Model Agnostic Answer Reranking System for
Adversarial Question Answering
Abstract
While numerous methods have been proposed as defenses against adversarial examples in question answering (QA), these techniques are often model specific, require retraining of the model, and give only marginal improvements in performance over vanilla models. In this work, we present a simple model-agnostic approach to this problem that can be applied directly to any QA model without any retraining. Our method employs an explicit answer candidate reranking mechanism that scores candidate answers on the basis of their content overlap with the question before making the final prediction. Combined with a strong base QA model, our method outperforms state-of-the-art defense techniques, calling into question how well these techniques are actually doing and strong these adversarial testbeds are.
1 Introduction
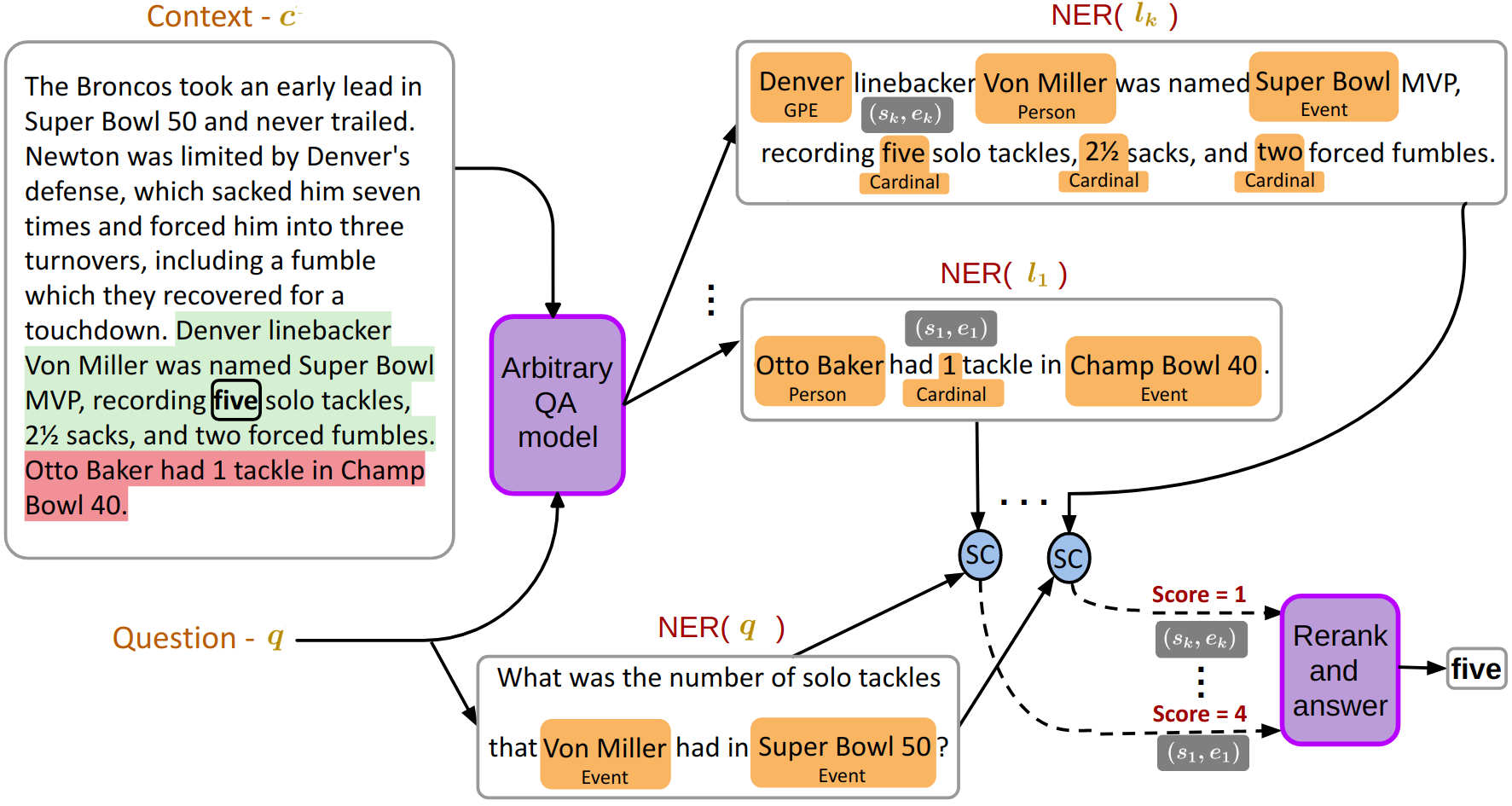
As reading comprehension datasets (Richardson et al., 2013; Weston et al., 2015; Hermann et al., 2015a; Rajpurkar et al., 2016; Joshi et al., 2017) and models (Sukhbaatar et al., 2015; Seo et al., 2016; Devlin et al., 2019) have advanced, QA research has increasingly focused on out-of-distribution generalization (Khashabi et al., 2020; Talmor and Berant, 2019) and robustness. Jia and Liang (2017) and Wallace et al. (2019) show that appending unrelated distractors to contexts can easily confuse a deep QA model, calling into question the effectiveness of these models. Although these attacks do not necessarily reflect a real-world threat model, they serve as an additional testbed for generalization: models that perform better against such adversaries might be expected to generalize better in other ways, such as on contrastive examples (Gardner et al., 2020).
In this paper, we propose a simple method for adversarial QA that explicitly reranks candidate answers predicted by a QA model according to a notion of content overlap with the question. Specifically, by identifying contexts where more named entities are shared with the question, we can extract answers that are more likely to be correct in adversarial conditions.
The impact of this is two-fold. First, our proposed method is model agnostic in that it can be applied post-hoc to any QA model that predicts probabilities of answer spans, without any retraining. Second but most important, we demonstrate that even this simple named entity based question-answer matching technique can be surprisingly useful. We show that our method outperforms state-of-the-art but more complex adversarial defenses with both BiDAF (Seo et al., 2016) and BERT (Devlin et al., 2019) on two standard adversarial QA datasets (Jia and Liang, 2017; Wallace et al., 2019). The fact that such a straightforward technique works well calls into question how reliable current datasets are for evaluating actual robustness of QA models.
2 Related Work
Over the years, various methods have been proposed for robustness in adversarial QA, the most prominent ones being adversarial training (Wang and Bansal, 2018; Lee et al., 2019; Yang et al., 2019b), data augmentation (Welbl et al., 2020) and posterior regularization (Zhou et al., 2019). Among these, we compare our method only with techniques that train on clean SQuAD Wu et al. (2019); Yeh and Chen (2019) for fairness. Wu et al. (2019) use a syntax-driven encoder to model the syntactic match between a question and an answer. Yeh and Chen (2019) use a prior approach (Hjelm et al., 2019) to maximize mutual information among contexts, questions, and answers to avoid overfitting to surface cues. In contrast, our technique is more closely related to retrieval-based methods for open-domain QA (Chen et al., 2017; Yang et al., 2019a) and multi-hop QA (Welbl et al., 2018; De Cao et al., 2019): we show that shallow matching can improve the reliability of deep models against adversaries in addition to these more complex settings.
Methods for (re)ranking of candidate passages/answers have often been explored in the context of information retrieval (Severyn and Moschitti, 2015), content-based QA (Kratzwald et al., 2019) and open-domain QA (Wang et al., 2018; Lee et al., 2018). Similar to our approach, these methods also exploit some measure of coverage of the query by the candidate answers or their supporting passages to decide the ranks. However, the main motive behind ranking in such cases is usually to narrow down the area of interest within the text to look for the answer. On the contrary, we use a reranking mechanism that allows our QA model to ignore distractors in adversarial QA and can also provide model- and task-agnostic behavior unlike the commonly used learning-based (re)ranking mechanisms.
In yet another related line of research, (Chen et al., 2016; Kaushik and Lipton, 2018) reveal the simplistic nature and certain important shortcomings of popular QA datasets. Chen et al. (2016) conclude that the simple nature of the questions in the CNN/Daily Mail reading comprehension dataset (Hermann et al., 2015b) allows a QA model to perform well by extracting single-sentence relations. Kaushik and Lipton (2018) perform an extensive study with multiple well-known QA benchmarks to show several troubling trends: basic model ablations, such as making the input question- or passage-only, can beat the state-of-the-art performance, and the answers are often localized in the last few lines, even in very long passages, thus possibly allowing models to achieve very strong performance through learning trivial cues. Although we also question the efficacy of well-known adversarial QA datasets in this work, our core focus is on exposing certain issues specifically with the design of the adversarial distractors rather than the underlying datasets.
3 Approach
Model | Original | AddSent | AddOneSent | ||
Adversarial | Mean | Adversarial | Mean | ||
BERT-S | 89.4/82.1 | 40.9/35.9 | 68.0/61.7 | 54.6/48.4 | 74.1/67.2 |
BERT-S + QAInfoMax | 87.7/82.1 | 41.8/37.2 | 67.5/62.3 | 55.5/49.7 | 73.5/67.8 |
BERT-S + MAARS | 80.2/71.1 | 61.2/53.6 | 71.8/63.4 | 71.3/63.5 | 76.3/67.8 |
Neural QA models are usually trained in a supervised fashion on labeled examples of contexts, questions, and answers to predict answer spans; we represent these as tuples, where represents the sentence and the candidate span. Prior work (Lewis and Fan, 2019; Mudrakarta et al., 2018; Yeh and Chen, 2019; Chen and Durrett, 2019) has noted that the end-to-end paradigm can overfit superficial biases in the data causing learning to stop when simple correlations are sufficient for the model to answer a question confidently. By explicitly enforcing content relevance between the predicted answer-containing sentence and the question, we can combat this poor generalization.
Specifically, we explicitly score the candidate sentences as per the word-level overlap in named entities common to both the question and a sentence. We refer to our method as Model Agnostic Answer Reranking System (MAARS).
Figure 1 illustrates the workflow of MAARS. MAARS can be applied to any arbitrary QA model that predicts answer span probabilities. First, we use the base QA model to compute the best answer spans for a context-question pair where is a hyperparameter. Any answer span not lying in a single sentence is broken into subspans that lie in separate sentences and is updated accordingly.
Next, we extract the set of candidate sentences from the context containing these answer spans. For the question and each sentence, we compute a set of named entity chunks using an open-source AllenNLP (Gardner et al., 2017) NER model. We then compute the set of words inside named entity chunks from each candidate sentence and the question ; note that NER() refers to a set of words and not a set of named entities. Each candidate sentence is then given a score and the answer spans are reranked per the scores of the sentences containing them. In the case of ties or if there are multiple spans in the same candidate sentence, they are reranked among themselves according to the original ordering as per the QA model. Finally, the span with the highest rank after reranking is chosen as the final answer.
Compared to the base QA model, this approach only relies on an additional NER model that can be used without any retraining of the base model. Note that the architecture doesn’t depend on any specific tagger, and the other content matching models like word matching could also be used in the system here.
4 Experiments
Model | Original | AddSent | |
Adv. | Mean | ||
BiDAF | 72.4/62.4 | 21.4/16.0 | 49.9/42.0 |
BiDAF + SLN | 72.3/62.4 | 22.8/17.2 | 50.5/42.5 |
BiDAF + MAARS | 72.3/62.9 | 45.4/38.0 | 60.4/51.9 |
4.1 Evaluation settings
Datasets and baselines.
We evaluate MAARS on two well-known adversarial QA datasets built on top of SQuAD v1.1: Adversarial SQuAD (Jia and Liang, 2017) and Universal Adversarial Triggers (Wallace et al., 2019). For brevity, we don’t include the adversarial distraction generation process for either of the datasets and point the interested reader to the original papers for exact details. For Adversarial SQuAD, we test MAARS with both BiDAF and BERT and compare against state-of-the-art baselines on adversary types used in the original papers. To the best of our knowledge, there is no pre-existing literature that proposes a defense technique for Universal Triggers. We also find that it fails to degrade the performance of our vanilla BERT model, probably because the attacks were originally generated for BiDAF. Thus, we only evaluate on this dataset in the BiDAF setting, using all four triggers Who, When, Where and Why.
For BiDAF, we compare MAARS against the Syntactic Leveraging Network (SLN) by Wu et al. (2019) on AddSent. SLN encodes predicate-argument structures from the context and question, a conceptually similar structure matching approach as MAARS but trained end-to-end with many more parameters. For BERT,
we benchmark MAARS against QAInfoMax (Yeh and Chen, 2019) on AddSent and AddOneSent. In addition to the standard loss for training QA models, QAInfoMax adds a loss to maximize the mutual information between the learned representations of words in context and their neighborhood, and also between those of the answer spans and the question.
Implementation details.
We use the uncased base (single) pretrained BERT from HuggingFace (Wolf et al., 2019) and finetune it using Adam with weight decay (Loshchilov and Hutter, 2019) optimizer and an initial learning rate of on SQuAD (Rajpurkar et al., 2016) v1.1 for 2 epochs for both vanilla BERT and BERT + QAInfoMax. We set the training batch size to 5 and the proportion of linear learning rate warmup for the optimizer to 10%.
Our BiDAF (Seo et al., 2016) model has a hidden state of size 100 and takes 100 dimensional GloVe (Pennington et al., 2014) embeddings as input. For character-level embedding, it uses 100 one-dimensional convolutional filters, each with a width of 5. A uniform dropout (Srivastava et al., 2014) of 0.2 is applied at the CNN layer for character embedding, all LSTM (Hochreiter and Schmidhuber, 1997) layers and at the layer before the logits. We train it with AdaDelta (Zeiler, 2012) and an initial learning rate of 0.5 for 50 epochs. We set the training batch size to 128. For our Syntactic Leveraging Network, we follow the exact hyperparameter settings of (Wu et al., 2019).
Other hyperparameters common to both BERT and BiDAF include an input sequence length of 400, maximum query length of 64, and 40 predicted answer spans per context-question pair. For NER tagging, we use an ELMo-based implementation from AllenNLP (Gardner et al., 2017) that has been finetuned on CoNLL-2003 (Tjong Kim Sang and De Meulder, 2003). Finally, we set the value of (the number of candidates considered for reranking) in MAARS to 10 across all our experiments.
Adv. type | BiDAF | BiDAF + MAARS |
Who | 74.4/67.3 | 76.3/68.9 |
When | 80.1/75.5 | 81.8/77.1 |
Where | 63.5/52.8 | 68.8/56.7 |
Why | 51.9/34.1 | 51.6/34.1 |
.
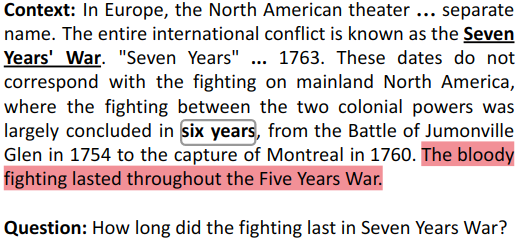
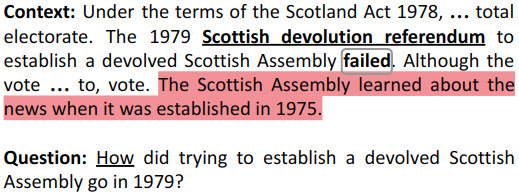
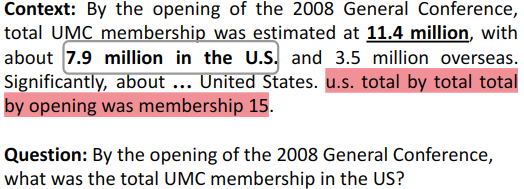
4.2 Results
In all our results tables, we report the macro-averaged F1 and exact match (EM) scores separated by a slash in each cell. In Tables 1 and 2, Original and Adversarial (Adv.) refer to a model’s performance on only clean and only adversarial data respectively. Mean denotes the weighted mean of the Original and Adversarial scores, weighted by the respective number of samples in the dataset. Both AddSent and AddOneSent have 1000 clean and 787 adversarial instances.
Adversarial SQuAD.
Table 1 shows the results with BERT-single (-S) on AddSent and AddOneSent. MAARS outperforms both the vanilla model and QAInfoMax on both Adversarial and Mean metrics. The performance gains are also substantial, especially on Adversarial where MAARS improves F1 over QAInfoMax by about 20 points on AddSent and 16 points on AddOneSent. This clearly shows that our method is much more capable of avoiding distractors in data and it is a much stronger defense technique in this setting. For both QAInfoMax and MAARS there is a drop in performance on clean data, but the drop for MAARS is larger. This drop naturally arises from the simplicity of the heuristic: matching words in named entities with the question sometimes assigns a higher score to a candidate sentence which has a higher overlap in terms of named entities with the question but doesn’t contain the right answer. One such example where MAARS fails to pick the correct top candidate after reranking is shown in fig. 2(a).
Table 2 details the results with BiDAF on .111BiDAF + MAARS gives a similar F1/EM trend on AddOneSent (Adversarial: 46.1/38.5, Mean: 60.8/52.1). Here, we also see significant performance gains over the vanilla model and the SLN baseline. MAARS results in an increase in adversarial F1 by 24 points over vanilla BiDAF and about 22 points over BiDAF + SLN. Interestingly, the performance on clean data doesn’t drop as in the case of BERT. This difference may be a result of BiDAF using more surface word matching itself, leading to a closer alignment between its predictions and the reranker’s choices. However, note that our simple heuristic still performs well even with a complex model like BERT.
Discussion.
Overall, our results on this dataset look promising for both BERT and BiDAF despite our method’s inherent simplicity. This raises two questions. First, how effective is the Adversarial SQuAD dataset as a testbed for adversarial attacks? When a simple method can achieve large gains, we cannot be sure that more complex methods are truly working as advertised rather than learning such heuristics. Second, how effective are these current defenses? They underperform a simple heuristic in this setting; however, because the full breadth of possible adversarial settings has not been explored, it’s hard to get a holistic sense of which methods are effective. Additional settings are needed to fully contrast these techniques.
Universal Adversarial Triggers.
We create a dataset that has purely adversarial instances using the open-source code from Wallace et al. (2019) and present the results in Table 3. In particular, we append the following distractors for different adversary types. The target answers in the distractors have been bolded.
-
•
Who: how ] ] there donald trump ; who who did
-
•
When: ; its time about january 2014 when may did british
-
•
Where: ; : ’ where new york may area where they
-
•
Why: why how ; known because : to kill american people .
Due to unavailability of prior work on trigger-specific defense and BERT-specific triggers, we report only vanilla BiDAF and BiDAF with MAARS. F1 drops by a small amount (0.3 points) from BiDAF to BiDAF with MAARS while the EM score doesn’t change at all for Why. The scores improve by around 1-2 points for the other adversary types. However, the gains are much lower in comparison to Adversarial SQuAD. These results indicate the promise of simple defenses, but more exhaustive evaluation of defenses on different types of attacks is needed to draw a more complete picture of the methods’ generalization abilities.
4.3 Failure cases
Besides the instances where the primary error source is picking a wrong top candidate (refer to Fig. 2(a)), we notice two other common failure case types with MAARS. One directly stems from MAARS’ inability to attend to the question type during reranking. In Fig. 2(b), the question word is How but MAARS picks Scottish devolution referendum which is not the appropriate type of answer here. The other type of failure occurs when multiple similar span types are present in the same candidate, thus creating ambiguity for the base QA model. In the example shown in Fig. 2(c), the QA model fails to distinguish between the two spans and retrieve specific information about the US. Better base QA models may resolve these issues, or a more powerful reranker could also be used. However, rerankers learned end-to-end would suffer from the same issues as BERT and require additional engineering to avoid overfitting the training data.
5 Conclusion
In this work, we introduce a simple and model agnostic post-hoc technique for adversarial question answering (QA) that predicts the final answer after re-ranking candidate answers from a generic QA model as per their overlap in relevant content with the question. Our results show the potential of our method through large performance gains over vanilla models and state-of-the-art methods. We also analyze common failure points in our method. Finally, we reiterate that our main contribution is not the heuristic defense itself but rather its ability to paint a more complete picture of the current state of affairs in adversarial QA. We seek to illustrate that our current adversaries are not strong and generic enough to attack a wide variety of QA methods, and we need a broader evaluation of our defenses to meaningfully gauge our progress in adversarial QA research.
References
- Chen et al. (2016) Danqi Chen, Jason Bolton, and Christopher D. Manning. 2016. A thorough examination of the CNN/daily mail reading comprehension task. In Proceedings of the 54th Annual Meeting of the Association for Computational Linguistics (Volume 1: Long Papers), pages 2358–2367, Berlin, Germany. Association for Computational Linguistics.
- Chen et al. (2017) Danqi Chen, Adam Fisch, Jason Weston, and Antoine Bordes. 2017. Reading Wikipedia to answer open-domain questions. In Proceedings of the 55th Annual Meeting of the Association for Computational Linguistics (Volume 1: Long Papers), pages 1870–1879, Vancouver, Canada. Association for Computational Linguistics.
- Chen and Durrett (2019) Jifan Chen and Greg Durrett. 2019. Understanding dataset design choices for multi-hop reasoning. In Proceedings of the 2019 Conference of the North American Chapter of the Association for Computational Linguistics: Human Language Technologies, Volume 1 (Long and Short Papers), pages 4026–4032, Minneapolis, Minnesota. Association for Computational Linguistics.
- De Cao et al. (2019) Nicola De Cao, Wilker Aziz, and Ivan Titov. 2019. Question answering by reasoning across documents with graph convolutional networks. In Proceedings of the 2019 Conference of the North American Chapter of the Association for Computational Linguistics: Human Language Technologies, Volume 1 (Long and Short Papers), pages 2306–2317, Minneapolis, Minnesota. Association for Computational Linguistics.
- Devlin et al. (2019) Jacob Devlin, Ming-Wei Chang, Kenton Lee, and Kristina Toutanova. 2019. BERT: Pre-training of deep bidirectional transformers for language understanding. In Proceedings of the 2019 Conference of the North American Chapter of the Association for Computational Linguistics: Human Language Technologies, Volume 1 (Long and Short Papers), pages 4171–4186, Minneapolis, Minnesota. Association for Computational Linguistics.
- Gardner et al. (2020) Matt Gardner, Yoav Artzi, Victoria Basmova, Jonathan Berant, Ben Bogin, Sihao Chen, Pradeep Dasigi, Dheeru Dua, Yanai Elazar, Ananth Gottumukkala, et al. 2020. Evaluating nlp models via contrast sets. arXiv preprint arXiv:2004.02709.
- Gardner et al. (2017) Matt Gardner, Joel Grus, Mark Neumann, Oyvind Tafjord, Pradeep Dasigi, Nelson F. Liu, Matthew Peters, Michael Schmitz, and Luke S. Zettlemoyer. 2017. Allennlp: A deep semantic natural language processing platform.
- Hermann et al. (2015a) Karl Moritz Hermann, Tomas Kocisky, Edward Grefenstette, Lasse Espeholt, Will Kay, Mustafa Suleyman, and Phil Blunsom. 2015a. Teaching machines to read and comprehend. In C. Cortes, N. D. Lawrence, D. D. Lee, M. Sugiyama, and R. Garnett, editors, Advances in Neural Information Processing Systems 28, pages 1693–1701. Curran Associates, Inc.
- Hermann et al. (2015b) Karl Moritz Hermann, Tomas Kocisky, Edward Grefenstette, Lasse Espeholt, Will Kay, Mustafa Suleyman, and Phil Blunsom. 2015b. Teaching machines to read and comprehend. In Advances in Neural Information Processing Systems, volume 28, pages 1693–1701. Curran Associates, Inc.
- Hjelm et al. (2019) R Devon Hjelm, Alex Fedorov, Samuel Lavoie-Marchildon, Karan Grewal, Phil Bachman, Adam Trischler, and Yoshua Bengio. 2019. Learning deep representations by mutual information estimation and maximization. In International Conference on Learning Representations.
- Hochreiter and Schmidhuber (1997) Sepp Hochreiter and Jürgen Schmidhuber. 1997. Long short-term memory. Neural Computation, 9(8):1735–1780.
- Jia and Liang (2017) Robin Jia and Percy Liang. 2017. Adversarial examples for evaluating reading comprehension systems. In Proceedings of the 2017 Conference on Empirical Methods in Natural Language Processing, pages 2021–2031, Copenhagen, Denmark. Association for Computational Linguistics.
- Joshi et al. (2017) Mandar Joshi, Eunsol Choi, Daniel Weld, and Luke Zettlemoyer. 2017. TriviaQA: A large scale distantly supervised challenge dataset for reading comprehension. In Proceedings of the 55th Annual Meeting of the Association for Computational Linguistics (Volume 1: Long Papers), pages 1601–1611, Vancouver, Canada. Association for Computational Linguistics.
- Kaushik and Lipton (2018) Divyansh Kaushik and Zachary C. Lipton. 2018. How much reading does reading comprehension require? a critical investigation of popular benchmarks. In Proceedings of the 2018 Conference on Empirical Methods in Natural Language Processing, pages 5010–5015, Brussels, Belgium. Association for Computational Linguistics.
- Khashabi et al. (2020) Daniel Khashabi, Tushar Khot, Ashish Sabharwal, Oyvind Tafjord, Peter Clark, and Hannaneh Hajishirzi. 2020. Unifiedqa: Crossing format boundaries with a single qa system. arXiv preprint arXiv:2005.00700.
- Kratzwald et al. (2019) Bernhard Kratzwald, Anna Eigenmann, and Stefan Feuerriegel. 2019. RankQA: Neural question answering with answer re-ranking. In Proceedings of the 57th Annual Meeting of the Association for Computational Linguistics, pages 6076–6085, Florence, Italy. Association for Computational Linguistics.
- Lee et al. (2018) Jinhyuk Lee, Seongjun Yun, Hyunjae Kim, Miyoung Ko, and Jaewoo Kang. 2018. Ranking paragraphs for improving answer recall in open-domain question answering. In Proceedings of the 2018 Conference on Empirical Methods in Natural Language Processing, pages 565–569, Brussels, Belgium. Association for Computational Linguistics.
- Lee et al. (2019) Seanie Lee, Donggyu Kim, and Jangwon Park. 2019. Domain-agnostic question-answering with adversarial training. In Proceedings of the 2nd Workshop on Machine Reading for Question Answering. Association for Computational Linguistics.
- Lewis and Fan (2019) Mike Lewis and Angela Fan. 2019. Generative question answering: Learning to answer the whole question. In International Conference on Learning Representations.
- Loshchilov and Hutter (2019) Ilya Loshchilov and Frank Hutter. 2019. Decoupled weight decay regularization. In International Conference on Learning Representations.
- Mudrakarta et al. (2018) Pramod Kaushik Mudrakarta, Ankur Taly, Mukund Sundararajan, and Kedar Dhamdhere. 2018. Did the model understand the question? In Proceedings of the 56th Annual Meeting of the Association for Computational Linguistics (Volume 1: Long Papers), pages 1896–1906, Melbourne, Australia. Association for Computational Linguistics.
- Pennington et al. (2014) Jeffrey Pennington, Richard Socher, and Christopher Manning. 2014. Glove: Global vectors for word representation. In Proceedings of the 2014 Conference on Empirical Methods in Natural Language Processing (EMNLP), pages 1532–1543, Doha, Qatar. Association for Computational Linguistics.
- Rajpurkar et al. (2016) Pranav Rajpurkar, Jian Zhang, Konstantin Lopyrev, and Percy Liang. 2016. SQuAD: 100,000+ questions for machine comprehension of text. In Proceedings of the 2016 Conference on Empirical Methods in Natural Language Processing, pages 2383–2392, Austin, Texas. Association for Computational Linguistics.
- Richardson et al. (2013) Matthew Richardson, Christopher J.C. Burges, and Erin Renshaw. 2013. MCTest: A challenge dataset for the open-domain machine comprehension of text. In Proceedings of the 2013 Conference on Empirical Methods in Natural Language Processing, pages 193–203, Seattle, Washington, USA. Association for Computational Linguistics.
- Seo et al. (2016) Min Joon Seo, Aniruddha Kembhavi, Ali Farhadi, and Hannaneh Hajishirzi. 2016. Bidirectional attention flow for machine comprehension. CoRR, abs/1611.01603.
- Severyn and Moschitti (2015) Aliaksei Severyn and Alessandro Moschitti. 2015. Learning to rank short text pairs with convolutional deep neural networks. In Proceedings of the 38th International ACM SIGIR Conference on Research and Development in Information Retrieval, SIGIR ’15, page 373–382, New York, NY, USA. Association for Computing Machinery.
- Srivastava et al. (2014) Nitish Srivastava, Geoffrey Hinton, Alex Krizhevsky, Ilya Sutskever, and Ruslan Salakhutdinov. 2014. Dropout: A simple way to prevent neural networks from overfitting. Journal of Machine Learning Research, 15(56):1929–1958.
- Sukhbaatar et al. (2015) Sainbayar Sukhbaatar, arthur szlam, Jason Weston, and Rob Fergus. 2015. End-to-end memory networks. In C. Cortes, N. D. Lawrence, D. D. Lee, M. Sugiyama, and R. Garnett, editors, Advances in Neural Information Processing Systems 28, pages 2440–2448. Curran Associates, Inc.
- Talmor and Berant (2019) Alon Talmor and Jonathan Berant. 2019. MultiQA: An empirical investigation of generalization and transfer in reading comprehension. In Proceedings of the 57th Annual Meeting of the Association for Computational Linguistics, pages 4911–4921, Florence, Italy. Association for Computational Linguistics.
- Tjong Kim Sang and De Meulder (2003) Erik F. Tjong Kim Sang and Fien De Meulder. 2003. Introduction to the CoNLL-2003 shared task: Language-independent named entity recognition. In Proceedings of the Seventh Conference on Natural Language Learning at HLT-NAACL 2003, pages 142–147.
- Wallace et al. (2019) Eric Wallace, Shi Feng, Nikhil Kandpal, Matt Gardner, and Sameer Singh. 2019. Universal adversarial triggers for attacking and analyzing NLP. In Proceedings of the 2019 Conference on Empirical Methods in Natural Language Processing and the 9th International Joint Conference on Natural Language Processing (EMNLP-IJCNLP), pages 2153–2162, Hong Kong, China. Association for Computational Linguistics.
- Wang et al. (2018) Shuohang Wang, Mo Yu, Jing Jiang, Wei Zhang, Xiaoxiao Guo, Shiyu Chang, Zhiguo Wang, Tim Klinger, Gerald Tesauro, and Murray Campbell. 2018. Evidence aggregation for answer re-ranking in open-domain question answering. In International Conference on Learning Representations.
- Wang and Bansal (2018) Yicheng Wang and Mohit Bansal. 2018. Robust machine comprehension models via adversarial training. In Proceedings of the 2018 Conference of the North American Chapter of the Association for Computational Linguistics: Human Language Technologies, Volume 2 (Short Papers), pages 575–581, New Orleans, Louisiana. Association for Computational Linguistics.
- Welbl et al. (2020) Johannes Welbl, Pasquale Minervini, Max Bartolo, Pontus Stenetorp, and Sebastian Riedel. 2020. Undersensitivity in neural reading comprehension. arXiv preprint arXiv:2003.04808.
- Welbl et al. (2018) Johannes Welbl, Pontus Stenetorp, and Sebastian Riedel. 2018. Constructing datasets for multi-hop reading comprehension across documents. Transactions of the Association for Computational Linguistics, 6:287–302.
- Weston et al. (2015) Jason Weston, Antoine Bordes, Sumit Chopra, and Tomas Mikolov. 2015. Towards ai-complete question answering: A set of prerequisite toy tasks. CoRR, abs/1502.05698.
- Wolf et al. (2019) Thomas Wolf, Lysandre Debut, Victor Sanh, Julien Chaumond, Clement Delangue, Anthony Moi, Pierric Cistac, Tim Rault, R’emi Louf, Morgan Funtowicz, and Jamie Brew. 2019. Huggingface’s transformers: State-of-the-art natural language processing. ArXiv, abs/1910.03771.
- Wu et al. (2019) Bowen Wu, Haoyang Huang, Zongsheng Wang, Qihang Feng, Jingsong Yu, and Baoxun Wang. 2019. Improving the robustness of deep reading comprehension models by leveraging syntax prior. In Proceedings of the 2nd Workshop on Machine Reading for Question Answering, pages 53–57, Hong Kong, China. Association for Computational Linguistics.
- Yang et al. (2019a) Wei Yang, Yuqing Xie, Aileen Lin, Xingyu Li, Luchen Tan, Kun Xiong, Ming Li, and Jimmy Lin. 2019a. End-to-end open-domain question answering with BERTserini. In Proceedings of the 2019 Conference of the North American Chapter of the Association for Computational Linguistics (Demonstrations), pages 72–77, Minneapolis, Minnesota. Association for Computational Linguistics.
- Yang et al. (2019b) Ziqing Yang, Yiming Cui, Wanxiang Che, Ting Liu, Shijin Wang, and Guoping Hu. 2019b. Improving machine reading comprehension via adversarial training. arXiv preprint arXiv:1911.03614.
- Yeh and Chen (2019) Yi-Ting Yeh and Yun-Nung Chen. 2019. QAInfomax: Learning robust question answering system by mutual information maximization. In Proceedings of the 2019 Conference on Empirical Methods in Natural Language Processing and the 9th International Joint Conference on Natural Language Processing (EMNLP-IJCNLP), pages 3370–3375, Hong Kong, China. Association for Computational Linguistics.
- Zeiler (2012) Matthew D. Zeiler. 2012. ADADELTA: an adaptive learning rate method. CoRR, abs/1212.5701.
- Zhou et al. (2019) Mantong Zhou, Minlie Huang, and Xiaoyan Zhu. 2019. Robust reading comprehension with linguistic constraints via posterior regularization. arXiv preprint arXiv:1911.06948.