On Approximation by Kantorovich Exponential Sampling Operators
Abstract
In this article, we extend our study of Kantorovich type exponential sampling operators introduced in [34]. We derive the Voronovskaya type theorem and its quantitative estimates for these operators in terms of an appropriate K-functional. Further, we improve the order of approximation by using the convex type linear combinations of these operators. Subsequently, we prove the estimates concerning the order of convergence for these linear combinations. Finally, we give some examples of kernels along with the graphical representations.
Keywords. Kantorovich type sampling operators. Order of approximation. K-functional. Mellin transform
2010 Mathematics Subject Classification. 41A35. 30D10. 94A20. 41A25.
1 Introduction
The sampling theory has been finding its great applications mainly in approximation theory and signal processing. One of the most prominent result of the sampling theory is named after Wittaker-Kotelnikov-Shannon (WKS). The WKS sampling theorem [4] provides an exact reconstruction formula for the band-limited functions. Butzer [25] initiated the study of generalized sampling series which generalizes the WKS theorem for the functions which are not necessarily band-limited. These operators have great importance in developing the models for the reconstruction of signals. The theory has been developed by many authors in various aspects, see eg., [24, 8, 30, 42, 6, 22, 23, 41].
In the past three decades, the exponential sampling has become an advantageous tool to deal with the problems arising in the wide areas of mathematics as well as physics, see eg. [19, 21, 32, 40]. Bartero, Pike [19] and Gori [32] proposed the exponential sampling formula to approximate the Mellin band-limited functions having exponentially spaced data. This formula is widely considered as the Mellin-version of the well known Shannon sampling theorem [25]. But, the pioneering idea of mathematical study of exponential sampling formula is credited to Butzer and Jansche (see [27]) by using the theory of Mellin transform. The separate study of Mellin’s theory has been initiated by Mamedov in [38] and carried forward in [26, 27, 28]. Bardaro et al. generalized the above theory by replacing the function in the exponential sampling formula by the more general kernel function in [16]. It allows to approximate a continuous function by using its values at the points But in practice, it is difficult to obtain the sample values at these nodes exactly. Thus, it is advantageous to replace the value by the mean value of in the interval for This led us to introduce and analyze the Kantorovich version of the generalized exponential sampling series in [34]. The Kantorovich type generalization of operators is a significant subject in approximation theory as the Lebesgue integrable functions can be approximated by using Kantorovich type operators. In the last few decades, the Kantorovich modifications of several operators have been constructed and analyzed, eg.[5, 7, 9, 25, 29, 31, 36, 35, 1, 33, 2]. We also refer some of the recent developments related to the theory of exponential sampling, see [16, 12, 17, 3, 18, 34].
In view of Corollary 2 of [16], it is evident that the convergence of the operator to is of order for under the assumptions that the higher order moments of the kernel are null. The situation becomes comparatively downer for the Kantorovich version of the above operator which is introduced and analyzed in [34]. In Theorem 2 of [34], we derived the following asymptotic formula under the assumption that the first order discrete moment vanishes. For and we have
It is clear from the above formula that the convergence of the operator to is of order for In view of Theorem 3.1 of section 3, it is evident that one can not improve the order of convergence even if the existence of the higher derivatives for the function is assured and the higher order moments for the kernel are vanishing on Subsequently, it is hard to find the examples of the kernel function such that the higher order moments of the kernel are null. This motivates us to investigate further about the order of convergence of the family of operators
The idea of considering the linear combination of the operators is mainly inspired from the pioneer study of Butzer [20] and by many authors in [37, 10, 11, 3]. In this paper, we implement this constructive approach to improve the order of convergence for the Kantorovich exponential sampling operators avoiding the constraint that the higher order moments for the kernels must vanish on
The paper is organized as follows. In section 3, we obtain the asymptotic formula and the quantitative estimates for the above family of operators in terms of Peetre’s K-functional. The section 4 is devoted to analyse the approximation properties of the linear combinations of Kantorovich exponential sampling operators. We also prove the better order of convergence for these operators. Finally we have shown the approximation of different functions by the Kantorovich exponential sampling operators and its linear combination of operators. The error estimates are also provided.
2 Preliminaries
In what follows, we denote by the space of all functions such that upto order derivatives are continuous and bounded on . Let ) be the space of all continuous and bounded functions on . A function ) is called log-uniformly continuous on , if for any given there exists such that whenever for any We denote the space of all log-uniformly continuous and bounded functions defined on by Similarly, denotes the space of functions which are n-times Mellin continuously differentiable and We consider as the class of all Lebesgue measurable functions on and as the space of all bounded functions on throughout this paper.
For , let ) be the space of all the Lebesgue measurable and -integrable functions defined on equipped with the usual norm . For , we define the space
equipped with the norm
The Mellin transform of a function is defined by
A function is called Mellin band-limited in the interval if for all
Let and Then, Mellin differential operator is defined by
We consider throughout this paper. The Mellin differential operator of order is defined by The basic properties of the Mellin transform can be found in [26].
2.1 Kantorovich type Exponential Sampling Operators
Let and The Kantorovich version of the exponential sampling operators is defined by ([34])
(2.1) |
where is locally integrable such that the above series is convergent for every . It is clear that for the above series is well defined for every Let be the kernel function which is continuous on such that it satisfies the following conditions:
(i) For every
(ii) For some and
uniformly with respect to
Remark 2.1
The condition (ii) implies that there holds
We define the algebraic moments of order for the kernel function as
Similarly, the absolute moment of order can be defined as
We define
3 Approximation Results
In this section, we establish some direct results e.g., Voronovskaya type asymptotic formula and its quantitative estimation for the Kantorovich exponential sampling operators (2.1).
Theorem 3.1
Let be the kernel function and Then we have
where represents an absolutely convergent series given by
Moreover, we have as
Proof. For the Taylor’s formula in terms of Mellin derivatives ([16]) can be written as
where is a bounded function such that In view of (2.1) we obtain
where For any fixed index we obtain
Next we estimate the remainder term
Using condition (ii) and the fact that is bounded such that we see that
This clearly shows that the series is absolutely convergent and as for arbitrary
On combining all the estimates, we obtain the desired result.
As a consequence of the above theorem, we establish the following corollary.
Corollary 3.1
Let be the kernel and Then, we have the following asymptotic formula
Moreover, if for then for we have
3.1 Quantitative estimates
Now we derive the quantitative estimates concerning the order of convergence for the family of operators by using the Peetre’s- K functional (see [13]). The Peetre’s K-functional for is defined by
Theorem 3.2
Let be the kernel function and Then the following estimate holds
Proof. From the Mellin’s Taylor formula for we have
Now we substitute Then, by using the estimate (see [13]), we can write
Taking infimum over we obtain
Theorem 3.3
Let and be the kernel such that for Then, we have
where and
Proof. From the Mellin’s Taylor formula, we have
Putting and using the estimate we obtain
Now taking infimum over we get the desired estimate.
4 Construction of linear combinations
This section is devoted to the study of approximation properties of the linear combinations of the Kantorovich exponential sampling operators. Our central aim is to construct the appropriate linear combination of the operators to produce the better order of convergence in the asymptotic formula. Let be non-zero real numbers such that For and we define the linear combination of the above operators as follows.
(4.2) |
Now we prove the asymptotic formula for the family of operators defined in (4.2).
Theorem 4.1
Let be the kernel function and Then, we have
where
Proof. From the condition we can write
Now using the order Mellin’s Taylor formula, we obtain
where Now proceeding along the lines of Theorem 3.1, it follows that as Hence, the proof is completed.
From the above theorem, we deduce the following Voronovskaja type asymptotic results.
Corollary 4.1
For we have
Furthermore, if for we have
Corollary 4.2
Under the assumptions of Theorem 4.1 and if for then for with we have
It is important to remark that does not vanish in general, even if the higher order moments for the kernel are zero on So, in order to have for we need to solve the following system
The solution of the above system yields a linear combination which provides the convergence of order at least for functions Let and then we have
On solving, we obtain and Then the linear combination is given by
(4.3) |
Now for the asymptotic formula for combination (4.3) acquires the form
which provides the convergence of order at least 2 for Similarly, if we take then for we need to solve the following system:
The solution gives and Then the following linear combination ensures the order of convergence atleast 3 for
(4.4) |
The corresponding asymptotic formula has the form
Theorem 4.2
Let be the kernel function and Then, we have
where and
Proof. We see that
In an analogous way to Theorem 3.2, we put for and using the estimate , we obtain
Now taking infimum over we get the desired result.
Now, we have the following corollary.
Corollary 4.3
Let be the kernel function and with for Then, we have the following estimate
where and
5 Examples and graphical representation
We begin with the well-known Mellin’s B-spline function(see [16]). The Mellin’s B-splines of order for are defined as
where Since is compactly supported and continuous on we have for any The Mellin transformation of is given by
(5.5) |
To show that the above kernel satisfies the assumptions (i) and (ii), we use the following Mellin’s Poisson summation formula
The Mellin B-spline satisfies all the assumptions of the presented theory (see [16, 3, 34]). Consider the Mellin B-spline of order 2
Now we show the approximation of by and obtained in (4.4) for and (Fig.1). It is evident from the graph that the combination (4.4) provides the better rate of convergence.
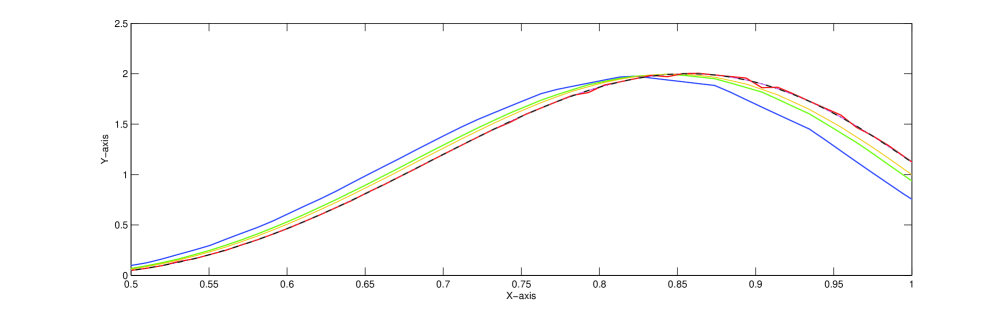
Table 1
Error estimation (upto decimal points) in the approximation of by and for and
Now let us consider order Mellin B-spline function From the Mellin’s Poisson summation formula, we obtain Now for we have the asymptotic formula as
But in view of (4.3) and Corollary 2, we obtain the following asymptotic formula
This clearly shows that the combination (4.3) provides the order of convergence atleast 2 in asymptotic formula for Subsequently, let and In view of Theorem 1, we have the following asymptotic formula
But the combination (4.4) provides the following asymptotic formula
which ensures the rate of convergence atleast 3 for
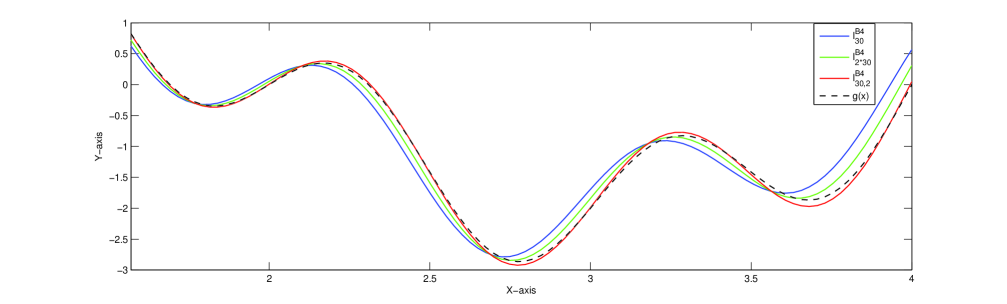
Table 2
Error estimation (upto decimal points) in the approximation of by and for and
Now we consider the linear combination of the Mellin’s B-spline functions of order as follows:
Here, represents h-translates of the function and is defined as The Mellin’s transformation of is given by
(5.6) | |||||
which gives
Again from (5.6), we write
In view of Lemma 1 in [34], we obtain
On solving for we get
In particular for and we obtain the following linear combination of the Mellin’s B-spline of order 4
Indeed, it satisfies all the assumptions of the kernel function. Again by using the Mellin’s Poisson summation formula, we obtain
Now for and we have the following asymptotic formulae for the operators and
and
Evidently, the combination (4.3) shows the convergence of order atleast 2 for
References
- [1] Angamuthu, S.K., Ponnaian, D.; Approximation by generalized bivariate Kantorovich sampling type series. J Anal. 27, 429-449 (2019).
- [2] Agrawal, P.N., Prasad, G; Degree of approximation to integrable functions by Kantorovich polynomials, Boll. Un. Mat. Ital. A (6) 4 , 323-326 (1985).
- [3] Balsamo, S., Mantellini, I.; On linear combinations of general exponential sampling series, Results Math. 74 (180) (2019).
- [4] Bardaro, C., Butzer, P.L., Stens, R.L., Vinti, G.; Approximation of the Whittaker Sampling Series in terms of an Average Modulus of Smoothness covering Discontinuous Signals. J. Math. Anal. Appl. 316, 269-306(2006).
- [5] Bardaro, C., Vinti, G., Butzer, P.L., Stens, R.; Kantorovich-type generalized sampling series in the setting of Orlicz spaces. Sampl. Theory Signal Image Process. 6, 29-52 (2007).
- [6] Bardaro, C., Mantellini, I.; A quantitative Voronovskaja formula for generalized sampling operators, East J. Approx. 15 (4) , 459-471(2009).
- [7] Bardaro, C., Mantellini, I.; Voronovskaya formulae for Kantorovich type generalized sampling series. Int. J. Pure Appl. Math. 62 , 247-262 (2010).
- [8] Bardaro, C., Butzer, P.L., Stens, R., Vinti, G.; Prediction by samples from the past with error estimates covering discontinuous signals, IEEE Transactions on Information Theory. 56(1), 614-633(2010).
- [9] Bardaro, C., Mantellini, I.; On convergence properties for a class of Kantorovich discrete operators. Numer. Funct. Anal. Optim. 33 , 374-396 (2012).
- [10] Bardaro, C., Mantellini, I.; Asymptotic formulae for linear combinations of generalized sampling type operators. Z. Anal. Anwend. 32(3), 279-298 (2013).
- [11] Bardaro, C., Mantellini, I; On Linear Combinations of Multivariate Generalized Sampling Type Series. Mediterr. J. Math. 10, 1833-1852 (2013).
- [12] Bardaro, C., Butzer, P.L., Mantellini, I.; The exponential sampling theorem of signal analysis and the reproducing kernel formula in the Mellin transform setting. Sampl. Theory Signal Image Process. 13, 35-66 (2014).
- [13] Bardaro, C., Mantellini, I.; On Mellin convolution operators: a direct approach to the asymptotic formulae. Integral Transforms Spec. Funct. 25, 182-195 (2014).
- [14] Bardaro, C., Butzer, P.L., Mantellini, I.; The Mellin-Parseval formula and its interconnections with the exponential sampling theorem of optical physics. Integral Transforms Spec. Funct. 27, 17-29(2016).
- [15] Bardaro, C., Butzer, P.L., Mantellini, I., Schmeisser, G.; On the Paley-Wiener theorem in the Mellin transform setting. J. Approx. Theory. 207, 60-75(2016).
- [16] Bardaro, C., Faina, L., Mantellini, I.; A generalization of the exponential sampling series and its approximation properties. Math. Slovaca 67 , 1481-1496 (2017).
- [17] Bardaro, C., Mantellini, I., Sch meisser, G.; Exponential sampling series: convergence in Mellin-Lebesgue spaces. Results Math. 74 (2019), no. 3, Art. 119, 20 pp.
- [18] Bardaro, C., Bevignani, G., Mantellini, I., Seracini, M.; Bivariate Generalized Exponential Sampling Series and Applications to Seismic Waves, Constructive Mathematical Analysis, 2 , 153-167(2019).
- [19] Bertero, M., Pike, E.R.; Exponential-sampling method for Laplace and other dilationally invariant transforms. II. Examples in photon correlation spectroscopy and Fraunhofer diffraction. Inverse Problems 7, 21-41(1991).
- [20] Butzer, P.L., Linear Combinations of Bernstein Polynomials. Canad. J. Math. 5, 559-567(1953).
- [21] Casasent, D.; Optical data processing, Springer, Berlin, 241-282 (1978).
- [22] Butzer, P. L., Ries, S., Stens, R. L.; Approximation of continuous and discontinuous functions by generalized sampling series, J. Approx. Theory. 50, 25-39(1987).
- [23] Butzer, P. L., Stens, R. L.; Prediction of non-bandlimited signals from past samples in terms of splines of low degree, Math. Nachr. 132 , 115-130(1987).
- [24] Butzer, P. L., Stens, R. L.; Sampling theory for not necessarily band-limited functions: an historical overview, SIAM Review. 34, 40-53(1992).
- [25] Butzer, P.L., Stens, R.L.; Linear prediction by samples from the past, In: Advanced Topics in Shannon Sampling and Interpolation Theory ,Springer Texts Electrical Eng., Springer, New York, 157-183(1993).
- [26] Butzer, P.L., Jansche, S.; A direct approach to the Mellin transform. J. Fourier Anal. Appl. 3, 325-376(1997).
- [27] Butzer, P.L., Jansche, S.; The exponential sampling theorem of signal analysis, Dedicated to Prof. C. Vinti (Italian) (Perugia, 1996), Atti. Sem. Mat. Fis. Univ. Modena, Suppl. 46 , 99-122(1998).
- [28] Butzer, P.L., Stens, R.L.; A self contained approach to Mellin transform analysis for square integrable functions;applications. Integral Transform. Spec. Funct. 8 ,175-198(1999).
- [29] Cluni, F., Costarelli, D., Minotti, A.M., Vinti, G.; Applications of sampling Kantorovich operators to thermographic images for seismic engineering. J. Comput. Anal. Appl. 19 , 602-617(2015).
- [30] Costarelli, D., Minotti, A.M., Vinti, G.; Approximation of discontinuous signals by sampling Kantorovich series. J. Math. Anal. Appl. 450, 1083-1103(2017).
- [31] Costarelli, D., Vinti, G.; Inverse results of approximation and the saturation order for the sampling Kantorovich series. J. Approx. Theory 242, 64-82(2019).
- [32] Gori, F.; Sampling in optics. Advanced topics in Shannon sampling and interpolation theory, 37-83, Springer Texts Electrical Engrg., Springer, New York, 1993.
- [33] Gupta, V., Tachev, G., Acu, A.M., Modified Kantorovich operators with better approximation properties. Numer. Algorithms 81, 125-149 (2019).
- [34] Kumar, A.S., Shivam, B.; Direct and inverse results for Kantorovich type exponential sampling series. arXiv:submit/2992999, (2020)
- [35] Kumar, A.S., Shivam, B.; Inverse approximation and GBS of bivariate Kantorovich type sampling series. RACSAM 114, 82 (2020).
- [36] Kumar, A. S., Pourgholamhossein, M., Tabatabaie, S. M.; Generalized Kantorovich sampling type series on hypergroups. Novi Sad J. Math. 48 ,117-127(2018).
- [37] May, C. P.; Saturation and inverse theorems for combinations of a class of exponential-type operators. Canadian J. Math. 28 , 1224-1250(1976).
- [38] Mamedov, R.G.; The Mellin transform and approximation theory , ”Elm”, Baku, 1991, 273 pp.
- [39] Orlova, O., Tamberg, G.; On approximation properties of generalized Kantorovich-type sampling operators, J. Approx. Theory, 201 , 73-86(2016).
- [40] Ostrowsky, N., Sornette, D., Parke, P., Pike, E.R.; Exponential sampling method for light scattering polydispersity analysis, Opt. Acta. 28 , 1059-1070(1994).
- [41] Ries, S., Stens, R. L.; Approximation by generalized sampling series, In Constructive Theory of Functions, Proc. Conf. Varna, 1984, edited by Bl. Sendov et al., Publ. House Bulgarian Acad. Sci, Sofia, 746-756(1984).
- [42] Vinti, G., Luca, Z., Approximation results for a general class of Kantorovich type operators. Adv. Nonlinear Stud. 14, 991-1011(2014).