2School of Computing and Mathematics, Charles Sturt University, NSW, Australia
[email protected], [email protected], [email protected]
Authors’ Instructions
Mental Health and Sensing
Abstract
Mental health is a global epidemic, affecting close to half a billion people worldwide. Chronic shortage of resources hamper detection and recovery of affected people. Effective sensing technologies can help fight the epidemic through early detection, prediction, and resulting proper treatment. Existing and novel technologies for sensing mental health state could address the aforementioned concerns by activating granular tracking of physiological, behavioral, and social signals pertaining to problems in mental health. Our paper focuses on the available methods of sensing mental health problems through direct and indirect measures. We see how active and passive sensing by technologies as well as reporting from relevant sources can contribute toward these detection methods. We also see available methods of therapeutic treatment available through digital means. We highlight a few key intervention technologies that are being developed by researchers to fight against mental illness issues.
Keywords:
Mental health, wearables, sensing.1 Introduction
Mental health can be termed as a concern that is plaguing the entire earth. There is a worrying number of 450 million around the globe that have been diagnosed with some form of mental or neurodevelopmental illnesses [1] and they often lead to various levels of disability [2]. Mental and neurodevelopmental illnesses give rise to a mortality rate that has been compared at a level more than double that of the general population, leading to approximately 8 million deaths [3]. Another impact of such sheer numbers related to these conditions is the financial burden, which generate from expenditure for care as well as the loss in productivity. The numbers related to economic loss has been estimated at more than $400 billion dollars, that only in the United States of America for a year [4].
Well-being of patients suffering from serious mental illness for a sustainable period can be ensured through treatment, management, and care and this can be achieved through granular symptom monitoring [5]. However, existing clinical tools and resources are limited in terms of accessibility and scalability [6]. Mobile health, often termed as mHealth for brevity, is where mobile (or electronic) devices converge with medical professionals and public health administration [7] and has been a well-researched area for exploring the scope of involving qualitative research methods with a view to providing accessible treatment, participant monitoring and retention, and progress of treatment. The growth in the number of mobile devices has also been a significant factor in lending more weight to this type of solutions, since close to 4 billion people around the world own at least one phone( the number is scheduled to double by 2022) [8]. This is remarkable when we consider the fact that studies found more than 70% people suffering from serious mental illness have mobile phones [9]. In addition, an increase of sensors embedded in mobile phones is giving birth to novel possibilities of utilizing these devices into mental health care based on digital evidence, such as quantitative functional and behavioral labels efficiently and without obstacles [10, 11].
What is holding an widespread adoption of sensors in mental health care and management is the scattered and restricted state of evidence that proves the connection between, on one hand, sensor data obtained using wearables and ubiquitous smartphones and, on the other hand, the prevalence and status of mental illnesses [6, 12]. In this paper, we show how technology can help in detection and sensing of mental health problems around the around. Specifically, we focus on the major mental illnesses that are tormenting billions of people across various countries. We see how active and passive sensing by technologies as well as reporting from relevant sources can contribute toward these detection methods. We also see available methods of therapeutic treatment available through digital means. We highlight a few key intervention technologies that are being developed by researchers to fight against mental illness issues.
2 Mental Health Problems
In this section, we do not aim to classify mental health disorders as that is not our target for this paper. Instead, our discussion would revolve around the prevalence of these disorder to provide a sense of their different presentations. Characteristics of major mental disorders include a permutation of irregular and atypical belief, concepts, attitude, and expression especially with people in the surrounding. Top mental disorders are schizophrenia, bipolar conditions (BP), depression and sadness, and developmental disorders together with autism [13]. Table 1 shows the number of people affected by these disorders in the world.
Prevalence: We use the World Health Organization fact sheet [13] to know the prevalence of these illnesses. Depression is a common psychiatric disorder that is a major concern responsible for various forms of disability. Around the world, a population of more than 200 million are affected by depression, majority of whom are women. Schizophrenia can make it difficult for people affected to work or study normally. More tan 40 million humans are affected by BP, which comprises of both manic and depressive periods mingled with regular behavior and mood states. Schizophrenia is another crippling psychiatric disorder that disables around 15 million. Schizophrenia and other psychoses can distort the ability to think, perceive, and express. Another concerning illness that affects more than 50 million worldwide is dementia, which is characterized by a deterioration in cognitive function that exacerbates the regular effects of aging on memory. Lastly, developmental disorders comprise various terms such as intellectual disability and pervasive developmental disorders including autism. 1 in 160 children in the world are estimated to be affected by autism [13].
|
|
||||
---|---|---|---|---|---|
Depression | 264 million | ||||
Bipolar Disorder | 45 million | ||||
Schizophrenia | 20 million | ||||
Dementia | 50 million | ||||
Autism Spectrum Disorder | 50 million |
3 Existing Detection Techniques
In this section, we discuss some of the widely popular methods that are used to detect the prevalence and existence of mental health problems in a person. We first discuss how the wide use of mobile phone technology is helping the revolution of mental health sensing methods. Then we discuss the currently available sensing technologies by dividing these technologies in three categories: biological or physiological sensing, behavioral or soft sensing, and social or proximity sensing methods.
3.1 Usage of Mobile Phones
The wide availability of mobile and sensor technology has enabled the opportunity for personalized data collection efficiently and without obstacles.
Real-world behavior sensing: A popular technique of estimating the status of one’s mental health is to use smartphones sensors to capture behavioral data of humans. We can use a plethora of sensors available in today’s smartphones. The aforementioned sensors can be used in different permutations to capture a wide range of human behavior, including mental and physical. A predecessor to this approach was used along with various sensors to capture and classify data of physical activity [14]. Another similar study was utilized to predict social isolation in older adults using sensors and body microphone [15].
Technology-mediated behavior: More and more work is exploring and analyzing online behavior to predict and care for mental health. For instance, a recent study by de Choudhury et al. demonstrated using information from social media to predict the early signs of depression [16].
3.2 Physiological Signals
-
1.
Facial expression: Facial expressions and associated emotions can convey a person’s emotional states and could be useful in diagnosing psychiatric illnesses [17]. Tron et al. tracked facial Action Units using 3D cameras in people with schizophrenia to differentiate patients from control participants [18]. Another study used a similar range of technology to detect the onset of suicidal thoughts in a person [19]. There are other similar studies [20, 21]. These ideas can be paired with the widespread use of mobile phones to diagnose mental illness.
-
2.
Heart rate variability: People affected with psychiatric conditions suffer from a high chance of facing cardiovascular morbidity compared to the general population [22, 23, 24]. A recent research argued that the reduction in heart rate variability (HRV) could be behind the connection between this heightened cardiac mortality and psychiatric illness [25]. If that is indeed true, then there is a link between HRV and depression and this could be detected through sensing. The same relationship is reported for people with post-traumatic stress disorder [26], anxiety disorders [27], and bipolar disorder and schizophrenia [28]. Traditional HRV measurement devices are heavy; therefore, smart devices can be an apt replacement. Some devices are already in place for this purpose [29].
-
3.
Eye movement: Data captured from fine changes in the movement of eyes can be used to draw conclusion and inferences about mental illness, as shown in research on schizophrenia [30] and depression [31, 32]. A technology called EOG glasses (Electrooculography in Wearable form as in glasses) can be used for detecting the movement of eyes and well as the frequency and pattern of blinking [33]. Additionally, web cameras can also be used for similar purposes to detect the beginning of dementia [34].
-
4.
Electrodermal activity: Electrodermal activity (or EDA for short) can be gauged from measuring changes in electrical properties in human skin [35]. Various research has utilized this technique in mental health detection setting to utilize the proposed relationship between heightened EDA and mental illness [36]. For instance, regarding BP, EDA signals can classify various mood states and subsequent swings [37, 38]. EDA can also be used to determine suicidal tendencies [39, 40].
3.3 Behavioral/Soft Signals
-
1.
Mobility and location: Patterns in our location can be used to predict social activities, which can provide a peek into a person’s mental status. A research tried to link lethargic lifestyle with depression [41], while another linked phone location data to depression severity [42]. There are various such other researches (see [43, 44]).
-
2.
Speech patterns: Characteristics of speech could be used to judge mental health. There is an established relation between depression and human voice [45], as well as speech monotone [46]. A recent research found that jitters in voice is important to establish a pattern that can identify patients having suicidal thoughts [47]. Three other research has done significant work in developing frameworks to collect audio data for similar purposes [48, 49, 50].
-
3.
Technology use: The patterns demonstrated in our day-to-day technology usage contain meaningful data and this can be leveraged in the fight against mental illnesses. For instance, patterns of phone use have been linked with people’s behaviors related to sleeping and waking up, something that has been explored in relation to screen lock information of modern smartphone devices [51]. These features were discovered to be significantly correlated with mood in BP [52].
Other features pertaining to the pattern of usage of smartphone by a person have been found helpful. Two very recent papers explored the connection between change of technology usage and different mood states of BP patients [53, 54]. Schizophrenia patients also found to display similar connection [44], as did patients of depression [43].
-
4.
Activity: While the connection between physical activity and mental state may not seem obvious, research has actually established a strong link between them. For instance, in BP, mania and depression are strongly related with overactivity and under-activity, respectively [55]. This information can be utilized to detect and predict the onset, existence, and duration of either of these mood states in a person. Studies earlier used actigraph to determine level of activity in a person [56, 57]. However, different smartphone sensors could be utilized in order to monitor an user activity level on a continuous basis [58]. Studies in recent years have looked at data of numerous physical activities to connect them with mental disorders line BP [59, 60], depression [42], and schizophrenia [44].
3.4 Social/Proximity Signals
-
1.
Social interaction: Sensing technologies that can capture data from nearby access points as well as other sensing devices are helpful in obtaining information about social interactions without much obstructions. Although not as accurate as user-filled data, these social proximity data can be used as a replacement which can serve to indicate the level of social interactions of a person with the help of a good algorithm [61, 62].
-
2.
Communication patterns: A person’s level of social engagement can be indicated by their communication technology usage data. Communication patterns of humans have been used to identify various mental illnesses such as BP [17] and schizophrenia [63, 44]. They found that symptoms are associated with a change of frequency and duration in outgoing SMS or phone calls.
-
3.
Social media: Since many individuals are comfortable in sharing their daily life stories on different social media channels, these channels can be a rich source of data to determine social engagement, social network characteristics, mood, and emotion that are related to one’s overall well-being. Recent research has worked with Twitter data to predict depression and PTSD [16, 64]. Images and videos in social media posts can be used to collect useful signals about a person. For example, mood [65] and depression [66] can be predicted from sentiment visible in social media photos. This idea has the potential to be scalable in this age of vast social media user engagement.
4 Design Interventions
In this section, we explore some of the available intervention techniques that are associated with digital sensing of mental health problems. There are various methods that are used to assist people in judging and caring for their mental health conditions. These technologies can range from using machine learning methods to detect mental illness to providing customized and personalized feedback or treatment routine. In the end, we discuss two popular methods that seamlessly integrate the sensing, inference, and partial treatment procedures.
If we want to a scientific model of how humans behave from the data that we capture from various wearable and mobile sensing devices, we need to develop one or more algorithms that will serve as a bridge between what we know and what we want to predict. This is useful for medical doctors as well as public health administrators alike to have useful insights about the spread and depth of mental illnesses. While statistical modeling can be used as for this purpose, they may not capture the high level of complexity present in human behavioral data [67]. For this reason, researchers have been opting for machine learning methods [68]. For instance, depression [42, 43] and social rhythm metric [69] has been inferred using smartphone data that was passively acquired. Specifically, deep learning has shown success in determining or predicting about the onset of mental illness [70].
4.1 Summary and Suggestion Display
Usually, there are two approaches for presenting the captured data using wearable and mobile sensors to the user for useful insights and feedback in the mHealth domain. First, we can summarize the collected data into short bursts of information or visual statistics or both (e.g., UbiFit [71] and BeWell [68]). Second, based on the collected data, we can predict a set of present and future states and based on this prediction, we can provide recommendations to the user. While the first method usually allows for the user to set their goal based on the presented statistics, the second method would often set a goal for the user and provide recommendation to reach that goal with minimum effort and maximum efficiency. The issue is that it is difficult to make maximum or even desired use of the complexity present in the capture data. Hence, the feedback or recommendation presented to people are only partially useful and even sometimes partially correct. Even more, because of the lack of insight into the data, the recommendations can even be dangerous sometimes. The good news is that researchers have been exploring different frameworks that would formalize the process of providing feedback and recommendation that would streamline the process and minimize risks. Additionally, researchers have explore variation in feedback in the forms of data change, augmentation, or subtraction to perceive the resulting change in user behavior [72]. Below, we discuss two such frameworks.
4.2 Frameworks for Extracting Useful Information into Recommendations and Feedback
A hierarchical framework extracts data from sensors and extract useful features in two sequential steps. This framework is depicted in Figure 1. The first level at the bottom (in green) has the raw inputs to the sensor device which is often a mobile phone. This data needs to be processed to extract any useful information. The second level (in yellow) is where the system merges statistical algorithms–expert in finding patterns in data– with human intelligence via brainstorming and relevant domain expertise to construct low-level features. The boxes at the top (in blue) combine the middle-level features into behavioral markers by using machine learning and deep learning.
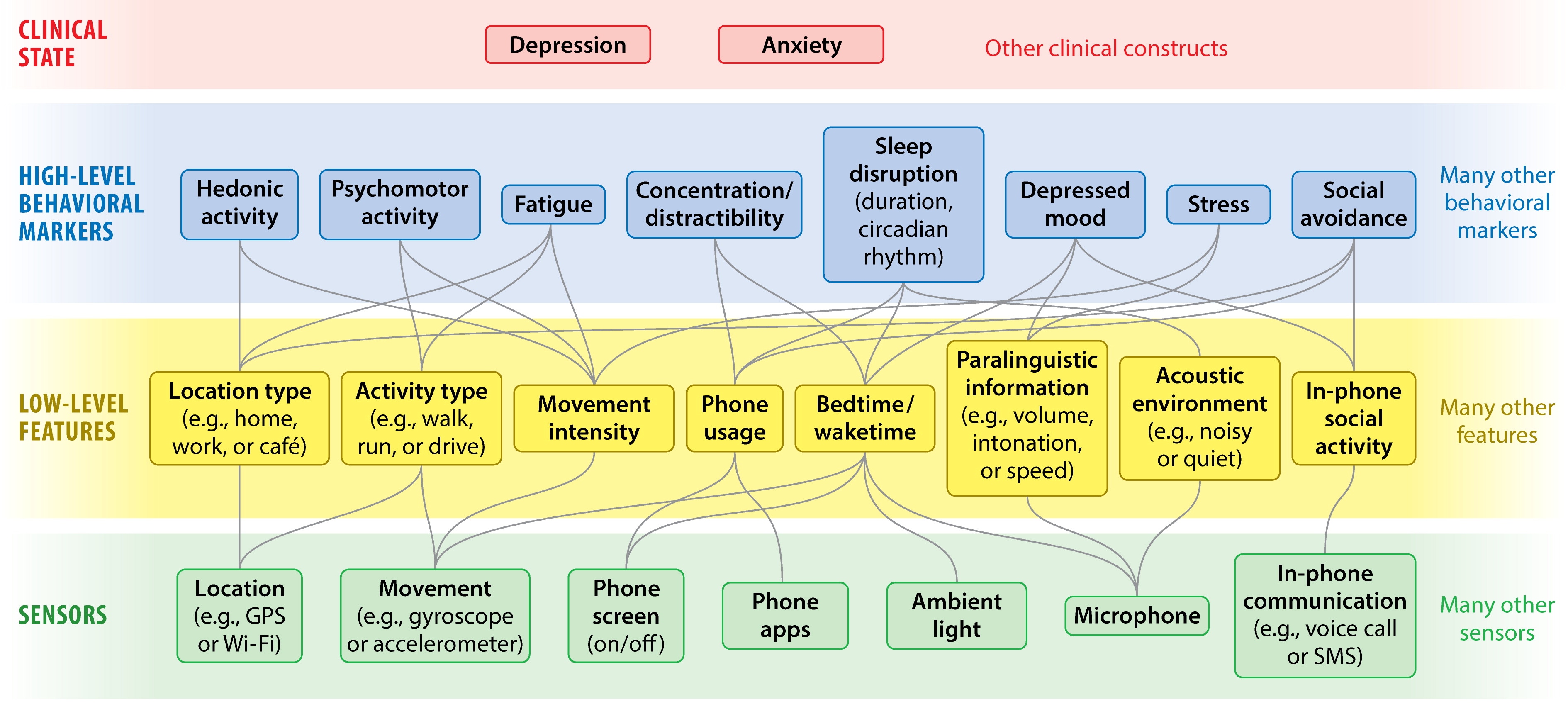
Aung et al. [73] propose a more comprehensive and inclusive three-stage framework for integrating behavior sensing into the domain of mental health. This model can take into account user data from various physical sensors as well as self-reported data. Figure 2 shows the entire process. The first stage takes into account data from sensors as well as self-reported procedures over a time to become more inclusive. Then, this raw data is processed using machine learning or other methods to create new features and gain useful insights into the data. The final phase works on integrating the inferences from the middle step toward the management of the condition if detected. This could include using the inferences alongside traditional mental health therapies to create personalized digital interventions and chrono-therapeutic interventions.
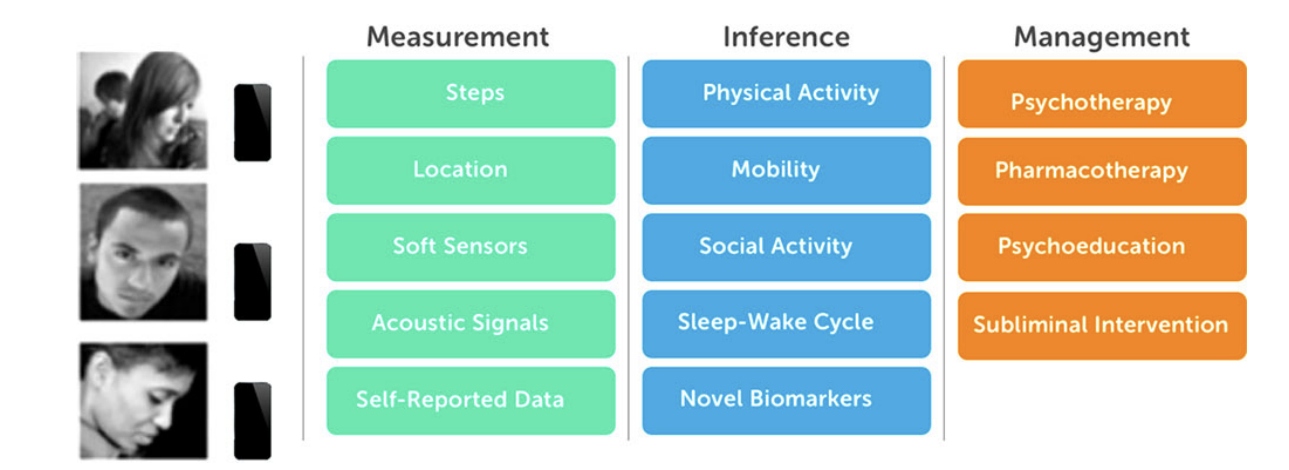
5 Discussion
The existing technologies for sensing mental health condition suffer from a number of limitations. First, studies do not demonstrate a significant level of effectiveness for and correlation with improving patient detection and care for mental health patients. The dearth of clinical evidence can be linked back to the insufficient amount of research, lack of significant sample size or population, an insignificant time over which studies were live (both in short term and medium term), as well as a lack of funding opportunities, which can again lead to a lack of awareness regarding the severity and spread of these problems in different societies. These issues can be addressed by improving the study design and procedures and seeking more funding [6]. Increasing awareness about mental health problems is another wing of solving these issues.
Second, not many studies strive to combine the effects of various types of sensing. If data is being collected about a single patient or the same group of patients and the result inferred from these sources are consistent, then the system could be more confident about its inference. The challenge of combining data from different streams is chiefly computational, where machine learning systems could provide to be a helping hand. However, machine learning methods also suffer from the lack of reproducibility [74]. These techniques used for mental health sensing suffer from a lack of expiration date as well as the curse of variability [11]. Additionally, how the errors associated with the predictions of a machine learning system will be explained, addressed, and incorporated in the future iterations of the same model is something that needs more significant research, which is presently absent. Such user-facing errors need to be addressed properly and with a convincing methodology, lest we should face affecting the quality of the experience of people using technology for sensing..
Third, the nature of sensing technologies dictate that they will need to obtain a gigantic amount of data that is related to personal use and has a potential to sensitive in nature (especially when the data is related to personal behavior or health problems). Furthermore, wearable sensing technologies are not advanced enough to differentiate between target participant and non-participants and hence, due to the availability and ease of use in any setting, can risk obtaining unexpected, irrelevant, and unnecessary data [75] that can lead to false diagnosis, false triggers, and lack of care for patients. Researchers should strive to identify potentially sensitive data items to deploy a plan of action of protecting this data from malicious parties. In addition, researchers should also think about how they can identify unexpected, irrelevant, and unnecessary data and separate valuable data from all the clutter.
Fourth, related to the previous concern is another that is the issue of privacy and security for passively collected data. Unfortunately, the existing research community members are in a disagreement regarding what to do about this risk [76]. A crucial aspect that may often get overlooked is the difficulty in stripping identifying information from data collected by sensors in mobile phone and wearables. Even seeming innocuous data such as location information [77], when obtained in sufficient quality, can expose the identification of a victim, exposing to a risk of facing social stigma [78]. While there are numerous existing techniques to help de-identify data (especially location data), they are not full-proof in preserving privacy as well as usefulness of data at the same time [79].
While an exponentially increasing amount of data is being captured from mobile and wearable sensors around the world, there are other, less structured challenges that can pose significant barriers toward capturing and treating mental illness using mobile devices. In Global South, mental health is a vastly neglected domain rife with misconceptions, maltreatment, and lack of treatment options [80]. Sometimes, people consult the local witches instead of medical doctors to treat illnesses that seem unusual to the community (like mental illness) [81]. Rural areas especially suffer from the lack of medical infrastructure and mental health facilities [82] and if we plan to offset this restriction by employing mobile devices, we face another different set of challenges [75, 83]. Furthermore, the low rate of literacy is often a hurdle for people to use smartphones [84]. These challenges should also be considered along with the more “high-tech” obstacles described above if we want to capture a holistic picture of the domain where we want to treat and manage psychiatric illnesses with sensors.
References
- Brundtland [2001] Brundtland GH (2001) Mental health: new understanding, new hope. Jama 286(19):2391–2391
- Organization et al [2003] Organization WH, et al (2003) Investing in mental health. WHO p 36
- Walker et al [2015] Walker ER, McGee RE, Druss BG (2015) Mortality in mental disorders and global disease burden implications: a systematic review and meta-analysis. JAMA psychiatry 72(4):334–341
- Insel [2015] Insel T (2015) Mental health awareness month: By the numbers. National Institute of Mental Health blog
- Morriss et al [2013] Morriss R, Vinjamuri I, Faizal MA, Bolton CA, McCarthy JP (2013) Training to recognize the early signs of recurrence in schizophrenia. Schizophrenia bulletin 39(2):255
- Abdullah and Choudhury [2018] Abdullah S, Choudhury T (2018) Sensing technologies for monitoring serious mental illnesses. IEEE MultiMedia 25(1):61–75
- Adibi [2015] Adibi S (2015) Mobile health: a technology road map, vol 5. Springer
- Cerwall et al [2015] Cerwall P, Jonsson P, Möller R, Bävertoft S, Carson S, Godor I (2015) Ericsson mobility report. On the Pulse of the Networked Society Hg v Ericsson
- Ben-Zeev et al [2013] Ben-Zeev D, Davis KE, Kaiser S, Krzsos I, Drake RE (2013) Mobile technologies among people with serious mental illness: opportunities for future services. Administration and Policy in Mental Health and Mental Health Services Research 40(4):340–343
- Gaggioli and Riva [2013] Gaggioli A, Riva G (2013) From mobile mental health to mobile wellbeing: opportunities and challenges. In: MMVR, pp 141–147
- Mohr et al [2017] Mohr DC, Zhang M, Schueller SM (2017) Personal sensing: understanding mental health using ubiquitous sensors and machine learning. Annual review of clinical psychology 13:23–47
- Or et al [2017] Or F, Torous J, Onnela JP (2017) High potential but limited evidence: Using voice data from smartphones to monitor and diagnose mood disorders. Psychiatric rehabilitation journal 40(3):320
- WHO [2019] WHO (2019) Mental disorders by world health organization. https://www.who.int/news-room/fact-sheets/detail/mental-disorders, accessed: 2019-12-24
- Lester et al [2006] Lester J, Choudhury T, Borriello G (2006) A practical approach to recognizing physical activities. In: International conference on pervasive computing, Springer, pp 1–16
- Rabbi et al [2011] Rabbi M, Ali S, Choudhury T, Berke E (2011) Passive and in-situ assessment of mental and physical well-being using mobile sensors. In: Proceedings of the 13th international conference on Ubiquitous computing, ACM, pp 385–394
- De Choudhury et al [2013] De Choudhury M, Gamon M, Counts S, Horvitz E (2013) Predicting depression via social media. In: Seventh international AAAI conference on weblogs and social media
- Gur et al [2006] Gur RE, Kohler CG, Ragland JD, Siegel SJ, Lesko K, Bilker WB, Gur RC (2006) Flat affect in schizophrenia: relation to emotion processing and neurocognitive measures. Schizophrenia bulletin 32(2):279–287
- Tron et al [2015] Tron T, Peled A, Grinsphoon A, Weinshall D (2015) Automated facial expressions analysis in schizophrenia: A continuous dynamic approach. In: International Symposium on Pervasive Computing Paradigms for Mental Health, Springer, pp 72–81
- Laksana et al [2017] Laksana E, Baltrušaitis T, Morency LP, Pestian JP (2017) Investigating facial behavior indicators of suicidal ideation. In: 2017 12th IEEE International Conference on Automatic Face & Gesture Recognition (FG 2017), IEEE, pp 770–777
- Valstar et al [2014] Valstar M, Schuller B, Smith K, Almaev T, Eyben F, Krajewski J, Cowie R, Pantic M (2014) Avec 2014: 3d dimensional affect and depression recognition challenge. In: Proceedings of the 4th International Workshop on Audio/Visual Emotion Challenge, ACM, pp 3–10
- Wang et al [2015] Wang R, Campbell AT, Zhou X (2015) Using opportunistic face logging from smartphone to infer mental health: challenges and future directions. In: Adjunct Proceedings of the 2015 ACM International Joint Conference on Pervasive and Ubiquitous Computing and Proceedings of the 2015 ACM International Symposium on Wearable Computers, ACM, pp 683–692
- Hennekens et al [2005] Hennekens CH, Hennekens AR, Hollar D, Casey DE (2005) Schizophrenia and increased risks of cardiovascular disease. American heart journal 150(6):1115–1121
- Weiner et al [2011] Weiner M, Warren L, Fiedorowicz JG (2011) Cardiovascular morbidity and mortality in bipolar disorder. Annals of clinical psychiatry: official journal of the American Academy of Clinical Psychiatrists 23(1):40
- Pratt et al [1996] Pratt LA, Ford DE, Crum RM, Armenian HK, Gallo JJ, Eaton WW (1996) Depression, psychotropic medication, and risk of myocardial infarction: prospective data from the baltimore eca follow-up. Circulation 94(12):3123–3129
- Kemp et al [2010] Kemp AH, Quintana DS, Gray MA, Felmingham KL, Brown K, Gatt JM (2010) Impact of depression and antidepressant treatment on heart rate variability: a review and meta-analysis. Biological psychiatry 67(11):1067–1074
- Tan et al [2011] Tan G, Dao TK, Farmer L, Sutherland RJ, Gevirtz R (2011) Heart rate variability (hrv) and posttraumatic stress disorder (ptsd): a pilot study. Applied psychophysiology and biofeedback 36(1):27–35
- Chalmers et al [2014] Chalmers JA, Quintana DS, Abbott MJ, Kemp AH, et al (2014) Anxiety disorders are associated with reduced heart rate variability: a meta-analysis. Frontiers in psychiatry 5:80
- Quintana et al [2016] Quintana DS, Westlye LT, Kaufmann T, Rustan Ø, Brandt CL, Haatveit B, Steen NE, Andreassen OA (2016) Reduced heart rate variability in schizophrenia and bipolar disorder compared to healthy controls. Acta Psychiatrica Scandinavica 133(1):44–52
- Bai et al [2018] Bai Y, Hibbing P, Mantis C, Welk GJ (2018) Comparative evaluation of heart rate-based monitors: Apple watch vs fitbit charge hr. Journal of sports sciences 36(15):1734–1741
- Levy et al [2010] Levy DL, Sereno AB, Gooding DC, O’Driscoll GA (2010) Eye tracking dysfunction in schizophrenia: characterization and pathophysiology. In: Behavioral Neurobiology of Schizophrenia and Its Treatment, Springer, pp 311–347
- Winograd-Gurvich et al [2006] Winograd-Gurvich C, Georgiou-Karistianis N, Fitzgerald PB, Millist L, White OB (2006) Ocular motor differences between melancholic and non-melancholic depression. Journal of affective disorders 93(1-3):193–203
- Alghowinem et al [2013] Alghowinem S, Goecke R, Wagner M, Parker G, Breakspear M (2013) Eye movement analysis for depression detection. In: 2013 IEEE International Conference on Image Processing, IEEE, pp 4220–4224
- Dhuliawala et al [2016] Dhuliawala M, Lee J, Shimizu J, Bulling A, Kunze K, Starner T, Woo W (2016) Smooth eye movement interaction using eog glasses. In: Proceedings of the 18th ACM International Conference on Multimodal Interaction, ACM, pp 307–311
- Cano et al [2017] Cano LAM, Beltrán J, Navarro R, García-Vázquez MS, Castro LA (2017) Towards early dementia detection by oculomotor performance analysis on leisure web content. In: Proceedings of the 2017 ACM International Joint Conference on Pervasive and Ubiquitous Computing and Proceedings of the 2017 ACM International Symposium on Wearable Computers, ACM, pp 800–804
- Critchley [2002] Critchley HD (2002) Electrodermal responses: what happens in the brain. The Neuroscientist 8(2):132–142
- Schell et al [2005] Schell AM, Dawson ME, Rissling A, Ventura J, Subotnik KL, Gitlin MJ, Nuechterlein KH (2005) Electrodermal predictors of functional outcome and negative symptoms in schizophrenia. Psychophysiology 42(4):483–492
- Lanata et al [2014] Lanata A, Greco A, Valenza G, Scilingo EP (2014) A pattern recognition approach based on electrodermal response for pathological mood identification in bipolar disorders. In: 2014 IEEE International Conference on Acoustics, Speech and Signal Processing (ICASSP), IEEE, pp 3601–3605
- Greco et al [2014] Greco A, Valenza G, Lanata A, Rota G, Scilingo EP (2014) Electrodermal activity in bipolar patients during affective elicitation. IEEE journal of biomedical and health informatics 18(6):1865–1873
- Jandl et al [2010] Jandl M, Steyer J, Kaschka WP (2010) Suicide risk markers in major depressive disorder: a study of electrodermal activity and event-related potentials. Journal of Affective Disorders 123(1-3):138–149
- Thorell et al [2013] Thorell LH, Wolfersdorf M, Straub R, Steyer J, Hodgkinson S, Kaschka W, Jandl M (2013) Electrodermal hyporeactivity as a trait marker for suicidal propensity in uni-and bipolar depression. Journal of psychiatric research 47(12):1925–1931
- Roshanaei-Moghaddam et al [2009] Roshanaei-Moghaddam B, Katon WJ, Russo J (2009) The longitudinal effects of depression on physical activity. General hospital psychiatry 31(4):306–315
- Canzian and Musolesi [2015] Canzian L, Musolesi M (2015) Trajectories of depression: unobtrusive monitoring of depressive states by means of smartphone mobility traces analysis. In: Proceedings of the 2015 ACM international joint conference on pervasive and ubiquitous computing, ACM, pp 1293–1304
- Saeb et al [2015] Saeb S, Zhang M, Karr CJ, Schueller SM, Corden ME, Kording KP, Mohr DC (2015) Mobile phone sensor correlates of depressive symptom severity in daily-life behavior: an exploratory study. Journal of medical Internet research 17(7):e175
- Wang et al [2016] Wang R, Aung MS, Abdullah S, Brian R, Campbell AT, Choudhury T, Hauser M, Kane J, Merrill M, Scherer EA, et al (2016) Crosscheck: toward passive sensing and detection of mental health changes in people with schizophrenia. In: Proceedings of the 2016 ACM International Joint Conference on Pervasive and Ubiquitous Computing, ACM, pp 886–897
- Nilsonne [1987] Nilsonne Å (1987) Acoustic analysis of speech variables during depression and after improvement. Acta Psychiatrica Scandinavica 76(3):235–245
- Moore et al [2004] Moore E, Clements M, Peifer J, Weisser L (2004) Comparing objective feature statistics of speech for classifying clinical depression. In: The 26th Annual International Conference of the IEEE Engineering in Medicine and Biology Society, IEEE, vol 1, pp 17–20
- Ozdas et al [2004] Ozdas A, Shiavi RG, Silverman SE, Silverman MK, Wilkes DM (2004) Investigation of vocal jitter and glottal flow spectrum as possible cues for depression and near-term suicidal risk. IEEE Transactions on Biomedical Engineering 51(9):1530–1540
- Faurholt-Jepsen et al [2016] Faurholt-Jepsen M, Munkholm K, Frost M, Bardram JE, Kessing LV (2016) Electronic self-monitoring of mood using it platforms in adult patients with bipolar disorder: a systematic review of the validity and evidence. BMC psychiatry 16(1):7
- Lu et al [2012] Lu H, Frauendorfer D, Rabbi M, Mast MS, Chittaranjan GT, Campbell AT, Gatica-Perez D, Choudhury T (2012) Stresssense: Detecting stress in unconstrained acoustic environments using smartphones. In: Proceedings of the 2012 ACM Conference on Ubiquitous Computing, ACM, pp 351–360
- Muaremi et al [2014] Muaremi A, Gravenhorst F, Grünerbl A, Arnrich B, Tröster G (2014) Assessing bipolar episodes using speech cues derived from phone calls. In: International Symposium on Pervasive Computing Paradigms for Mental Health, Springer, pp 103–114
- Abdullah et al [2014] Abdullah S, Matthews M, Murnane EL, Gay G, Choudhury T (2014) Towards circadian computing: early to bed and early to rise makes some of us unhealthy and sleep deprived. In: Proceedings of the 2014 ACM international joint conference on pervasive and ubiquitous computing, ACM, pp 673–684
- Frost et al [2013] Frost M, Doryab A, Faurholt-Jepsen M, Kessing LV, Bardram JE (2013) Supporting disease insight through data analysis: refinements of the monarca self-assessment system. In: Proceedings of the 2013 ACM international joint conference on Pervasive and ubiquitous computing, ACM, pp 133–142
- Matthews et al [2017] Matthews M, Murnane E, Snyder J, Guha S, Chang P, Doherty G, Gay G (2017) The double-edged sword: A mixed methods study of the interplay between bipolar disorder and technology use. Computers in Human Behavior 75:288–300
- Alvarez-Lozano et al [2014] Alvarez-Lozano J, Osmani V, Mayora O, Frost M, Bardram J, Faurholt-Jepsen M, Kessing LV (2014) Tell me your apps and i will tell you your mood: correlation of apps usage with bipolar disorder state. In: Proceedings of the 7th International Conference on PErvasive Technologies Related to Assistive Environments, ACM, p 19
- Wolff et al [1985] Wolff EA, Putnam FW, Post RM (1985) Motor activity and affective illness: the relationship of amplitude and temporal distribution to changes in affective state. Archives of General Psychiatry 42(3):288–294
- Walther et al [2015] Walther S, Stegmayer K, Horn H, Razavi N, Müller TJ, Strik W (2015) Physical activity in schizophrenia is higher in the first episode than in subsequent ones. Frontiers in psychiatry 5:191
- John and Freedson [2012] John D, Freedson P (2012) Actigraph and actical physical activity monitors: a peek under the hood. Medicine and science in sports and exercise 44(1 Suppl 1):S86
- Wu et al [2012] Wu W, Dasgupta S, Ramirez EE, Peterson C, Norman GJ (2012) Classification accuracies of physical activities using smartphone motion sensors. Journal of medical Internet research 14(5):e130
- Osmani et al [2013] Osmani V, Maxhuni A, Grünerbl A, Lukowicz P, Haring C, Mayora O (2013) Monitoring activity of patients with bipolar disorder using smart phones. In: Proceedings of International Conference on Advances in Mobile Computing & Multimedia, ACM, p 85
- Beiwinkel et al [2016] Beiwinkel T, Kindermann S, Maier A, Kerl C, Moock J, Barbian G, Rössler W (2016) Using smartphones to monitor bipolar disorder symptoms: a pilot study. JMIR mental health 3(1):e2
- Aharony et al [2011] Aharony N, Pan W, Ip C, Khayal I, Pentland A (2011) Social fmri: Investigating and shaping social mechanisms in the real world. Pervasive and Mobile Computing 7(6):643–659
- Ben-Zeev et al [2015] Ben-Zeev D, Wang R, Abdullah S, Brian R, Scherer EA, Mistler LA, Hauser M, Kane JM, Campbell A, Choudhury T (2015) Mobile behavioral sensing for outpatients and inpatients with schizophrenia. Psychiatric services 67(5):558–561
- Faurholt-Jepsen et al [2016] Faurholt-Jepsen M, Vinberg M, Frost M, Debel S, Margrethe Christensen E, Bardram JE, Kessing LV (2016) Behavioral activities collected through smartphones and the association with illness activity in bipolar disorder. International journal of methods in psychiatric research 25(4):309–323
- Reece et al [2017] Reece AG, Reagan AJ, Lix KL, Dodds PS, Danforth CM, Langer EJ (2017) Forecasting the onset and course of mental illness with twitter data. Scientific reports 7(1):13,006
- Abdullah et al [2015] Abdullah S, Murnane EL, Costa JM, Choudhury T (2015) Collective smile: Measuring societal happiness from geolocated images. In: Proceedings of the 18th ACM Conference on Computer Supported Cooperative Work & Social Computing, ACM, pp 361–374
- Reece and Danforth [2017] Reece AG, Danforth CM (2017) Instagram photos reveal predictive markers of depression. EPJ Data Science 6(1):15
- Breiman [2000] Breiman L (2000) Randomizing outputs to increase prediction accuracy. Machine Learning 40(3):229–242
- Lane et al [2011] Lane ND, Mohammod M, Lin M, Yang X, Lu H, Ali S, Doryab A, Berke E, Choudhury T, Campbell A (2011) Bewell: A smartphone application to monitor, model and promote wellbeing. In: 5th international ICST conference on pervasive computing technologies for healthcare, pp 23–26
- Abdullah et al [2016] Abdullah S, Matthews M, Frank E, Doherty G, Gay G, Choudhury T (2016) Automatic detection of social rhythms in bipolar disorder. Journal of the American Medical Informatics Association 23(3):538–543
- Martínez and Yannakakis [2014] Martínez HP, Yannakakis GN (2014) Deep multimodal fusion: Combining discrete events and continuous signals. In: Proceedings of the 16th International conference on multimodal interaction, ACM, pp 34–41
- Consolvo et al [2008] Consolvo S, McDonald DW, Toscos T, Chen MY, Froehlich J, Harrison B, Klasnja P, LaMarca A, LeGrand L, Libby R, et al (2008) Activity sensing in the wild: a field trial of ubifit garden. In: Proceedings of the SIGCHI conference on human factors in computing systems, ACM, pp 1797–1806
- Costa et al [2016] Costa J, Adams AT, Jung MF, Guimbretière F, Choudhury T (2016) Emotioncheck: leveraging bodily signals and false feedback to regulate our emotions. In: Proceedings of the 2016 ACM International Joint Conference on Pervasive and Ubiquitous Computing, ACM, pp 758–769
- Aung et al [2017] Aung MH, Matthews M, Choudhury T (2017) Sensing behavioral symptoms of mental health and delivering personalized interventions using mobile technologies. Depression and anxiety 34(7):603–609
- Asselbergs et al [2016] Asselbergs J, Ruwaard J, Ejdys M, Schrader N, Sijbrandij M, Riper H (2016) Mobile phone-based unobtrusive ecological momentary assessment of day-to-day mood: an explorative study. Journal of medical Internet research 18(3):e72
- Ahmed et al [2017] Ahmed SI, Haque MR, Chen J, Dell N (2017) Digital privacy challenges with shared mobile phone use in bangladesh. Proceedings of the ACM on Human-Computer Interaction 1(CSCW):1–20
- Shilton and Sayles [2016] Shilton K, Sayles S (2016) ” we aren’t all going to be on the same page about ethics”: Ethical practices and challenges in research on digital and social media. In: 2016 49th Hawaii International Conference on System Sciences (HICSS), IEEE, pp 1909–1918
- De Montjoye et al [2013] De Montjoye YA, Hidalgo CA, Verleysen M, Blondel VD (2013) Unique in the crowd: The privacy bounds of human mobility. Scientific reports 3:1376
- Sweeney [2000] Sweeney L (2000) Simple demographics often identify people uniquely. Health (San Francisco) 671:1–34
- Brush et al [2010] Brush A, Krumm J, Scott J (2010) Exploring end user preferences for location obfuscation, location-based services, and the value of location. In: Proceedings of the 12th ACM international conference on Ubiquitous computing, ACM, pp 95–104
- Tushar et al [2020] Tushar AK, Antara IJ, Das D, Chandra P, Soron TR, Haque MM, Ahamed SI, Ahmed SI (2020) “we need more power to stand up”: Designing to combat stigmatization of the caregivers of children with autism in urban bangladesh. Proceedings of the 11th International Conference on Information and Communication Technologies and Development p to appear
- Sultana and Ahmed [2019] Sultana S, Ahmed SI (2019) Witchcraft and hci: Morality, modernity, and postcolonial computing in rural bangladesh. In: Proceedings of the 2019 CHI Conference on Human Factors in Computing Systems, pp 1–15
- Sultana et al [2019] Sultana S, Ahmed SI, Fussell SR (2019) ” parar-daktar understands my problems better” disentangling the challenges to designing better access to healthcare in rural bangladesh. Proceedings of the ACM on Human-Computer Interaction 3(CSCW):1–27
- Ahmed et al [2017] Ahmed SI, Haque MR, Guha S, Rifat MR, Dell N (2017) Privacy, security, and surveillance in the global south: A study of biometric mobile sim registration in bangladesh. In: Proceedings of the 2017 CHI Conference on Human Factors in Computing Systems, pp 906–918
- Ahmed et al [2013] Ahmed SI, Jackson SJ, Zaber M, Morshed MB, Ismail MHB, Afrose S (2013) Ecologies of use and design: individual and social practices of mobile phone use within low-literate rickshawpuller communities in urban bangladesh. In: Proceedings of the 4th Annual Symposium on Computing for Development, pp 1–10