Learning of Visual Relations: The Devil is in the Tails
Abstract
Significant effort has been recently devoted to modeling visual relations. This has mostly addressed the design of architectures, typically by adding parameters and increasing model complexity. However, visual relation learning is a long-tailed problem, due to the combinatorial nature of joint reasoning about groups of objects. Increasing model complexity is, in general, ill-suited for long-tailed problems due to their tendency to overfit. In this paper, we explore an alternative hypothesis, denoted the Devil is in the Tails. Under this hypothesis, better performance is achieved by keeping the model simple but improving its ability to cope with long-tailed distributions. To test this hypothesis, we devise a new approach for training visual relationships models, which is inspired by state-of-the-art long-tailed recognition literature. This is based on an iterative decoupled training scheme, denoted Decoupled Training for Devil in the Tails (DT2). DT2 employs a novel sampling approach, Alternating Class-Balanced Sampling (ACBS), to capture the interplay between the long-tailed entity and predicate distributions of visual relations. Results show that, with an extremely simple architecture, DT2-ACBS significantly outperforms much more complex state-of-the-art methods on scene graph generation tasks. This suggests that the development of sophisticated models must be considered in tandem with the long-tailed nature of the problem.
1 Introduction
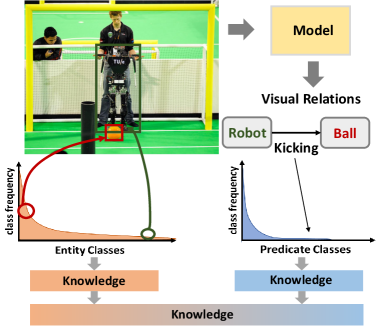
Scene graphs provide a compact structured description of complex scenes and the semantic relationships between objects/entities. Modeling and learning such visual relations benefit several high-level Vision-and-Language tasks such as caption generation [45, 44], visual question answering [16], image retrieval [20, 34], image generation [19, 24, 33] and robotic manipulation planning [29]. Scene graph generation requires the understanding of the locations and the class associated with the entity as well as the relationship between a pair of entities. The relationship between a pair of entities is usually formulated as a tuple, where subject and object are two entities. Scene graph generation (SGG) faces the challenges from both the long-tailed entity recognition problem and visual relation recognition problem.
While long-tailed entity recognition has been addressed in the literature [28, 1, 5, 21], the imbalance becomes more prevalent for the SGG tasks, owing to the severe long-tailed nature of the predicate distribution. Take Figure 1 for example. While the class of the subject (“ball”) is popular, the class of the object (“robot”) and the predicate (“kicking”) can be infrequent, leading to the rare occurrence of the tuple “robot-kicking-ball”. This shows that even when the entity class distribution is balanced, the imbalanced predicate class distribution can lead to a more imbalanced tuple distribution. Of course, such imbalance issues can be exacerbated if both entity classes and predicate classes are skewed (e.g. “tripod-mounted-on-donkey”). The combination of long-tailed entity and predicate classes makes SGG a more challenging problem.
While the long-tailed problem poses a great challenge to SGG tasks, it has not been well addressed in the SGG literature. Existing works [48, 43, 3, 32, 49] instead focused on designing more complex models, primarily by adding architectural enhancements that increase model size. While this has enabled encouraging performance under the Recall@k (R@k) metric, this metric is biased toward the highly populated classes. This suggests that prior works may be overfitting on popular predicate classes (e.g. on/has), but their performances could degrade on the less frequent classes (e.g. eating/riding). Such a bias towards the populated classes is problematic, because predicates lying in the tails often provide more informative depictions of scene content. The failure to predict tail classes could lead to a less informative scene graph , limiting the effectiveness of scene graphs for intended applications. In this paper, we explore the hypothesis that the Devil is in the tails. Under this hypothesis, visual relation learning is better addressed by a simple model of improved ability to cope with long-tailed distributions.
To investigate this hypothesis, we first analyze the distribution of entity and predicate classes in the Visual Genome dataset. As shown in Figure 2, both distributions are heavily skewed, but with different magnitude. The imbalance in the predicate distribution is more severe than that in the entity distribution. To the best of our knowledge, none of the existing SGG methods considered the jointly long-tailed distributions of entity and predicate classes. To address this, we propose a new approach to visual relationship learning, based on a simpler architecture than those in the literature but a more sophisticated training procedure, denoted Decoupled Training for Devil in the Tails (DT2).
DT2 is a generalization of the decoupled training procedures that have recently become popular for long-tailed recognition [21]. It consists of an alternative sampling scheme that produces distributions balanced for entities and predicates. This is accompanied by a novel sampling scheme, Alternating Class-Balanced Sampling (ACBS), which captures the interplay between the two different long-tailed distributions through an implementation of learning without forgetting [26] based on a mechanism that introduces memory between the sampling iterations, using knowledge distillation. With DT2, we show that a simple architecture with 10 fewer parameters significantly outperforms prior, and more sophisticated, architectures designed for SGG, under the mRecall@K metric, which is suited for measuring the performance of a long-tailed dataset. Ablation studies of different sampling schemes as well as analysis of performance on classes of different popularity further validate our hypothesis.
Overall, the paper makes three contributions. 1) We devise a simple model architecture with the decoupled training scheme, namely DT2, suited for the long-tailed SGG tasks. 2) We propose a novel sampling strategy, Alternating Class-Balanced Sampling (ACBS), to capture the interplay between different long-tailed distributions of entities and relations. 3) The combined DT2-ACBS significantly outperforms state-of-the-art methods of more complex architectures on all SGG tasks on the Visual Genome benchmark. The code is available on the project website111http://www.svcl.ucsd.edu/projects/DT2-ACBS.
2 Related work
2.1 Scene graph generation
Several works have addressed the generation of scene graphs for images [46, 42, 47, 14, 38, 41, 48, 43, 25, 9, 3, 32, 17, 49, 7]. Most approaches focus on either sophisticated architecture design or contextual feature fusion strategies, such as message passing and recurrent neural networks [48, 32], to optimize SGG performance on the Visual Genome dataset [22] under the Recall@K metric. While these approaches achieved gains for highly populated classes, underrepresented classes tend to have much poorer performance. Recently, [3, 31, 42, 37, 23] started to address the learning bias induced by the dataset statistics, by using a more suitable evaluation metric, mRecall@K, which averages recall values across classes. To address the dataset bias, TDE [31] employed causal inference in the prediction stage , whereas [37] used a pseudo-siamese network to extract balanced visual features, and PCPL [42] harnessed implicit correlations among predicate classes and used a complex graph encoding module consisting of a number of stacked encoders and attention heads. A concurrent work [23] introduces confidence-based gating with bi-level data resampling to mitigate the training bias. These methods considered, at most, the long-tailed distribution of either predicates or entities and do not disentangle the gains of sampling from those of complex architectures. For example, [42] proposed a contextual feature generator via graph encoding with 6 stacked encoders, each with 12 attention heads and a feed-forward network. We argue that long-tailed distributions should be considered for both entities and predicates and show that, when this is done, better results can be achieved with a much simpler architecture.
2.2 Long-tailed recognition
Prior work addresses the long-tailed issue in 3 directions: data re-sampling, cost-sensitive loss and transfer learning.
Data resampling [12, 10, 51, 11, 8, 2] is a popular strategy to oversample tail (underrepresented) classes and undersample head (populated) classes. Oversampling is achieved either by duplicating samples or by synthesizing data [10, 51, 2]. While producing a more uniform training distribution, recent works [21, 50] argue that this strategy is unsuitable for deep representation learning like CNN. [21] decouples the representation learning from the classifier learning, adopting different sampling strategies in the two stages, whereas [50] proposes a two-stream model with a mixed sampling strategy. The proposed method lies in this direction, since we consider different distributions of entity and predicate classes, and adopt different sampling strategies for training different model components.
Cost-sensitive losses [6, 5, 1, 27] assign different costs to the incorrect prediction of different samples, according to class frequency [5, 1] or difficulty [6, 27]. This is implemented by assigning higher weights or enforcing larger margins for classes with fewer samples. Weights can be proportional to inverse class frequency or effective number [5] and can be estimated by meta-learning [18]. This re-weighting strategy was recently applied to the scene graph literature [42] to overcome long-tailed distributions.
Transfer learning methods transfer information from head to tail classes. [35, 36] learns to predict few-shot model parameters from many-shot model parameters, and [28] proposes a meta-memory for knowledge sharing. [39] leverages a hierarchical classifier to share knowledge among classes. [40] learn an expert model for each class popularity, and combine them by knowledge distillation.
3 Formulation and data statistics
In this section, we review the problem of learning visual relations and discuss its long-tailed nature.
3.1 Definitions
The inference of the visual relationships in a scene is usually formulated as a three stage process. The objects/entities in the scene are detected, classified, and the relationships between each pair of entities, in the form of predicates, are finally inferred. [20] formulated these stages with a Scene Graph. Let and be the set of entity and predicate classes, respectively. Each entity is composed by a bounding box and a class label . A relation is a three-tuple, connecting a subject and an object identities (), through a predicate . For example, person-riding-bike. The scene graph of an image contains a set of entities and a set of relations extracted from the image. This can be further decomposed into a set of bounding boxes , a set of class labels , and a set of relations .
The generation of a scene graph from an image is naturally mapped into the probabilistic model
(1) |
where is a bounding box prediction model, an entity class model and is a predicate class model. Joint inference of the three tasks is referred to as Scene Graph Detection (SGDet). However, because bounding box prediction has been widely studied in object detection [30], it is possible to simply adopt an off-the-shelf detector. This motivates two other tasks: Predicate classification (PredCls), where both bounding boxes and entity classes are given, and Scene Graph Classification (SGCls), where only bounding boxes are known.
3.2 Long-tailed visual relations
Long-tailed distributions are a staple of the natural world, where different classes occur with very different frequencies. For example, while some entity classes (e.g. chair) occur very frequently, others (e.g donkey) are much less frequent. Long tails are problematic because, under standard loss functions and evaluation metrics, they encourage machine learning systems to overfit on a few head classes and ignore a large number of tail classes. Recent works [28, 5, 50, 21] have shown that sampling techniques which de-emphasize popular classes, giving more weight to rare ones, can induce very large recognition gains when distributions are long-tailed. However, the issue has not been thoroughly considered in the visual relations literature.
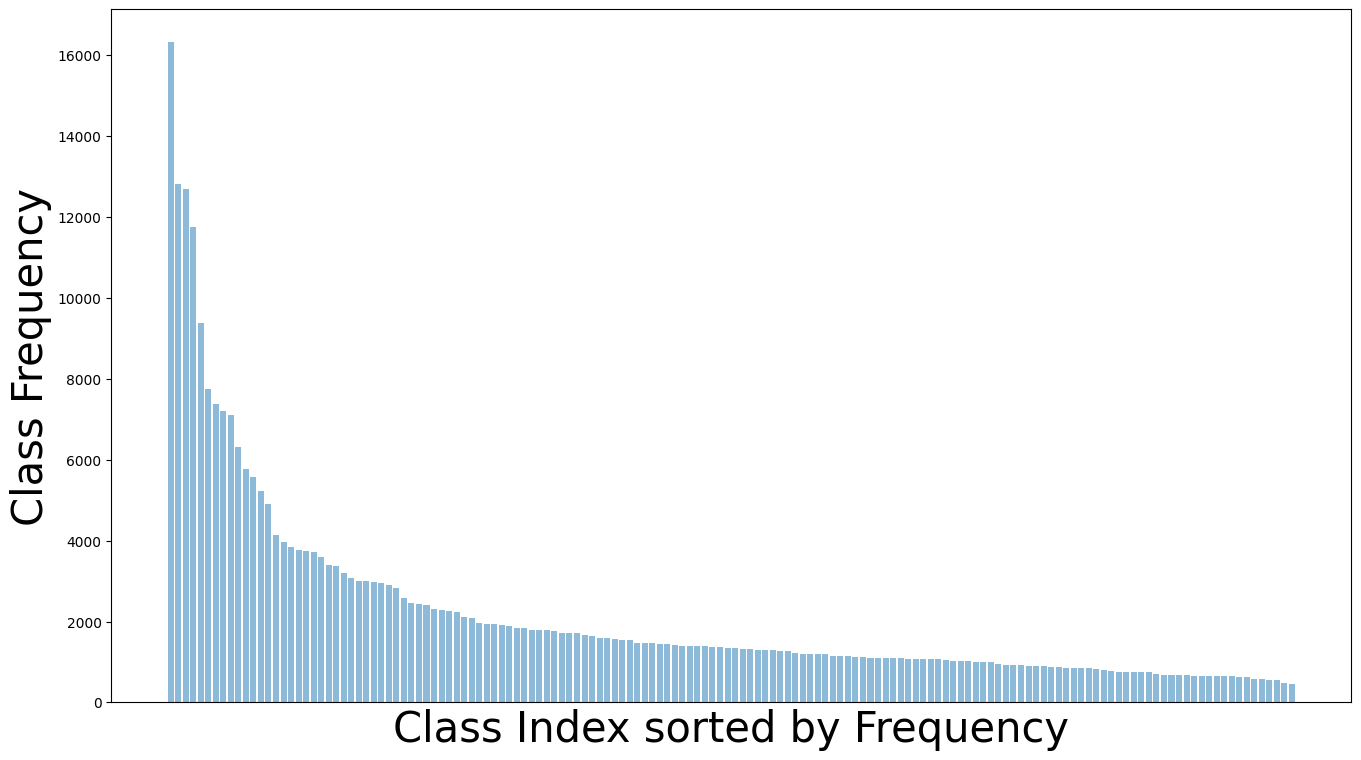
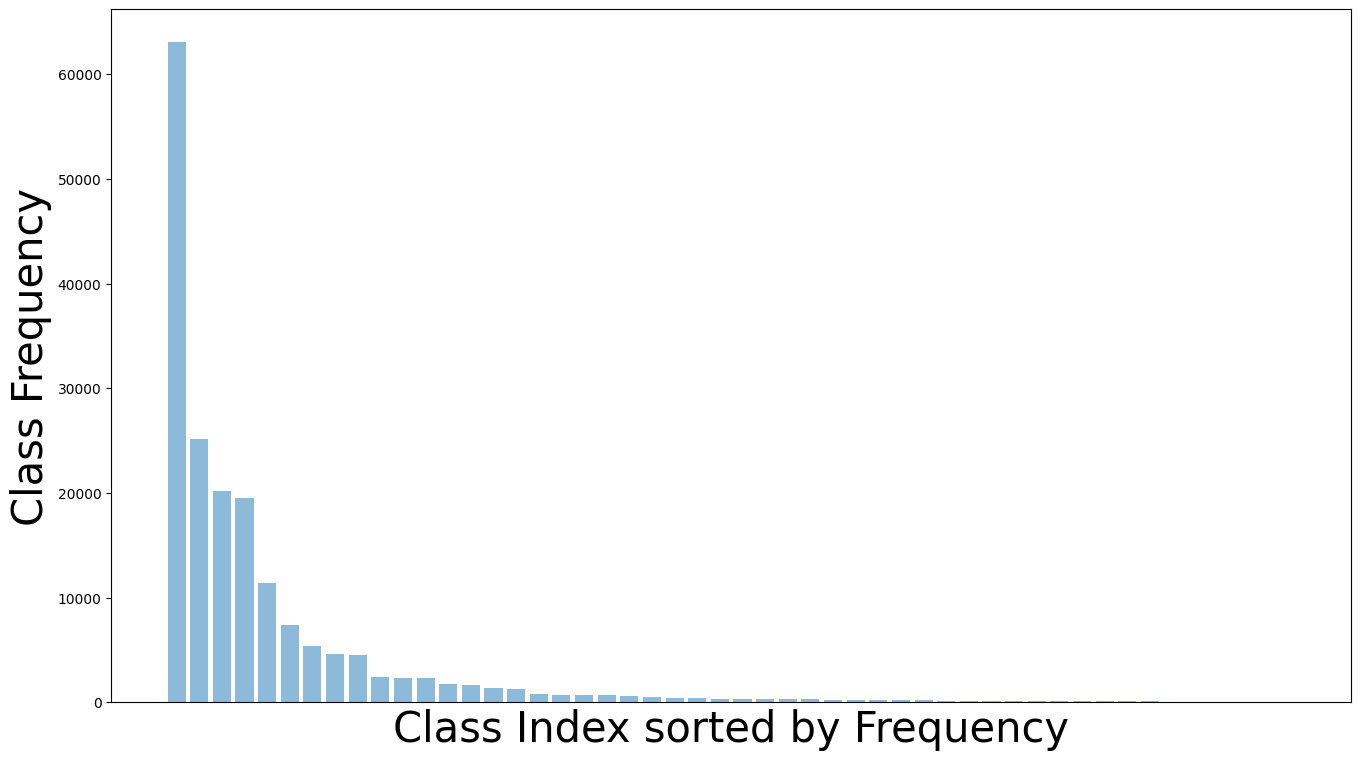
This is somewhat surprising, given the combinatorial dependence of visual relationships on entities and predicates. Since entities are long-tailed, relationships between pairs of entities have even more skewed distributions. For example, because the entity classes “donkey” and “cliff” are less frequent than “chair” and “leg”, the relation “donkey-on-cliff” is much less frequent than “chair-has-leg”. This, however, is not the only source of skew, since predicates can be rare even when associated entity classes are popular, e.g. playing is much less popular than has. Finally, relationships can be rare even when involving frequent entities and predicates, e.g. the relation “car-has-wheel” is much more likely than “car-has-camera”. For all these reasons, very long tails are unavoidable for visual relations. This is quite visible in the widely used Visual Genome [22] dataset. As shown in Figure 2, both the distribution of entity and predicate classes are long-tailed. For entities, the most populated class is larger than the least populated. For predicates, the former is larger than the latter ( if the least frequent predicate class is discarded). Note that this is much larger than the square of the ratio between entity classes () suggested by the factorial nature of relationships.
The long-tailed problem is exacerbated by the evaluation protocol, based on the Recall@K (R@K) measure, adopted in most of the scene graphs literature. This measures the average percentage of ground truth relation triplets that appear in the top predictions and, like any average, is dominated by the most frequent relationship classes. Hence, it does not penalize solutions that simply ignore infrequent relationship classes. Since most works, e.g. [32, 7, 3], focus on designing ever more complex network architectures to optimize R@K performance, it is unclear whether all that is being accomplished is stronger overfitting to a few dominant classes (e.g. “on”). This is undesirable for two reasons. First, the number of infrequent relations is much larger than that of dominant relationships. Second, while dominant relations include many obvious contextual relationships (e.g.“car-has-wheels”), infrequent ones are potentially more informative (e.g. “monkey-playing-ball”) of the scene content. In summary, the focus on optimizing R@K could lead to systems that are only capable of detecting a few relationships of relatively low information content.
This problem has been recognized in the recent literature, where some works [3, 31] have started to adopt the mRecall@K (mR@K) metric, which first averages the recall of triplets within the same predicate class and then averages the class recalls over all the predicate classes. While this is a step in the right direction, it is not sufficient to account for class imbalance only at the evaluation stage. Instead, the learning algorithm should explicitly address this imbalance. This leads to an alternative hypothesis that we explore in this work: Is the devil in the tails? Or, in other words, can a simple model designed explicitly to cope with the long-tailed nature of visual relations outperform existing models, which are much more complex but ignore this property? To investigate this hypothesis, we introduce a solution that uses a model much simpler than recently proposed architectures, but is much more sophisticated in its use of sampling techniques that target the long-tailed nature of visual relationship.

4 Method
In this section, we introduce the proposed network architecture, losses, and the training procedure.
4.1 Notations
For a relation tuple in image , is the ground truth predicate class, while and are the subject and object entities, composed of its associated bounding box coordinates (e.g. ) and ground truth entity class (e.g. ). The bounding boxes of an entity can be either the ground truth coordinates or the predictions from a detection model, depending on the task of interest (i.e. SGCls or SGDet). With the bounding boxes, the corresponding image patch and for the subject and object can be cropped from the image .
In addition, we define as a probability vector at the output of the softmax function with temperature , and its entry is formulated as
(2) |
where is a feature vector, is the matrix of weight parameters .
4.2 Model architecture
Figure 3 summarizes the architecture of the Decoupled Training for Devil in the Tails (DT2) model. This combines an entity encoder , as shown in the right part of Figure 3, and a predicate classifier . DT2 takes the bounding box coordinates , [4] and the corresponding cropped image patches and as input. The entity encoder is then applied to both and , to extract a pair of subject-object feature vectors that represent both the appearance and semantics of entities and . These are then concatenated with an embedding of the bounding box coordinates and , and fed to a predicate classifier . Implementation details of the entity encoder and the predicate classifier are elaborated below.
Entity encoder first maps image patch of entity through a feature extractor, implemented with the first three convolutional blocks of a pretrained ResNet101 [13]. We use a faster R-CNN pre-trained for object detection on Visual Genome under regular sampling (all images are sampled uniformly). The resulting feature vector is then mapped to two feature vectors, and , that encode semantics and appearance information respectively, through two different branches sharing identical architecture. The semantic branch of parameter is implemented with a stack of convolution layers (the last convolutional block of ResNet101). Its output is then fed to a softmax layer that predicts the probability of the class of the entity , i.e.
(3) |
where is the matrix of the entity classifier weights and of in (2) is set to 1. The one-hot encoding can be generated by taking the argmax of , which is then mapped into a semantic feature vector with a single fully connected layer.
While the semantic branch would be, in principle, sufficient to convey the entity identity to the remainder of the network, this does not suffice to infer visual relationships. For example, the detection of the “people” and “bike” entities in Figure 3 is not enough to infer whether the relationship is “person-standing by-bike” or “person-riding-bike”. This problem is addressed by introducing the appearance branch of parameter , which outputs a feature vector with no pre-defined semantics, simply encoding entity appearance. Finally, the feature vectors and are concatenated into a vector that represents both the appearance and semantics of entity .
Predicate classifier takes the subject and object feature vectors as input. These vectors are then concatenated with an embedding of subject and object bounding boxes produced by a fully-connected layer, to create a joint encoding of the semantics, appearance, and location of the subject-object patches and . The predicate classifier is implemented with a small feature extractor , consisting of three layers that perform dimension reduction. The input is first transformed into a -dimension vector with a fully connected layer, followed by a batch normalization and a ReLU layer, the output of which is finally passed through a fully connected layer with a tanh non-linearity, to produce a final feature vector . This is fed to a softmax layer to produce the probability of the predicate class
(4) |
where is the weight matrix of the predicate classifier.
4.3 Training
DT2 is trained with standard cross-entropy losses targeted on entity and predicate classification. The former is defined as
(5) |
where denotes the cross-entropy loss, is the output probability prediction of (3) and is the ground truth one-hot code of the entity in the set from image . This is complemented by a predicate classification loss
(6) |
where is the output probability of (4) and the ground truth one-hot code for the predicate in the set of visual relations in image . Both (5) and (6) are important to guarantee that the network can learn from both entities and predicate relationships.
4.4 Sampling strategies
While encapsulating both semantics and appearance information, the proposed training loss in Sec. 4.3 requires a complementary sampling strategy tailored for long-tailed data. This long-tailed problem has been studied mostly in the object recognition literature, where an image patch is fed to a feature extractor with the parameter and the softmax layer of (2) with weight matrix . A popular training strategy is to use different sampling strategies to train the two network components [21]. The intuition is that, because the bulk of the network parameters are in the feature extractor (), this should be learned with the largest possible amount of data. Hence, the entire network is first trained with Standard Random Sampling (SRS), which samples images uniformly, independent of their class labels.
While this produces a good feature extractor, the resulting classifier usually overfits to the head classes, which are represented by many more images and have a larger weight on the cost function. The problem is addressed by fine-tuning the network on a balanced distribution, obtained with Class Balanced Sampling (CBS). This consists of sampling uniformly over classes, rather than images, and guarantees that all classes are represented with equal frequencies. However, because images from tail classes are resampled more frequently than those of head classes, it carries some risk of overfitting to the former. To avoid overfitting, the fine-tuning is restricted to the weights of the softmax layer. In summary, the network is trained in two stages. First, the parameters and are jointly learned with SRS. Second, the feature extractor () is fixed and the softmax layer parameters are relearned with CBS.
4.5 Sampling for visual relationships
Similar to long-tailed object recognition, it is sensible to train a model for visual relations in two stages. In the first stage, the goal is to learn the parameters of the feature extractors (see Sec. 4.2), which are the overwhelming majority of the network parameters. As in object recognition, the network should be trained with SRS. In the second stage, the goal is to fine-tune the softmax parameters and to avoid overfitting to head classes. However, unlike long-tailed object recognition, Figure 2 shows that predicates and entities can have very different distributions, which makes the learning of long-tailed visual relations a distinct problem. This indicates that two class-balanced sampling strategies are required to accommodate the distribution difference between predicate and entity classes.
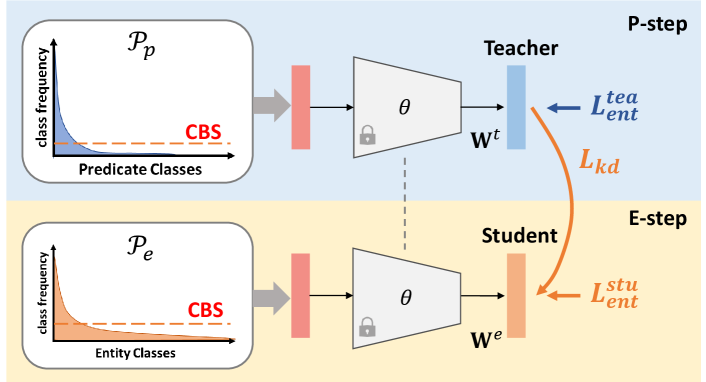
A straightforward solution is to introduce a 2-step iterative training procedure, namely entity-optimization step (E-step) and predicate-optimization step (P-step), to optimize the weight of and respectively. In E-step, images are sampled from a distribution that is uniform with respect to entity classes, which is denoted as Entity-CBS. While in P-step, they are sampled from a distribution uniform with respect to predicate classes, denoted as Predicate-CBS. However, since the uniform sampling of is not class-balanced for entity classes, P-step would lead to the overfitting of the entity classification parameters .
To address this problem, we propose a novel sampling strategy, Alternating CBS (ACBS), tailored for long-tailed visual relations. ACBS contains a memory mechanism to maintain the entity predictions of the P-step, making sure that what was learned is not forgotten in the E-step. It is implemented with distillation [15] between the P-step and E-step and an auxiliary teacher entity classifier of weight matrix . The teacher entity classifier is inserted in parallel with the entity classifier of weight matrix in (3), which is its student, and produces a second set of entity prediction probabilities as
(7) |
With the introduction of the teacher entity classifier, we rewrite (5) into and , where the former operates on of (3) and the latter operates on . Furthermore, to distill knowledge from the teacher entity classifier, a Kullback-Leibler divergence (KL) loss () is defined as
(8) |
where the two inputs to are the smooth version of (3) and (7) with temperature .
In summary, the P-step updates parameters of the predicate classifier and of the teacher with (6) and respectively, while the student parameters are kept fixed. In the E-step, and (teacher) are kept fixed, and (student) is optimized with and (8). This implements learning without forgetting [26] between the two steps, encouraging the student classifier to mimic the predictions of the teacher classifier, and enabling the network to learn the new parameters for one distribution, e.g. , without forgetting the one, e.g. , previously learned for the other. The training procedure is detailed in Algorithm 1.
5 Experiments
In this section, several experiments are performed to validate the effectiveness of DT2-ACBS.
Predicate Classification | Scene Graph Classification | Scene Graph Detection | |||||||
Method | mR@20 | mR@50 | mR@100 | mR@20 | mR@50 | mR@100 | mR@20 | mR@50 | mR@100 |
IMP+ [41] | - | 9.8 | 10.5 | - | 5.8 | 6.0 | - | 3.8 | 4.4 |
FREQ [48] | 8.3 | 13.0 | 16.0 | 5.1 | 7.2 | 8.5 | 4.5 | 6.1 | 7.1 |
MOTIFS [48] | 10.8 | 14.0 | 15.3 | 6.3 | 7.7 | 8.2 | 4.2 | 5.7 | 6.6 |
MOTIFS [48] | 13.2 | 16.3 | 17.5 | 7.1 | 8.8 | 9.3 | 4.9 | 6.7 | 8.2 |
KERN [3] | - | 17.7 | 19.2 | - | 9.4 | 10.0 | - | 6.4 | 7.3 |
VCTree [32] | 14.0 | 17.9 | 19.4 | 8.2 | 10.1 | 10.8 | 5.2 | 6.9 | 8.0 |
GBNet [46] | - | 22.1 | 24.0 | - | 12.7 | 13.4 | - | 7.1 | 8.5 |
TDE-MOTIFS-SUM [31] | 18.5 | 25.5 | 29.1 | 9.8 | 13.1 | 14.9 | 5.8 | 8.2 | 9.8 |
TDE-MOTIFS-SUM [31] | 17.9 | 24.8 | 28.6 | 9.6 | 13.0 | 14.7 | 5.6 | 7.7 | 9.1 |
TDE-VCTree-SUM [31] | 18.4 | 25.4 | 28.7 | 8.9 | 12.2 | 14.0 | 6.9 | 9.3 | 11.1 |
TDE-VCTree-GATE [31] | 17.2 | 23.3 | 26.6 | 8.9 | 11.8 | 13.4 | 6.3 | 8.6 | 10.3 |
PCPL [42] | - | 35.2 | 37.8 | - | 18.6 | 19.6 | - | 9.5 | 11.7 |
DT2-ACBS (ours) | 27.4 | 35.9 | 39.7 | 18.7 | 24.8 | 27.5 | 16.7 | 22.0 | 24.4 |
Predicate Classification | Scene Graph Classification | Scene Graph Detection | |||||||
Method | Head (16) | Middle (17) | Tail (17) | Head (16) | Middle (17) | Tail (17) | Head (16) | Middle (17) | Tail (17) |
MOTIFS [48] | 42.3 | 9.8 | 0.6 | 24.6 | 4.0 | 0.1 | 20.2 | 4.6 | 0.4 |
TDE-MOTIFS-SUM [31] | 44.9 | 35.8 | 6.1 | 25.6 | 15.8 | 3.3 | 22.2 | 5.6 | 0.1 |
DT2-ACBS (ours) | 35.1 | 45.2 | 38.6 | 24.6 | 29.1 | 28.6 | 22.3 | 26.7 | 24.0 |
5.1 Dataset
Visual Genome (VG) [22] is composed of 108k images across 75k object categories and 37k predicate categories, but of the predicates have less than 10 instances. Following prior works, we use the original splits of the popular subset (i.e. VG150) for training and evaluation. It contains the most frequent 150 object classes and 50 predicate classes. The distribution remains highly long-tailed. To perform balanced sampling during training, predicate classes with less than 5 instances, e.g. “flying in,” are ignored.
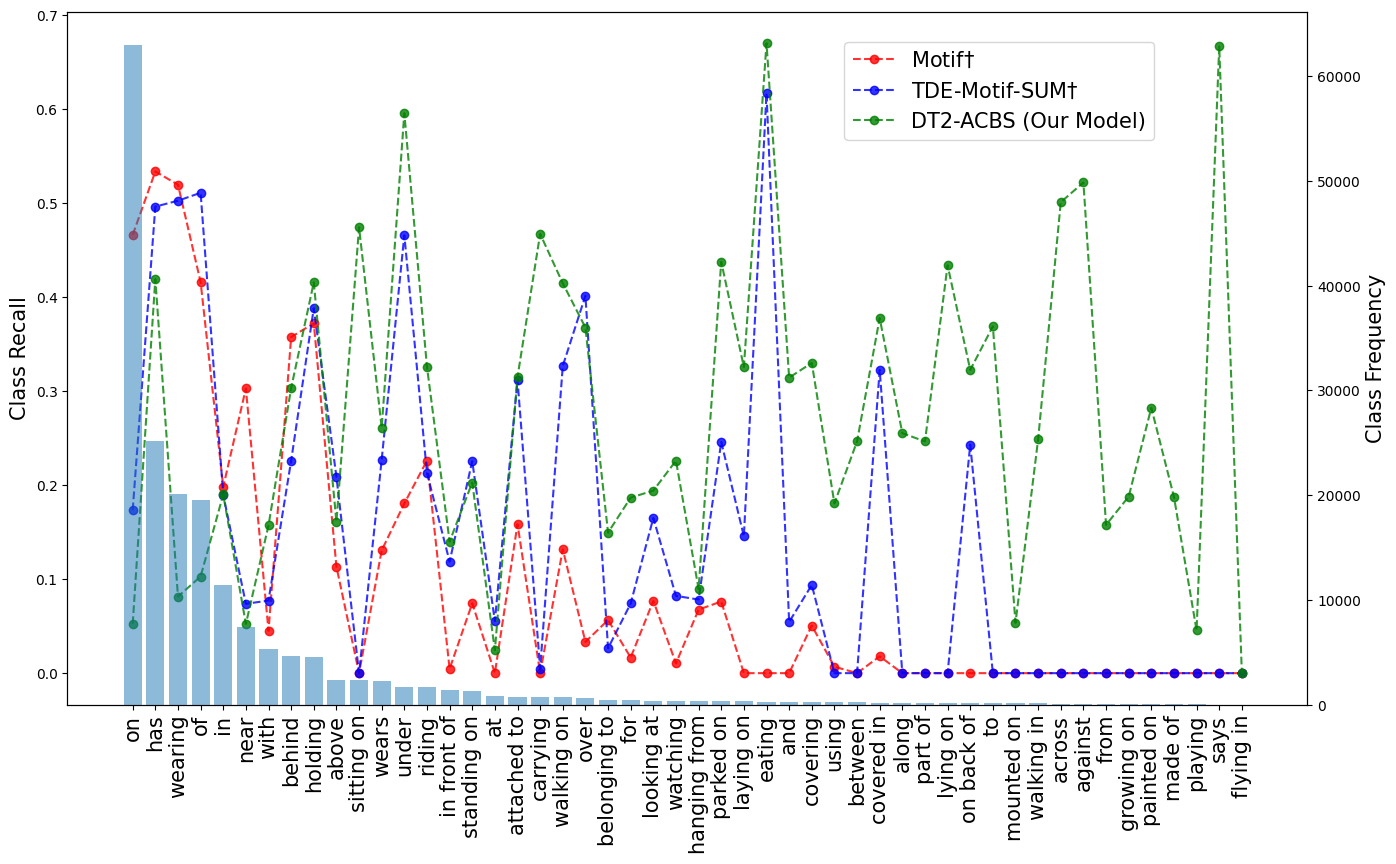
5.2 Comparison to SOTA
To validate our hypothesis, we compare DT2-ACBS with the state-of-the-art methods on PredCls, SGCls and SGDet task on the popular subset VG150 of VG [22], under the mRecall@K metric. As shown in Table 1, compared baselines include 1) simple frequency-based method [48], 2) sophisticated architecture design for contextual representation learning [41, 3, 32, 46] and 3) recent works that tackle the long-tailed bias of predicate classes [31, 42]. Several observations can be made. First, DT2-ACBS outperforms all baselines in the first two groups by a large margin (mR@100 gain larger than ) on the PredCls task, where entity bounding boxes and categories are given. The baselines in the third group [31, 42], which address the long-tailed bias of the predicate distribution, are similar in spirit to DT2-ACBS. However, the latter relies on a simpler model design and a more sophisticated decoupled training scheme to overcome overfitting. This enables a improvement on mR@100 ( relative improvement), showing the efficacy of the proposed sampling mechanism for tackling the long-tailed problem in predicates distribution.
Next, when predicting both predicate and entity class given the ground truth bounding boxes (SGCls task), DT2-ACBS outperforms all existing methods by a larger mR@100 margin ( on PredCls vs on SGCls, equivalently relative improvement of 5% in PredCls vs 40% in SGCls). This significant improvement in SGCls performance can be ascribed to the decoupled training of ACBS, which better captures the interplay between the different distributions of entities and predicates.
Finally, we also ran DT2-ACBS on proposal boxes generated by a pre-trained Faster-RCNN for the SGDet task.Table 1 shows that DT2-ACBS outperforms existing methods by a significantly larger mR@100 margin of ( relative improvement) on the SGDet task.
![]() |
![]() |
Class-wise performance analysis: To study the performance of classes with different popularity, we sort the 50 relation classes by their frequencies and divide them into 3 equal parts, head (16), middle (17) and tail (17). Table 2 presents the mR@100 performance on these partitions for each SGG task. As observed in prior long-tailed recognition work [28, 21], a performance drop in head classes is hard to avoid while improving tail class performance. The goal, instead, is to achieve the best balance among all the classes, which DT2-ACBS clearly does with notable improvements in the middle and tail classes. It should also be noted that the drop in head performance can be deceiving, due to dataset construction problems like “wearing” and “wears” appearing as two different relationship classes. Most importantly, many VG150 tail categories (e.g. “standing on”, “sitting on”) are fine-grained versions of a head category (“on”). Some of the degradation in head class performance is just due to the predicates being pushed to the fine-grained classes, which is more informative. We notice that one of the high-frequency predicate classes On has a low recall value (Figure 5) and observe that DT2-ACBS often instead predicts its fine-grained sub-categories, such as standing on, sitting on, mounted on. In particular, there are ground truth instances of On predicate in the test set, and DT2-ACBS predicts On-subcategories times on PredCls, which constitutes incorrect predictions as per the metric. Overall, DT2-ACBS performs significantly better in middle and tail classes on SGG tasks, and performs comparably on head classes for SGCls and SGDet, reaching the best balance across all the classes.
Method | mR@20 | mR@50 | mR@100 |
---|---|---|---|
Single Stage-SRS | 6.4 | 9.6 | 11.2 |
Single Stage-Indep. CBS | 8.5 | 11.2 | 12.4 |
DT2-Predicate-CBS | 10.0 | 13.0 | 14.3 |
DT2-Indep. CBS | 17.3 | 23.9 | 26.7 |
DT2-ACBS (ours) | 18.7 | 24.8 | 27.5 |
5.3 Ablations on sampling strategies
SGCls performance is affected by the intertwined entity and predicate distributions. In this section, we conduct ablation studies in Table 3 on 1) single-stage vs two-stage training and 2) different sampling schemes. The first half of the table shows the performances of single-stage training, where the representation and the classifier are learned together. This clearly under-performs the two-stage training, which is listed in the second half of the table, where we compare different sampling strategies in the second stage of DT2. For the predicate classifier, it can be trained based on either SRS or class-balanced sampling for predicates (Predicate-CBS). Since each relation comes with a subject and an object, it is possible to train the entity classifier with respect to Predicate-CBS, indicating the entity classifier can be trained based on SRS, Predicate-CBS or class-balanced sampling for entities (Entity-CBS). Note that the predicate classifier can not be trained with Entity-CBS, since an entity does not always belong to a visual relation tuple. From the second half of the table, we find that considering the distribution differences in predicates and entities is important, because DT2-Predicate CBS (i.e. Predicate-CBS for both entity and predicate classifier) does not perform as well as DT2-Indep. CBS (i.e. Entity-CBS for the entity classifier and Predicate-CBS for the predicate classifier). The observation that DT2-Indep. CBS already performs better than existing methods (Table 1) supports our claim that visual relations can be effectively modeled with a simple architecture if the long-tailed aspect of the problem is considered. Nevertheless, the proposed ACBS further improves the SGCls performance by distilling the knowledge between P-step and E-step (see Algorithm 1).
5.4 Qualitative results
Figure 6 presents qualitative results of DT2-ACBS. In PredCls task, DT2-ACBS can correctly predict populated predicate classes (has & wearing) as well as non-populated predicate classes (walking on). Not only robust to long-tailed predicate classes, DT2-ACBS is also able to classify entities ranging from more populated classes (boy) to tail classes (sneaker). We can observe that while the predicted predicates can be different from the ground truth, the relation can still be reasonable (e.g. a subclass or a synonym of the ground truth). For example, the predicted predicate “walking on” is actually a subclass of the ground truth predicate “on”. These examples show that DT2-ACBS is able to predict more fine-grained predicates in tail classes and provide more exciting descriptions of the scene.
6 Conclusions
Learning visual relations is inherently a long-tailed problem. Existing approaches have mostly proposed complex models to learn visual relations. However, complex models are ill-suited for long-tailed problems, due to their tendency to overfit. In this paper, we consider the uniqueness of visual relations, where entities and relations have skewed distributions. We propose a simple model, namely DT2, along with an alternating sampling strategy (ACBS) to tackle the long-tailed visual relation problem. Extensive experiments on the benchmark VG150 dataset show that DT2-ACBS significantly outperforms the state-of-the-art methods of more complex architectures.
Acknowledgements This work was funded by NSF awards IIS-1924937, IIS-2041009 and a gift from Amazon.
References
- [1] Kaidi Cao, Colin Wei, Adrien Gaidon, Nikos Arechiga, and Tengyu Ma. Learning imbalanced datasets with label-distribution-aware margin loss. In Advances in Neural Information Processing Systems (NIPS), 2019.
- [2] Nitesh V. Chawla, Kevin W. Bowyer, Lawrence O. Hall, and W. Philip Kegelmeyer. Smote: Synthetic minority over-sampling technique. J. Artif. Int. Res., 16(1):321–357, June 2002.
- [3] Tianshui Chen, Weihao Yu, Riquan Chen, and Liang Lin. Knowledge-embedded routing network for scene graph generation. In Conference on Computer Vision and Pattern Recognition, 2019.
- [4] Vincent S. Chen, Paroma Varma, Ranjay Krishna, Michael Bernstein, Christopher Re, and Li Fei-Fei. Scene graph prediction with limited labels. In The IEEE International Conference on Computer Vision (ICCV), October 2019.
- [5] Yin Cui, Menglin Jia, Tsung-Yi Lin, Yang Song, and Serge Belongie. Class-balanced loss based on effective number of samples. In IEEE Conference on Computer Vision and Pattern Recognition (CVPR), 2019.
- [6] Qi Dong, Shaogang Gong, and Xiatian Zhu. Class rectification hard mining for imbalanced deep learning. In International Conference on Computer Vision (ICCV), 10 2017.
- [7] Apoorva Dornadula, Austin Narcomey, Ranjay Krishna, Michael Bernstein, and Li Fei-Fei. Visual relationships as functions: Enabling few-shot scene graph prediction. CoRR, abs/1906.04876, 2019.
- [8] Chris Drummond and Robert Holte. C4.5, class imbalance, and cost sensitivity: Why under-sampling beats oversampling. Proceedings of the ICML’03 Workshop on Learning from Imbalanced Datasets, 01 2003.
- [9] Jiuxiang Gu, Handong Zhao, Zhe Lin, Sheng Li, Jianfei Cai, and Mingyang Ling. Scene graph generation with external knowledge and image reconstruction. In The IEEE Conference on Computer Vision and Pattern Recognition (CVPR), June 2019.
- [10] Haibo He, Yang Bai, E. A. Garcia, and Shutao Li. Adasyn: Adaptive synthetic sampling approach for imbalanced learning. In 2008 IEEE International Joint Conference on Neural Networks (IEEE World Congress on Computational Intelligence), pages 1322–1328, 2008.
- [11] Hui Han, Wen-Yuan Wang, and Bing-Huan Mao. Borderline-smote: A new over-sampling method in imbalanced data sets learning. Advances in Intelligent Computing, 3644:878–887, 09 2005.
- [12] H. He and E. A. Garcia. Learning from imbalanced data. IEEE Transactions on Knowledge and Data Engineering, 21(9):1263–1284, Sep. 2009.
- [13] Kaiming He, Xiangyu Zhang, Shaoqing Ren, and Jian Sun. Deep residual learning for image recognition. In IEEE Conference on Computer Vision and Pattern Recognition (CVPR), 2016.
- [14] Roei Herzig, Moshiko Raboh, Gal Chechik, Jonathan Berant, and Amir Globerson. Mapping images to scene graphs with permutation-invariant structured prediction. CoRR, abs/1802.05451, 2018.
- [15] Geoffrey Hinton, Oriol Vinyals, and Jeff Dean. Distilling the knowledge in a neural network. arXiv preprint arXiv:1503.02531, 2015.
- [16] Drew A. Hudson and Christopher D. Manning. GQA: A new dataset for real-world visual reasoning and compositional question answering. In The IEEE Conference on Computer Vision and Pattern Recognition (CVPR), June 2019.
- [17] Zih-Siou Hung, Arun Mallya, and Svetlana Lazebnik. Union visual translation embedding for visual relationship detection and scene graph generation. CoRR, abs/1905.11624, 2019.
- [18] Muhammad Abdullah Jamal, Matthew Brown, Ming-Hsuan Yang, Liqiang Wang, and Boqing Gong. Rethinking class-balanced methods for long-tailed visual recognition from a domain adaptation perspective. In Proceedings of the IEEE/CVF Conference on Computer Vision and Pattern Recognition (CVPR), June 2020.
- [19] Justin Johnson, Agrim Gupta, and Li Fei-Fei. Image generation from scene graphs. In Proceedings of the IEEE Conference on Computer Vision and Pattern Recognition (CVPR), June 2018.
- [20] J. Johnson, R. Krishna, M. Stark, L. Li, D. A. Shamma, M. S. Bernstein, and L. Fei-Fei. Image retrieval using scene graphs. In 2015 IEEE Conference on Computer Vision and Pattern Recognition (CVPR), volume 00, pages 3668–3678, June 2015.
- [21] Bingyi Kang, Saining Xie, Marcus Rohrbach, Zhicheng Yan, Albert Gordo, Jiashi Feng, and Yannis Kalantidis. Decoupling representation and classifier for long-tailed recognition. In International Conference on Learning Representations (ICLR), 2020.
- [22] Ranjay Krishna, Yuke Zhu, Oliver Groth, Justin Johnson, Kenji Hata, Joshua Kravitz, Stephanie Chen, Yannis Kalantidis, Li-Jia Li, David A. Shamma, Michael S. Bernstein, and Fei-Fei Li. Visual genome: Connecting language and vision using crowdsourced dense image annotations. CoRR, abs/1602.07332, 2016.
- [23] Rongjie Li, Songyang Zhang, Bo Wan, and Xuming He. Bipartite graph network with adaptive message passing for unbiased scene graph generation. In CVPR, 2021.
- [24] Yikang Li, Tao Ma, Yeqi Bai, Nan Duan, Sining Wei, and Xiaogang Wang. Pastegan: A semi-parametric method to generate image from scene graph. In Advances in Neural Information Processing Systems 32: Annual Conference on Neural Information Processing Systems 2019, NeurIPS 2019, 8-14, December 2019, Vancouver, BC, Canada, pages 3950–3960, 2019.
- [25] Yikang Li, Wanli Ouyang, Bolei Zhou, Jianping Shi, Chao Zhang, and Xiaogang Wang. Factorizable net: An efficient subgraph-based framework for scene graph generation. In The European Conference on Computer Vision (ECCV), September 2018.
- [26] Z. Li and D. Hoiem. Learning without forgetting. IEEE Transactions on Pattern Analysis and Machine Intelligence, 40(12):2935–2947, 2018.
- [27] Tsung-Yi Lin, Priyal Goyal, Ross Girshick, Kaiming He, and Piotr Dollar. Focal loss for dense object detection. IEEE Transactions on Pattern Analysis and Machine Intelligence, PP:1–1, 07 2018.
- [28] Ziwei Liu, Zhongqi Miao, Xiaohang Zhan, Jiayun Wang, Boqing Gong, and Stella X. Yu. Large-scale long-tailed recognition in an open world. In IEEE Conference on Computer Vision and Pattern Recognition (CVPR), 2019.
- [29] Son T. Nguyen, Ozgur S. Oguz, Valentin N. Hartmann, and Marc Toussaint. Self-supervised learning of scene-graph representations for robotic sequential manipulation planning. In 4rd Annual Conference on Robot Learning, CoRL 2020, Proceedings, Proceedings of Machine Learning Research. PMLR, 2020.
- [30] Shaoqing Ren, Kaiming He, Ross Girshick, and Jian Sun. Faster r-cnn: Towards real-time object detection with region proposal networks. In C. Cortes, N. Lawrence, D. Lee, M. Sugiyama, and R. Garnett, editors, Advances in Neural Information Processing Systems, volume 28, pages 91–99. Curran Associates, Inc., 2015.
- [31] Kaihua Tang, Yulei Niu, Jianqiang Huang, Jiaxin Shi, and Hanwang Zhang. Unbiased scene graph generation from biased training. In 2020 IEEE/CVF Conference on Computer Vision and Pattern Recognition, CVPR 2020, Seattle, WA, USA, June 13-19, 2020, pages 3713–3722. IEEE, 2020.
- [32] Kaihua Tang, Hanwang Zhang, Baoyuan Wu, Wenhan Luo, and Wei Liu. Learning to compose dynamic tree structures for visual contexts. In The IEEE Conference on Computer Vision and Pattern Recognition (CVPR), June 2019.
- [33] Hung-Yu Tseng, Hsin ying Lee, Lu Jiang, Ming-Hsuan Yang, and Weilong Yang. RetrieveGAN: Image synthesis via differentiable patch retrieval. In ECCV, 2020.
- [34] Sijin Wang, Ruiping Wang, Ziwei Yao, Shiguang Shan, and Xilin Chen. Cross-modal scene graph matching for relationship-aware image-text retrieval. In IEEE Winter Conference on Applications of Computer Vision, WACV 2020, Snowmass Village, CO, USA, March 1-5, 2020, pages 1497–1506. IEEE, 2020.
- [35] Yu-Xiong Wang and Martial Hebert. Learning to learn: Model regression networks for easy small sample learning. In European Conference on Computer Vision (ECCV), 2016.
- [36] Yu-Xiong Wang, Deva Ramanan, and Martial Hebert. Learning to model the tail. In Advances in Neural Information Processing Systems (NIPS), 2017.
- [37] Bin Wen, Jie Luo, Xianglong Liu, and Lei Huang. Unbiased scene graph generation via rich and fair semantic extraction, 2020.
- [38] Sanghyun Woo, Dahun Kim, Donghyeon Cho, and In So Kweon. Linknet: Relational embedding for scene graph. In S. Bengio, H. Wallach, H. Larochelle, K. Grauman, N. Cesa-Bianchi, and R. Garnett, editors, Advances in Neural Information Processing Systems 31, pages 560–570. Curran Associates, Inc., 2018.
- [39] Tz-Ying Wu, Pedro Morgado, Pei Wang, Chih-Hui Ho, and Nuno Vasconcelos. Solving long-tailed recognition with deep realistic taxonomic classifier. In European Conference on Computer Vision (ECCV), 2020.
- [40] Liuyu Xiang and G. Ding. Learning from multiple experts: Self-paced knowledge distillation for long-tailed classification. In European Conference on Computer Vision (ECCV), 2020.
- [41] Danfei Xu, Yuke Zhu, Christopher Choy, and Li Fei-Fei. Scene graph generation by iterative message passing. In Computer Vision and Pattern Recognition (CVPR), 2017.
- [42] Shaotian Yan, Chen Shen, Zhongming Jin, Jianqiang Huang, Rongxin Jiang, Yaowu Chen, and Xian-Sheng Hua. PCPL: predicate-correlation perception learning for unbiased scene graph generation. In MM ’20: The 28th ACM International Conference on Multimedia, Virtual Event / Seattle, WA, USA, October 12-16, 2020, pages 265–273, 2020.
- [43] Jianwei Yang, Jiasen Lu, Stefan Lee, Dhruv Batra, and Devi Parikh. Graph r-cnn for scene graph generation. In The European Conference on Computer Vision (ECCV), September 2018.
- [44] Xu Yang, Kaihua Tang, Hanwang Zhang, and Jianfei Cai. Auto-encoding scene graphs for image captioning. In The IEEE Conference on Computer Vision and Pattern Recognition (CVPR), June 2019.
- [45] Ting Yao, Yingwei Pan, Yehao Li, and Tao Mei. Exploring visual relationship for image captioning. In The European Conference on Computer Vision (ECCV), September 2018.
- [46] Alireza Zareian, Svebor Karaman, and Shih-Fu Chang. Bridging knowledge graphs to generate scene graphs. In Proceedings of the European conference on computer vision (ECCV), August 2020.
- [47] Alireza Zareian, Svebor Karaman, and Shih-Fu Chang. Weakly supervised visual semantic parsing. In IEEE/CVF Conference on Computer Vision and Pattern Recognition (CVPR), June 2020.
- [48] Rowan Zellers, Mark Yatskar, Sam Thomson, and Yejin Choi. Neural motifs: Scene graph parsing with global context. CoRR, abs/1711.06640, 2017.
- [49] Ji Zhang, Kevin J. Shih, Ahmed Elgammal, Andrew Tao, and Bryan Catanzaro. Graphical contrastive losses for scene graph generation. CoRR, abs/1903.02728, 2019.
- [50] Boyan Zhou, Quan Cui, Xiu-Shen Wei, and Zhao-Min Chen. BBN: Bilateral-branch network with cumulative learning for long-tailed visual recognition. In CVPR, pages 1–8, 2020.
- [51] Yang Zou, Zhiding Yu, B.V.K. Vijaya Kumar, and Jinsong Wang. Unsupervised domain adaptation for semantic segmentation via class-balanced self-training. In European Conference on Computer Vision (ECCV), 2018.
Appendix A Model complexity
The model complexity is quite low for DT2, which has 10 fewer trainable parameters than most of the recent approaches in the literature. For example, the SGCls model sizes of DT2, MOTIFS [48], VCTree [32] and TDE-MOTIFS [31] are 224 MB, 1.68 GB, 1.65 GB and 2.1 GB respectively. This is by design, since our goal is to emphasize the importance of accounting for long tails during training.
![]() |
![]() |
![]() |
![]() |
![]() |
![]() |
Appendix B Additional results
In this section, we provide additional results of the proposed DT2-ACBS.
B.1 More visual examples
In addition to section 5.4, Figure 7 presents more visual examples of PredCls (left column) and SGCls (right column) generated by DT2-ACBS. These examples show that DT2-ACBS can predict predicates ranging from head classes such as has and wearing to less populated classes like walking on. While some examples are counted as incorrect predictions under the metric, as discussed in the main paper, they are still reasonable predictions such as a subclass or a synonym of the ground truth. For example, belonging to/of and wears/wearing. In the SGCls task, DT2-ACBS correctly predicts head entities (boy, horse, and head) and tail entities (racket and sock).
B.2 Ablations on appearance branch
As discussed in section 4.2, the goal of appearance branch is to convey the image information not encoded in the entity labels but relevant to predicate predictions. We tested the effectiveness of the appearance branch by removing it and training the network with ACBS. Table 4 shows that entity classification accuracy remains similar, but PredCls and SGCls performance drops dramatically, i.e. the appearance branch contributes substantially to predicate classification. Note that the gains hold even when the ground truth entity labels are used (PredCls), confirming the argument that simply knowing entity classes is not enough for predicate prediction.
B.3 E-step as teacher
Note that the predicate and entity weight matrices are interdependent. Using E-step as the teacher would negatively affect . In ACBS, receives class-balanced entity supervision, so there is no risk of overfitting. The role of the teacher is to guarantee that the E-step update of is not incompatible with the P-step update of . This distillation is exactly how ACBS fuses the knowledge learnt with different distributions. Using E-step as the teacher has weaker results, as shown in Table 5.
B.4 Recall values
The metric of Recall@K is highly biased toward dominated classes (such as “on”), and thus it is not suitable for long-tailed visual relations, as discussed in the main paper. However, we provide the numbers in Table 6 for reference.
Method | PredCls | SGCls | (subj, obj) |
mR@ 20 / 50 / 100 | mR@ 20 / 50 / 100 | Acc. | |
w/o | 18.1 / 24.5 / 26.8 | 11.0 / 14.7 / 16.3 | 25.77 |
w/ (ours) | 27.4 / 35.9 / 39.7 | 18.7 / 24.8 / 27.5 | 26.26 |
Teacher | mR@ 20 / 50 / 100 |
---|---|
E-step | 15.2 / 20.2 / 22.0 |
P-step (ours) | 18.7 / 24.8 / 27.5 |
Method | Predicate Classification | Scene Graph Classification | Scene Graph Detection | |||
---|---|---|---|---|---|---|
R@50 / 100 | mR@50 / 100 | R@50 / 100 | mR@50 / 100 | R@50 / 100 | mR@50 / 100 | |
KERN [3] | 65.8 / 67.6 | 17.7 / 19.2 | 36.7 / 37.4 | 9.4 / 10.0 | 27.1 / 29.8 | 6.4 / 7.3 |
TDE-MOTIFS-SUM [31] | 46.2 / 51.4 | 25.5 / 29.1 | 27.7 / 29.9 | 13.1 / 14.9 | 16.9 / 20.3 | 8.2 / 9.8 |
TDE-VCTree-SUM [31] | 47.2 / 51.6 | 25.4 / 28.7 | 25.4 / 27.9 | 12.2 / 14.0 | 19.4 / 23.2 | 9.3 / 11.1 |
PCPL [42] | 50.8 / 52.6 | 35.2 / 37.8 | 27.6 / 28.4 | 18.6 / 19.6 | 14.6 / 18.6 | 9.5 / 11.7 |
DT2-ACBS (ours) | 23.3 / 25.6 | 35.9 / 39.7 | 16.2 / 17.6 | 24.8 / 27.5 | 15.0 / 16.3 | 22.0 / 24.4 |
Appendix C Implementation Details
DT2-ACBS is a two-stage training process. While SRS is adopted in the first stage when training the parameter of , and , the proposed ACBS is adopted in the second stage to learn the classifiers. Apart from the differences in sampling strategies, both stages share a similar optimization scheme, where the Adam optimizer with initial learning rate is adopted, with the learning rate decay of for every 5 epochs. The batch size in the first stage is , while in the second stage, objects and predicates are sampled with and samples per class respectively. The hyperparameters , and are set to , and respectively using the validation set. For evaluation on SGG tasks, we adopt the protocol of [48, 32] to filter out the subject-object pairs that do not have a relationship.