M2PT: Multimodal Prompt Tuning for Zero-shot Instruction Learning
Abstract
Multimodal Large Language Models (MLLMs) demonstrate remarkable performance across a wide range of domains, with increasing emphasis on enhancing their zero-shot generalization capabilities for unseen tasks across various modalities. Instruction tuning has emerged as an effective strategy for achieving zero-shot generalization by finetuning pretrained models on diverse multimodal tasks. As the scale of MLLMs continues to grow, parameter-efficient finetuning becomes increasingly critical. However, most existing parameter-efficient approaches focus only on single modalities and often overlook the multimodal characteristics during finetuning. In this work, we introduce a novel Multimodal Prompt Tuning (M2PT) approach for efficient instruction tuning of MLLMs. M2PT effectively integrates visual and textual prompts into the vision encoder and language processor respectively during finetuning, facilitating the extraction and alignment of features across modalities. Empirical results on various multimodal evaluation datasets demonstrate the superior performance of our approach compared to several state-of-the-art baselines. A comprehensive set of ablation studies validates the effectiveness of our prompt design and the efficiency of our approach.
M2PT: Multimodal Prompt Tuning for Zero-shot Instruction Learning
Taowen Wang1††thanks: Equal contribution., Yiyang Liu111footnotemark: 1, James Chenhao Liang1,11, Junhan Zhao2, Yiming Cui3, Yuning Mao4, Shaoliang Nie4, Jiahao Liu 5, Fuli Feng6, Zenglin Xu7,8, Cheng Han9, Lifu Huang10, Qifan Wang4, Dongfang Liu1 1Rochester Institute of Technology, 2Harvard Medical School, 3ByteDance, 4Meta AI, 5Meituan, 6University of Science and Technology of China, 7Shanghai Academy of AI for Science, 8Fudan University, 9University of Missouri - Kansas City, 10University of California - Davis 11U.S. Naval Research Laboratory {tw9146, dongfang.liu}@rit.edu
1 Introduction
Human cognition intricately integrates various sensory modalities to perceive, interpret, and engage with the environment, fostering a comprehensive understanding of the surrounding world Liu et al. (2023c); Dumas et al. (2009); Chen et al. (2024); Jin et al. (2024a, c). The development of Multimodal Large Language Models (MLLMs) Alayrac et al. (2022); Yin et al. (2023); Han et al. (2024a, c); Jin et al. (2024b, d) marks a significant advancement in emulating this capability, playing a pivotal role in bridging the gap between human and machine intelligence. A key focus in advancing MLLMs is enhancing their zero-shot generalization to new multimodal tasks. In this pursuit, multimodal instruction tuning Liu et al. (2023c, b); Xu et al. (2023), which finetunes pretrained models using diverse and instruction-based multimodal tasks, has proven effective in improving zero-shot generalization to unseen multimodal domains.
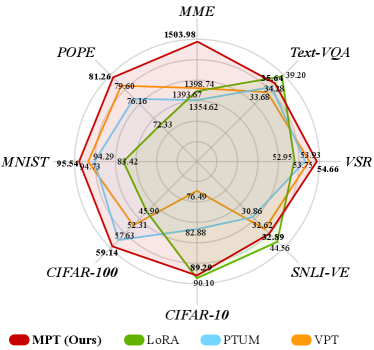
Despite considerable advancements, finetuning MLLMs for specific domain knowledge poses significant challenges. As the scale and complexity of these models increase, the training overhead for downstream tasks grows exponentially Wu et al. (2024a); Xu et al. (2024a); Zhang et al. (2024). These escalating demands render the current tuning schemes for MLLMs obsolete and unsustainable, impeding their widespread utility. A promising solution is the utilization of parameter-efficient finetuning (PEFT) approaches Lester et al. (2021); Hu et al. (2022); Chowdhury et al. (2023); Dong et al. (2023b); Wang et al. (2023a), which have been widely applied and achieved notable success in natural language processing Liu et al. (2021); Wang et al. (2022) and computer vision tasks Jia et al. (2022); Han et al. (2023); Ju et al. (2022a). However, most existing PEFT approaches only focus on single modality tuning while overlooking multimodal instructing learning. A primary challenge is the intricate process of finetuning data of multiple modalities within a cohesive model, extracting and aligning feature representations across modalities. Additionally, there is a pressing need to enhance the zero-shot generalization capabilities for unseen multimodal tasks while minimizing training costs.
In light of this, we present a novel Multimodal Prompt Tuning (M2PT) approach with efficient and effective MLLM adaptation for zero-shot instruction learning. M2PT demonstrates competitive performance across a wide spectrum of tasks (see Fig. 1) while tuning 0.09% of overall parameters. Specifically, we introduce two sets of soft prompts: visual prompts and textual prompts, which are prepended to the visual and instruction inputs, respectively. The learned embeddings of the visual prompts are projected into the embedding space of the textual prompts. The cross-modality interactions between the two sets of prompts are enforced during instruction tuning, facilitating the alignment and learning of the feature representation between the two modalities. In this way, M2PT provides explicit guidance and clear directives through instruction tuning, enabling models to understand context and reduce ambiguity in zero-shot settings.
To effectively assess our method, we conduct comprehensive experiments to evaluate its performance. In §4.2, we demonstrate that M2PT surpasses several state-of-the-art PEFT techniques while tuning only 0.09% of the trainable parameters. We further conduct activation analysis to show the effectiveness of the learned prompts during the attention computation. In §5, we provide various ablation analysis on the impact of model components, prompt length, prompt location, and data volumes in detail. Furthermore, we conduct case studies to better understand the advantage of M2PT and its limitations. We hope this work offers valuable insights into related fields. We summarize the main contributions as follows:
-
•
We present multimodal prompt tuning by introducing both visual and textual prompts into vision encoder and language processor respectively. These modality specific prompts play a crucial role in effectively guiding the model’s fine-tuning process, enabling fast and accurate multimodal model adaptation.
-
•
We design the cross-modality interaction between the prompts from different modalities during the instruction tuning. By doing so, M2PT leverages the strengths of each modality, resulting in comprehensive and coherent learning results. This synergy empowers the model to perform complex tasks that require the integration from multimodal perspectives.
-
•
We conduct comprehensive experiments on various multimodal tasks in the zero-shot setting, demonstrating the effectiveness of the proposed approach over several state-of-the-art parameter efficient finetuning methods.
2 Related Work
Multimodal Large Language Models.
MLLMs Dai et al. (2023); Driess et al. (2023); Liu et al. (2023c); Yao et al. (2023); Sun et al. (2024a) integrate multimodal information (e.g., audio, image, video), extending beyond the textual semantic information processed by conventional Large Language Models (LLMs).A general structure of MLLMs includes three main components Li et al. (2024a): a pre-trained modality encoder to encode multimodality data, a pre-trained LLM to reason encoded multimodal data and perform generation tasks, and a connection layer to project modality information into tokens. During the standard full finetuning process Liu et al. (2023c), a substantial amount of weights within all intermediate layers and the pre-trained LLM are continuously updated. Conceptually different, our approach can elegantly fine-tune the model with adjusting a minimum of weights.
Instruction Tuning.
To enhance the zero-shot and in-context learning Brown et al. (2020); Li et al. (2024b) capabilities of large language models (LLMs) Zhao et al. (2023), researchers have explored instruction tuning Ouyang et al. (2022); Zhang et al. (2023), a technique that enables pre-trained LLMs to be more adaptable for intricate multimodal tasks. Specifically, instruction-tuning is a process that refines LLMs by finetuning them on meticulously curated instruction-following datasets encapsulating user intent and desired outputs Ouyang et al. (2022). With the rapid advancement of multimodal models, instruction tuning has emerged not only as a state-of-the-art approach for natural language processing (NLP) tasks but also naturally extend to vision-related tasks such as image captioning He et al. (2020); Cornia et al. (2020), image classification Radford et al. (2021), visual question answering (VQA) Antol et al. (2015).
Parameter-Efficient Finetuning.
With the drastic growth in the scale of AI models, especially MLLMs and LLMs, Parameter-efficient Finetuning (PEFT) Hu et al. (2022); He et al. (2023); Jie et al. (2023); Yan et al. (2023) have shown its capability to efficiently adapt pre-trained models to diverse downstream tasks without updating significant amount of model parameters. Generally, current PEFT strategies can be categorized into partial tuning Chen et al. (2021); Jia et al. (2021), extra module such as Low-Rank Adaptation (LoRA) Jie et al. (2023) and prompt tuning Jia et al. (2022); Ju et al. (2022b); Dong et al. (2023a). However, partial tuning faces limitations, including unsatisfactory performance relative to full finetuning Jia et al. (2022); Chen et al. (2021); Jia et al. (2021). Extra module exhibits limited adaptability when considering various model backbones. In contrast, prompt tuning Lester et al. (2021); Ma et al. (2022); He et al. (2022a); Liu et al. (2023d); Qiu et al. (2020) provides a general and straightforward solution with powerful performance gains. It introduces a set of learnable parameters to the input sequence of backbone models, updating only these parameters during finetuning. Despite its simplicity, the applicability of prompt tuning within the multimodal paradigm remains largely unexplored. Unlike approaches such as MaPLe Khattak et al. (2023), CoOp Zhou et al. (2022b), CoCoOp Zhou et al. (2022a) and MoPE Wu et al. (2024b), which focus on crafting CLIP-based prompts for classification tasks, our work targets enhancing the capabilities of MLLMs in zero-shot instruction-following scenarios, resulting in a fundamentally distinct method design. Furthermore, PromptFuse Liang et al. (2022) only introduces learnable prompts into textual modality, neglecting the synergy of multimodality. Our approach offers flexibility in prompt design, allowing prompts to be independently tailored for each modality and inserted at various layers. This flexibility significantly enhances the MLLMs’ adaptability while reducing the number of parameters, improving performance across various datasets.
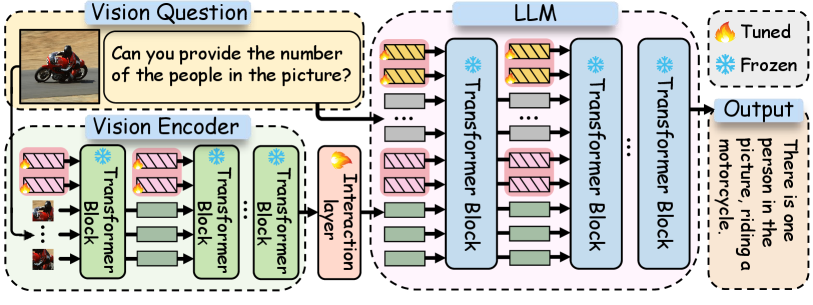
3 Methodology
3.1 Preliminaries
Multimodal Large Language Models
integrate visual and language processing capabilities, leveraging the power of LLMs to enhance the comprehension of multimodal information. A prevalent workflow of MLLMs begins with the utilization of pre-trained vision encoders (e.g., LLaVA Liu et al. (2023c) and its variants Li et al. (2022); Sun et al. (2023)), encoding visual input and extracting output through remarkable vision understanding ability. Subsequently, the vision output is further projected into language space, aligning with the textual embedding and enabling the model to understand and respond to instructions effectively. Ultimately, the integrated LLM assimilates and text embedding . Harnessing the extensive knowledge of LLM, integrating multimodal inputs to generate coherent and contextually relevant language response , represented as:
(1) |
Prompt Tuning is a form of PEFT approach, demonstrating exceptional efficacy within single-modality settings under both visual Han et al. (2023) and textual Wang et al. (2023a) domains. It entails learnable continuous soft prompts into the input space while concurrently preserving the majority of parameters within the backbone frozen. Specifically, given a layer transformer-based model , soft prompts combined with the input of -th layer to obtain the output as:
(2) | ||||
where . and indicate frozen and tunable parameter during finetuning, respectively. is the embedded vector of initial inputs.
3.2 Multimodal Prompt Tuning
In this section, we formally introduce M2PT, a novel multimodal prompt tuning approach for the effective and efficient finetuning of MLLMs. The overall model architecture is depicted in Figure 2. Fundamentally, our model necessitates the training of only three targeted components, while keeping the backbone parameters from both visual encoder and LLM frozen. Specifically, these components include ① Visual Prompt (), which is the learnable parameter (i.e., soft prompt) integrated into the visual encoder; ② Textual Prompt () is incorporated into the LLM in order to capture the nuanced semantic relationships across modalities; ③ Cross-modality Interaction, where parameters are learned to enhance the alignment between features extracted by the vision encoder and textual representations. In summary, prompts from these distinct modalities (i.e., ①-②) facilitate the model’s acquisition of knowledge from multimodal finetuning datasets, capturing the distinctive characteristics inherent in each modality and fostering cross-modal interaction.
Visual Prompt. We denote the set of visual prompts as , where indicates the set of visual prompts in the -th layer of the visual encoder, consistent with previous practice for prompt tuning Jia et al. (2022); Han et al. (2023). Each prompt is a -dimensional vector with the same dimensions as the original vision tokens. Prompts in each layer are placed at the leftmost positions within the sequence to interact with other vision tokens. Formally, we have:
(3) | ||||
where , and is the -th layer vision embedding.
Textual Prompt. Visual prompts serve as an effective tool for capturing semantic content within the visual inputs, as well as gaining the ability to interact with the text modality through the mapping from visual domain. Nevertheless, the optimization of visual elements does not directly affect the inherent representation of LLMs in text modality. Naturally, to further enhance the text modality’s processing ability, we introduce the textual prompts to capture text patterns and influence the inner representation within the LLM. Specifically, textual prompts are denoted as , where indicates the prompt inject position of the -th layer in a total layers LLM. Each prompt is a -dimensional vector with the same dimensionality as the original text tokens. Formally, we incorporate textual prompts into the LLM as:
(4) | ||||
where , is the textual output of the MLLM, is the textual embedding, and is the task-specific head in order to decode the embeddings into texts.
Cross-modality Interaction. To achieve alignment between visual and textual modality, we introduce a tunable interaction layer , which is specifically designed to align the output produced by the visual encoder and the textual embedding, through a linear projection:
(5) |
Method # para MME Text-VQA VSR SNLI-VE CIFAR-10 CIFAR-100 MNIST POPE MMAvg LLaVAAlign Liu et al. (2023c) - 1110.82 32.62 50.16 34.51 80.00 58.04 52.79 59.10 52.46 LLaVAFT Liu et al. (2023c) 100% 1587.26 37.26 53.76 43.35 92.97 63.73 94.27 80.82 66.59 LoRA Hu et al. (2022) 0.63% 1393.67 39.20 52.95 44.56 90.10 45.90 83.42 72.33 61.21 APrompt Wang et al. (2023a) 0.23% 1406.63 35.26 53.12 45.58 85.74 50.27 84.63 76.16 61.52 PTUM Yang et al. (2023a) 0.12% 1354.62 34.28 53.75 30.86 82.88 57.63 94.29 80.31 62.00 VPT Han et al. (2024b) 0.06% 1398.74 33.68 53.93 32.62 76.49 52.31 94.73 79.60 60.48 M2PT10/10 (Ours) 0.08% 1490.17 35.64 54.66 32.53 87.92 57.80 94.53 81.29 63.48 M2PT10/20 (Ours) 0.09% 1503.98 34.48 53.19 32.89 89.29 59.14 95.54 81.26 63.68
Here represents the aligned vision embedding. This transformation ensures that the visual encoder’s output is effectively mapped onto a common textual representation space. The projected visual tokens then interact with the textual tokens through all LLM layers, facilitating the integration of visual and textual information. Our elegant design of M2PT enjoys a few appealing characteristics:
-
•
Cross-modal Integration. Our M2PT model employs a unified prompt tuning paradigm. This approach not only captures the distinctive attributes of each modality but also facilitates the fluid exchange of cross-modal information, enhancing the model’s capability to comprehend and generate multimodal data effectively.
-
•
Optimized Parameter Utilization. M2PT demonstrates superior parameter efficiency by focusing only on the training of a minimal set of parameters while keeping the majority of the model’s parameters frozen, allowing a significant reduction in the number of parameters required (0.09%). Despite this reduction, M2PT maintains superior performance on multimodal tasks (see Table 1) with a balance between computational efficiency and overall effectiveness in zero-shot setting.
3.3 Implementation Details
We employ LLaVA Liu et al. (2023c) with CLIP-L Radford et al. (2021) (i.e., 24 transformer blocks) as the visual encoder and Vicuna-7B-v1.3 Zheng et al. (2023) (i.e., 32 transformer blocks) as the base LLM for all variants. For the cross-modality interaction, we use a linear layer to map the embedding dimension from to to ensure the alignment between the two modalities. For the prompt initialization, we employ Xavier Glorot and Bengio (2010) initialization on both visual and textual prompt to ensure stable modal information delivery from these prompts at the early stages of training, thereby facilitating rapid convergence of the model. More implementation details are provided in §4.1 and Appendix S2.
4 Experiments
4.1 Experiment Setup
Datasets.
For training, we conduct instruction tuning on Vision-Flan Xu et al. (2024b), which is a human-annotated multimodal instruction tuning dataset with diverse tasks. To reduce computational costs, we follow common practice Shen et al. (2024) and employ a scaled-down version containing up to instances per task, resulting in a total of instances. For zero-shot evaluation, we examine our approach on the comprehensive multimodal evaluation benchmark MME Fu et al. (2023), measuring both perception and cognition abilities across subtasks. We further evaluate the model’s capabilities using multimodal datasets. Specifically, for Optical Character Recognition, we utilize the Text-VQA Singh et al. (2019), and for reasoning, we employ the Visual Spatial Reasoning (VSR) Liu et al. (2023a). Following Zhai et al. (2023); Shen et al. (2024), the perception capability is tested on CIFAR-10/100 Krizhevsky et al. (2009) and MNIST Deng (2012). SNLI-VE Xie et al. (2019) evaluates Visual Entailment capabilities, while the POPE Li et al. (2023) dataset examines the tendency towards object hallucination. More details are provided in the Appendix S1.
Training Details. Following previous works Han et al. (2023); Shen et al. (2024); Jia et al. (2022), we conduct grid search to match the best tuning hyperparameters, learning rate (i.e., [, , , , , ]), textual prompt length (i.e., [0, 5, 10, 20, 40]) and visual prompt length (i.e., [0, 5, 10, 20, 40]). For all models, the learning rate is scheduled following a cosine decay policy, the warm up ratio is set at 0.03 and trained for 3 epochs except in training epoch experiment. We follow the same batch size setting in Shen et al. (2024) as 128 for instruction tuning. Further details are provided in the Appendix S2. M2PT is implemented in Pytorch Paszke et al. (2019). Experiments are conducted on 8 NVIDIA A100 GPUs. Our code is available at https://github.com/William-wAng618/M2PT.
Evaluation Metrics. The MME incorporates both Perception and Cognition metrics111https://github.com/BradyFU/Awesome-Multimodal-Large-Language-Models/tree/Evaluation. For other multimodal datasets, we use Vicuna-13B-v1.5 Zheng et al. (2023) to assess the accuracy of each prediction compared to the groundtruth. Further details are provided in the Appendix S3.
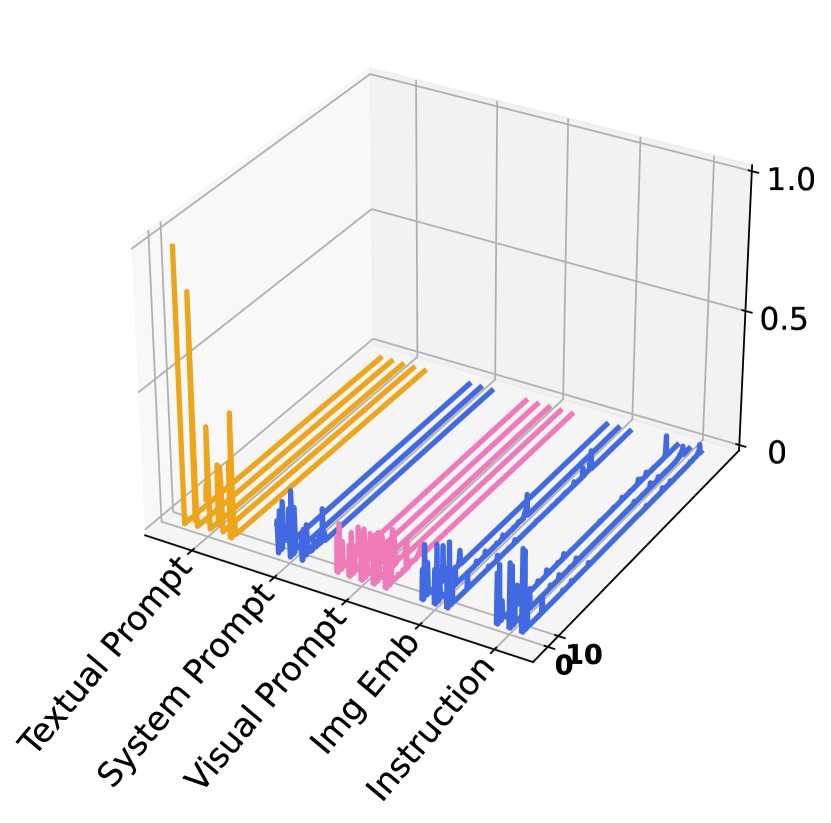
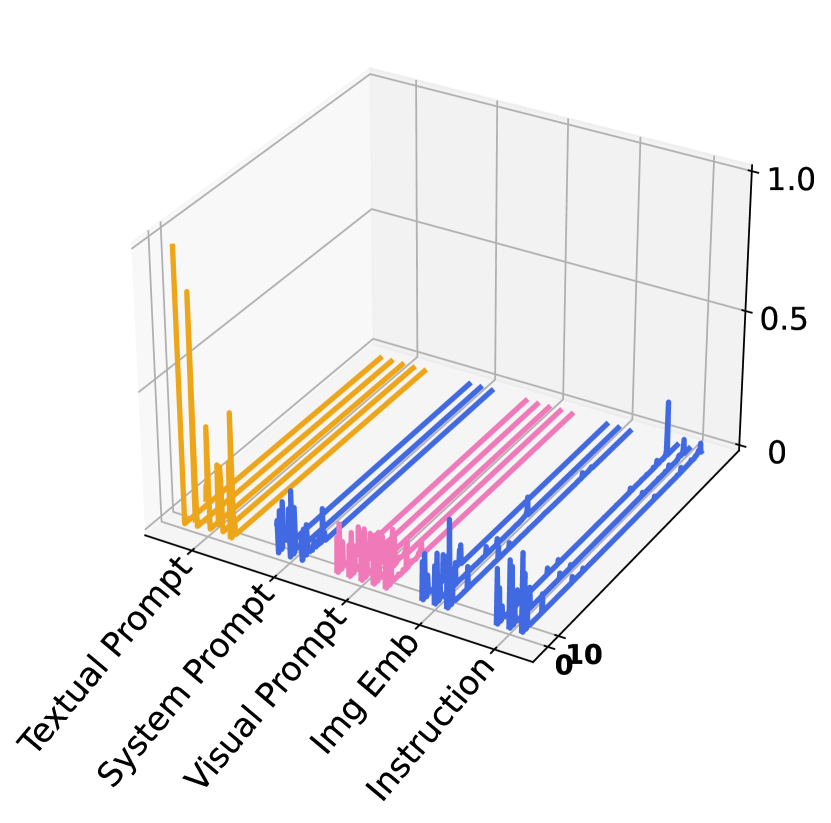
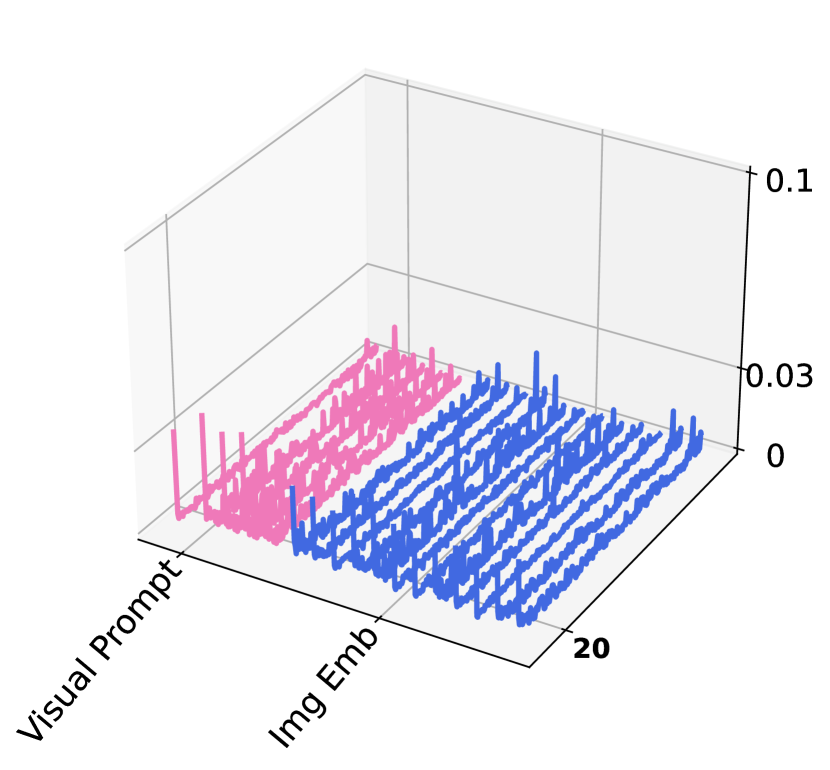
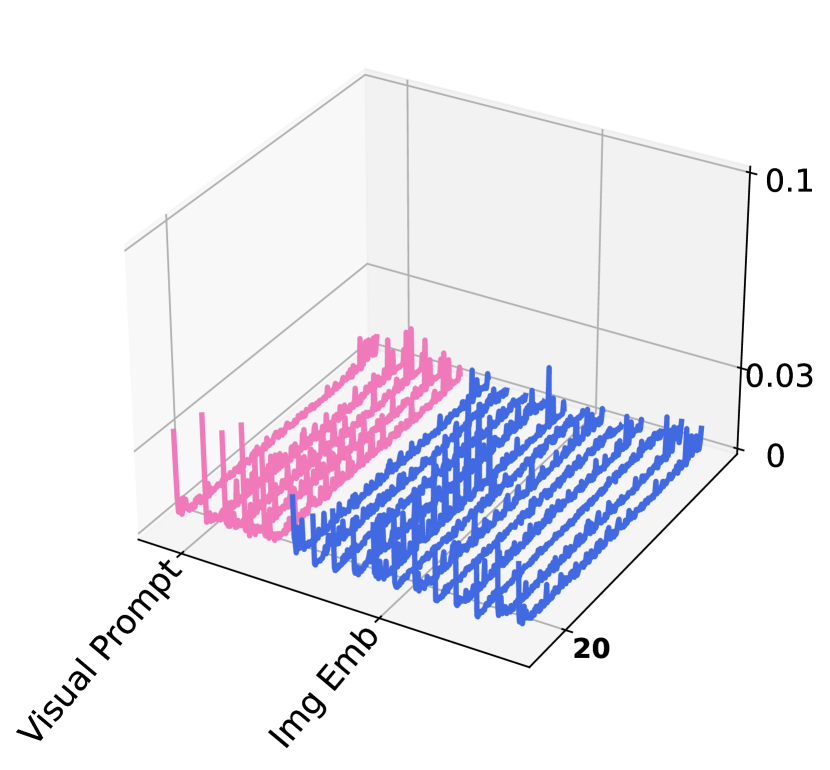
4.2 Main Result
In Table 1, our main result exhibits a comprehensive zero-shot evaluation of M2PT with several baselines on eight multimodal datasets. Specifically, we consider four state-of-the-art PEFT approaches, including LoRA Hu et al. (2022), APrompt Wang et al. (2023a), PTUM Yang et al. (2023a) and VPT Han et al. (2024b). Full fine-tuned LLaVA (i.e., LLaVAFT Liu et al. (2023c)) serves as an upper-bound of multimodal evaluation. We report the performance of M2PT under two different settings, with M2PT10/10 and M2PT10/20.
There are several key observations from these results. First, M2PT achieves the best performance among all PEFT approaches in most cases, demonstrating the effective design of visual and textual prompts. For example, on MME task, M2PT demonstrates a significant improvement of 6.90% and 7.51% compared to two strong prompt tuning methods, Aprompt and VPT, respectively. This highlights the limitation of existing prompt tuning approaches that primarily focus on single modality, failing to capture the cross-modality interactions. In contrast, the interaction layer together with the multimodal LLM employed by our approach successfully bridges this gap, resulting in enhanced performance. Second, the performance of M2PT reaches 94.75% of the full finetuning performance while considering only 0.09% of the total model parameters, demonstrating the parameter efficiency of M2PT. Moreover, we observe that M2PT outperforms the full finetuning LLaVA on VSR, MNIST and POPE tasks, showing its strong capability in zero/few shot learning. This is consistent with the observations in several previous works Han et al. (2023); Yang et al. (2023b). Third, it can be seen that M2PT does not perform very well on the visual entailment task, SNLI-VE. Our hypothesis is that logical relationships or causal scenario understanding is critical in this task, which might not be fully captured by prompt tuning based approaches.
5 Analysis and Discussion
Attention Activation Pattern Analysis.
Following common practice Sun et al. (2024b), we extract and discuss the activation maps from the attention block of MLLMs, and investigate the influence of visual and textual prompts in Fig. 3. We randomly select two samples from the MME dataset and visualize their corresponding activation maps of the attention block in the last layers of both visual encoder (Fig. 3(a)) and LLM (Fig. 3(b)). To analyze the impact of textual and visual prompts to the frozen components, we categorize them, according to LLaVa’s model structure, into textual prompts, system text tokens, visual prompts, image tokens and instructions. Several findings can be observed.
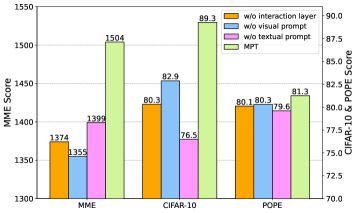
First, in the LLM attention activation map, we observe that the token regions corresponding to textual prompts exhibit elevated activation levels, indicating their significant role in shaping the model’s responses. The activation levels of visual prompts within the LLM, while comparatively lower, remain notable relative to most other regions. This suggests a secondary yet substantial role in multimodal inference. Second, in the activation maps from Visual Encoder, the activation levels associated with visual prompts are noticeably higher than those of most other tokens, underscoring the critical role of visual prompts in processing visual information during tuning. These observations support that visual prompts effectively interact with the textual prompts, enhancing the alignment between modalities and thereby improving the model’s performance on the zero-shot instruction learning. Our component analysis study below further strengthens this claim quantitatively.
Impact of Different Components.
To investigate the impact of different components in M2PT across various datasets (i.e., visual prompts, textual prompts, and interaction layer), we conducted component ablation experiments in Fig. 4 by removing each component at a time from M2PT10/20. The results demonstrate that the model performance drops when any of the trainable prompts is removed, which aligns with our expectations. Moreover, the model performance also decreases without the trainable interaction layer, indicating the importance of this layer. Furthermore, we observe that the importance of different components varies across the tasks. For example, textual prompts play the most important role in CIFAR-10 and POPE, while visual prompts lift the performance most on MME. Once again, it is worth noting that combining the multimodal prompts with the interaction layer in M2PT leads to the best performance.
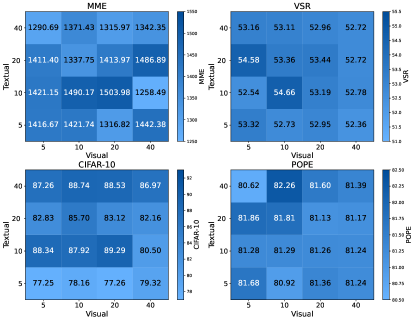
Impact of Prompt Length.
In M2PT, prompt length is the only hyperparameter that needs to be tuned. To further analyze the impact of different prompt lengths on model performance, we conduct a comprehensive study on the lengths of visual and textual prompts on the Vision-Flan dataset to better understand their properties. Following common practice Han et al. (2023); Jia et al. (2022); Han et al. (2024b), we use the grid search to span a range from to on both visual and textual prompt lengths, reported in Fig. 5. We stop at a prompt length of because performance saturation is observed around this point. Thus, extending the prompt length further would result in increased parameter usage without significant performance gains (i.e., M2PT shows a slight decrease in performance on MME. We argue that this may be due to overparameterization Han et al. (2023); Jia et al. (2022)). When visual prompt length extends from to , and textual prompt length extends from to , noticeable performance gains can be observed. It can be seen that there is no universal optimal prompt length that consistently achieves the best performance across different tasks. For instance, the optimal performance of the model on MME is achieved with a configuration of visual prompts and textual prompts, while visual prompts and textual prompts achieve the highest performance on POPE. We hypothesize that different tasks exhibit distinct data distributions, with ‘difficult’ tasks potentially requiring longer prompts to effectively capture the underlying patterns. Nonetheless, we observed that the performance of M2PT remains relatively stable within a certain range of prompt lengths.
Prompt Location | MME | CIFAT-10 | POPE |
(a) First Layer | 1320.99 | 82.96 | 79.48 |
(b) Odd Layer | 1396.87 | 87.65 | 75.79 |
(c) Top Half | 1473.42 | 85.82 | 80.31 |
(d) Latter Half | 1249.39 | 83.72 | 79.34 |
(e) All | 1503.98 | 89.29 | 81.26 |
Impact of Prompt Location.
Following Jia et al. (2022), Table 2 studies the insertion of prompts at different layers. We design five distinct settings in which prompts are integrated into both the Vision Encoder and LLM but at different locations. Specifically, we introduce prompts into: (a) the first layer; (b) every odd-number layer (i.e., , ); (c) the first half of the layers (i.e., 1-12, 1-16); (d) the latter half of the layers (i.e., 12-24, 16-32); and (e) all layers. Each variant reports the best prompt length combination selected with MME evaluation. Generally, M2PT’s performance is positively correlated with prompt depth. Yet the accuracy drops when inserting prompts from top to bottom, suggesting that prompts at earlier layers matter more than those at latter layers, which is consistent with the observations in Jia et al. (2022); Wang et al. (2023a).
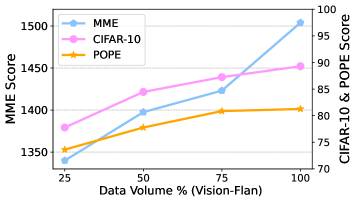
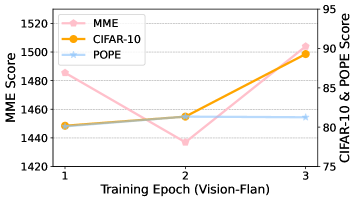
Effect of Data Volume and Training Epoch.
In Fig. 6, we randomly sample data from Vision-Flan at different scales (i.e., 25%, 50%, 75%, 100%) to evaluate the performance of the model with limited data. The results demonstrate that M2PT maintains excellent performance despite significant data reduction, highlighting efficiency and robustness regarding data quantity. This property indicates that M2PT exhibits substantial tolerance to data scale, suggesting a promising future for real-world applications with constrained data.
In Fig. 7, we analyze the M2PT’s performance on different training epochs. This experiment is conducted using the optimal hyperparameter combination of textual and visual prompts. It can be seen that the model performance generally improves with more training epochs, confirming that M2PT can achieve remarkable performance after sufficient training and demonstrate robustness in adapting to different training epochs.
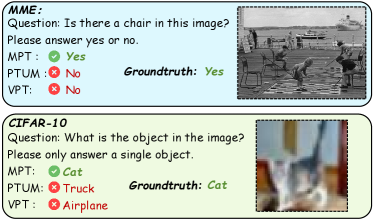
Case Study.
In Fig. 8, we present cases where M2PT demonstrates success on the MME and CIFAR-10, while other approaches fail (i.e., VPT, PTUM). For example, on MME, while M2PT correctly identifies “a chair” in the image, both VPT and PTUM fail to capture this concept and respond with the wrong answer. On CIFAR-10, VPT and PTUM both misidentify “cat” as a different category. We posit that the insufficiency of VPT may stem from its inadequate understanding of logical relationships or causal scenarios. PTUM employs single-modality tuning exclusively, rendering it inadequate for managing complex multi-modal behavior and capturing interactions between modalities. In sharp contrast, M2PT takes an aspect of multimodal, enhancing both visual modality comprehension and textual modality causal inference.
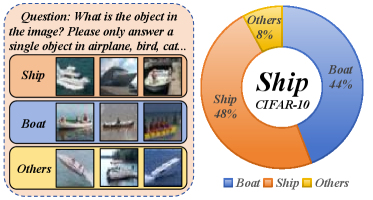
We further conduct failure case studies for M2PT on CIFAR-10 dataset. The results in Fig. 9 indicate that the predominant misclassification within the CIFAR-10 (i.e., 44% or 443 examples of all such misclassification) is the misidentification of “ships” as “boats”. This high error rate could potentially be attributed to the limited resolution of the images within the CIFAR-10, coupled with the inherent similarity in the semantic characteristics between “ship” and “boat”. Additional case studies and discussions are provided in Appendix S4.
6 Conclusion
We introduce M2PT, a novel framework in multimodal prompt tuning for zero-shot instruction learning. Our framework offers several advantages: i) it introduces visual and textual prompts elegantly into vision encoder and LLM, respectively, enabling fast and accurate multimodal adaptation; ii) it synergizes modalities by cross-modality interaction, enjoying coherent integration from multimodal perspectives; and iii) it significantly reduces the number of trainable parameters compared to conventional finetuning methods, while maintaining robust performance across zero-shot tasks. As a whole, we conclude that the outcomes elucidated in this paper impart essential understandings and necessitate further exploration within this realm.
Limitations
For potential limitations, M2PT requires two hyperparameters on prompt length searching (i.e., Visual Prompt, Textual Prompt). Though in practice, we find both lengths vary into a relatively narrow range (see §5), and are sufficient enough to outperform current methods, there is still possible integration He et al. (2022b) of a local searching network to generate optimal combinations of lengths. Another potential limitation is that M2PT, akin to other PEFT approaches Han et al. (2023); Jia et al. (2022), lacks ad-hoc explainability Biehl et al. (2016); Wang et al. (2023b). While in §4, we demonstrates that activation maps from MLLMs are significantly influenced by visual and textual prompts, further research is necessary to elucidate the underlying nature of these prompts.
7 Acknowledgements
This research was supported by the National Science Foundation under Grant No. 2242243. The views and conclusions contained herein are those of the authors and should not be interpreted as necessarily representing the official policies or endorsements, either expressed or implied, of U.S. Naval Research Laboratory (NRL) or the U.S. Government. The U.S. Government is authorized to reproduce and distribute reprints for Government purposes notwithstanding any copyright notation herein.
References
- Alayrac et al. (2022) Jean-Baptiste Alayrac, Jeff Donahue, Pauline Luc, Antoine Miech, Iain Barr, Yana Hasson, Karel Lenc, Arthur Mensch, Katherine Millican, Malcolm Reynolds, Roman Ring, Eliza Rutherford, Serkan Cabi, Tengda Han, Zhitao Gong, Sina Samangooei, Marianne Monteiro, Jacob L. Menick, Sebastian Borgeaud, Andy Brock, Aida Nematzadeh, Sahand Sharifzadeh, Mikolaj Binkowski, Ricardo Barreira, Oriol Vinyals, Andrew Zisserman, and Karén Simonyan. 2022. Flamingo: a visual language model for few-shot learning. In Advances in Neural Information Processing Systems 35: Annual Conference on Neural Information Processing Systems 2022, NeurIPS 2022, New Orleans, LA, USA, November 28 - December 9, 2022.
- Antol et al. (2015) Stanislaw Antol, Aishwarya Agrawal, Jiasen Lu, Margaret Mitchell, Dhruv Batra, C. Lawrence Zitnick, and Devi Parikh. 2015. VQA: visual question answering. In 2015 IEEE International Conference on Computer Vision, ICCV 2015, Santiago, Chile, December 7-13, 2015, pages 2425–2433. IEEE Computer Society.
- Arrieta et al. (2020) Alejandro Barredo Arrieta, Natalia Díaz Rodríguez, Javier Del Ser, Adrien Bennetot, Siham Tabik, Alberto Barbado, Salvador García, Sergio Gil-Lopez, Daniel Molina, Richard Benjamins, Raja Chatila, and Francisco Herrera. 2020. Explainable artificial intelligence (XAI): concepts, taxonomies, opportunities and challenges toward responsible AI. Inf. Fusion, 58:82–115.
- Biehl et al. (2016) Michael Biehl, Barbara Hammer, and Thomas Villmann. 2016. Prototype-based models in machine learning. Wiley Interdisciplinary Reviews: Cognitive Science, 7(2):92–111.
- Brown et al. (2020) Tom B. Brown, Benjamin Mann, Nick Ryder, Melanie Subbiah, Jared Kaplan, Prafulla Dhariwal, Arvind Neelakantan, Pranav Shyam, Girish Sastry, Amanda Askell, Sandhini Agarwal, Ariel Herbert-Voss, Gretchen Krueger, Tom Henighan, Rewon Child, Aditya Ramesh, Daniel M. Ziegler, Jeffrey Wu, Clemens Winter, Christopher Hesse, Mark Chen, Eric Sigler, Mateusz Litwin, Scott Gray, Benjamin Chess, Jack Clark, Christopher Berner, Sam McCandlish, Alec Radford, Ilya Sutskever, and Dario Amodei. 2020. Language models are few-shot learners. In Advances in Neural Information Processing Systems 33: Annual Conference on Neural Information Processing Systems 2020, NeurIPS 2020, December 6-12, 2020, virtual.
- Chen et al. (2024) Mingkai Chen, Taowen Wang, James Chenhao Liang, Chuan Liu, Chunshu Wu, Qifan Wang, Ying Nian Wu, Michael Huang, Chuang Ren, Ang Li, Tong Geng, and Dongfang Liu. 2024. Inertial confinement fusion forecasting via llms. CoRR, abs/2407.11098.
- Chen et al. (2021) Xinlei Chen, Saining Xie, and Kaiming He. 2021. An empirical study of training self-supervised vision transformers. In 2021 IEEE/CVF International Conference on Computer Vision, ICCV 2021, Montreal, QC, Canada, October 10-17, 2021, pages 9620–9629. IEEE.
- Chowdhury et al. (2023) Sanjoy Chowdhury, Sayan Nag, and Dinesh Manocha. 2023. Apollo : Unified adapter and prompt learning for vision language models. In Proceedings of the 2023 Conference on Empirical Methods in Natural Language Processing, EMNLP 2023, Singapore, December 6-10, 2023, pages 10173–10187. Association for Computational Linguistics.
- Cornia et al. (2020) Marcella Cornia, Matteo Stefanini, Lorenzo Baraldi, and Rita Cucchiara. 2020. Meshed-memory transformer for image captioning. In 2020 IEEE/CVF Conference on Computer Vision and Pattern Recognition, CVPR 2020, Seattle, WA, USA, June 13-19, 2020, pages 10575–10584. Computer Vision Foundation / IEEE.
- Dai et al. (2023) Wenliang Dai, Junnan Li, Dongxu Li, Anthony Meng Huat Tiong, Junqi Zhao, Weisheng Wang, Boyang Li, Pascale Fung, and Steven C. H. Hoi. 2023. Instructblip: Towards general-purpose vision-language models with instruction tuning. In Advances in Neural Information Processing Systems 36: Annual Conference on Neural Information Processing Systems 2023, NeurIPS 2023, New Orleans, LA, USA, December 10 - 16, 2023.
- Deng (2012) Li Deng. 2012. The MNIST database of handwritten digit images for machine learning research [best of the web]. IEEE Signal Process. Mag., 29(6):141–142.
- Dong et al. (2023a) Shaohua Dong, Yunhe Feng, Qing Yang, Yan Huang, Dongfang Liu, and Heng Fan. 2023a. Efficient multimodal semantic segmentation via dual-prompt learning. CoRR, abs/2312.00360.
- Dong et al. (2023b) Wei Dong, Dawei Yan, Zhijun Lin, and Peng Wang. 2023b. Efficient adaptation of large vision transformer via adapter re-composing. In Advances in Neural Information Processing Systems 36: Annual Conference on Neural Information Processing Systems 2023, NeurIPS 2023, New Orleans, LA, USA, December 10 - 16, 2023.
- Dosovitskiy et al. (2021) Alexey Dosovitskiy, Lucas Beyer, Alexander Kolesnikov, Dirk Weissenborn, Xiaohua Zhai, Thomas Unterthiner, Mostafa Dehghani, Matthias Minderer, Georg Heigold, Sylvain Gelly, Jakob Uszkoreit, and Neil Houlsby. 2021. An image is worth 16x16 words: Transformers for image recognition at scale. In 9th International Conference on Learning Representations, ICLR 2021, Virtual Event, Austria, May 3-7, 2021. OpenReview.net.
- Driess et al. (2023) Danny Driess, Fei Xia, Mehdi S. M. Sajjadi, Corey Lynch, Aakanksha Chowdhery, Brian Ichter, Ayzaan Wahid, Jonathan Tompson, Quan Vuong, Tianhe Yu, Wenlong Huang, Yevgen Chebotar, Pierre Sermanet, Daniel Duckworth, Sergey Levine, Vincent Vanhoucke, Karol Hausman, Marc Toussaint, Klaus Greff, Andy Zeng, Igor Mordatch, and Pete Florence. 2023. Palm-e: An embodied multimodal language model. In International Conference on Machine Learning, ICML 2023, 23-29 July 2023, Honolulu, Hawaii, USA, volume 202 of Proceedings of Machine Learning Research, pages 8469–8488. PMLR.
- Dumas et al. (2009) Bruno Dumas, Denis Lalanne, and Sharon L. Oviatt. 2009. Multimodal interfaces: A survey of principles, models and frameworks. In Denis Lalanne and Jürg Kohlas, editors, Human Machine Interaction, Research Results of the MMI Program, volume 5440 of Lecture Notes in Computer Science, pages 3–26. Springer.
- Fu et al. (2023) Chaoyou Fu, Peixian Chen, Yunhang Shen, Yulei Qin, Mengdan Zhang, Xu Lin, Zhenyu Qiu, Wei Lin, Jinrui Yang, Xiawu Zheng, Ke Li, Xing Sun, and Rongrong Ji. 2023. MME: A comprehensive evaluation benchmark for multimodal large language models. CoRR, abs/2306.13394.
- Glorot and Bengio (2010) Xavier Glorot and Yoshua Bengio. 2010. Understanding the difficulty of training deep feedforward neural networks. In Proceedings of the Thirteenth International Conference on Artificial Intelligence and Statistics, AISTATS 2010, Chia Laguna Resort, Sardinia, Italy, May 13-15, 2010, volume 9 of JMLR Proceedings, pages 249–256. JMLR.org.
- Han et al. (2024a) Cheng Han, James Chenhao Liang, Qifan Wang, Majid Rabbani, Sohail A. Dianat, Raghuveer Rao, Ying Nian Wu, and Dongfang Liu. 2024a. Image translation as diffusion visual programmers. In The Twelfth International Conference on Learning Representations, ICLR 2024, Vienna, Austria, May 7-11, 2024. OpenReview.net.
- Han et al. (2023) Cheng Han, Qifan Wang, Yiming Cui, Zhiwen Cao, Wenguan Wang, Siyuan Qi, and Dongfang Liu. 2023. Evpt: An effective and efficient approach for visual prompt tuning. In IEEE/CVF International Conference on Computer Vision, ICCV 2023, Paris, France, October 1-6, 2023, pages 17445–17456. IEEE.
- Han et al. (2024b) Cheng Han, Qifan Wang, Yiming Cui, Wenguan Wang, Lifu Huang, Siyuan Qi, and Dongfang Liu. 2024b. Facing the elephant in the room: Visual prompt tuning or full finetuning? CoRR, abs/2401.12902.
- Han et al. (2024c) Cheng Han, Qifan Wang, Sohail A. Dianat, Majid Rabbani, Raghuveer M. Rao, Yi Fang, Qiang Guan, Lifu Huang, and Dongfang Liu. 2024c. AMD: automatic multi-step distillation of large-scale vision models. CoRR, abs/2407.04208.
- He et al. (2020) Sen He, Wentong Liao, Hamed R. Tavakoli, Michael Ying Yang, Bodo Rosenhahn, and Nicolas Pugeault. 2020. Image captioning through image transformer. In Computer Vision - ACCV 2020 - 15th Asian Conference on Computer Vision, Kyoto, Japan, November 30 - December 4, 2020, Revised Selected Papers, Part IV, volume 12625 of Lecture Notes in Computer Science, pages 153–169. Springer.
- He et al. (2023) Xuehai He, Chunyuan Li, Pengchuan Zhang, Jianwei Yang, and Xin Eric Wang. 2023. Parameter-efficient model adaptation for vision transformers. In Thirty-Seventh AAAI Conference on Artificial Intelligence, AAAI 2023, Thirty-Fifth Conference on Innovative Applications of Artificial Intelligence, IAAI 2023, Thirteenth Symposium on Educational Advances in Artificial Intelligence, EAAI 2023, Washington, DC, USA, February 7-14, 2023, pages 817–825. AAAI Press.
- He et al. (2022a) Yun He, Huaixiu Steven Zheng, Yi Tay, Jai Prakash Gupta, Yu Du, Vamsi Aribandi, Zhe Zhao, YaGuang Li, Zhao Chen, Donald Metzler, Heng-Tze Cheng, and Ed H. Chi. 2022a. Hyperprompt: Prompt-based task-conditioning of transformers. In International Conference on Machine Learning, ICML 2022, 17-23 July 2022, Baltimore, Maryland, USA, volume 162 of Proceedings of Machine Learning Research, pages 8678–8690. PMLR.
- He et al. (2022b) Yun He, Huaixiu Steven Zheng, Yi Tay, Jai Prakash Gupta, Yu Du, Vamsi Aribandi, Zhe Zhao, YaGuang Li, Zhao Chen, Donald Metzler, Heng-Tze Cheng, and Ed H. Chi. 2022b. Hyperprompt: Prompt-based task-conditioning of transformers. In International Conference on Machine Learning, ICML 2022, 17-23 July 2022, Baltimore, Maryland, USA, volume 162 of Proceedings of Machine Learning Research, pages 8678–8690. PMLR.
- Hu et al. (2022) Edward J. Hu, Yelong Shen, Phillip Wallis, Zeyuan Allen-Zhu, Yuanzhi Li, Shean Wang, Lu Wang, and Weizhu Chen. 2022. Lora: Low-rank adaptation of large language models. In The Tenth International Conference on Learning Representations, ICLR 2022, Virtual Event, April 25-29, 2022. OpenReview.net.
- Jia et al. (2022) Menglin Jia, Luming Tang, Bor-Chun Chen, Claire Cardie, Serge J. Belongie, Bharath Hariharan, and Ser-Nam Lim. 2022. Visual prompt tuning. CoRR, abs/2203.12119.
- Jia et al. (2021) Menglin Jia, Zuxuan Wu, Austin Reiter, Claire Cardie, Serge J. Belongie, and Ser-Nam Lim. 2021. Exploring visual engagement signals for representation learning. In 2021 IEEE/CVF International Conference on Computer Vision, ICCV 2021, Montreal, QC, Canada, October 10-17, 2021, pages 4186–4197. IEEE.
- Jie et al. (2023) Shibo Jie, Haoqing Wang, and Zhi-Hong Deng. 2023. Revisiting the parameter efficiency of adapters from the perspective of precision redundancy. In IEEE/CVF International Conference on Computer Vision, ICCV 2023, Paris, France, October 1-6, 2023, pages 17171–17180. IEEE.
- Jin et al. (2024a) Mingyu Jin, Haochen Xue, Zhenting Wang, Boming Kang, Ruosong Ye, Kaixiong Zhou, Mengnan Du, and Yongfeng Zhang. 2024a. ProLLM: Protein chain-of-thoughts enhanced LLM for protein-protein interaction prediction. In First Conference on Language Modeling.
- Jin et al. (2024b) Mingyu Jin, Qinkai Yu, Jingyuan Huang, Qingcheng Zeng, Zhenting Wang, Wenyue Hua, Haiyan Zhao, Kai Mei, Yanda Meng, Kaize Ding, Fan Yang, Mengnan Du, and Yongfeng Zhang. 2024b. Exploring concept depth: How large language models acquire knowledge at different layers? CoRR, abs/2404.07066.
- Jin et al. (2024c) Mingyu Jin, Qinkai Yu, Dong Shu, Chong Zhang, Lizhou Fan, Wenyue Hua, Suiyuan Zhu, Yanda Meng, Zhenting Wang, Mengnan Du, Yongfeng Zhang, and Yanda Meng. 2024c. Health-llm: Personalized retrieval-augmented disease prediction system. CoRR, abs/2402.00746.
- Jin et al. (2024d) Mingyu Jin, Qinkai Yu, Dong Shu, Haiyan Zhao, Wenyue Hua, Yanda Meng, Yongfeng Zhang, and Mengnan Du. 2024d. The impact of reasoning step length on large language models. In Findings of the Association for Computational Linguistics, ACL 2024, Bangkok, Thailand and virtual meeting, August 11-16, 2024, pages 1830–1842. Association for Computational Linguistics.
- Ju et al. (2022a) Chen Ju, Tengda Han, Kunhao Zheng, Ya Zhang, and Weidi Xie. 2022a. Prompting visual-language models for efficient video understanding. In Computer Vision - ECCV 2022 - 17th European Conference, Tel Aviv, Israel, October 23-27, 2022, Proceedings, Part XXXV, volume 13695 of Lecture Notes in Computer Science, pages 105–124. Springer.
- Ju et al. (2022b) Chen Ju, Tengda Han, Kunhao Zheng, Ya Zhang, and Weidi Xie. 2022b. Prompting visual-language models for efficient video understanding. In Computer Vision - ECCV 2022 - 17th European Conference, Tel Aviv, Israel, October 23-27, 2022, Proceedings, Part XXXV, volume 13695 of Lecture Notes in Computer Science, pages 105–124. Springer.
- Khattak et al. (2023) Muhammad Uzair Khattak, Hanoona Abdul Rasheed, Muhammad Maaz, Salman H. Khan, and Fahad Shahbaz Khan. 2023. Maple: Multi-modal prompt learning. In IEEE/CVF Conference on Computer Vision and Pattern Recognition, CVPR 2023, Vancouver, BC, Canada, June 17-24, 2023, pages 19113–19122. IEEE.
- Krizhevsky et al. (2009) Alex Krizhevsky, Geoffrey Hinton, et al. 2009. Learning multiple layers of features from tiny images. pages 32–33.
- Laugel et al. (2019) Thibault Laugel, Marie-Jeanne Lesot, Christophe Marsala, Xavier Renard, and Marcin Detyniecki. 2019. The dangers of post-hoc interpretability: Unjustified counterfactual explanations. In Proceedings of the Twenty-Eighth International Joint Conference on Artificial Intelligence, IJCAI 2019, Macao, China, August 10-16, 2019, pages 2801–2807. ijcai.org.
- Lester et al. (2021) Brian Lester, Rami Al-Rfou, and Noah Constant. 2021. The power of scale for parameter-efficient prompt tuning. In Proceedings of the 2021 Conference on Empirical Methods in Natural Language Processing, EMNLP 2021, Virtual Event / Punta Cana, Dominican Republic, 7-11 November, 2021, pages 3045–3059. Association for Computational Linguistics.
- Li et al. (2024a) Chunyuan Li, Zhe Gan, Zhengyuan Yang, Jianwei Yang, Linjie Li, Lijuan Wang, and Jianfeng Gao. 2024a. Multimodal foundation models: From specialists to general-purpose assistants. Found. Trends Comput. Graph. Vis., 16(1-2):1–214.
- Li et al. (2024b) Juncheng Li, Kaihang Pan, Zhiqi Ge, Minghe Gao, Wei Ji, Wenqiao Zhang, Tat-Seng Chua, Siliang Tang, Hanwang Zhang, and Yueting Zhuang. 2024b. Fine-tuning multimodal llms to follow zero-shot demonstrative instructions. Preprint, arXiv:2308.04152.
- Li et al. (2022) Junnan Li, Dongxu Li, Caiming Xiong, and Steven C. H. Hoi. 2022. BLIP: bootstrapping language-image pre-training for unified vision-language understanding and generation. In International Conference on Machine Learning, ICML 2022, 17-23 July 2022, Baltimore, Maryland, USA, volume 162 of Proceedings of Machine Learning Research, pages 12888–12900. PMLR.
- Li et al. (2023) Yifan Li, Yifan Du, Kun Zhou, Jinpeng Wang, Wayne Xin Zhao, and Ji-Rong Wen. 2023. Evaluating object hallucination in large vision-language models. In Proceedings of the 2023 Conference on Empirical Methods in Natural Language Processing, EMNLP 2023, Singapore, December 6-10, 2023, pages 292–305. Association for Computational Linguistics.
- Liang et al. (2022) Sheng Liang, Mengjie Zhao, and Hinrich Schütze. 2022. Modular and parameter-efficient multimodal fusion with prompting. In Findings of the Association for Computational Linguistics: ACL 2022, Dublin, Ireland, May 22-27, 2022, pages 2976–2985. Association for Computational Linguistics.
- Liu et al. (2023a) Fangyu Liu, Guy Emerson, and Nigel Collier. 2023a. Visual spatial reasoning. Transactions of the Association for Computational Linguistics, 11:635–651.
- Liu et al. (2023b) Haotian Liu, Chunyuan Li, Yuheng Li, and Yong Jae Lee. 2023b. Improved baselines with visual instruction tuning. CoRR, abs/2310.03744.
- Liu et al. (2023c) Haotian Liu, Chunyuan Li, Qingyang Wu, and Yong Jae Lee. 2023c. Visual instruction tuning. In Advances in Neural Information Processing Systems 36: Annual Conference on Neural Information Processing Systems 2023, NeurIPS 2023, New Orleans, LA, USA, December 10 - 16, 2023.
- Liu et al. (2023d) Pengfei Liu, Weizhe Yuan, Jinlan Fu, Zhengbao Jiang, Hiroaki Hayashi, and Graham Neubig. 2023d. Pre-train, prompt, and predict: A systematic survey of prompting methods in natural language processing. ACM Comput. Surv., 55(9):195:1–195:35.
- Liu et al. (2021) Xiao Liu, Kaixuan Ji, Yicheng Fu, Zhengxiao Du, Zhilin Yang, and Jie Tang. 2021. P-tuning v2: Prompt tuning can be comparable to fine-tuning universally across scales and tasks. CoRR, abs/2110.07602.
- Ma et al. (2022) Fang Ma, Chen Zhang, Lei Ren, Jingang Wang, Qifan Wang, Wei Wu, Xiaojun Quan, and Dawei Song. 2022. Xprompt: Exploring the extreme of prompt tuning. In Proceedings of the 2022 Conference on Empirical Methods in Natural Language Processing, EMNLP 2022, Abu Dhabi, United Arab Emirates, December 7-11, 2022, pages 11033–11047. Association for Computational Linguistics.
- Ouyang et al. (2022) Long Ouyang, Jeffrey Wu, Xu Jiang, Diogo Almeida, Carroll L. Wainwright, Pamela Mishkin, Chong Zhang, Sandhini Agarwal, Katarina Slama, Alex Ray, John Schulman, Jacob Hilton, Fraser Kelton, Luke Miller, Maddie Simens, Amanda Askell, Peter Welinder, Paul F. Christiano, Jan Leike, and Ryan Lowe. 2022. Training language models to follow instructions with human feedback. In Advances in Neural Information Processing Systems 35: Annual Conference on Neural Information Processing Systems 2022, NeurIPS 2022, New Orleans, LA, USA, November 28 - December 9, 2022.
- Paszke et al. (2019) Adam Paszke, Sam Gross, Francisco Massa, Adam Lerer, James Bradbury, Gregory Chanan, Trevor Killeen, Zeming Lin, Natalia Gimelshein, Luca Antiga, Alban Desmaison, Andreas Köpf, Edward Z. Yang, Zachary DeVito, Martin Raison, Alykhan Tejani, Sasank Chilamkurthy, Benoit Steiner, Lu Fang, Junjie Bai, and Soumith Chintala. 2019. Pytorch: An imperative style, high-performance deep learning library. In Advances in Neural Information Processing Systems 32: Annual Conference on Neural Information Processing Systems 2019, NeurIPS 2019, December 8-14, 2019, Vancouver, BC, Canada, pages 8024–8035.
- Qiu et al. (2020) Xipeng Qiu, Tianxiang Sun, Yige Xu, Yunfan Shao, Ning Dai, and Xuanjing Huang. 2020. Pre-trained models for natural language processing: A survey. CoRR, abs/2003.08271.
- Radford et al. (2021) Alec Radford, Jong Wook Kim, Chris Hallacy, Aditya Ramesh, Gabriel Goh, Sandhini Agarwal, Girish Sastry, Amanda Askell, Pamela Mishkin, Jack Clark, Gretchen Krueger, and Ilya Sutskever. 2021. Learning transferable visual models from natural language supervision. In Proceedings of the 38th International Conference on Machine Learning, ICML 2021, 18-24 July 2021, Virtual Event, volume 139 of Proceedings of Machine Learning Research, pages 8748–8763. PMLR.
- Rudin (2019) Cynthia Rudin. 2019. Stop explaining black box machine learning models for high stakes decisions and use interpretable models instead. Nat. Mach. Intell., 1(5):206–215.
- Shen et al. (2024) Ying Shen, Zhiyang Xu, Qifan Wang, Yu Cheng, Wenpeng Yin, and Lifu Huang. 2024. Multimodal instruction tuning with conditional mixture of lora. CoRR, abs/2402.15896.
- Singh et al. (2019) Amanpreet Singh, Vivek Natarajan, Meet Shah, Yu Jiang, Xinlei Chen, Dhruv Batra, Devi Parikh, and Marcus Rohrbach. 2019. Towards VQA models that can read. In IEEE Conference on Computer Vision and Pattern Recognition, CVPR 2019, Long Beach, CA, USA, June 16-20, 2019, pages 8317–8326. Computer Vision Foundation / IEEE.
- Sun et al. (2024a) Guangyan Sun, Mingyu Jin, Zhenting Wang, Cheng-Long Wang, Siqi Ma, Qifan Wang, Ying Nian Wu, Yongfeng Zhang, and Dongfang Liu. 2024a. Visual agents as fast and slow thinkers. CoRR, abs/2408.08862.
- Sun et al. (2024b) Mingjie Sun, Xinlei Chen, J. Zico Kolter, and Zhuang Liu. 2024b. Massive activations in large language models. CoRR, abs/2402.17762.
- Sun et al. (2023) Quan Sun, Yuxin Fang, Ledell Wu, Xinlong Wang, and Yue Cao. 2023. EVA-CLIP: improved training techniques for CLIP at scale. CoRR, abs/2303.15389.
- Wang et al. (2023a) Qifan Wang, Yuning Mao, Jingang Wang, Hanchao Yu, Shaoliang Nie, Sinong Wang, Fuli Feng, Lifu Huang, Xiaojun Quan, Zenglin Xu, and Dongfang Liu. 2023a. Aprompt: Attention prompt tuning for efficient adaptation of pre-trained language models. In Proceedings of the 2023 Conference on Empirical Methods in Natural Language Processing, EMNLP 2023, Singapore, December 6-10, 2023, pages 9147–9160. Association for Computational Linguistics.
- Wang et al. (2023b) Wenguan Wang, Cheng Han, Tianfei Zhou, and Dongfang Liu. 2023b. Visual recognition with deep nearest centroids. In The Eleventh International Conference on Learning Representations, ICLR 2023, Kigali, Rwanda, May 1-5, 2023. OpenReview.net.
- Wang et al. (2022) Zifeng Wang, Zizhao Zhang, Sayna Ebrahimi, Ruoxi Sun, Han Zhang, Chen-Yu Lee, Xiaoqi Ren, Guolong Su, Vincent Perot, Jennifer G. Dy, and Tomas Pfister. 2022. Dualprompt: Complementary prompting for rehearsal-free continual learning. In Computer Vision - ECCV 2022 - 17th European Conference, Tel Aviv, Israel, October 23-27, 2022, Proceedings, Part XXVI, volume 13686 of Lecture Notes in Computer Science, pages 631–648. Springer.
- Wu et al. (2024a) Qiong Wu, Weihao Ye, Yiyi Zhou, Xiaoshuai Sun, and Rongrong Ji. 2024a. Not all attention is needed: Parameter and computation efficient transfer learning for multi-modal large language models. CoRR, abs/2403.15226.
- Wu et al. (2024b) Zichen Wu, Hsiu-Yuan Huang, Fanyi Qu, and Yunfang Wu. 2024b. Mixture-of-prompt-experts for multi-modal semantic understanding. In Proceedings of the 2024 Joint International Conference on Computational Linguistics, Language Resources and Evaluation, LREC/COLING 2024, 20-25 May, 2024, Torino, Italy, pages 11381–11393. ELRA and ICCL.
- Xie et al. (2019) Ning Xie, Farley Lai, Derek Doran, and Asim Kadav. 2019. Visual entailment: A novel task for fine-grained image understanding. CoRR, abs/1901.06706.
- Xu et al. (2024a) Lin Xu, Yilin Zhao, Daquan Zhou, Zhijie Lin, See-Kiong Ng, and Jiashi Feng. 2024a. Pllava : Parameter-free llava extension from images to videos for video dense captioning. CoRR, abs/2404.16994.
- Xu et al. (2024b) Zhiyang Xu, Chao Feng, Rulin Shao, Trevor Ashby, Ying Shen, Di Jin, Yu Cheng, Qifan Wang, and Lifu Huang. 2024b. Vision-flan: Scaling human-labeled tasks in visual instruction tuning. CoRR, abs/2402.11690.
- Xu et al. (2023) Zhiyang Xu, Ying Shen, and Lifu Huang. 2023. Multiinstruct: Improving multi-modal zero-shot learning via instruction tuning. In Proceedings of the 61st Annual Meeting of the Association for Computational Linguistics (Volume 1: Long Papers), ACL 2023, Toronto, Canada, July 9-14, 2023, pages 11445–11465. Association for Computational Linguistics.
- Yan et al. (2023) Liqi Yan, Cheng Han, Zenglin Xu, Dongfang Liu, and Qifan Wang. 2023. Prompt learns prompt: Exploring knowledge-aware generative prompt collaboration for video captioning. In Proceedings of the Thirty-Second International Joint Conference on Artificial Intelligence, IJCAI 2023, 19th-25th August 2023, Macao, SAR, China, pages 1622–1630. ijcai.org.
- Yang et al. (2023a) Hao Yang, Junyang Lin, An Yang, Peng Wang, and Chang Zhou. 2023a. Prompt tuning for unified multimodal pretrained models. In Findings of the Association for Computational Linguistics: ACL 2023, Toronto, Canada, July 9-14, 2023, pages 402–416. Association for Computational Linguistics.
- Yang et al. (2023b) Li Yang, Qifan Wang, Jingang Wang, Xiaojun Quan, Fuli Feng, Yu Chen, Madian Khabsa, Sinong Wang, Zenglin Xu, and Dongfang Liu. 2023b. Mixpave: Mix-prompt tuning for few-shot product attribute value extraction. In Findings of the Association for Computational Linguistics: ACL 2023, Toronto, Canada, July 9-14, 2023, pages 9978–9991. Association for Computational Linguistics.
- Yao et al. (2023) Hantao Yao, Rui Zhang, and Changsheng Xu. 2023. Visual-language prompt tuning with knowledge-guided context optimization. In IEEE/CVF Conference on Computer Vision and Pattern Recognition, CVPR 2023, Vancouver, BC, Canada, June 17-24, 2023, pages 6757–6767. IEEE.
- Yin et al. (2023) Shukang Yin, Chaoyou Fu, Sirui Zhao, Ke Li, Xing Sun, Tong Xu, and Enhong Chen. 2023. A survey on multimodal large language models. CoRR, abs/2306.13549.
- Zhai et al. (2023) Yuexiang Zhai, Shengbang Tong, Xiao Li, Mu Cai, Qing Qu, Yong Jae Lee, and Yi Ma. 2023. Investigating the catastrophic forgetting in multimodal large language models. CoRR, abs/2309.10313.
- Zhang et al. (2024) Chong Zhang, Xinyi Liu, Mingyu Jin, Zhongmou Zhang, Lingyao Li, Zhenting Wang, Wenyue Hua, Dong Shu, Suiyuan Zhu, Xiaobo Jin, Sujian Li, Mengnan Du, and Yongfeng Zhang. 2024. When AI meets finance (stockagent): Large language model-based stock trading in simulated real-world environments. CoRR, abs/2407.18957.
- Zhang et al. (2023) Shengyu Zhang, Linfeng Dong, Xiaoya Li, Sen Zhang, Xiaofei Sun, Shuhe Wang, Jiwei Li, Runyi Hu, Tianwei Zhang, Fei Wu, and Guoyin Wang. 2023. Instruction tuning for large language models: A survey. CoRR, abs/2308.10792.
- Zhao et al. (2023) Wayne Xin Zhao, Kun Zhou, Junyi Li, Tianyi Tang, Xiaolei Wang, Yupeng Hou, Yingqian Min, Beichen Zhang, Junjie Zhang, Zican Dong, Yifan Du, Chen Yang, Yushuo Chen, Zhipeng Chen, Jinhao Jiang, Ruiyang Ren, Yifan Li, Xinyu Tang, Zikang Liu, Peiyu Liu, Jian-Yun Nie, and Ji-Rong Wen. 2023. A survey of large language models. CoRR, abs/2303.18223.
- Zheng et al. (2023) Lianmin Zheng, Wei-Lin Chiang, Ying Sheng, Siyuan Zhuang, Zhanghao Wu, Yonghao Zhuang, Zi Lin, Zhuohan Li, Dacheng Li, Eric P. Xing, Hao Zhang, Joseph E. Gonzalez, and Ion Stoica. 2023. Judging llm-as-a-judge with mt-bench and chatbot arena. In Advances in Neural Information Processing Systems 36: Annual Conference on Neural Information Processing Systems 2023, NeurIPS 2023, New Orleans, LA, USA, December 10 - 16, 2023.
- Zhou et al. (2022a) Kaiyang Zhou, Jingkang Yang, Chen Change Loy, and Ziwei Liu. 2022a. Conditional prompt learning for vision-language models. In IEEE/CVF Conference on Computer Vision and Pattern Recognition (CVPR).
- Zhou et al. (2022b) Kaiyang Zhou, Jingkang Yang, Chen Change Loy, and Ziwei Liu. 2022b. Learning to prompt for vision-language models. International Journal of Computer Vision (IJCV).
SUMMARY OF THE APPENDIX
This appendix contains additional experimental results and discussions of our work, organized as:
-
•
§S1 includes additional introduction on datasets applied in our paper.
-
•
§S2 provides more training details on our proposed M2PT.
- •
-
•
§S4 further includes more case studies of M2PT on its perceptual proficiency.
-
•
§S5 discuss the relations of M2PT with previous works and their connections.
-
•
§S6 provides discussions and additional results on the impact of prompt initialization.
-
•
§S7 provides discussions on licenses, reproducibility, social impact, and directions of our future work.
Appendix S1 Data Statistics
Table S1 shows details of 9 multimodal datasets for our finetuning and evaluation. Vision-Flan Xu et al. (2024b) includes 191 different multimodal tasks which is ideal for our finetuning process. Each multimodal tasks contains up to 1,000 instances, resulting in a total of 191,105 instances. MME Yin et al. (2023) serves as our comprehensive multimodal evaluation benchmark, measuring both perception and cognition capabilities across 14 subtasks. We further leverage 7 multimodal datasets for our evaluation. Specifically, for Optical Character Recognition, we utilize the Text-VQA Singh et al. (2019), and for reasoning, we employ the Visual Spatial Reasoning (VSR) Liu et al. (2023a). Following Zhai et al. (2023); Shen et al. (2024), the perception capability is tested on CIFAR-10/100 Krizhevsky et al. (2009) and MNIST Deng (2012). SNLI-VE Xie et al. (2019) evaluates Visual Entailment capabilities, while the POPE Li et al. (2023) dataset examines the tendency towards object hallucination. The MME metric is the sum of accuracy values across all subtasks, while for the other 7 multimodal evaluation datasets, the metric used is just accuracy.
Dataset Examples Task Categories Vision-Flan 191K Diverse MME 2374 Diverse Text-VQA 5000 OCR VSR 1222 Reasoning SNLI-VE 17901 Entailment CIFAR-10 10000 Perception CIFAR-100 10000 Perception MNIST 10000 Perception POPE 9000 Object Hallucination
Appendix S2 Implementation Details
Stage-one LLaVA Liu et al. (2023c) is utilized as our pre-trained multimodal model. Specifically, we employ LLaVA with Vicuna-7B-v1.3 as the base LLM and CLIP-L as the vision encoder for all variants. The finetuning process for M2PT10/20 takes approximately 9 hours on 4 A100 GPUs (40G), with a batch size of 8 per GPU and a gradient accumulation step of 4 (128 in total), with the same data preprocess and normalize method as LLaVA. Additional configurations of M2PT are shown in Table S2. For LoRA, we directly import the best results from Shen et al. (2024). For the prompt tuning baselines, APrompt Wang et al. (2023a) and PTUM Yang et al. (2023a) add textual/attention prompts into the LLM, while VPT Han et al. (2024b) only appends visual prompts to the vision encoder. We use the optimal settings in the original papers to train their models, with grid search on the best learning rate. For the other configuration, we adopt LLaVA’s Liu et al. (2023c) default settings as provided in its codebase.
Learning Rate | |
Batch Size | 128 |
Lr Scheduler | cosine |
Warmup Ratio | 0.03 |
Activation Type | bfloat16 |
Weight Decay | 0 |
Model Max Length | 1024 |
Appendix S3 Evaluation Metrics
For evluation, we utilize MME Yin et al. (2023) and the other 7 multimodal datasets (see §S1). For MME, we employ the official evaluation tool Yin et al. (2023) of MME, including the Perception and Cognition metrics. For the other 7 multimodal datasets, following Shen et al. (2024), we employ the same prompt template to guide Vicuna-13B-v1.5 Zheng et al. (2023) in evaluating the accuracy of each prediction, considering the specified task instructions and the groundtruth target output. All tasks are classification tasks and we calculated the final score of each multimodal dataset based on the percentage of vicuna 13b answering “Yes.”
Appendix S4 More Case Study
To further investigate the model’s performance and delineate instances of its suboptimal functioning, we conduct an in-depth visual assessment of a sample cohort drawn from eight distinct Zero-Shot datasets, as illustrated in Fig. S1. This visualization facilitates a comprehensive understanding of the model’s efficacy across a diverse array of tasks and data, while concurrently revealing potential constraints inherent to the model and the underlying causes of its occasional shortcomings. From the listed failure cases, we summarize 2 failure patterns, which are: (a) Small objects perception failure in Text-VQA, CIFAR-10, MNIST. Small targets in images pose a challenge to perception, impacting the quality of VQA. Further research is essential to enhance accuracy in diverse contexts. (b) Semantic similar failure in CIFAR-10, MNIST, POPE, the inability to distinguish between semantically similar objects results in MLLMs generating wrong answers. Developing methods that can effectively differentiate between similar objects is essential for real-world applications. This involves enhancing the model’s capacity to learn fine-grained features and contextual information, thereby improving its overall accuracy and robustness.
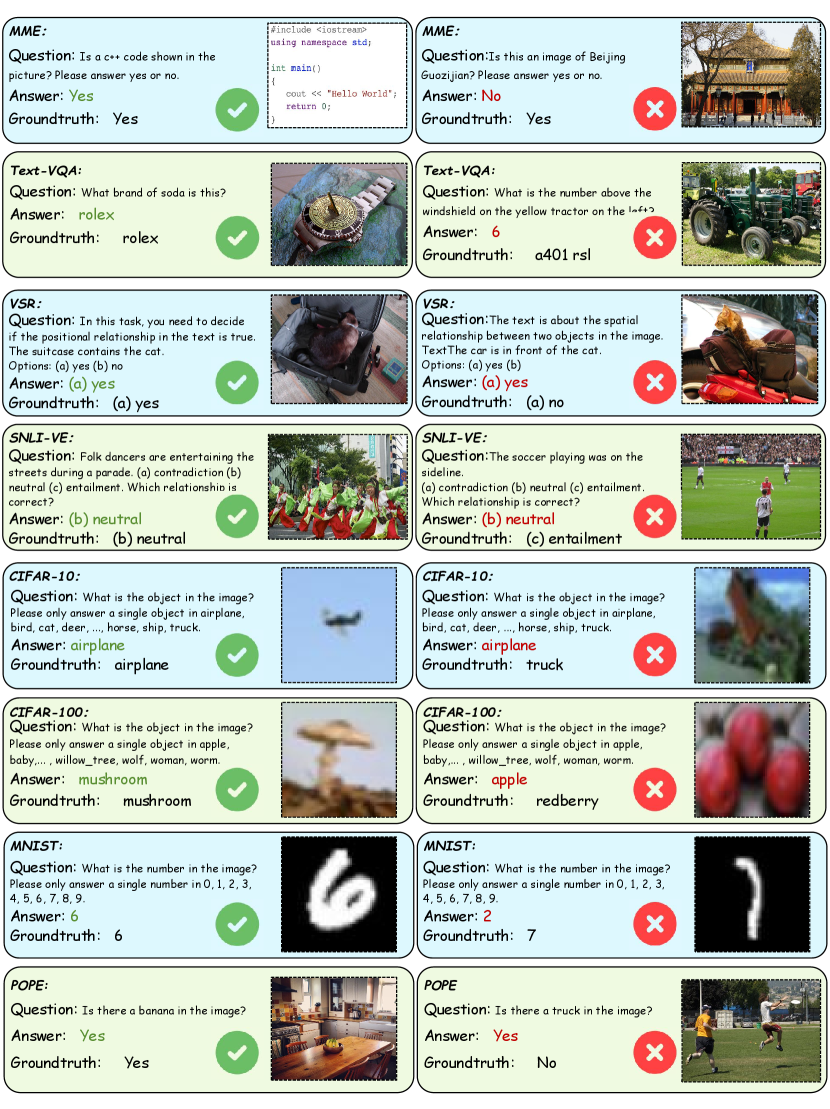
Appendix S5 Discussion with Previous Works
This section provides discussion that connects M2PT with previous methods. If we remove the textual prompts and the interaction layer, our model architecture degenerates to the visual prompt tuning approaches Jia et al. (2022); Han et al. (2024b, 2023). If we completely freeze the vision encoder by only introducing the textual prompts, our model is similar to those traditional prompt tuning methods Lester et al. (2021); Ma et al. (2022); Yang et al. (2023a) in LLM. Moreover, if we further incorporate the attention prompts in the LLM, our model is close to the APrompt approach Wang et al. (2023a); Han et al. (2023). Nevertheless, very limited work focuses on the efficient tuning of multimodal large language models.
Method Initialization MME M2PT10/20 Random 1405.67 M2PT10/20 Xavier 1503.98
Appendix S6 Prompt Initialization
Table S3 reports the performance of M2PT with respect to x widely adopted initialization methods: Xavier Glorot and Bengio (2010) and random on MME. The results show that Xavier generally provides more stable and preferable performances. In conclusion, M2PT shows robustness on different initialization methods and is able to achieve comparable performance with full finetuning.
Appendix S7 Discussion
S7.1 Asset License and Consent
The majority of VPT Jia et al. (2022), Text-VQA, is licensed under CC-BY-NC 4.0. Portions of Jia et al. (2022) are available under separate license terms: google-research/task_adaptation, huggingface/transformers, LLaVA, Vicuna, VSR is licensed under Apache-2.0. ViT-pytorch Dosovitskiy et al. (2021) and POPE is licensed under MIT; SNLI-VE are under BSD 3-Clause
S7.2 Reproducibility
M2PT is implemented in Pytorch Paszke et al. (2019). Experiments are conducted on NVIDIA A100 GPUs. To guarantee reproducibility, our full implementation shall be publicly released upon paper acceptance.
S7.3 Social Impact
This work introduces M2PT possessing strong performance gains over state-of-the-art baselines in §4, with considerably low parameter usage for MLLMs. Our approach advances model accuracy, and is valuable in parameter-sensitive training applications, e.g., MLLMs on devices and fast adaptation with limited resources.
S7.4 Potential Risks
Consider the tuning process of LLM, which has potential risks for energy usage. Finetuning requires significant computational power, leading to high energy use and increased environmental impact.
S7.5 Future Work
Despite M2PT’s systemic effectiveness and efficacy during instruction tuning, it also comes with new challenges and unveils some intriguing questions. For instance, incorporating an advanced network into M2PT to search the optimal combinations of prompt lengths (i.e., Visual Prompt, Textual Prompt) might significantly reduce the search space of lengths and lead to further performance gains. Another essential future direction is the design and analysis of network interpretability Arrieta et al. (2020); Laugel et al. (2019); Rudin (2019) and ad-hoc explainability Biehl et al. (2016); Wang et al. (2023b), which limits current adoption of M2PT in decision-critical, real-world applications. Overall, we believe the results presented in this paper warrant further exploration.