guo et al., 2019), which can be used in the reranking stage. Other toolkits, OpenNIR (MacAvaney, 2020) and Capreolus666https://github.com/capreolus-ir/capreolus, further help to build a whole IR pipeline with sparse retrieval and neural model based reranking. Some widely used Neu-IR models are incorporated, such as K-NRM (Xiong et al., 2017), Conv-KNRM (Dai et al., 2018), CEDR (MacAvaney et al., 2019), and BERT (Devlin et al., 2018). However, these toolkits mainly focus on the architectures and variety of Neu-IR models rather than the problems that Neu-IR research usually faces, such as baseline implementation, model optimization and few-shot training. OpenMatch implements widely-used Neu-IR models, provides reproducible ranking results and advanced few-shot training methods, benefiting the Neu-IR research.
6. Conclusion
With the development of deep neural networks, Neu-IR models have attracted lots of attention from the IR community. In practice, the performance of Neu-IR models determined by the model implementation, experimental details and training strategies. To help researchers implement standard Neu-IR models, OpenMatch provides different modules severed for data preprocessing, model building, Neu-IR model training, ranking feature ensemble and ranking performance evaluation. Using our OpenMatch, researchers can implement different neural/non-neural models for retrieval and reranking and establish tailored IR pipelines for experiments. Besides, OpenMatch guarantees researchers to reproduce ranking results of previous work by providing reproducible ranking results, experimental methodology and model implementation.
7. Acknowledgements
We thank Si Sun, Shi Yu, Yizhi Li and Aowei Lu as the contributors of OpenMatch. Part of this work is supported by the National Key Research and Development Program of China (No. 2020AAA0106501) and Beijing Academy of Artificial Intelligence (BAAI).
References
- (1)
- Arora et al. (2018) Piyush Arora, Murhaf Hossari, Alfredo Maldonado, Clare Conran, and Gareth JF Jones. 2018. Challenges in the development of effective systems for professional legal search. In Joint Proceedings of the First International Workshop on Professional Search (ProfS2018); the Second Workshop on Knowledge Graphs and Semantics for Text Retrieval, Analysis, and Understanding (KG4IR); and the International Workshop on Data Search (DATA:SEARCH’18) Co-located with (ACM SIGIR 2018), Ann Arbor, Michigan, USA, July 12, 2018.
- Bordes et al. (2013) Antoine Bordes, Nicolas Usunier, Alberto García-Durán, J. Weston, and Oksana Yakhnenko. 2013. Translating Embeddings for Modeling Multi-relational Data. In NIPS.
- Burges et al. (2005) Chris Burges, Tal Shaked, Erin Renshaw, Ari Lazier, Matt Deeds, Nicole Hamilton, and Greg Hullender. 2005. Learning to rank using gradient descent. In Proceedings of ICML. 89–96.
- Callan et al. (2009) Jamie Callan, Mark Hoy, Changkuk Yoo, and Le Zhao. 2009. Clueweb09 data set.
- Chen et al. (2017) Danqi Chen, Adam Fisch, Jason Weston, and Antoine Bordes. 2017. Reading Wikipedia to Answer Open-Domain Questions. In Proceedings of ACL. 1870–1879. https://www.aclweb.org/anthology/P17-1171
- Chirita et al. (2005) Paul Alexandru Chirita, Wolfgang Nejdl, Raluca Paiu, and Christian Kohlschütter. 2005. Using ODP metadata to personalize search. In Proceedings of SIGIR. 178–185.
- Clark et al. (2020) Kevin Clark, Minh-Thang Luong, Quoc V Le, and Christopher D Manning. 2020. Electra: Pre-training text encoders as discriminators rather than generators. arXiv preprint arXiv:2003.10555 (2020).
- Cutler et al. (2012) Adele Cutler, D Richard Cutler, and John R Stevens. 2012. Random forests. In Ensemble machine learning. Springer, 157–175.
- Dai and Callan (2019) Zhuyun Dai and Jamie Callan. 2019. Deeper text understanding for IR with contextual neural language modeling. In Proceedings of SIGIR. 985–988.
- Dai et al. (2018) Zhuyun Dai, Chenyan Xiong, Jamie Callan, and Zhiyuan Liu. 2018. Convolutional neural networks for soft-matching n-grams in ad-hoc search. In Proceedings of WSDM. 126–134.
- Dalton et al. (2020a) Jeffrey Dalton, Chenyan Xiong, and Jamie Callan. 2020a. CAsT 2020: The Conversational Assistance Track Overview. (2020).
- Dalton et al. (2020b) Jeffrey Dalton, Chenyan Xiong, Vaibhav Kumar, and Jamie Callan. 2020b. Cast-19: A dataset for Conversational Information Seeking. In Proceedings of SIGIR. 1985–1988.
- Devlin et al. (2018) Jacob Devlin, Ming-Wei Chang, Kenton Lee, and Kristina Toutanova. 2018. Bert: Pre-training of deep bidirectional transformers for language understanding. arXiv preprint arXiv:1810.04805 (2018).
- Guo et al. (2019) Jiafeng Guo, Yixing Fan, Xiang Ji, and Xueqi Cheng. 2019. MatchZoo: a learning, practicing, and developing system for neural text matching. In Proceedings of SIGIR. 1297–1300.
- Hawking (2004) David Hawking. 2004. Challenges in Enterprise Search.. In Proceedings of ADC. 15–24.
- Hofstätter et al. (2020) Sebastian Hofstätter, Markus Zlabinger, and Allan Hanbury. 2020. Interpretable & time-budget-constrained contextualization for re-ranking. arXiv preprint arXiv:2002.01854 (2020).
- Karpukhin et al. (2020) Vladimir Karpukhin, Barlas Oğuz, Sewon Min, Ledell Wu, Sergey Edunov, Danqi Chen, and Wen-tau Yih. 2020. Dense Passage Retrieval for Open-Domain Question Answering. arXiv preprint arXiv:2004.04906 (2020).
- Kwok et al. (2004) Kui-Lam Kwok, Laszlo Grunfeld, HL Sun, Peter Deng, and N Dinstl. 2004. TREC 2004 Robust Track Experiments Using PIRCS.. In Proceedings of TREC.
- Lavrenko and Croft (2017) Victor Lavrenko and W. Bruce Croft. 2017. Relevance-Based Language Models. SIGIR Forum 51, 2 (2017), 260–267.
- Lin et al. (2021) Jimmy Lin, Xueguang Ma, Sheng-Chieh Lin, Jheng-Hong Yang, Ronak Pradeep, and Rodrigo Nogueira. 2021. Pyserini: An Easy-to-Use Python Toolkit to Support Replicable IR Research with Sparse and Dense Representations. arXiv preprint arXiv:2102.10073 (2021).
- Liu et al. (2019) Yinhan Liu, Myle Ott, Naman Goyal, Jingfei Du, Mandar Joshi, Danqi Chen, Omer Levy, Mike Lewis, Luke Zettlemoyer, and Veselin Stoyanov. 2019. Roberta: A robustly optimized bert pretraining approach. arXiv preprint arXiv:1907.11692 (2019).
- Liu et al. (2018) Zheng-Hao Liu, Chenyan Xiong, Maosong Sun, and Zhiyuan Liu. 2018. Entity-Duet Neural Ranking: Understanding the Role of Knowledge Graph Semantics in Neural Information Retrieval. In Proceedings of ACL. 2395–2405.
- MacAvaney (2020) Sean MacAvaney. 2020. OpenNIR: A Complete Neural Ad-Hoc Ranking Pipeline. In Proceedings of WSDM. 845–848.
- MacAvaney et al. (2019) Sean MacAvaney, Andrew Yates, Arman Cohan, and Nazli Goharian. 2019. CEDR: Contextualized embeddings for document ranking. In Proceedings of SIGIR. 1101–1104.
- Metzler and Croft (2005) Donald Metzler and W Bruce Croft. 2005. A Markov random field model for term dependencies. In Proceedings of SIGIR. 472–479.
- Metzler and Croft (2007) Donald Metzler and W Bruce Croft. 2007. Linear feature-based models for information retrieval. Information Retrieval 10, 3 (2007), 257–274.
- Nguyen et al. (2016) Tri Nguyen, Mir Rosenberg, Xia Song, Jianfeng Gao, Saurabh Tiwary, Rangan Majumder, and Li Deng. 2016. MS MARCO: A human generated machine reading comprehension dataset. In CoCo@ NIPS.
- Ponte and Croft (1998) Jay M Ponte and W Bruce Croft. 1998. A language modeling approach to information retrieval. In Proceedings of SIGIR. 275–281.
- Qiao et al. (2019) Yifan Qiao, Chenyan Xiong, Zheng-Hao Liu, and Zhiyuan Liu. 2019. Understanding the Behaviors of BERT in Ranking. CoRR abs/1904.07531 (2019).
- Qu et al. (2020) Chen Qu, Liu Yang, Cen Chen, Minghui Qiu, W Bruce Croft, and Mohit Iyyer. 2020. Open-Retrieval Conversational Question Answering. arXiv preprint arXiv:2005.11364 (2020).
- Roberts et al. (2020) Kirk Roberts, Tasmeer Alam, Steven Bedrick, Dina Demner-Fushman, Kyle Lo, Ian Soboroff, Ellen Voorhees, Lucy Lu Wang, and William R Hersh. 2020. TREC-COVID: Rationale and Structure of an Information Retrieval Shared Task for COVID-19. Journal of the American Medical Informatics Association (2020).
- Robertson and Zaragoza (2009) Stephen Robertson and Hugo Zaragoza. 2009. The probabilistic relevance framework: BM25 and beyond. Now Publishers Inc.
- Robertson et al. (1995) Stephen E Robertson, Steve Walker, Susan Jones, Micheline M Hancock-Beaulieu, Mike Gatford, et al. 1995. Okapi at TREC-3. Nist Special Publication Sp 109 (1995), 109.
- Salton and Buckley (1988) Gerard Salton and Christopher Buckley. 1988. Term-weighting approaches in automatic text retrieval. Information processing & management 24, 5 (1988), 513–523.
- Salton et al. (1983) Gerard Salton, Edward A Fox, and Harry Wu. 1983. Extended Boolean information retrieval. Commun. ACM 26, 11 (1983), 1022–1036.
- Sun et al. (2020) Si Sun, Yingzhuo Qian, Zhenghao Liu, Chenyan Xiong, Kaitao Zhang, Jie Bao, Zhiyuan Liu, and Paul Bennett. 2020. Meta Adaptive Neural Ranking with Contrastive Synthetic Supervision. arXiv preprint arXiv:2012.14862 (2020).
- Thorne et al. (2018) James Thorne, Andreas Vlachos, Oana Cocarascu, Christos Christodoulopoulos, and Arpit Mittal. 2018. The Fact Extraction and VERification (FEVER) Shared Task. In Proceedings of the First Workshop on Fact Extraction and VERification (FEVER). 1–9. https://www.aclweb.org/anthology/W18-5501/
- Vaswani et al. (2017) Ashish Vaswani, Noam Shazeer, Niki Parmar, Jakob Uszkoreit, Llion Jones, Aidan N Gomez, Łukasz Kaiser, and Illia Polosukhin. 2017. Attention is all you need. In Advances in neural information processing systems. 5998–6008.
- Wang et al. (2020) Lucy Lu Wang, Kyle Lo, Yoganand Chandrasekhar, Russell Reas, Jiangjiang Yang, Darrin Eide, Kathryn Funk, Rodney Kinney, Ziyang Liu, William Merrill, et al. 2020. CORD-19: The Covid-19 Open Research Dataset. (2020).
- Wolf et al. (2019) Thomas Wolf, Lysandre Debut, Victor Sanh, Julien Chaumond, Clement Delangue, Anthony Moi, Pierric Cistac, Tim Rault, R’emi Louf, Morgan Funtowicz, and Jamie Brew. 2019. HuggingFace’s Transformers: State-of-the-art Natural Language Processing. ArXiv abs/1910.03771 (2019).
- Wu et al. (2010) Qiang Wu, Christopher JC Burges, Krysta M Svore, and Jianfeng Gao. 2010. Adapting boosting for information retrieval measures. Information Retrieval 13, 3 (2010), 254–270.
- Xiong et al. (2017) Chenyan Xiong, Zhuyun Dai, Jamie Callan, Zhiyuan Liu, and Russell Power. 2017. End-to-end neural ad-hoc ranking with kernel pooling. In Proceedings of SIGIR. 55–64.
- Xiong et al. (2020) Lee Xiong, Chenyan Xiong, Ye Li, Kwok-Fung Tang, Jialin Liu, Paul Bennett, Junaid Ahmed, and Arnold Overwijk. 2020. Approximate Nearest Neighbor Negative Contrastive Learning for Dense Text Retrieval. arXiv preprint arXiv:2007.00808 (2020).
- Yang et al. (2017) Peilin Yang, Hui Fang, and Jimmy Lin. 2017. Anserini: Enabling the use of Lucene for information retrieval research. In Proceedings of SIGIR. 1253–1256.
- Yang et al. (2019) Wei Yang, Kuang Lu, Peilin Yang, and Jimmy Lin. 2019. Critically Examining the ”Neural Hype”: Weak Baselines and the Additivity of Effectiveness Gains from Neural Ranking Models. In Proceedings of SIGIR. 1129–1132.
- Yu et al. (2020) Shi Yu, Jiahua Liu, Jingqin Yang, Chenyan Xiong, Paul Bennett, Jianfeng Gao, and Zhiyuan Liu. 2020. Few-Shot Generative Conversational Query Rewriting. In Proceedings of the 43rd International ACM SIGIR Conference on Research and Development in Information Retrieval. 1933–1936.
- Yu et al. (2021) Shi Yu, Zhenghao Liu, Chenyan Xiong, Tao Feng, and Zhiyuan Liu. 2021. Few-Shot Conversational Dense Retrieval. In Proceedings of SIGIR.
- Zhang et al. (2020) Kaitao Zhang, Chenyan Xiong, Zhenghao Liu, and Zhiyuan Liu. 2020. Selective Weak Supervision for Neural Information Retrieval. In Proceedings of WWW. 474–485.