On Releasing Annotator-Level Labels and Information in Datasets
Abstract
A common practice in building NLP datasets, especially using crowd-sourced annotations, involves obtaining multiple annotator judgements on the same data instances, which are then flattened to produce a single “ground truth” label or score, through majority voting, averaging, or adjudication. While these approaches may be appropriate in certain annotation tasks, such aggregations overlook the socially constructed nature of human perceptions that annotations for relatively more subjective tasks are meant to capture. In particular, systematic disagreements between annotators owing to their socio-cultural backgrounds and/or lived experiences are often obfuscated through such aggregations. In this paper, we empirically demonstrate that label aggregation may introduce representational biases of individual and group perspectives. Based on this finding, we propose a set of recommendations for increased utility and transparency of datasets for downstream use cases.
1 Introduction
Obtaining multiple annotator judgements on the same data instances is a common practice in NLP in order to improve the quality of final labels Snow et al. (2008); Nowak and Rüger (2010). Cases of disagreement between annotations are often resolved through majority voting, averaging, or adjudication in order to derive a single “ground truth”, often with the aim of training supervised machine learning models. However, in relatively subjective tasks such as sentiment analysis or offensiveness detection, there often exists no single “right” answer (Alm, 2011). Enforcing such a single ground truth in such tasks will sacrifice valuable nuances about the task that are embedded in annotators’ assessments of the stimuli, especially their disagreements Aroyo and Welty (2013).
Annotators’ socio-demographic factors, moral values, and lived experiences often influence their interpretations of language, especially in subjective tasks such as identifying political stances (Luo et al., 2020), sentiment Díaz et al. (2018), and online abuse Waseem (2016); Patton et al. (2019). For instance, feminist and anti-racist activists systematically disagree with crowd workers in their hate speech annotations Waseem (2016). Similarly, annotators’ political affiliation is shown to correlate with how they annotate the neutrality of political stances (Luo et al., 2020). A potential adverse effect of majority voting in such cases is that it may sideline minority perspectives in data.
In this paper, we analyze annotated data for eight different tasks across three different datasets to study the impact of majority voting as an aggregation approach. We answer two questions:
-
•
Q1: Does aggregated data uniformly capture all annotators’ perspectives, when available?
-
•
Q2: Does aggregated data reflect certain socio-demographic groups’ perspectives more so than others?
Our analysis demonstrates that in the annotations for many tasks, the aggregated majority vote does not uniformly reflect the perspectives of all annotators in the annotator pool. For many tasks in our analysis, a significant proportion of the annotators had very low agreement scores (0 to 0.4) with the majority vote label. While certain individual annotator’s labels may have low agreement with the majority label due to valid/expected reasons (e.g., if they produced noisy labels), we further show that these agreement scores may vary significantly across different socio-demographic groups that annotators identify with. This finding has important fairness implications, as it demonstrates how the aggregation step can sometimes cause the final dataset to under-represent certain groups’ perspectives.
Meaningfully addressing such issues in multiply-annotated datasets requires understanding and accounting for systematic disagreements between annotators. However, most annotated datasets often only release the aggregated labels, without any annotator-level information. We argue that dataset developers should consider including annotator-level labels as well as annotators’ socio-demographic information (when viable to do so responsibly) when releasing datasets, especially those capturing relatively subjective tasks. Inclusion of this information will enable more research on how to account for systematic disagreements between annotators in training tasks.
2 Background
NLP has a long history of developing techniques to interpret subjective language Wiebe et al. (2004); Alm (2011). While all human judgments embed some degree of subjectivity, some tasks such as sentiment analysis Liu et al. (2010), affect modeling Alm (2008); Liu et al. (2003), emotion detection Hirschberg et al. (2003), and hate speech detection Warner and Hirschberg (2012) are agreed upon as relatively more subjective in nature. As Alm (2011) points out, achieving a single real ‘ground truth’ is not possible, nor essential in case of such subjective tasks. Instead, we should investigate how to model the subjective interpretations of the annotators, and how to account for them in application scenarios.
However, the current practice in the NLP community continues to be applying different aggregation strategies to arrive at a single score or label that makes it amenable to train and evaluate supervised machine learning models. Oftentimes, datasets are released with only the final scores/labels, essentially obfuscating important nuances in the task. The information released about the annotations can be at one of the following four levels of information-richness.
Firstly, the most common approach is one in which multiple annotations obtained for a data instance are aggregated to derive a single “ground truth” label, and these labels are the only annotations included in the released dataset (e.g., Founta et al. (2018)). The aggregation strategy most commonly used, especially in large datasets, is majority voting, although smaller datasets sometimes use adjudication by an ‘expert’ (often one of the study authors themselves) to arrive at a single label (e.g., in Waseem and Hovy (2016)) when there are substantial disagreements between annotators. These aggregation approaches rely on the assumption that there always exist a single correct label, and that either the majority label or the ‘expert’ label is more likely to be that correct label. What it fails to account for is the fact that in many subjective tasks, e.g., detecting hate speech, the perceptions of individual annotators may be as valuable as an ‘expert’ perspective.
Secondly, some datasets (e.g., Jigsaw (2018); Davidson et al. (2017)) release the distribution across labels rather than a single aggregated label. In binary classification tasks, this corresponds to the percentage of annotators who chose one of the labels. In multi-class classification, this may be the distribution across labels obtained for an instance. While this provides more information than a single aggregated label does (e.g., identifies the instances with high disagreement), it fails to capture annotator-level systematic differences.
Thirdly, some datasets release annotations made by each individual annotators in an anonymous fashion (e.g., Kennedy et al. (2020); Jigsaw (2019)). Such annotator-level labels allow downstream dataset users to investigate and account for systematic differences between individual annotators’ perspectives on the tasks, although they do not contain any information about each annotators’ socio-cultural backgrounds. Finally, some recent datasets (e.g., Díaz et al. (2018)) also release such socio-demographic information about the annotators in addition to annotator-level labels. This information may include various identity subgroups the annotators self-identify with (e.g., gender, race, age range, etc.), or survey responses from the annotators that capture their value systems, lived experiences, or expertise, as they relate to the specific task at hand. Such information, while tricky to share responsibly, would help enable analysis around representation of marginalized perspectives in datasets, as we demonstrate in the next section.
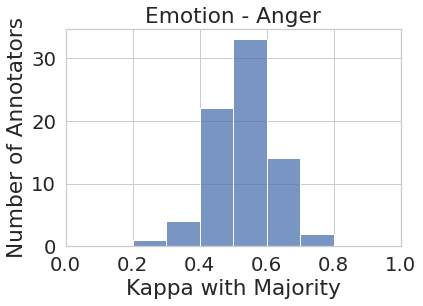
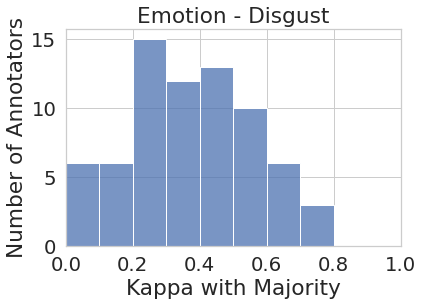
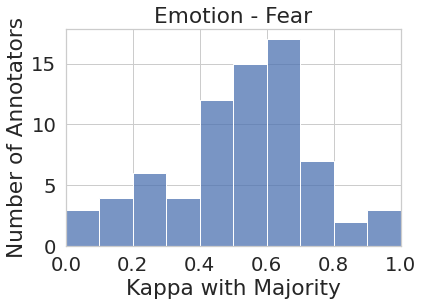
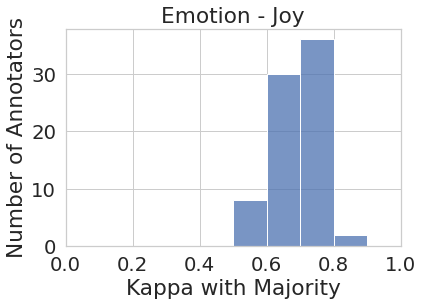
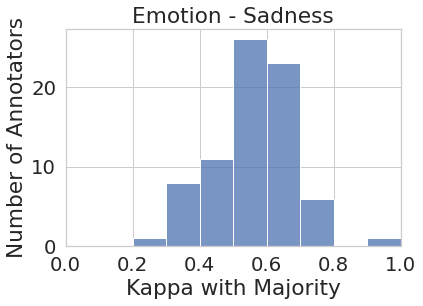
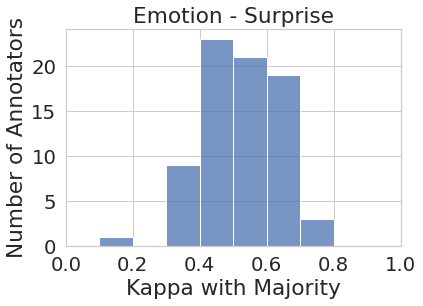
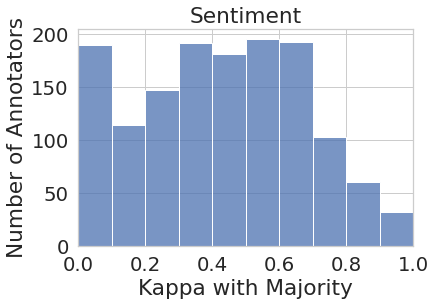
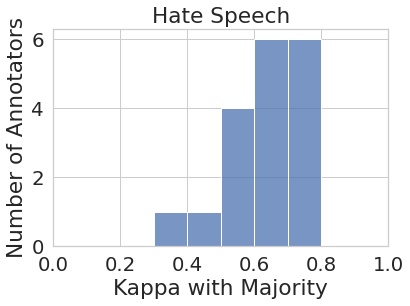
3 Impacts of Aggregation
In this section, we investigate how the aggregation of multiple annotations to a single label impact representations of individual and group perspectives in the resulting datasets. We analyze annotations for eight binary classification tasks, across three different datasets: hate-speech Kennedy et al. (2020), sentiment Díaz et al. (2018), and emotion Demszky et al. (2020). Table 1 shows the number of instances, annotators and individual annotations present in the datasets. For hate-speech and emotion datasets, we use the binary label in the raw annotations, whereas for the sentiment dataset, we map the 5-point ordinal labels (-2, -1, 0, +1, +2) in the raw data to a binary distinction denoting whether the text was deemed positive or negative.111We do this mapping for the purposes of this analysis, where we are focusing on binary tasks. Ideally, a more nuanced 5-point labeling schema will be more useful. While the emotion dataset contains annotations for 28 different emotions, in this work, for brevity, we focused on the annotations for only the six standard Ekman emotions Ekman (1992) — anger, disgust, fear, joy, sadness, and surprise. In particular, we use the raw annotations for these six emotions, rather than the mapping of all 28 emotions onto these six emotions that Demszky et al. (2020) use in some of their experiments.
Dataset | #instances | #annotators | #annotations |
---|---|---|---|
Hate-speech | 27,665 | 18 | 86,529 |
Sentiment | 14,071 | 1,481 | 59,240 |
Emotion | 58,011 | 82 | 211,225 |
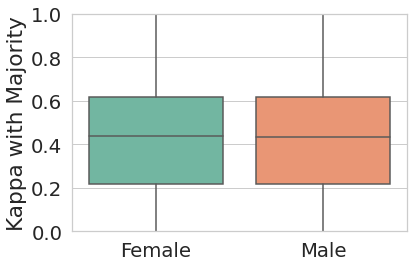
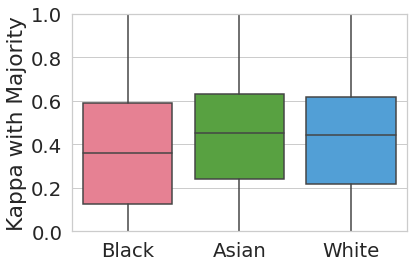
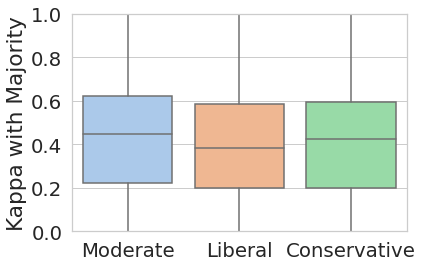
3.1 Q1: Do Aggregated Labels Represent Individual Annotators Uniformly?
First, we investigate whether the aggregated labels obtained through majority labels provide a more or less equal representation for all annotator perspectives. For this analysis, we calculate the majority label for each instance as the label that half or more annotators who annotated that instance agreed on. We then measure Cohen’s Kappa agreement score for each individual annotator’s labels and the majority labels on the subset of instances they annotated. While lower agreement scores between some individual annotators and the majority vote is expected (e.g., if the annotator produced noisy labels, or they misunderstood the task), the assumption is that the majority label captures the perspective of the ‘average human annotator’ within the annotator pool.
Figure 1 represents the histogram of annotators’ agreement scores with majority votes for all eight tasks. While the majority vote in some tasks such as joy and sadness (to some extent) do represent most of the annotator pool more or less uniformly (i.e., majority vote agrees with most annotators at around the same rate), in most cases, the majority vote under-represents or outright ignores the perspectives of a substantial number of annotators. For instance, majority vote for disgust has very low agreement () with almost one-third (27 out of 82) of the annotator pool. Similarly, majority vote for sentiment has very low agreement with around one-third (450+) of their annotator pool.
3.2 Q2: Do Aggregated Labels Represent All Social Groups Uniformly?
While the analysis on Q1 reveals that certain annotator perspectives are more likely to be ignored in the majority vote, it is especially problematic from a fairness perspective, if these differences vary across different social groups. Here, we investigate whether specific socio-demographic groups and their perspectives are unevenly disregarded through annotation aggregation. To this end, we analyze the sentiment analysis dataset (Díaz et al., 2018) since it includes raw annotations as well as annotators’ self-identified socio-demographic information. Furthermore, as observed in Figure 1, a large subset of annotators in this dataset are in low agreement with the aggregated labels.
We study three demographic attributes, namely race, gender, and political affiliation and compare the agreement scores between the aggregated labels and the individual annotators’ labels within each group. Figure 2 shows the average and standard deviation of annotators’ agreement scores with aggregated labels for each demographic group: race (Asian, Black, and White), gender (Male, and Female), and political affiliation (Conservative, Moderate, and Liberal).222We removed social groups with fewer than 50 annotators from this analysis for lack of sufficient data points. These include other racial groups such as ‘Middle Eastern’ with 2 annotators, ‘Native Hawaiian or Pacific Islander’ with 4 annotators, and non-binary gender identity with one annotator).
We perform three one-way ANOVA tests to test whether annotators belonging to different demographic groups have significantly different agreement scores with the aggregated labels, on average. The results show significant differences among racial groups (=3.77, =0.02); in particular, White annotators show an average agreement of 0.42 (=0.26), significantly higher (=0.03 according to a post-hoc Tukey test) than Black annotators with average of 0.37 (=0.27). The difference between average agreement scores across different political groups are not statistically significant, although moderate annotators on average have higher agreement (0.42) compared to conservative and liberal annotators (0.40 and 0.38, respectively). Similarly, annotation agreements of male and female annotators are not significantly different.
4 Utility of Annotator-level Labels
Another argument in favor of retaining annotator-level labels is their utility in modeling disagreement during training and evaluation. Prabhakaran et al. (2012) and Plank et al. (2014) incorporated annotator disagreement in the loss functions used during training to improve predictive performance. Cohn and Specia (2013) and Fornaciari et al. (2021) use a multi-task approach to incorporate annotator disagreements to improve machine translation and part-of-speech tagging performance, respectively. Chou and Lee (2019) and Guan et al. (2018) developed learning architectures that model individual annotators as a way to improved performance. Wich et al. (2020) show the utility of detecting clusters of annotators in hate-speech detection based on how often they agree with each other. Finally, Davani et al. (2021) introduce a multi-annotator architecture that models each annotators’ perspectives separately using a multi-task approach. They demonstrate that this architecture helps to model uncertainty in predictions, without any significant loss of accuracy or efficiency. This array of recent work further demonstrates the utility of retaining annotator-level information in the datasets for downstream modeling steps.
5 Discussion and Conclusion
Building models to predict or measure subjective phenomena based on human annotations should involve explicit consideration for the unique perspectives each annotator brings forth in their annotations. Annotators are not interchangeable– that is, they draw from their socially-embedded experiences and knowledge when making annotation judgments. As a result, retaining their perspectives separately in the datasets will enable dataset users to account for these differences according to their needs. We demonstrated that annotation aggregation may unfairly disregard perspectives of certain annotators, and sometimes certain socio-demographic groups. Based on our analysis, we propose three recommendations aimed to avoid these issues:
Annotator-level labels:
We urge dataset developers to release the annotator-level labels, preferably in an anonymous fashion, and leave open the choice of whether and how to utilize or aggregate these labels for the dataset users.
Socio-demographic information:
Sociodemographic identity of the annotators is crucial to ascertain that the datasets (and the models trained on them) equitably represent perspectives of various social groups. We urge dataset developers to include socio-demographic information of annotators, when viable to do so responsibly.
Documentation about recruitment, selection, and assignment of annotators:
Finally, we urge dataset developers to document how the annotators were recruited, the criteria used to select them and assign data to them, and any efforts to ensure representational diversity, through transparency artefacts such datasheets Gebru et al. (2018) or data statements Bender and Friedman (2018).
Acknowledgements
We thank Ben Hutchinson for valuable feedback on the manuscript. We also thank the anonymous reviewers for their feedback.
References
- Alm (2011) Cecilia Ovesdotter Alm. 2011. Subjective natural language problems: Motivations, applications, characterizations, and implications. In Proceedings of the 49th Annual Meeting of the Association for Computational Linguistics: Human Language Technologies, pages 107–112.
- Alm (2008) Ebba Cecilia Ovesdotter Alm. 2008. Affect in* Text and Speech. Ph.D. thesis, University of Illinois at Urbana-Champaign.
- Aroyo and Welty (2013) Lora Aroyo and Chris Welty. 2013. Crowd truth: Harnessing disagreement in crowdsourcing a relation extraction gold standard. WebSci2013. ACM, 2013.
- Bender and Friedman (2018) Emily M Bender and Batya Friedman. 2018. Data statements for natural language processing: Toward mitigating system bias and enabling better science. Transactions of the Association for Computational Linguistics, 6:587–604.
- Chou and Lee (2019) Huang-Cheng Chou and Chi-Chun Lee. 2019. Every rating matters: Joint learning of subjective labels and individual annotators for speech emotion classification. In ICASSP 2019-2019 IEEE International Conference on Acoustics, Speech and Signal Processing (ICASSP), pages 5886–5890. IEEE.
- Cohn and Specia (2013) Trevor Cohn and Lucia Specia. 2013. Modelling annotator bias with multi-task gaussian processes: An application to machine translation quality estimation. In Proceedings of the 51st Annual Meeting of the Association for Computational Linguistics (Volume 1: Long Papers), pages 32–42.
- Davani et al. (2021) Aida Mostafazadeh Davani, Mark Diaz, and Vinodkumar Prabhakaran. 2021. Dealing with disagreements: Looking beyond the majority vote in subjective annotations. Transactions of the Association for Computational Linguistics.
- Davidson et al. (2017) Thomas Davidson, Dana Warmsley, Michael Macy, and Ingmar Weber. 2017. Automated hate speech detection and the problem of offensive language. Proceedings of the International AAAI Conference on Web and Social Media, 11(1).
- Demszky et al. (2020) Dorottya Demszky, Dana Movshovitz-Attias, Jeongwoo Ko, Alan Cowen, Gaurav Nemade, and Sujith Ravi. 2020. GoEmotions: A dataset of fine-grained emotions. In Proceedings of the 58th Annual Meeting of the Association for Computational Linguistics, Online. Association for Computational Linguistics.
- Díaz et al. (2018) Mark Díaz, Isaac Johnson, Amanda Lazar, Anne Marie Piper, and Darren Gergle. 2018. Addressing age-related bias in sentiment analysis. In Proceedings of the 2018 CHI Conference on Human Factors in Computing Systems, pages 1–14.
- Ekman (1992) Paul Ekman. 1992. An argument for basic emotions. Cognition & emotion, 6(3-4):169–200.
- Fornaciari et al. (2021) Tommaso Fornaciari, Alexandra Uma, Silviu Paun, Barbara Plank, Dirk Hovy, and Massimo Poesio. 2021. Beyond black & white: Leveraging annotator disagreement via soft-label multi-task learning. In Proceedings of the 2021 Conference of the North American Chapter of the Association for Computational Linguistics: Human Language Technologies, pages 2591–2597, Online. Association for Computational Linguistics.
- Founta et al. (2018) Antigoni Maria Founta, Constantinos Djouvas, Despoina Chatzakou, Ilias Leontiadis, Jeremy Blackburn, Gianluca Stringhini, Athena Vakali, Michael Sirivianos, and Nicolas Kourtellis. 2018. Large scale crowdsourcing and characterization of twitter abusive behavior. In Twelfth International AAAI Conference on Web and Social Media.
- Gebru et al. (2018) Timnit Gebru, Jamie Morgenstern, Briana Vecchione, Jennifer Wortman Vaughan, Hanna Wallach, Hal Daumé III, and Kate Crawford. 2018. Datasheets for datasets. arXiv preprint arXiv:1803.09010.
- Guan et al. (2018) Melody Guan, Varun Gulshan, Andrew Dai, and Geoffrey Hinton. 2018. Who said what: Modeling individual labelers improves classification. Proceedings of the AAAI Conference on Artificial Intelligence, 32(1).
- Hirschberg et al. (2003) Julia Hirschberg, Jackson Liscombe, and Jennifer Venditti. 2003. Experiments in emotional speech. In ISCA & IEEE Workshop on Spontaneous Speech Processing and Recognition.
- Jigsaw (2018) Jigsaw. 2018. Toxic comment classification challenge. Accessed: 2021-05-01.
- Jigsaw (2019) Jigsaw. 2019. Unintended bias in toxicity classification. Accessed: 2021-05-01.
- Kennedy et al. (2020) Brendan Kennedy, Mohammad Atari, Aida Mostafazadeh Davani, Leigh Yeh, Ali Omrani, Yehsong Kim, Kris Coombs Jr., Shreya Havaldar, Gwenyth Portillo-Wightman, Elaine Gonzalez, Joe Hoover, Aida Azatian, Gabriel Cardenas, Alyzeh Hussain, Austin Lara, Adam Omary, Christina Park, Xin Wang, Clarisa Wijaya, Yong Zhang, Beth Meyerowitz, and Morteza Dehghani. 2020. The gab hate corpus: A collection of 27k posts annotated for hate speech.
- Liu et al. (2010) Bing Liu et al. 2010. Sentiment analysis and subjectivity. Handbook of natural language processing, 2(2010):627–666.
- Liu et al. (2003) Hugo Liu, Henry Lieberman, and Ted Selker. 2003. A model of textual affect sensing using real-world knowledge. In Proceedings of the 8th international conference on Intelligent user interfaces, pages 125–132.
- Luo et al. (2020) Yiwei Luo, Dallas Card, and Dan Jurafsky. 2020. Detecting stance in media on global warming. In Findings of the Association for Computational Linguistics: EMNLP 2020, pages 3296–3315, Online. Association for Computational Linguistics.
- Nowak and Rüger (2010) Stefanie Nowak and Stefan Rüger. 2010. How reliable are annotations via crowdsourcing: a study about inter-annotator agreement for multi-label image annotation. In Proceedings of the international conference on Multimedia information retrieval, pages 557–566.
- Patton et al. (2019) Desmond Patton, Philipp Blandfort, William Frey, Michael Gaskell, and Svebor Karaman. 2019. Annotating social media data from vulnerable populations: Evaluating disagreement between domain experts and graduate student annotators. In Proceedings of the 52nd Hawaii International Conference on System Sciences.
- Plank et al. (2014) Barbara Plank, Dirk Hovy, and Anders Søgaard. 2014. Learning part-of-speech taggers with inter-annotator agreement loss. In Proceedings of the 14th Conference of the European Chapter of the Association for Computational Linguistics, pages 742–751.
- Prabhakaran et al. (2012) Vinodkumar Prabhakaran, Michael Bloodgood, Mona Diab, Bonnie Dorr, Lori Levin, Christine Piatko, Owen Rambow, and Benjamin Van Durme. 2012. Statistical modality tagging from rule-based annotations and crowdsourcing. In Proceedings of the Workshop on Extra-Propositional Aspects of Meaning in Computational Linguistics, pages 57–64.
- Snow et al. (2008) Rion Snow, Brendan O’connor, Dan Jurafsky, and Andrew Y Ng. 2008. Cheap and fast–but is it good? evaluating non-expert annotations for natural language tasks. In Proceedings of the 2008 conference on empirical methods in natural language processing, pages 254–263.
- Warner and Hirschberg (2012) William Warner and Julia Hirschberg. 2012. Detecting hate speech on the world wide web. In Proceedings of the second workshop on language in social media, pages 19–26.
- Waseem (2016) Zeerak Waseem. 2016. Are you a racist or am i seeing things? annotator influence on hate speech detection on twitter. In Proceedings of the first workshop on NLP and computational social science, pages 138–142.
- Waseem and Hovy (2016) Zeerak Waseem and Dirk Hovy. 2016. Hateful symbols or hateful people? predictive features for hate speech detection on twitter. In Proceedings of the NAACL student research workshop, pages 88–93.
- Wich et al. (2020) Maximilian Wich, Hala Al Kuwatly, and Georg Groh. 2020. Investigating annotator bias with a graph-based approach. In Proceedings of the Fourth Workshop on Online Abuse and Harms, pages 191–199.
- Wiebe et al. (2004) Janyce Wiebe, Theresa Wilson, Rebecca Bruce, Matthew Bell, and Melanie Martin. 2004. Learning subjective language. Computational linguistics, 30(3):277–308.