MELM: Data Augmentation with Masked Entity
Language Modeling for Low-Resource NER
Abstract
Data augmentation is an effective solution to data scarcity in low-resource scenarios. However, when applied to token-level tasks such as NER, data augmentation methods often suffer from token-label misalignment, which leads to unsatsifactory performance. In this work, we propose Masked Entity Language Modeling (MELM) as a novel data augmentation framework for low-resource NER. To alleviate the token-label misalignment issue, we explicitly inject NER labels into sentence context, and thus the fine-tuned MELM is able to predict masked entity tokens by explicitly conditioning on their labels. Thereby, MELM generates high-quality augmented data with novel entities, which provides rich entity regularity knowledge and boosts NER performance. When training data from multiple languages are available, we also integrate MELM with code-mixing for further improvement. We demonstrate the effectiveness of MELM on monolingual, cross-lingual and multilingual NER across various low-resource levels. Experimental results show that our MELM presents substantial improvement over the baseline methods.111Our code is available at https://github.com/RandyZhouRan/MELM/.
1 Introduction
Named entity recognition (NER) is a fundamental NLP task which aims to locate named entity mentions and classify them into predefined categories. As a subtask of information extraction, it serves as a key building block for information retrieval (Banerjee et al., 2019), question answering (Fabbri et al., 2020) and text summarization systems (Nallapati et al., 2016) etc. However, except a few high-resource languages / domains, the majority of languages / domains have limited amount of labeled data.
Since manually annotating sufficient labeled data for each language / domain is expensive, low-resource NER (Cotterell and Duh, 2017; Feng et al., 2018; Zhou et al., 2019; Rijhwani et al., 2020) has received increasing attention in the research community over the past years. As an effective solution to data scarcity in low-resource scenarios, data augmentation enlarges the training set by applying label-preserving transformations. Typical data augmentation methods for NLP include (1) word-level modification (Wei and Zou, 2019; Kobayashi, 2018; Wu et al., 2019; Kumar et al., 2020) and (2) back-translation (Sennrich et al., 2016; Fadaee et al., 2017; Dong et al., 2017; Yu et al., 2018).
Despite the effectiveness on sentence-level tasks, they suffer from the token-label misalignment issue when applied to token-level tasks like NER. More specifically, word-level modification might replace an entity with alternatives that mismatch the original label. Back-translation creates augmented texts that largely preserve the original content. However, it hinges on external word alignment tools for propagating the labels from the original input to the augmented text, which has proved to be error-prone.
To apply data augmentation on token-level tasks, Dai and Adel (2020) proposed to randomly substitute entity mentions with existing entities of the same class. They avoided the token-label misalignment issue but the entity diversity does not increase. Besides, the substituted entity might not fit into the original context. Li et al. (2020a) avoided the token-label misalignment issue by only diversifying the context, where they replaced context (having ‘O’ label) tokens using MASS (Song et al., 2019) and left the entities (i.e. aspect terms in their task) completely unchanged. However, according to the NER evaluations in Lin et al. (2020), augmentation on context gave marginal improvement on pretrained-LM-based NER models.
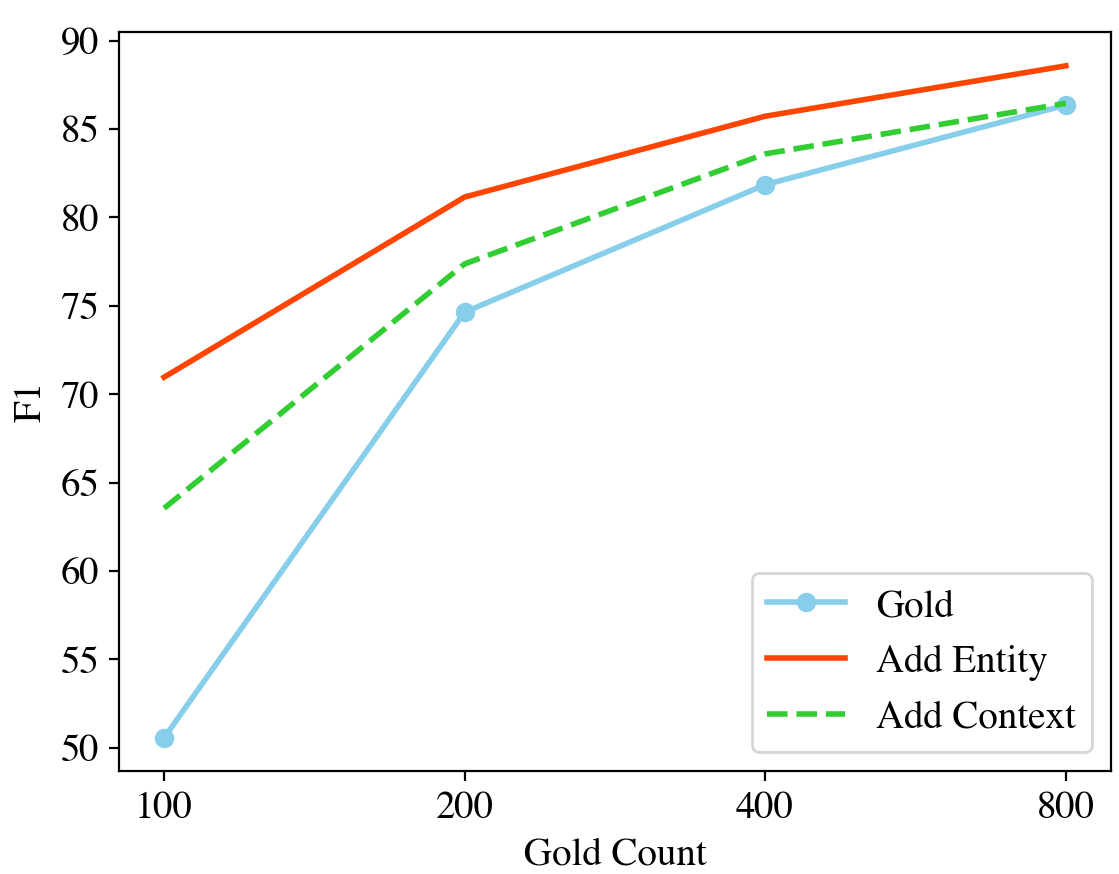
Our preliminary results on low-resource NER (see Figure 1) also demonstrate that diversifying entities in the training data is more effective than introducing more context patterns. Inspired by the aforementioned observations, we propose Masked Entity Language Modeling (MELM) as a data augmentation framework for low-resource NER, which generates augmented data with diverse entities while alleviating the challenge of token-label misalignment. MELM is built upon pretrained Masked Language Models (MLM), and it is further fine-tuned on corrupted training sentences with only entity tokens being randomly masked to facilitate entity-oriented token replacement. Simply masking and replacing entity tokens using the finetuned MLM is still insufficient because the predicted entity might not align with the original label. Taking the sentence shown in Figure 2(b) as an example, after masking the named entity “European Union” (Organization), the finetuned MLM could predict it as “Washington has”. Such prediction fits the context but it is not aligned with the original labels. To alleviate the misalignment, our MELM additionally introduces a labeled sequence linearization strategy, which respectively inserts one label token before and after each entity token and regards the inserted label tokens as the normal context tokens during masked language modeling. Therefore, the prediction of the masked token is conditioned on not only the context but the entity’s label as well.
After injecting label information and finetuning on the label-enhanced NER data, our MELM can exploit rich knowledge from pre-training to increase entity diversity while greatly reducing token-label misalignment. Code-mixing (Singh et al., 2019; Qin et al., 2020; Zhang et al., 2021) achieved promising results by creating additional code-mixed samples using the available multilingual training sets, which is particularly beneficial when the training data of each language is scarce. Fortunately, in the scenarios of multilingual low-resource NER, our MELM can also be applied on the code-mixed examples for further performance gains. We first apply code-mixing by replacing entities in a source language sentence with the same type entities of a foreign language. However, even though token-label alignment is guaranteed by replacing with entities of the same type, the candidate entity might not best fit into the original context (for example, replacing a government department with a football club). To solve this problem, we propose an entity similarity search algorithm based on bilingual embedding to retrieve the most semantically similar entity from the training entities in other languages. Finally, after adding language markers to the code-mixed data, we use them to fine-tune MELM for generating more code-mixed augmented data.
To summarize, the main contributions of this paper are as follows: (1) we present a novel framework which jointly exploits sentence context and entity labels for entity-based data augmentation. It consistently achieves substantial improvement when evaluated on monolingual, cross-lingual, and multilingual low-resource NER; (2) the proposed labeled sequence linearization strategy effectively alleviates the problem of token-label misalignment during augmentation; (3) an entity similarity search algorithm is developed to better bridge entity-based data augmentation and code-mixing in multilingual scenarios.

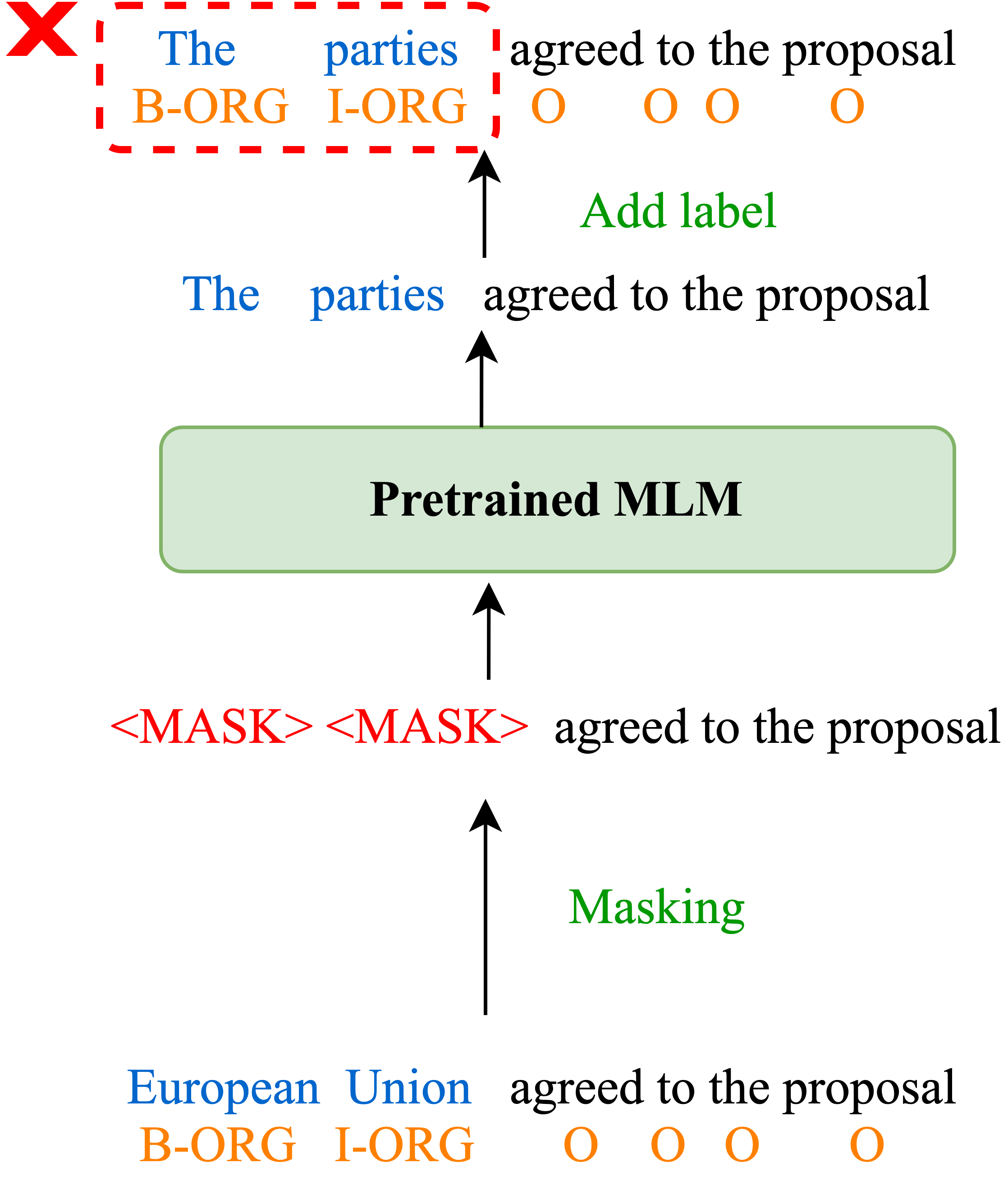
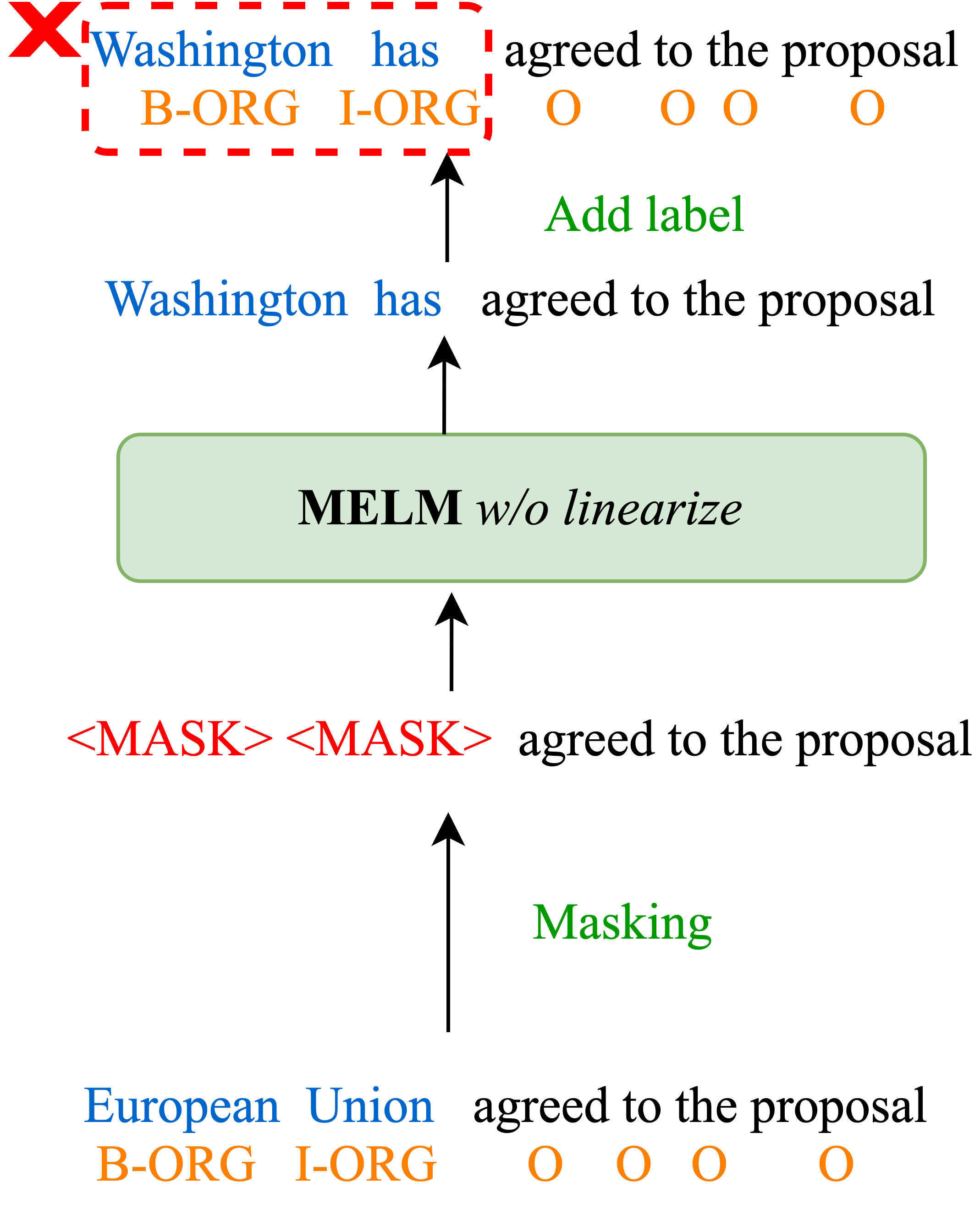
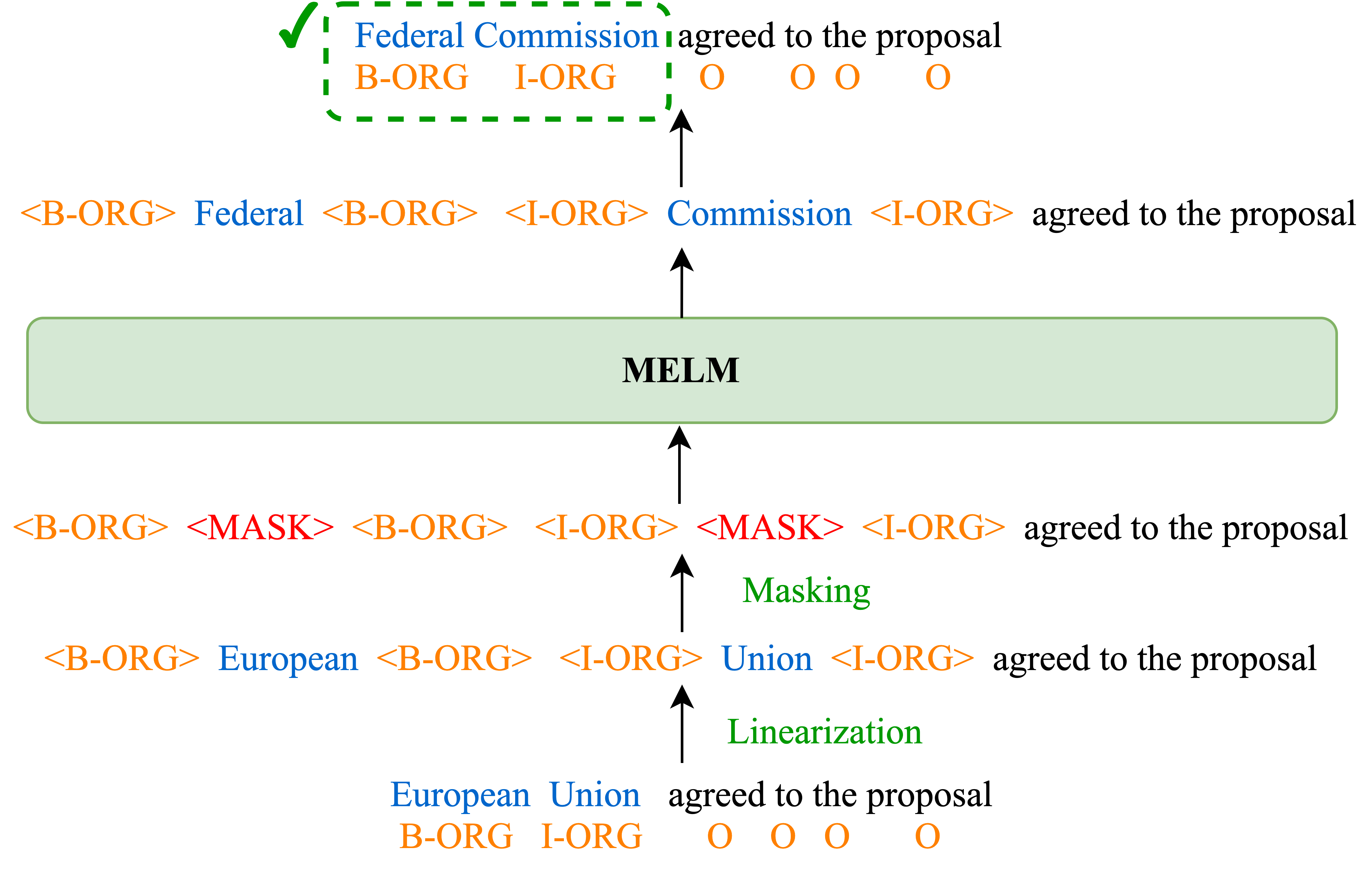
2 Method
Fig. 2(c) presents the work flow of our proposed data augmentation framework. We first perform labeled sequence linearization to insert the entity label tokens into the NER training sentences (Section 2.1). Then, we fine-tune the proposed MELM on linearized sequences (Section 2.2) and create augmented data by generating diverse entities via masked entity prediction (Section 2.3).
The augmented data undergoes post-processing (Section 2.4) and is combined with the original training set for training the NER model. Algorithm 1 gives the pseudo-code for the overall framework. Under multilingual scenarios, we propose an entity similarity search algorithm as a refined code-mixing strategy (Section 2.5) and apply our MELM on the union set of gold training data and code-mixed data for further performance improvement.
2.1 Labeled Sequence Linearization
To minimize the amount of generated tokens incompatible with the original labels, we design a labeled sequence linearization strategy to explicitly take label information into consideration during masked language modeling. Specifically, as shown in Figure 2(c), we add the label token before and after each entity token and treat them as normal context tokens. The yielded linearized sequence is utilized to further finetune our MELM so that its prediction is additionally conditioned on the inserted label tokens. Note that, we initialize the embeddings of label tokens with those of tokens semantically related to the label names (e.g., “organization” for B-ORG ). By doing so, the linearized sequence is semantically closer to a natural sentence and the difficulty of finetuning on linearized sequence could be reduced Kumar et al. (2020).
2.2 Fine-tuning MELM
Unlike MLM, only entity tokens are masked during MELM fine-tuning. At the beginning of each fine-tuning epoch, we randomly mask entity tokens in the linearized sentence with masking ratio .
Then, given the corrupted sentence as input, our MELM is trained to maximize the probabilities of the masked entity tokens and reconstruct the linearized sequence :
(1) |
where represents the parameters of MELM, is the number of tokens in , is the original token in , if is masked and otherwise . Through the above fine-tuning process, the proposed MELM learns to make use of both contexts and label information to predict the masked entity tokens. As we will demonstrate in Section 4.1, the predictions generated by the fine-tuned MELM are significantly more coherent with the original entity label, compared to those from other methods.
2.3 Data Generation
To generate augmented training data for NER, we apply the fine-tuned MELM to replace entities in the original training samples. Specifically, given a corrupted sequence, MELM outputs the probability of each token in the vocabulary being the masked entity token. However, as the MELM is fine-tuned on the same training set, directly picking the most probable token as the replacement is likely to return the masked entity token in the original training sample, and might fail to produce a novel augmented sentence. Therefore, we propose to randomly sample the replacement from the top k most probable components of the probability distribution. Formally, given the probability distribution for a masked token, we first select a set of the most likely candidates. Then, we fetch the replacement via random sampling from . After obtaining the generated sequence, we remove the label tokens and use the remaining parts as the augmented training data. For each sentence in the original training set, we repeat the above generation procedure rounds to produce augmented examples.
To increase the diversity of augmented data, we adopt a different masking strategy from train time. For each entity mention comprising of tokens, we randomly sample a dynamic masking rate from Gaussian distribution , where the Gaussian variance is set as . Thus, the same sentence will have different masking results in each of the augmentation rounds, resulting in more varied augmented data.
2.4 Post-Processing
To remove noisy and less informative samples from the augmented data, the generated augmented data undergoes post-processing. Specifically, we train a NER model with the available gold training samples and use it to automatically assign NER tags to each augmented sentence. Only augmented sentences whose predicted labels are consistent with the their original labels are kept. The post-processed augmented training set is combined with the gold training set to train the final NER tagger.
2.5 Extending to Multilingual Scenarios
When extending low-resource NER to multilingual scenarios, it is straightforward to separately apply the proposed MELM on language-specific data for performance improvement. Nevertheless, it offers higher potential to enable MELM on top of code-mixing techniques, which proved to be effective in enhancing multilingual learning (Singh et al., 2019; Qin et al., 2020; Zhang et al., 2021). In this paper, with the aim of bridging MELM augmentation and code-mixing, we propose an entity similarity search algorithm to perform MELM-friendly code-mixing.
Specifically, given the gold training sets over a set of languages, we first collect label-wise entity sets , which consists of the entities appearing in and belonging to class . To apply code-mixing on a source language sentence , we aim to substitute a mentioned entity of label with a target language entity , where the target language is sampled as . Instead of randomly selecting from , we choose to retrieve the entity with the highest semantic similarity to as . Practically, we introduce MUSE bilingual embeddings (Conneau et al., 2017) and calculate the entity’s embedding by averaging the embeddings of the entity tokens:
(2) |
where denotes the aligned embeddings and is the -th token of . Next, we obtain the target-language entity semantically closest to as follows:
(3) |
here is the cosine similarity function. The output entity is then used to replace to create a code-mixed sentence more suitable for MELM augmentation. To generate more augmented data with diverse entities, we further apply MELM on the gold and code-mixed data. Since the training data now contains entities from multiple languages, we also prepend a language marker to the entity token to help MELM differentiate different languages, as shown in Figure 3.
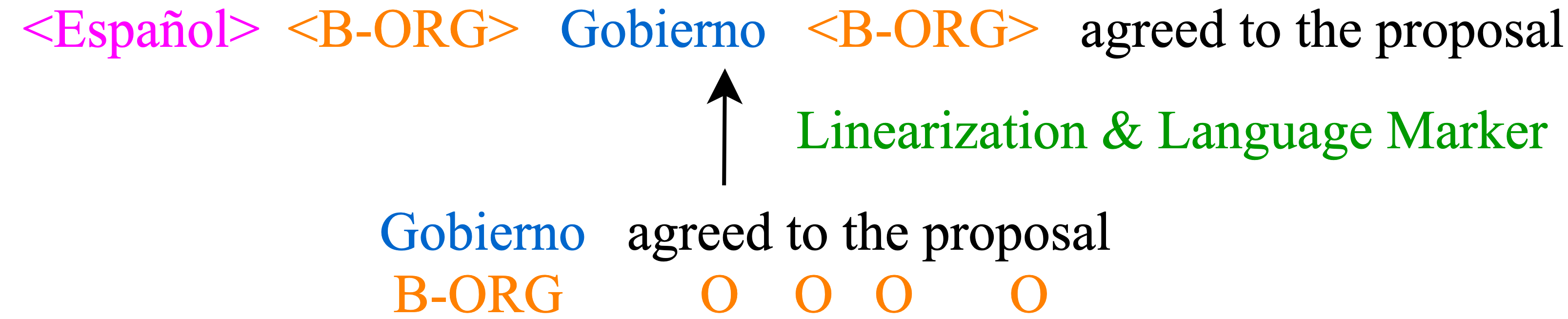
3 Experiments
To comprehensively evaluate the effectiveness of the proposed MELM on low-resource NER, we consider three evaluation scenarios: monolingual, zero-shot cross-lingual and multilingual low-resource NER.
3.1 Dataset
We conduct experiments on CoNLL NER dataset (Tjong Kim Sang, 2002; Tjong Kim Sang and De Meulder, 2003) of four languages where = {English (En), German (De), Spanish (Es), Dutch (Nl)}. For each language , we first sample sentences from the full training set as , where to simulate different low-resource levels. For a realistic data split ratio, we also downscale the full development set to samples as . The full test set for each language is adopted as for evaluation.
For monolingual experiments on language with low-resource level , we use as the gold training data, as the development set and as the test set. For zero-shot cross-lingual experiments with low-resource level , we use as the source language gold training data, as the development set and , and as target language test sets. Under multilingual settings where training data from each language is available , we use as the gold training data, as the development set and evaluate on , , and , respectively.
3.2 Experimental Setting
MELM Fine-tuning
NER Model
We use XLM-RoBERTa-Large (Conneau et al., 2020) with CRF head (Lample et al., 2016) as the NER model for our experiments222https://github.com/allanj/pytorch_neural_crf. We adopt Adamw optimizer (Loshchilov and Hutter, 2019) with learning rate set to and set batch size to 16. The NER model is trained for 10 epochs and the best model is selected according to dev set performance. The trained model is evaluated on test sets and we report the averaged Micro-F1 scores over 3 runs.
Hyperparameter Tuning
The masking rate in MELM fine-tuning, the Gaussian mean for MELM generation and the number of MELM augmentation rounds are set as 0.7, 0.5 and 3, respectively. All of these hyperparameters are tuned on the dev set with grid search. Details of the hyperparameter tuning can be found in Appendix A.1
3.3 Baseline Methods
To elaborate the effectiveness of the proposed MELM, we compare it with the following methods:
Gold-Only The NER model is trained on only the original gold training set.
Label-wise Substitution Dai and Adel (2020) randomly substituted named entities with existing entities of the same entity type from the original training set.
MLM-Entity We randomly mask entity tokens and directly utilize a pretrained MLM for data augmentation without fine-tuning and labeled sequence linearization as used in MELM. The prediction of a masked entity token does not consider label information but solely relies on the context words.
DAGA Ding et al. (2020) firstly linearized NER labels into the input sentences and then use them to train an autoregressive language model. The language model was used to synthesize augmented data from scratch, where both context and entities are generated simultaneously.
MulDA Liu et al. (2021) fine-tuned mBART(Liu et al., 2020) on linearized multilingual NER data to generate augmented data with new context and entities.
#Gold | Method | Monolingual | Cross-lingual | |||||||
En | De | Es | Nl | Avg | EnDe | EnEs | EnNl | Avg | ||
100 | Gold-Only | 50.57 | 39.47 | 42.93 | 21.63 | 38.65 | 39.54 | 37.40 | 39.27 | 38.74 |
Label-wise | 61.34 | 55.00 | 59.54 | 27.85 | 50.93 | 45.85 | 43.74 | 50.51 | 46.70 | |
MLM-Entity | 61.22 | 50.96 | 61.29 | 46.59 | 55.02 | 47.96 | 45.42 | 49.34 | 47.57 | |
DAGA | 68.06 | 59.15 | 69.33 | 45.64 | 60.54 | 52.95 | 46.72 | 54.63 | 51.43 | |
MELM w/o linearize | 70.01 | 61.92 | 65.07 | 59.76 | 64.19 | 48.70 | 49.10 | 53.37 | 50.39 | |
MELM (Ours) | 75.21 | 64.12 | 75.85 | 66.57 | 70.44 | 56.56 | 53.83 | 60.62 | 57.00 | |
200 | Gold-Only | 74.64 | 62.85 | 72.64 | 55.96 | 66.52 | 54.95 | 51.26 | 60.71 | 55.64 |
Label-wise | 76.82 | 67.31 | 78.34 | 66.52 | 72.25 | 55.01 | 53.14 | 63.30 | 57.15 | |
MLM-Entity | 79.16 | 70.01 | 78.45 | 66.69 | 73.58 | 60.44 | 57.72 | 68.37 | 62.18 | |
DAGA | 79.11 | 69.82 | 78.95 | 68.53 | 74.10 | 59.58 | 57.68 | 65.74 | 61.00 | |
MELM w/o linearize | 81.77 | 71.41 | 80.43 | 72.92 | 76.63 | 62.57 | 63.49 | 70.18 | 65.41 | |
MELM (Ours) | 82.91 | 72.71 | 80.46 | 77.02 | 78.27 | 65.01 | 63.71 | 70.37 | 66.36 | |
400 | Gold-Only | 81.85 | 70.77 | 80.02 | 74.60 | 76.81 | 65.76 | 61.57 | 71.04 | 66.12 |
Label-wise | 84.62 | 74.33 | 81.01 | 77.87 | 79.46 | 66.18 | 67.43 | 71.93 | 68.51 | |
MLM-Entity | 83.82 | 74.66 | 81.08 | 77.90 | 79.37 | 67.41 | 70.28 | 74.31 | 70.67 | |
DAGA | 84.36 | 72.95 | 82.83 | 78.99 | 79.78 | 66.77 | 67.13 | 72.40 | 68.77 | |
MELM w/o linearize | 85.16 | 75.42 | 82.34 | 79.34 | 80.56 | 68.02 | 66.01 | 72.98 | 69.00 | |
MELM (Ours) | 85.73 | 77.50 | 83.31 | 80.92 | 81.87 | 68.08 | 70.37 | 75.78 | 71.74 | |
800 | Gold-Only | 86.35 | 78.35 | 83.23 | 83.86 | 82.95 | 65.31 | 68.28 | 72.07 | 68.55 |
Label-wise | 86.72 | 78.21 | 84.42 | 84.26 | 83.40 | 65.60 | 72.22 | 74.77 | 70.86 | |
MLM-Entity | 86.50 | 78.30 | 84.09 | 83.93 | 83.20 | 65.42 | 69.10 | 74.85 | 69.79 | |
DAGA | 86.61 | 77.66 | 84.64 | 84.90 | 83.45 | 68.76 | 70.97 | 75.02 | 71.58 | |
MELM w/o linearize | 87.35 | 78.58 | 84.59 | 84.94 | 83.99 | 67.37 | 71.53 | 75.20 | 71.37 | |
MELM (Ours) | 87.59 | 79.32 | 85.40 | 85.17 | 84.37 | 67.95 | 75.72 | 75.25 | 72.97 |
3.4 Experimental Results
3.4.1 Monolingual and Cross-lingual NER
As illustrated on the left side of Table 1, the proposed MELM consistently achieves the best averaged results across different low-resource levels, demonstrating its effectiveness on monolingual NER. Compared to the best-performing baselines, our MELM obtains 6.3, 1.6, 1.3, 0.38 absolute gains on 100, 200, 400 and 800 levels, respectively. Cross-lingual NER results are shown on the right side of Table 2. Again, on each of the designed low-resource levels, our MELM is superior to baseline methods in terms of the averaged F1 scores. We also notice that, given 100 Nl training samples, the Gold-Only method without data augmentation almost fails to converge while the monolingual F1 of our MELM reaches 66.6, suggesting that data augmentation is crucial for NER when the annotated training data is extremely scarce.
To assess the efficacy of the proposed labeled sequence linearization (Section 2.1), we directly fine-tune MELM on masked sentences without linearization (as shown in Figure 2(b)), denoted as MELM w/o linearize in Table 1. We observe a considerable performance drop compared with MELM, which proves the label information injected via linearization indeed helps MELM differentiate different entity types, and generate entities compatible with the original label.
Taking a closer look at the baseline methods, we notice that the monolingual performance of Label-wise is still unsatisfactory in most cases. One probable reason is that only existing entities within the training data are used for replacement and the entity diversity after augmentation is not increased. Moreover, randomly sampling an entity of the same type for replacement is likely to cause incompatibility between the context and the entity, yielding a noisy augmented sample for NER training. Although MLM-Entity tries to mitigate these two issues by employing a pretrained MLM to generate novel tokens that fit into the context, the generated tokens might not be consistent with the original labels. Our MELM also promotes the entity diversity of augmented data by exploiting pretrained model for data augmentation.
In the meantime, equipped with the labeled sequence linearization strategy, MELM augmentation is explicitly guided by the label information and the token-label misalignment is largely alleviated, leading to superior results in comparison to Lable-wise and MLM-Entity.
We also compare with DAGA (Ding et al., 2020), which generates augmented data from scratch using an autoregressive language model trained on gold NER data. Although DAGA is competitive on low-resource levels of 400 and 800, it still underperforms the proposed MELM by a large margin when the training size reduces to 100 or 200. We attribute this to the disfluent and ungrammatical sentences generated from the undertrained language model. Instead of generating augmented data from scratch, MELM focuses on modifying entity tokens and leave the context unchanged, which guarantees the quality of augmented sentences even under extremely low-resource settings.
3.4.2 Multilingual NER
#Gold | Method | En | De | Es | Nl | Avg |
100 4 | Gold-Only | 75.62 | 69.35 | 75.85 | 74.33 | 73.79 |
MulDA | 73.67 | 70.47 | 75.53 | 72.40 | 73.02 | |
MELM-gold (Ours) | 78.71 | 74.79 | 81.25 | 78.85 | 78.40 | |
Code-Mix-random | 77.38 | 70.58 | 78.61 | 76.45 | 75.75 | |
Code-Mix-ess (Ours) | 79.55 | 71.56 | 79.58 | 76.49 | 76.80 | |
MELM (Ours) | 80.96 | 75.61 | 81.47 | 80.14 | 79.54 | |
200 4 | Gold-Only | 83.06 | 76.39 | 82.71 | 79.19 | 80.34 |
MulDA | 82.32 | 74.57 | 82.73 | 79.06 | 79.67 | |
MELM-gold (Ours) | 82.90 | 78.05 | 85.93 | 81.00 | 81.97 | |
Code-Mix-random | 82.86 | 75.70 | 83.13 | 79.08 | 80.19 | |
Code-Mix-ess (Ours) | 83.34 | 76.64 | 82.02 | 82.27 | 81.07 | |
MELM (Ours) | 83.56 | 78.24 | 84.98 | 82.79 | 82.39 | |
400 4 | Gold-Only | 83.92 | 77.40 | 83.22 | 84.04 | 82.14 |
MulDA | 84.37 | 78.41 | 84.54 | 83.09 | 82.60 | |
MELM-gold (Ours) | 86.04 | 79.09 | 85.76 | 84.83 | 83.93 | |
Code-Mix-random | 85.04 | 77.91 | 84.44 | 83.56 | 82.74 | |
Code-Mix-ess (Ours) | 85.74 | 80.03 | 85.18 | 85.36 | 84.08 | |
MELM (Ours) | 86.14 | 80.33 | 86.60 | 85.99 | 84.76 |
For multilingual low-resource NER, we firstly directly apply MELM on the concatenation of training sets from multiple languages. As shown in Table 2, MELM-gold achieves substantial improvement over the Gold-only baseline, which is consistent with monolingual and cross-lingual results. We compare with MulDA (Liu et al., 2021) as a baseline data augmentation method. MulDA generates augmented data autoregressively with an mBART model, which is fine-tuned on NER data with inserted label tokens. At the low-resource levels in our experimental settings, MulDA is less effective and even leads to deteriorated performance. The unsatisfactory performance mainly results from the discrepancy between pretraining and fine-tuning due to the inserted label tokens. Given very few training samples, it is difficult to adapt mBART to capture the distribution of the inserted label tokens, and thus MulDA struggles to generate fluent and grammatical sentences from scratch. In comparison, our proposed method preserves the original context and introduce less syntactic noise in the augmented data. To further leverage the benefits of code-mixing in multilingual NER, we experiment with two code-mixing methods: (1) Code-Mix-random, which randomly substitutes entities with existing entities of the same type from other languages, and (2) Code-Mix-ess, which adopts the proposed entity similarity search algorithm in Section 2.5 as the code-mixing strategy.
Experimental results in Table 2 show that both methods are able to achieve improved performance over Gold-Only. This observation suggests that code-mixing techniques, either random code-mixing or code-mixing via our entity similarity search, are indeed helpful for multilingual NER. Comparing these two methods, the performance gains brought by Code-Mix-ess are more significant and consistent across different low-resource levels, which demonstrates the effectiveness of our proposed entity similarity search algorithm. Applying MELM on both gold data and code-mixed data from Code-Mix-ess, the multilingual NER results are further improved. In summary, our proposed MELM is well-suited for multilingual NER, which can be integrated with our code-mixing technique to achieve further improvement.
4 Further Analysis
4.1 Case Study
Text | EU | rejects | German | call to boycott | British | Lamb |
Label | B-ORG | O | B-MISC | O O O | B-MISC | O |
MLM | Britain, EU,UK, Trump, US | US, a, UN, the, UK | the, a, black, white, young | |||
MELM w/o linearize | EU, Australia, US, UN, Israel | German, Indian, the, Washington, Union | Chinese, British, raw, California, Australian | |||
MELM | EU, Greenpeace, Amnesty, UN, Reuters | German, British, Dutch, French, EU | African, British, Guinean, white, French | |||
Text | Clinton | aide | resigns , | NBC | says | |
Label | B-PER | O | O O | B-ORG | O | |
MLM | my, his, My, When, her | he, she, it, and, who | ||||
MELM w/o linearize | French, German, British, Swiss, Russian | Reuters, Pompeo, Blair Hill, AFP | ||||
MELM | French, White, Walker, Ferguson, David | NBC, AFP, Greenpeace, BBC, Anonymous |
Apart from the quantitative results, we further analyze the augmented data to demonstrate the effectiveness of our MELM in maintaining the consistency between the original label and the augmented token. Table 3 presents examples of the top-5 predictions from pretrained MLM, MELM w/o linearize and MELM. As we can see, the pretrained MLM, which does not introduce any design or contraint on data augmentation, tends to generate high-frequency words such as “the”, “he” and “she”, and the majority of generated words do not belong to the original entity class. Being finetuned on NER data with entity-oriented masking, MELM w/o linearize is able to generate more entity-related tokens.
However, without the explicit guidance from entity labels, it is still too difficult for MELM w/o linearize to make valid predictions solely based on the ambiguous context (e.g., both “Pompeo” (PER) and “Reuters” (ORG) are compatible with the context of Example #2), which leads to token-label misalignment. Compared to the above methods, our MELM take both label information and context into consideration, and thus generates more entities that fit into the context and align with the original label as well. Moreover, it is noteworthy that MELM can leverage the knowledge from pretrained model to generate real-world entities that do not exist in the original NER dataset (e.g., “Greenpeace” and “Amnesty”), which essentially increases the entity diversity in training data.
4.2 Number of Unique Entities
As demonstrated in Lin et al. (2020) and our preliminary experiments in Figure 1, introducing unseen entities can effectively provide more entity regularity knowledge, and helps to improve NER performance. Therefore, we examine the amount of unique entities introduced by different methods. As there might be token-label misalignment in the augmented data, we firstly train an ‘oracle’ NER model on the full CoNLL dataset and then use it to tag training data of MELM and different baseline methods. For each method, we count the total number of unique entities whose labels match the labels assigned by the ‘oracle’ model. As shown in Figure 4, while many augmented entities from MLM-Entity, DAGA and MELM w/o linearize are filtered out due to token-label misalignment, we note that MELM introduces a significantly larger number of unseen entities in the augmented data. Therefore MELM is able to provide richer entity regularity knowledge, which explains its superiority over the baseline methods.
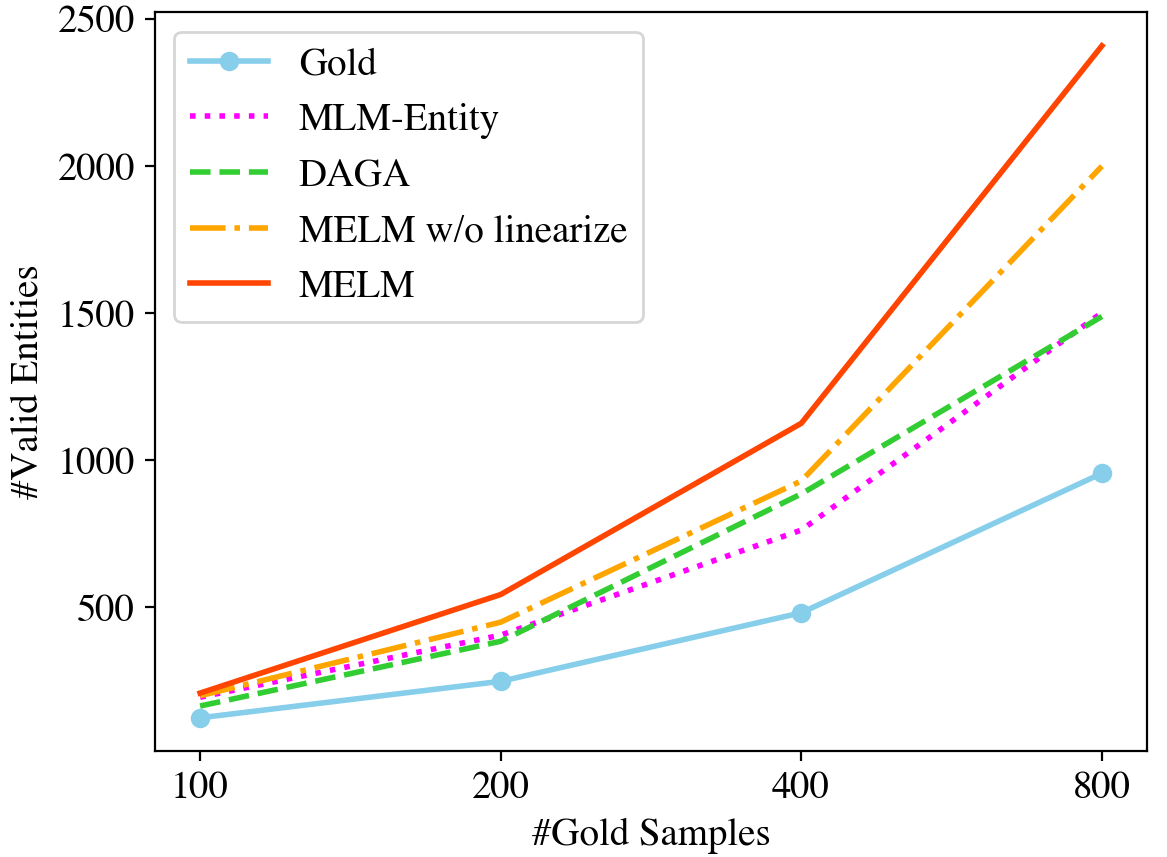
5 Related Work
On sentence level tasks, one line of data augmentation methods are built upon word-level modifications, which can be based on synonym replacement (Wei and Zou, 2019), LSTM language model (Kobayashi, 2018), MLM (Wu et al., 2019; Kumar et al., 2020), auto-regressive pretrained LM (Kumar et al., 2020), or constituent-based tagging schemes (Zhong et al., 2020). However, these methods suffer from token-label misalignment when applied to token-level tasks such as NER, which requires sophisticated post-processing to remove noisy samples in augmented data (Bari et al., 2021; Zhong and Cambria, 2021).
Existing works avoid token-label misalignment by replacing entities with existing entities of the same class (Dai and Adel, 2020), or only modifying context works and leaving entities / aspect terms unchanged Li et al. (2020a). Others attempt to produce augmented data by training / fine-tuning a generative language model on linearized labeled sequences (Ding et al., 2020; Liu et al., 2020).
Backtranslation (Sennrich et al., 2016; Fadaee et al., 2017; Dong et al., 2017; Yu et al., 2018) translates source language sentences into a target language, and subsequently back to the source language, which preserve the overall semantics of the original sentences. On token-level tasks, however, they hinge on external word alignment tools for label propagation, which are often error-prone (Tsai et al., 2016; Li et al., 2020b).
6 Conclusion
We have proposed MELM as a data augmentation framework for low-resource NER. Through labeled sequence linearization, we enable MELM to explicitly condition on label information when predicting masked entity tokens. Thus, our MELM effectively alleviates the token-label misalignment issue and generates augmented data with novel entities by exploiting pretrained knowledge. Under multilingual settings, we integrate MELM with code-mixing for further performance gains. Extensive experiments show that the proposed framework demonstrates encouraging performance gains on monolingual, cross-lingual and multilingual NER across various low-resource levels.
Acknowledgements
This research is partly supported by the Alibaba-NTU Singapore Joint Research Institute, Nanyang Technological University. Erik Cambria would like to thank the support by the Agency for Science, Technology and Research (A*STAR) under its AME Programmatic Funding Scheme (Project #A18A2b0046).
References
- Banerjee et al. (2019) Partha Sarathy Banerjee, Baisakhi Chakraborty, Deepak Tripathi, Hardik Gupta, and Sourabh S Kumar. 2019. A information retrieval based on question and answering and ner for unstructured information without using sql. Wireless Personal Communications, 108(3):1909–1931.
- Bari et al. (2021) M Saiful Bari, Tasnim Mohiuddin, and Shafiq Joty. 2021. UXLA: A robust unsupervised data augmentation framework for zero-resource cross-lingual NLP. In Proceedings of the Annual Meeting of the Association for Computational Linguistics and the International Joint Conference on Natural Language Processing, pages 1978–1992.
- Conneau et al. (2020) Alexis Conneau, Kartikay Khandelwal, Naman Goyal, Vishrav Chaudhary, Guillaume Wenzek, Francisco Guzmán, Edouard Grave, Myle Ott, Luke Zettlemoyer, and Veselin Stoyanov. 2020. Unsupervised cross-lingual representation learning at scale. In Proceedings of the 58th Annual Meeting of the Association for Computational Linguistics, pages 8440–8451, Online. Association for Computational Linguistics.
- Conneau et al. (2017) Alexis Conneau, Guillaume Lample, Marc’Aurelio Ranzato, Ludovic Denoyer, and Hervé Jégou. 2017. Word translation without parallel data. arXiv preprint arXiv:1710.04087.
- Cotterell and Duh (2017) Ryan Cotterell and Kevin Duh. 2017. Low-resource named entity recognition with cross-lingual, character-level neural conditional random fields. In Proceedings of the Eighth International Joint Conference on Natural Language Processing (Volume 2: Short Papers), pages 91–96, Taipei, Taiwan. Asian Federation of Natural Language Processing.
- Dai and Adel (2020) Xiang Dai and Heike Adel. 2020. An analysis of simple data augmentation for named entity recognition. In Proceedings of the 28th International Conference on Computational Linguistics, pages 3861–3867, Barcelona, Spain (Online). International Committee on Computational Linguistics.
- Ding et al. (2020) Bosheng Ding, Linlin Liu, Lidong Bing, Canasai Kruengkrai, Thien Hai Nguyen, Shafiq Joty, Luo Si, and Chunyan Miao. 2020. DAGA: Data augmentation with a generation approach for low-resource tagging tasks. In Proceedings of the 2020 Conference on Empirical Methods in Natural Language Processing (EMNLP), pages 6045–6057, Online. Association for Computational Linguistics.
- Dong et al. (2017) Li Dong, Jonathan Mallinson, Siva Reddy, and Mirella Lapata. 2017. Learning to paraphrase for question answering. In Proceedings of the 2017 Conference on Empirical Methods in Natural Language Processing, pages 875–886, Copenhagen, Denmark. Association for Computational Linguistics.
- Fabbri et al. (2020) Alexander Fabbri, Patrick Ng, Zhiguo Wang, Ramesh Nallapati, and Bing Xiang. 2020. Template-based question generation from retrieved sentences for improved unsupervised question answering. In Proceedings of the 58th Annual Meeting of the Association for Computational Linguistics, pages 4508–4513, Online. Association for Computational Linguistics.
- Fadaee et al. (2017) Marzieh Fadaee, Arianna Bisazza, and Christof Monz. 2017. Data augmentation for low-resource neural machine translation. In Proceedings of the 55th Annual Meeting of the Association for Computational Linguistics (Volume 2: Short Papers), pages 567–573, Vancouver, Canada. Association for Computational Linguistics.
- Feng et al. (2018) Xiaocheng Feng, Xiachong Feng, Bing Qin, Zhangyin Feng, and Ting Liu. 2018. Improving low resource named entity recognition using cross-lingual knowledge transfer. In Proceedings of the International Joint Conference on Artificial Intelligence, IJCAI-18, pages 4071–4077.
- Kingma and Ba (2015) Diederik P. Kingma and Jimmy Ba. 2015. Adam: A method for stochastic optimization. In 3rd International Conference on Learning Representations, ICLR 2015, San Diego, CA, USA, May 7-9, 2015, Conference Track Proceedings.
- Kobayashi (2018) Sosuke Kobayashi. 2018. Contextual augmentation: Data augmentation by words with paradigmatic relations. In Proceedings of the 2018 Conference of the North American Chapter of the Association for Computational Linguistics: Human Language Technologies, Volume 2 (Short Papers), pages 452–457, New Orleans, Louisiana. Association for Computational Linguistics.
- Kumar et al. (2020) Varun Kumar, Ashutosh Choudhary, and Eunah Cho. 2020. Data augmentation using pre-trained transformer models. In Proceedings of the 2nd Workshop on Life-long Learning for Spoken Language Systems, pages 18–26.
- Lample et al. (2016) Guillaume Lample, Miguel Ballesteros, Sandeep Subramanian, Kazuya Kawakami, and Chris Dyer. 2016. Neural architectures for named entity recognition. In Proceedings of the 2016 Conference of the North American Chapter of the Association for Computational Linguistics: Human Language Technologies, pages 260–270, San Diego, California. Association for Computational Linguistics.
- Li et al. (2020a) Kun Li, Chengbo Chen, Xiaojun Quan, Qing Ling, and Yan Song. 2020a. Conditional augmentation for aspect term extraction via masked sequence-to-sequence generation. In Proceedings of the 58th Annual Meeting of the Association for Computational Linguistics, pages 7056–7066, Online. Association for Computational Linguistics.
- Li et al. (2020b) Xin Li, Lidong Bing, Wenxuan Zhang, Zheng Li, and Wai Lam. 2020b. Unsupervised cross-lingual adaptation for sequence tagging and beyond. arXiv preprint arXiv:2010.12405.
- Lin et al. (2020) Hongyu Lin, Yaojie Lu, Jialong Tang, Xianpei Han, Le Sun, Zhicheng Wei, and Nicholas Jing Yuan. 2020. A rigorous study on named entity recognition: Can fine-tuning pretrained model lead to the promised land? In Proceedings of the 2020 Conference on Empirical Methods in Natural Language Processing (EMNLP), pages 7291–7300, Online. Association for Computational Linguistics.
- Liu et al. (2021) Linlin Liu, Bosheng Ding, Lidong Bing, Shafiq Joty, Luo Si, and Chunyan Miao. 2021. MulDA: A multilingual data augmentation framework for low-resource cross-lingual NER. In Proceedings of the 59th Annual Meeting of the Association for Computational Linguistics and the 11th International Joint Conference on Natural Language Processing (Volume 1: Long Papers), pages 5834–5846, Online. Association for Computational Linguistics.
- Liu et al. (2020) Yinhan Liu, Jiatao Gu, Naman Goyal, Xian Li, Sergey Edunov, Marjan Ghazvininejad, Mike Lewis, and Luke Zettlemoyer. 2020. Multilingual denoising pre-training for neural machine translation. Transactions of the Association for Computational Linguistics, 8:726–742.
- Loshchilov and Hutter (2019) Ilya Loshchilov and Frank Hutter. 2019. Decoupled weight decay regularization. In International Conference on Learning Representations.
- Nallapati et al. (2016) Ramesh Nallapati, Bowen Zhou, Caglar Gulcehre, Bing Xiang, et al. 2016. Abstractive text summarization using sequence-to-sequence rnns and beyond. arXiv preprint arXiv:1602.06023.
- Qin et al. (2020) Libo Qin, Minheng Ni, Yue Zhang, and Wanxiang Che. 2020. Cosda-ml: Multi-lingual code-switching data augmentation for zero-shot cross-lingual nlp.
- Rijhwani et al. (2020) Shruti Rijhwani, Shuyan Zhou, Graham Neubig, and Jaime Carbonell. 2020. Soft gazetteers for low-resource named entity recognition. arXiv preprint arXiv:2005.01866.
- Sennrich et al. (2016) Rico Sennrich, Barry Haddow, and Alexandra Birch. 2016. Improving neural machine translation models with monolingual data. In Proceedings of the 54th Annual Meeting of the Association for Computational Linguistics (Volume 1: Long Papers), pages 86–96, Berlin, Germany. Association for Computational Linguistics.
- Singh et al. (2019) Jasdeep Singh, Bryan McCann, Nitish Shirish Keskar, Caiming Xiong, and Richard Socher. 2019. Xlda: Cross-lingual data augmentation for natural language inference and question answering. arXiv preprint arXiv:1905.11471.
- Song et al. (2019) Kaitao Song, Xu Tan, Tao Qin, Jianfeng Lu, and Tie-Yan Liu. 2019. Mass: Masked sequence to sequence pre-training for language generation. In International Conference on Machine Learning, pages 5926–5936. PMLR.
- Tjong Kim Sang (2002) Erik F. Tjong Kim Sang. 2002. Introduction to the CoNLL-2002 shared task: Language-independent named entity recognition. In COLING-02: The 6th Conference on Natural Language Learning 2002 (CoNLL-2002).
- Tjong Kim Sang and De Meulder (2003) Erik F. Tjong Kim Sang and Fien De Meulder. 2003. Introduction to the CoNLL-2003 shared task: Language-independent named entity recognition. In Proceedings of the Seventh Conference on Natural Language Learning at HLT-NAACL 2003, pages 142–147.
- Tsai et al. (2016) Chen-Tse Tsai, Stephen Mayhew, and Dan Roth. 2016. Cross-lingual named entity recognition via wikification. In Proceedings of The 20th SIGNLL Conference on Computational Natural Language Learning, pages 219–228.
- Wei and Zou (2019) Jason Wei and Kai Zou. 2019. EDA: Easy data augmentation techniques for boosting performance on text classification tasks. In Proceedings of the 2019 Conference on Empirical Methods in Natural Language Processing and the 9th International Joint Conference on Natural Language Processing (EMNLP-IJCNLP), pages 6382–6388, Hong Kong, China. Association for Computational Linguistics.
- Wu et al. (2019) Xing Wu, Shangwen Lv, Liangjun Zang, Jizhong Han, and Songlin Hu. 2019. Conditional bert contextual augmentation. In International Conference on Computational Science, pages 84–95. Springer.
- Yu et al. (2018) Adams Wei Yu, David Dohan, Quoc Le, Thang Luong, Rui Zhao, and Kai Chen. 2018. Fast and accurate reading comprehension by combining self-attention and convolution. In International Conference on Learning Representations.
- Zhang et al. (2021) Wenxuan Zhang, Ruidan He, Haiyun Peng, Lidong Bing, and Wai Lam. 2021. Cross-lingual aspect-based sentiment analysis with aspect term code-switching. In Proceedings of the 2021 Conference on Empirical Methods in Natural Language Processing, pages 9220–9230, Online and Punta Cana, Dominican Republic. Association for Computational Linguistics.
- Zhong and Cambria (2021) Xiaoshi Zhong and Erik Cambria. 2021. Time Expression and Named Entity Recognition. Springer.
- Zhong et al. (2020) Xiaoshi Zhong, Erik Cambria, and Amir Hussain. 2020. Extracting time expressions and named entities with constituent-based tagging schemes. Cognitive Computation, 12(4):844–862.
- Zhou et al. (2019) Joey Tianyi Zhou, Hao Zhang, Di Jin, Hongyuan Zhu, Meng Fang, Rick Siow Mong Goh, and Kenneth Kwok. 2019. Dual adversarial neural transfer for low-resource named entity recognition. In Proceedings of the 57th Annual Meeting of the Association for Computational Linguistics, pages 3461–3471, Florence, Italy. Association for Computational Linguistics.
Appendix A Appendix
A.1 Hyperparameter Tuning
Masking hyperparameters. To determine the optimal setting for fine-tune mask rate and generation masking parameter , we conduct a grid search on both hyperparameters in range . We finetune MELM and generate English augmented data on CoNLL following our method in Section 2. The augmented data is used to train a NER tagger and its performance on English dev set is recorded. As shown in Table 4, we achieve the best dev set F1 when and , which is adopted for the rest of this work.
0.3 | 0.5 | 0.7 | ||
0.3 | 76.90 | 75.64 | 78.08 | |
0.5 | 76.16 | 78.06 | 78.56 | |
0.7 | 75.94 | 78.09 | 78.37 |
Number of augmentation rounds. Merging augmented data from multiple rounds increase entity diversity until it saturates at certain point. Continuing adding in more augmented data begins to amplify the noise in augmented data and leads to decreasing performance. To determine the optimum number of augmentation rounds , we merge different amount of augmented data with English gold data to train a NER tagger, with ranging from 1 to 6. As shown in Table 5, dev set F1 increases with increasing amount of augmented data until =3, and starts to drop further beyond. Therefore, we choose for all of our experiments.
R | 1 | 2 | 3 | 4 | 5 | 6 |
Dev F1 | 92.35 | 92.36 | 92.84 | 92.72 | 92.59 | 92.39 |
A.2 Statistics for Reproducibility
In this section, we present the validation F1 averaged among 3 runs of MELM under different languages and low-resource levels. We also summarize the estimated time for fine-tuning MELM and the number of parameters used. We separately show the statistics of monolingual (Table 6), cross-lingual (Table 7) and multilingual (Table 8) NER.
#Gold | En | De | Es | Nl | time | #Paramerter |
100 | 82.38 | 71.11 | 71.77 | 71.01 | ~ 7min | 270M |
200 | 85.93 | 77.96 | 83.25 | 79.53 | ~ 10min | 270M |
400 | 89.01 | 82.95 | 85.10 | 81.40 | ~ 15min | 270M |
800 | 92.01 | 84.82 | 86.65 | 85.61 | ~ 20min | 270M |
#Gold | dev F1 | time | #Paramerter |
100 | 82.38 | ~ 7min | 270M |
200 | 85.93 | ~ 10min | 270M |
400 | 89.01 | ~ 15min | 270M |
800 | 92.01 | ~ 20min | 270M |
#Gold per language | dev F1 | time | #Paramerter |
100 | 83.21 | ~ 20min | 270M |
200 | 84.83 | ~ 30min | 270M |
400 | 87.07 | ~ 45min | 270M |
A.3 Computing Infrastructure
Our experiments are conducted on NVIDIA V100 GPU.