Note on approximating the Laplace transform of a Gaussian on a complex disk
Abstract
In this short note we study how well a Gaussian distribution can be approximated by distributions supported on . Perhaps, the natural conjecture is that for large the almost optimal choice is given by truncating the Gaussian to . Indeed, such approximation achieves the optimal rate of in terms of the -distance between characteristic functions. However, if we consider the -distance between Laplace transforms on a complex disk, the optimal rate is , while truncation still only attains . The optimal rate can be attained by the Gauss-Hermite quadrature. As corollary, we also construct a “super-flat” Gaussian mixture of components with means in and whose density has all derivatives bounded by in the -neighborhood of the origin.
1 Approximating the Gaussian
We study the best approximation of a Gaussian distribution by compact support measures, in the sense of the uniform approximation of the Laplace transform on a complex disk. Let be the Laplace transform, , of the measure and be its characteristic function. Denote and the Laplace transform and the characteristic function corresponding to the standard Gaussian with density
How well can a measure with support on approximate ? Perhaps the most natural choice for is the truncated :
(1) |
where . Indeed, truncation is asymptotically optimal (as ) in approximating the characteristic function, as made preicse by the following result:
Proposition 1.
There exists some such that for all and any probability measure supported on we have
(2) |
Furthermore, truncation (1) satisfies (for )
(3) |
Proof.
Let us define , a holomorphic (entire) function on . Note that if then
and thus for
(4) |
On the other hand, for every we have
(5) |
Applying the Hadamard three-lines theorem to , we conclude that is convex and hence
(6) |
Since the left-hand side of (2) equals , (2) then follows from (4)-(6).
For the converse part, in view of (1), the total variation between and its conditional version is given by
Therefore for the Fourier transform of we get
∎
Despite this evidence, it turns out that for the purpose of approximating Laplace transform in a neighborhood of , there is a much better approximation than (1).
Theorem 2.
There exists some constant such that for any probability measure supported on , , we have
(7) |
Furthermore, there exists an absolute constant so that for all and all there exists distribution (the Gauss-Hermite quadrature) supported on such that
Taking implies that the bound (7) is order-optimal.
Remark 1.
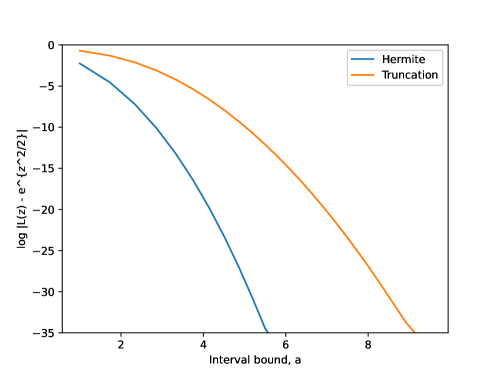
Proof.
As above, denote , and define
From (5) we have for any
and from we also have (for any ):
Applying the Hadamard three-circles theorem, we have is convex, and hence
where and . From here we obtain for some constant
which proves (7).
For the upper bound, take to be the -point Gauss-Hermite quadrature of (cf. [SB02, Section 3.6]). This is the unique -atomic distribution that matches the first moments of . Specifically, we have:
-
•
is supported on the roots of the degree- Hermite polynomial, which lie in [Sze75, Theorem 6.32];
-
•
The -th moment of , denoted by , satisfies for all .
-
•
is symmetric so that all odd moments are zero.
We set , so that is supported on .
Let us denote and . By Taylor expansion we get
Now, we will bound , , . This implies that for all we have
where in the last step we used for some absolute constant . In all, we have that whenever we get
∎
Remark 2.
Note that our proof does not show that for any supported on , its characteristic function restricted on must satisfy:
It is natural to conjecture that this should hold, though.
Remark 3.
Note also that the Gauss-Hermite quadrature considered in the theorem, while essentially optimal on complex disks, is not uniformly better than the naive truncation. For example, due to its finite support, the Gauss-Hermite quadrature is a very bad approximation in the sense of (2). Indeed, for any finite discrete distribution we have , thus only attaining the trivial bound of in the right-hand side of (2). (To see this, note that . By simultaneous rational approximation (see, e.g., [Cas72, Theorem VI, p. 13]), we have infinitely many values such that for all for some . In turn, this implies that , and that along the subsequence of attaining .)
2 Super-flat Gaussian mixtures
As a corollary of construction in the previous section we can also derive a curious discrete distribution supported on such that its convolution with the Gaussian kernel is maximally flat near the origin. More precisely, we have the following result.
Corollary 3.
There exist constants such that for every there exists , with and , , such that
Proof.
Consider the distribution claimed by Theorem 2 for . Then (here and below designates some absolute constant, possibly different in every occurence) we have
Note that the function is also bounded on and thus we have
By Cauchy formula, this also implies that derivatives of the two functions inside must satisfy the same estimate on a smaller disk, i.e.
(8) |
Now, define , where . We then have an identity:
Plugging this into (8) and noticing that we get the result. ∎
Remark 4.
This corollary was in fact the main motivation of this note. More exactly, in the study of the properties of non-parametric maximum-likelihood estimation of Gaussian mixtures, we conjectured that certain mixtures must possess some special in the unit disk on such that . The stated corollary shows that this is not true for all mixtures. See [PW20, Section 5.3] for more details on why lower-bounding the derivative is important. In particular, one open question is whether the lower bound holds (with high probability) for the case when and , are iid samples of , while ’s can be chosen arbitrarily given ’s.
References
- [Cas72] J. W. S. Cassels. An Introduction to Diophantine Approximation. Cambridge University Press, Cambridge, United Kingdom, 1972.
- [PW20] Yury Polyanskiy and Yihong Wu. Self-regularizing property of nonparametric maximum likelihood estimator in mixture models. Arxiv preprint arXiv:2008.08244, Aug 2020.
- [SB02] J. Stoer and R. Bulirsch. Introduction to Numerical Analysis. Springer-Verlag, New York, NY, 3rd edition, 2002.
- [Sze75] G. Szegö. Orthogonal polynomials. American Mathematical Society, Providence, RI, 4th edition, 1975.