Leveraging Open-Source Large Language Models for encoding Social Determinants of Health using an Intelligent Router
Abstract
Social Determinants of Health (SDOH) play a significant role in patient health outcomes. The Center of Disease Control (CDC) introduced a subset of ICD-10 codes called Z-codes in an attempt to officially recognize and measure SDOH in the health care system. However, these codes are rarely annotated in a patient’s Electronic Health Record (EHR), and instead, in many cases, need to be inferred from clinical notes. Previous research has shown that large language models (LLMs) show promise on extracting unstructured data from EHRs. However, with thousands of models to choose from with unique architectures and training sets, it’s difficult to choose one model that performs the best on coding tasks. Further, clinical notes contain trusted health information making the use of closed-source language models from commercial vendors difficult, so the identification of open source LLMs that can be run within health organizations and exhibits high performance on SDOH tasks is an urgent problem. Here, we introduce an intelligent routing system for SDOH coding that uses a language model router to direct medical record data to open source LLMs that demonstrate optimal performance on specific SDOH codes. The intelligent routing system exhibits state of the art performance of 97.4% accuracy averaged across 5 codes, including homelessness and food insecurity, on par with closed models such as GPT-4o. In order to train the routing system and validate models, we also introduce a synthetic data generation and validation paradigm to increase the scale of training data without needing privacy protected medical records. Together, we demonstrate an architecture for intelligent routing of inputs to task-optimal language models to achieve high performance across a set of medical coding sub-tasks.
1 Introduction
Social determinants of health (SDOH) are defined as non-medical factors that strongly influence health outcomes. SDOH are increasingly regarded as significant risk factors across a broad range of health outcomes and generate measures of well-being [14]. SDOH generally include a broad range of factors like economic stability and access to resources such as food, housing, education, health care. Ideally a holistic, whole-person approach to physical health should include a focus on these social determinants of health because it is not possible to treat acute or chronic conditions in a vacuum. For example, it may be challenging for an unhoused patient to afford, purchase, and administer medications for chronic conditions when their primary, daily survival focus is around finding a safe place to sleep at night. Given the central importance of SDOH, there is an increased focus on screening for SDOH and applying interventions in the form of social services to impact SDOH. An important limitation for SDOH scoring is related to data - historically, many of the notes related to SDOH domains were free-texted into the Social History section of the EMR, so it may be difficult to extract for both reporting and clinical intervention purposes.
Large Language Models (LLMs) show promise for extracting data from unstructured medical notes. They have already been used on a variety of clinical tasks such as predicting readmission rates from Electronic Health Records (EHRs) and answering clinical questions [2][12][11]. There has even been a publication to date using LLMs to identify generally ’adverse’ SDOH codes[4]. However, achieving high accuracy results generally use closed-model architectures like GPT4 or Claude, or require significant fine-tuning [4][10][12]. Closed-model architectures - LLMs whose training data, weights, and prompting strategy are proprietary, and are hosted on industrial cloud computing infrastructure, require the transmission of health data to be taken advantage of. As an alternative to closed source models, open source language models have proliferated in recent years with models like LLAMA and Mistral achieving performance on par with closed source models [13, 7]. However, there are currently over 100,000 open source language models on the Hugging Face platform, and it is difficult to discover open source LLMs that might be optimal for a given task [5, 6]. Further, fine-tuning open-source models requires valuable clinical data, where the lack thereof or quality-issues of this data can cause model performance to suffer [3].
Here we introduce an intelligent routing architecture that leverages multiple non-fine-tuned open-source LLMs to achieve state of the art accuracy. Our router not only navigates the problem of open-source models being subject to a variety of training architectures and possessing mixed quality data [15][1][8], but in fact takes advantage of this by choosing LLMs where training data may have lent itself better to higher accuracy on certain SDOH codes. We also introduce a new synthetic data generation and validation paradigm to address the issue of lack of high-quality clinical data that is often hidden behind privacy protected medical records. Our router system trained on this synthetic data, without fine-tuning or using closed-source models, is able to achieve 97.4% accuracy, on par with much bigger, top-of-the-line models like GPT-4o.
2 Results
Identification of open source models for specific SDOH codes
Language models are trained on distinct data domains and show differential performance on tasks including the identification of specific SDOH codes [5, 6]. SDOH codes span a variety of underlying topics from housing to family relationships to incarceration status. Hence, coding is well suited to an ensemble approach where expert models are used to analyze clinical notes for specific SDOH factors. Therefore, we developed an intelligent routing system for SDOH coding that consists of a router model and an ensemble of down-stream open source models that show expert performance on coding for specific SDOH factors.
To identify optimal open source models, we generated a data set that contains both 500 medical notes from the MIMIC-III dataset [9], as well as synthetic examples generated using an LLM. We, first, analyzed the performance of a set of open source language models on each of seven codes (Figure 1), and, then, trained a router to route incoming coding tasks to the optimal down-stream model (Figure 8).
Figure 1 shows the performance of ten open-source large language models (LLMs) across seven social determinants of health code (SDOH) on a dataset of 50 notes from the MIMIC-III database [9]. From this graph, we were able to identify at least one model that achieved >80% accuracy on each code, indicating overall that open-source LLMs have the ability to extract SDOH information from unstructured medical charts. However, we observed variable performance on these notes, where a different model performs the best on a given code. We also see that some codes, such as unemployment, demonstrate high accuracy across models, while others, like relative needing care, possess more variable and lower overall accuracy scores.
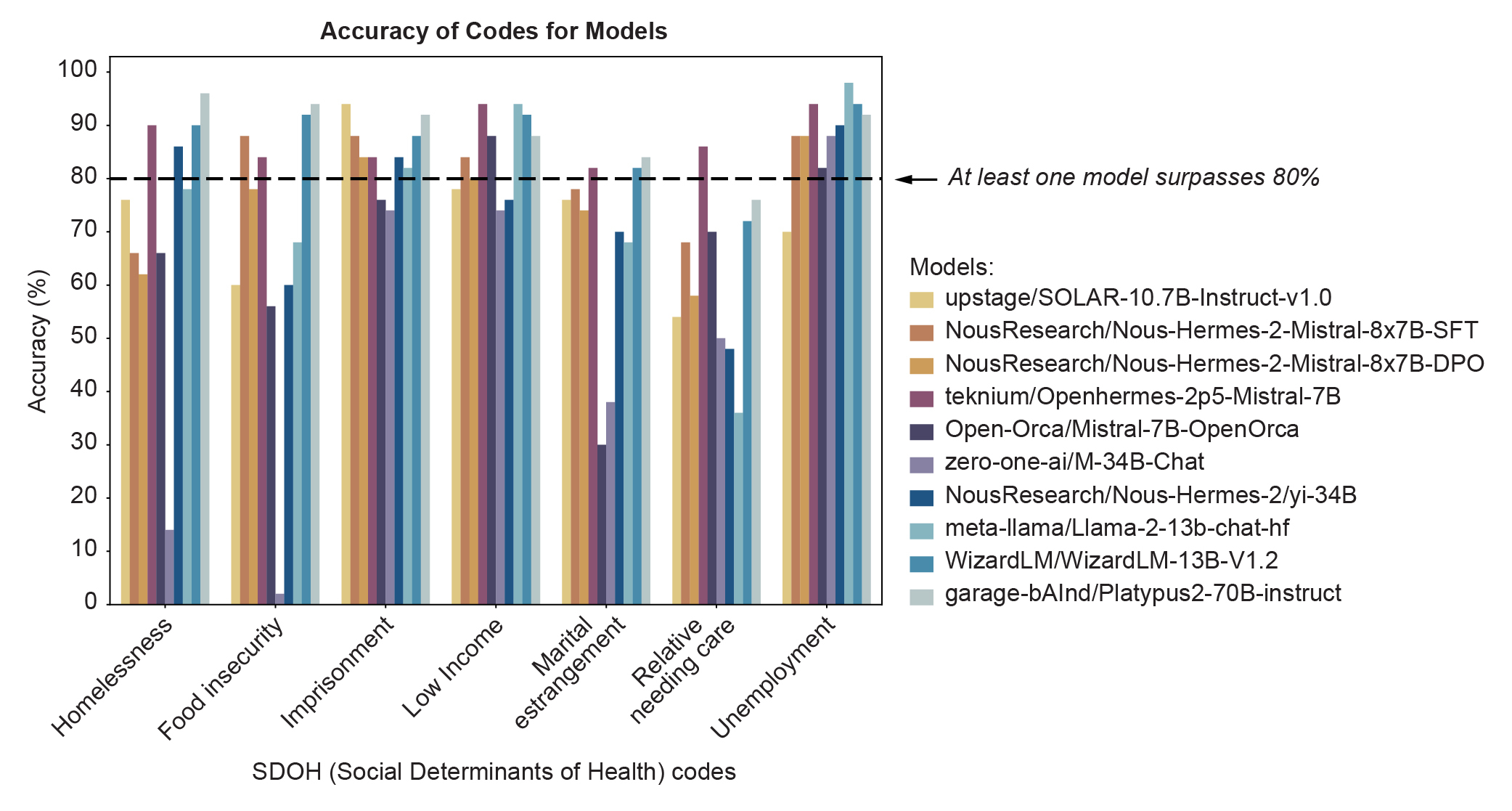
Only a few sentences from the medical notes (<1% of the sentences) contained evidence of specific SDOH codes, so we generated and validated synthetic data. Furthermore, after manually labeling 500 medical notes from the MIMIC-III dataset[9], we distilled our validation set to test only 5 SDOH codes (homelessness, food insecurity, imprisonment or other incarceration, marital estrangement, and relative needing care) for a proof of concept. Training a router to pick a best model for a given SDOH factor from the medical note, we observed the performance shown in Figure 3. On a 1000 sentence validation set, containing sentences from 500 labeled medical notes found in the MIMIC-III dataset [9] and synthetic data, our router picked a model that achieved >90% accuracy and F1 score for every single code. The highest performing code was homelessness, where the router picked model identified this code with 99.0% accuracy (0.984 F1 score). The lowest performing code was imprisonment or other incarceration, at 94.7% accuracy (0.918 F1 score). Comparing the performance of the router picked model to GPT-4o, our chosen model beats that of GPT for 4 of the 5 codes, only exhibiting marginally worse performance for the relative needing care SDOH factor (97.5% vs 97.8% accuracy for our router picked model vs GPT-4o).
SDOH Code | Model Chosen |
---|---|
Homelessness | NousResearch/Nous-Hermes-2-Yi-34B |
Food Insecurity | Zero-one-ai/Yi-34B-Chat |
Imprisonment or Other Incarceration | NousResearch/Nous-Hermes-2-Yi-34B |
Marital Estrangement | NousResearch/Nous-Hermes-2-Yi-34B |
Relative Needing Care | Meta-llama/Llama-2-13b-chat-hf |
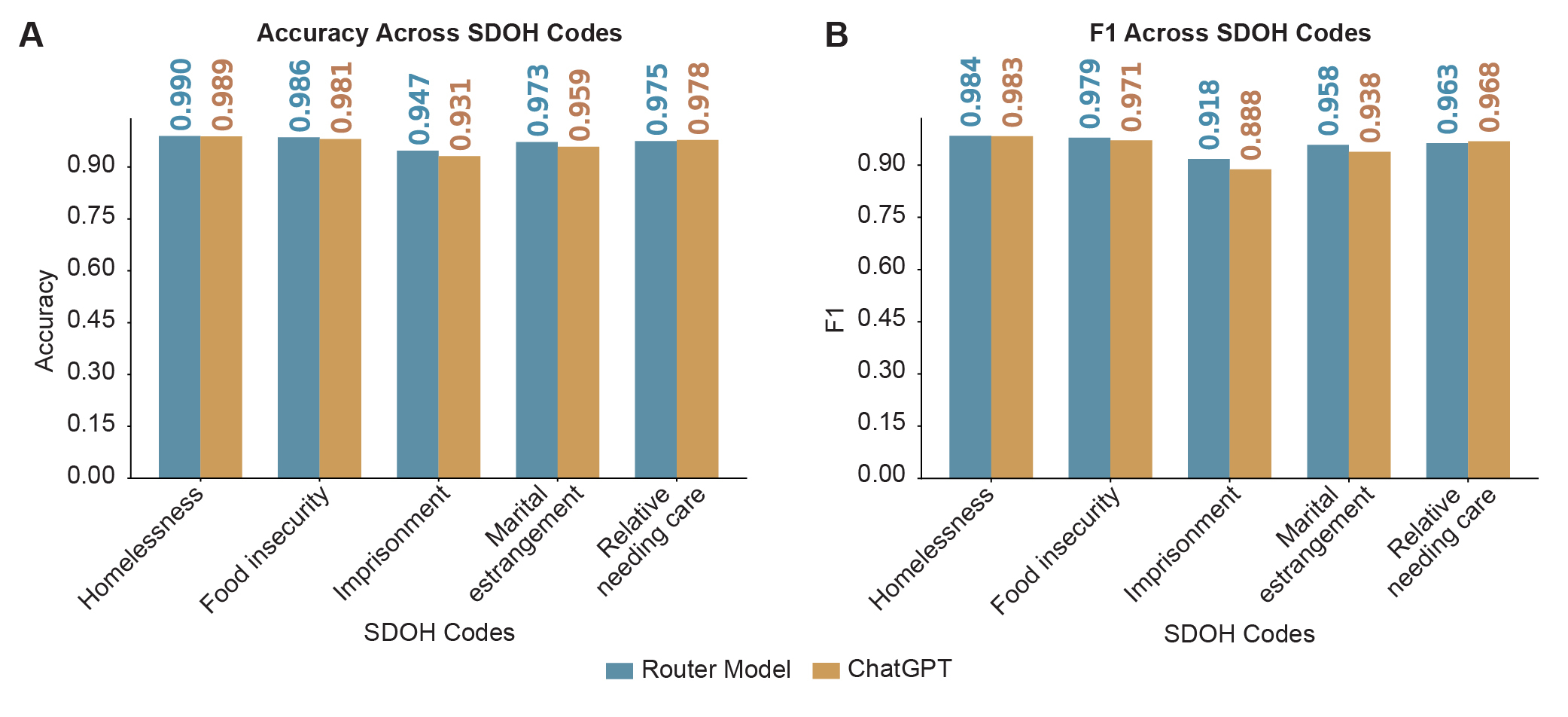
In Figure 2, we see that the router selected a different best open-source performing model based on the SDOH code to achieve the performance seen in Figure 3. The most common model picked was NousResearch/Nous-Hermes-2-Yi-34B, chosen for 3 of the 5 SDOH codes. The LLMs Zero-one-ai/Yi-34B-Chat and Meta-llama/Llama-2-13b-chat-hf were chosen for one code each. All of these models were evaluated without fine-tuning, are completely open-source, and can be found on HuggingFace.
3 Methods
Our data came from patient electronic health records (EHRs) stored in the MIMIC-III dataset [9]. We extracted medical charts with a ‘Social History’ section, on the assumption that these notes would be more likely to contain evidence of an SDOH code. The factors we tested included homelessness, food insecurity, imprisonment or other incarceration, low income, and marital estrangement, relatives needing care, and unemployment. A medical coder annotated 500 medical notes for by parsing through the notes, and indicating information verbatim from the medical notes that corresponded to a specific SDOH code. Noticing that these codes were assigned based on a phrase from the note, we determined that a sentence had enough context for an LLM to appropriately identify the codes bearing evidence in the medical records. We prompted a number of open source models with the Together API using the prompt template in Figure 4.
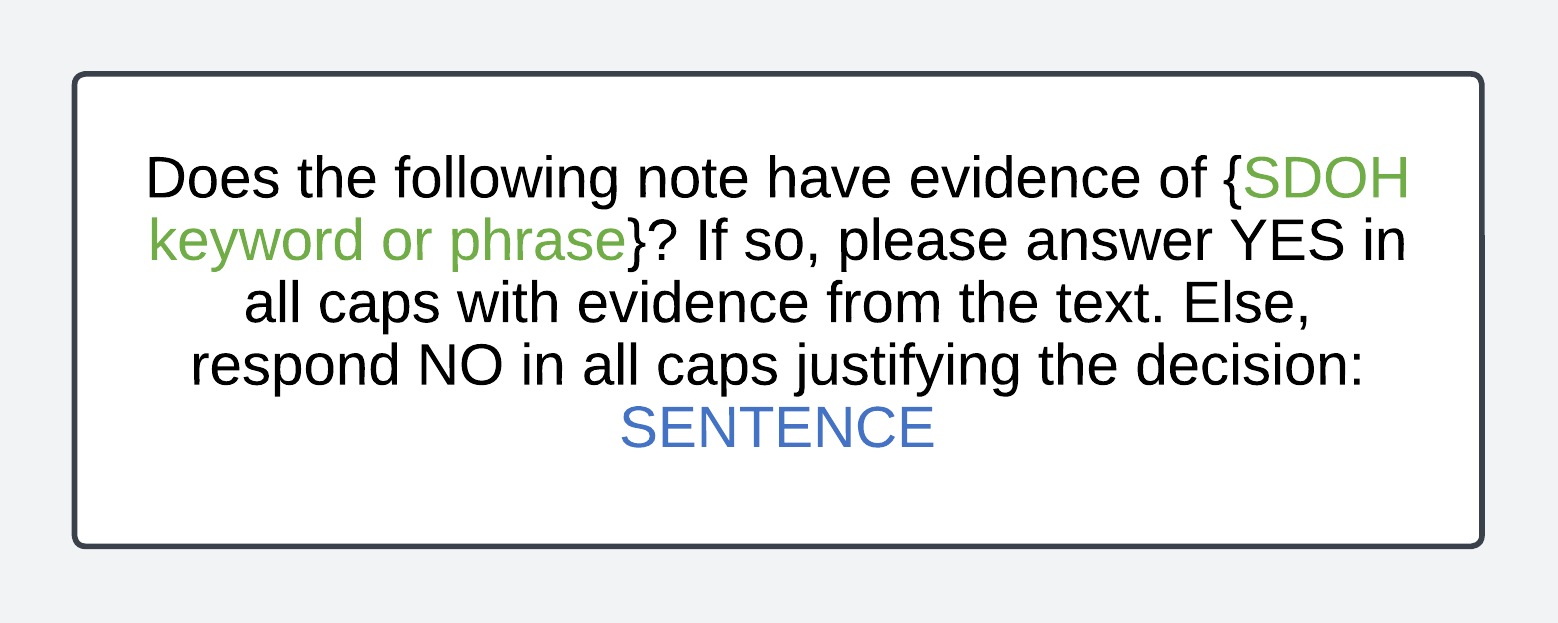
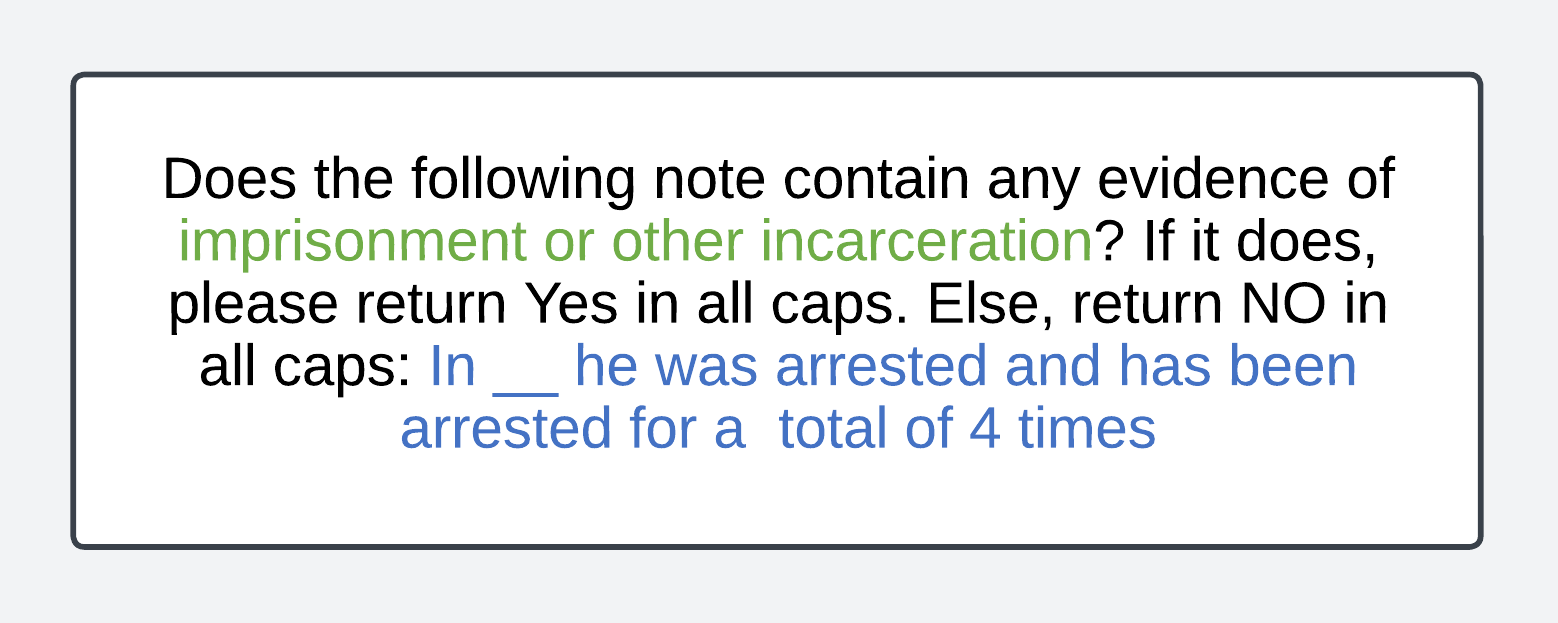
Even after annotating 500 medical notes, sentences with evidence of specific SDOH codes were sparse (<1% of the total number of sentences). In order to address this, we introduced a novel synthetic data generation and validation paradigm, outlined in Figure 5. Our synthetic data generation scheme involved passing in random sentences corresponding to a specific SDOH code from the medical note into Claude-3-opus as references. Using two distinct prompts, one explicitly asking Claude to not use the SDOH keyword or phrase for complexity, we generated a couple hundred synthetic sentences corresponding to SDOH codes. We subsequently also had Claude verify the synthetic sentences it generated by asking Claude to determine if the generated synthetic sentence actually had evidence of a specific SDOH code, dropping the sentences that did not pass this test. This scheme ultimately resulting in a validation set distribution seen in Figure 6.
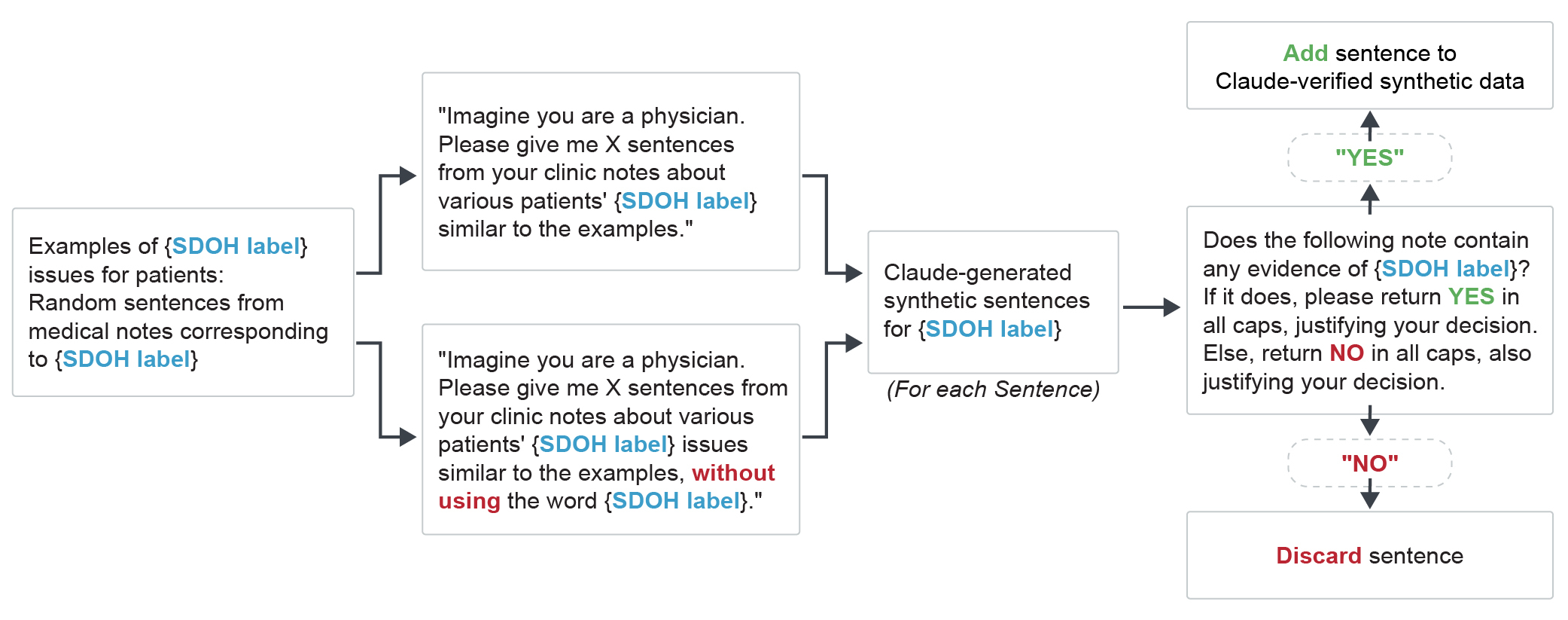
SDOH Code | Gold Data | Synthetic Data | Negative Data |
---|---|---|---|
Homelessness | 28 | 318 | 704 |
Food Insecurity | 2 | 349 | 702 |
Imprisonment or Other Incarceration | 17 | 313 | 660 |
Marital Estrangement | 53 | 312 | 730 |
Relative Needing Care | 3 | 320 | 646 |
The validation set consisted of approximately 33/67% splits of positive/negative labels, with approximately 1000 sentences for each SDOH code. Positive labels consisted of both data containing evidence of an SDOH code from the medical note (gold data) and verified synthetic data (generated and verified by scheme shown in Figure 5). Negative data consisted of sentences from medical notes not containing evidence of an SDOH code. This was the dataset used both to train the router, and was tested against to ultimately determine the performance seen in Figure 3. Interestingly, we observed that Claude had the ability to verify synthetic data it generated itself, resulting in ‘dropped’ data numbers seen in Figure 7.
SDOH Code | Claude Dropped |
---|---|
Homelessness | 12 |
Food Insecurity | 51 |
Imprisonment or Other Incarceration | 7 |
Marital Estrangement | 8 |
Relative Needing Care | 0 |
We used the synthetic data generated by the paradigm introduced in Figure 5 in order to train an “Oracle Router”. This intelligent router (whose schematic is shown in Figure 8) takes in a SDOH keyword or phrase, and outputs the open-source LLM that will perform the best on a given task. The router was trained on both synthetic data (scheme shown in Figure 5) and labelled sentences from the MIMIC-III dataset [9], and executes it’s function by choosing the model that maximizes accuracy for a given SDOH coding task.
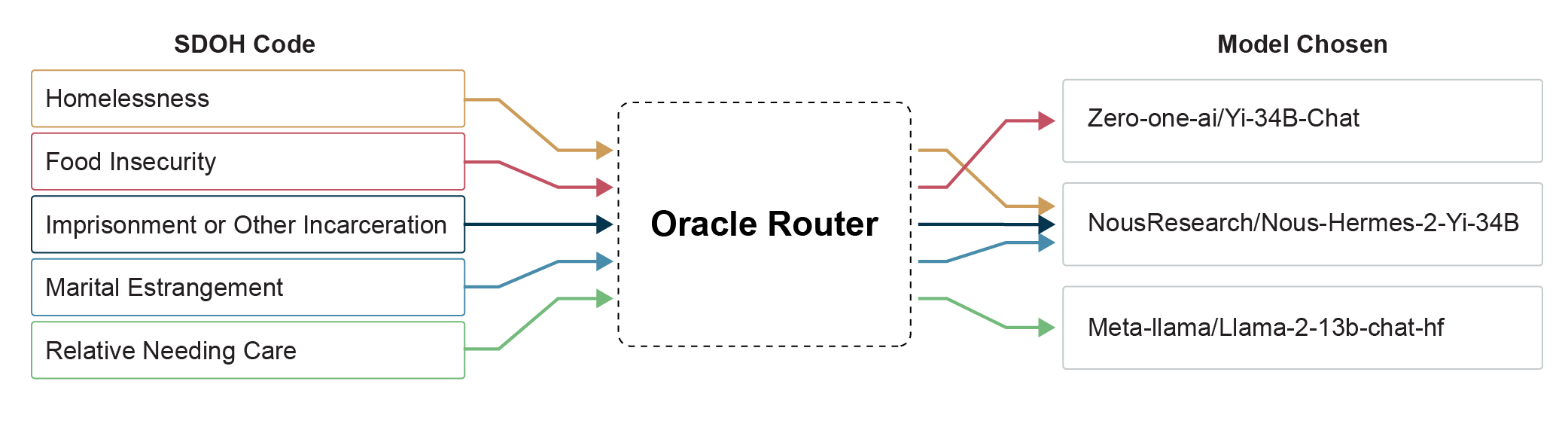
The metrics we used were the following: We evaluated models both based on accuracy and F1 score. In order to calculate these values, we define true positive (TP), true negative (TN), false positive (FP), and false negative (FN) as follows:
-
•
TP: Sentence or note correctly identified as containing evidence of SDOH code
-
•
TN: Sentence or note correctly identified as not containing evidence of SDOH code
-
•
FP: Sentence or note incorrectly identified as containing evidence of SDOH code
-
•
FN: Sentence or note incorrectly identified as not containing evidence of SDOH code
Thus, the formulas for accuracy and F1 score are as follows:
Accuracy | (1) | |||
Precision | (2) | |||
Recall | (3) | |||
F1 | (4) |
4 Conclusion
We demonstrate the the high performance of our intelligent router, which utilizes the power of multiple open-source accuracy to perform slightly better than state-of-the-art models sucuh as GPT-4o. We also introduced a novel synthetic data generation and validation scheme. The high accuracy we achieved was without any prompt-tuning or fine-tuning that could potentially boost performance even more. We also plan to expand this system to account for more SDOH codes. However, in this paper, we demonstrated an the power of an architecture utilizing an intelligent routing of inputs to optimal language models for identifying SDOH codes in unstructured medical notes.
References
- Bommasani et al. [2021] Rishi Bommasani, Drew A. Hudson, Ehsan Adeli, Russ Altman, Simran Arora, Sydney von Arx, Michael S. Bernstein, Jeannette Bohg, Antoine Bosselut, Emma Brunskill, Erik Brynjolfsson, Shyamal Buch, Dallas Card, Rodrigo Castellon, Niladri Chatterji, Annie Chen, Kathleen Creel, Jared Quincy Davis, Dora Demszky, Chris Donahue, Moussa Doumbouya, Esin Durmus, Stefano Ermon, John Etchemendy, Kawin Ethayarajh, Li Fei-Fei, Chelsea Finn, Trevor Gale, Lauren Gillespie, Karan Goel, Noah Goodman, Shelby Grossman, Neel Guha, Tatsunori Hashimoto, Peter Henderson, John Hewitt, Daniel E. Ho, Jenny Hong, Kyle Hsu, Jing Huang, Thomas Icard, Saahil Jain, Dan Jurafsky, Pratyusha Kalluri, Siddharth Karamcheti, Geoff Keeling, Fereshte Khani, Omar Khattab, Pang Wei Koh, Mark Krass, Ranjay Krishna, Rohith Kuditipudi, Ananya Kumar, Faisal Ladhak, Mina Lee, Tony Lee, Jure Leskovec, Isabelle Levent, Xiang Lisa Li, Xuechen Li, Tengyu Ma, Ali Malik, Christopher D. Manning, Suvir Mirchandani, Eric Mitchell, Zanele Munyikwa, Suraj Nair, Avanika Narayan, Deepak Narayanan, Ben Newman, Allen Nie, Juan Carlos Niebles, Hamed Nilforoshan, Julian Nyarko, Giray Ogut, Laurel Orr, Isabel Papadimitriou, Joon Sung Park, Chris Piech, Eva Portelance, Christopher Potts, Aditi Raghunathan, Rob Reich, Hongyu Ren, Frieda Rong, Yusuf Roohani, Camilo Ruiz, Jack Ryan, Christopher Ré, Dorsa Sadigh, Shiori Sagawa, Keshav Santhanam, Andy Shih, Krishnan Srinivasan, Alex Tamkin, Rohan Taori, Armin W. Thomas, Florian Tramèr, Rose E. Wang, William Wang, Bohan Wu, Jiajun Wu, Yuhuai Wu, Sang Michael Xie, Michihiro Yasunaga, Jiaxuan You, Matei Zaharia, Michael Zhang, Tianyi Zhang, Xikun Zhang, Yuhui Zhang, Lucia Zheng, Kaitlyn Zhou, and Percy Liang. On the Opportunities and Risks of Foundation Models, 2021. URL https://arxiv.org/abs/2108.07258. Version Number: 3.
- Clusmann et al. [2023] Jan Clusmann, Fiona R. Kolbinger, Hannah Sophie Muti, Zunamys I. Carrero, Jan-Niklas Eckardt, Narmin Ghaffari Laleh, Chiara Maria Lavinia Löffler, Sophie-Caroline Schwarzkopf, Michaela Unger, Gregory P. Veldhuizen, Sophia J. Wagner, and Jakob Nikolas Kather. The future landscape of large language models in medicine. Commun Med, 3(1):1–8, October 2023. ISSN 2730-664X. doi: 10.1038/s43856-023-00370-1. URL https://www.nature.com/articles/s43856-023-00370-1. Publisher: Nature Publishing Group.
- Cook et al. [2021] Lily A Cook, Jonathan Sachs, and Nicole G Weiskopf. The quality of social determinants data in the electronic health record: a systematic review. J Am Med Inform Assoc, 29(1):187–196, October 2021. ISSN 1067-5027. doi: 10.1093/jamia/ocab199. URL https://www.ncbi.nlm.nih.gov/pmc/articles/PMC8714289/.
- Guevara et al. [2024] Marco Guevara, Shan Chen, Spencer Thomas, Tafadzwa L. Chaunzwa, Idalid Franco, Benjamin H. Kann, Shalini Moningi, Jack M. Qian, Madeleine Goldstein, Susan Harper, Hugo J. W. L. Aerts, Paul J. Catalano, Guergana K. Savova, Raymond H. Mak, and Danielle S. Bitterman. Large language models to identify social determinants of health in electronic health records. npj Digit. Med., 7(1):1–14, January 2024. ISSN 2398-6352. doi: 10.1038/s41746-023-00970-0. URL https://www.nature.com/articles/s41746-023-00970-0. Publisher: Nature Publishing Group.
- Hari and Thomson [2023a] Surya Narayanan Hari and Matt Thomson. Herd: Using multiple, smaller LLMs to match the performances of proprietary, large LLMs via an intelligent composer, 2023a. URL https://arxiv.org/abs/2310.19902. Version Number: 1.
- Hari and Thomson [2023b] Surya Narayanan Hari and Matt Thomson. Tryage: Real-time, intelligent Routing of User Prompts to Large Language Models, 2023b. URL https://arxiv.org/abs/2308.11601. Version Number: 2.
- Jiang et al. [2023] Albert Q. Jiang, Alexandre Sablayrolles, Arthur Mensch, Chris Bamford, Devendra Singh Chaplot, Diego de las Casas, Florian Bressand, Gianna Lengyel, Guillaume Lample, Lucile Saulnier, Lélio Renard Lavaud, Marie-Anne Lachaux, Pierre Stock, Teven Le Scao, Thibaut Lavril, Thomas Wang, Timothée Lacroix, and William El Sayed. Mistral 7B, 2023. URL https://arxiv.org/abs/2310.06825. Version Number: 1.
- Jin et al. [2022] Xisen Jin, Xiang Ren, Daniel Preotiuc-Pietro, and Pengxiang Cheng. Dataless Knowledge Fusion by Merging Weights of Language Models, 2022. URL https://arxiv.org/abs/2212.09849. Version Number: 5.
- Johnson et al. [2015] Alistair Johnson, Tom Pollard, and Roger Mark. MIMIC-III Clinical Database, 2015. URL https://physionet.org/content/mimiciii/1.4/.
- Lybarger et al. [2021] Kevin Lybarger, Mari Ostendorf, and Meliha Yetisgen. Annotating Social Determinants of Health Using Active Learning, and Characterizing Determinants Using Neural Event Extraction. J Biomed Inform, 113:103631, January 2021. ISSN 1532-0464. doi: 10.1016/j.jbi.2020.103631. URL https://www.ncbi.nlm.nih.gov/pmc/articles/PMC7856628/.
- Mehandru et al. [2024] Nikita Mehandru, Brenda Y. Miao, Eduardo Rodriguez Almaraz, Madhumita Sushil, Atul J. Butte, and Ahmed Alaa. Evaluating large language models as agents in the clinic. npj Digit. Med., 7(1):1–3, April 2024. ISSN 2398-6352. doi: 10.1038/s41746-024-01083-y. URL https://www.nature.com/articles/s41746-024-01083-y. Publisher: Nature Publishing Group.
- Omiye et al. [2024] Jesutofunmi A. Omiye, Haiwen Gui, Shawheen J. Rezaei, James Zou, and Roxana Daneshjou. Large Language Models in Medicine: The Potentials and Pitfalls : A Narrative Review. Ann Intern Med, 177(2):210–220, February 2024. ISSN 1539-3704. doi: 10.7326/M23-2772.
- Touvron et al. [2023] Hugo Touvron, Thibaut Lavril, Gautier Izacard, Xavier Martinet, Marie-Anne Lachaux, Timothée Lacroix, Baptiste Rozière, Naman Goyal, Eric Hambro, Faisal Azhar, Aurelien Rodriguez, Armand Joulin, Edouard Grave, and Guillaume Lample. LLaMA: Open and Efficient Foundation Language Models, 2023. URL https://arxiv.org/abs/2302.13971. Version Number: 1.
- Waltman [2024] Belinda Waltman. The Margins Matter. JAMA, 331(1):23, January 2024. ISSN 0098-7484. doi: 10.1001/jama.2023.25361. URL https://jamanetwork.com/journals/jama/fullarticle/2812973.
- Wang et al. [2024] Guan Wang, Sijie Cheng, Xianyuan Zhan, Xiangang Li, Sen Song, and Yang Liu. OpenChat: Advancing Open-source Language Models with Mixed-Quality Data, March 2024. URL http://arxiv.org/abs/2309.11235. arXiv:2309.11235 [cs].