Learning a Sketch Tensor Space for Image Inpainting of Man-made Scenes
Abstract
This paper studies the task of inpainting man-made scenes. It is very challenging due to the difficulty in preserving the visual patterns of images, such as edges, lines, and junctions. Especially, most previous works are failed to restore the object/building structures for images of man-made scenes. To this end, this paper proposes learning a Sketch Tensor (ST) space for inpainting man-made scenes. Such a space is learned to restore the edges, lines, and junctions in images, and thus makes reliable predictions of the holistic image structures. To facilitate the structure refinement, we propose a Multi-scale Sketch Tensor inpainting (MST) network, with a novel encoder-decoder structure. The encoder extracts lines and edges from the input images to project them into an ST space. From this space, the decoder is learned to restore the input images. Extensive experiments validate the efficacy of our model. Furthermore, our model can also achieve competitive performance in inpainting general nature images over the competitors.
![[Uncaptioned image]](https://cdn.awesomepapers.org/papers/12eff473-f223-424b-a29f-1f5c99224d28/x1.png)
1 Introduction
As a long-standing problem, image inpainting has been studied to address the problem of filling in the missing parts of the images being semantically consistent and visually realistic with plausible results. Thus, image inpainting is useful to many real-world applications, e.g. image restoration, image editing, and object removal [6].
Intrinsically as an inverse problem, the inpainting is challenging in both restoring the missed global structure (semantically consistent), and generating realistic regions locally coherent to unmasked regions (visually consistent). Especially, it is hard to reconstruct the missed image regions from the complex man-made scenes and structures, due to the difficulty in preserving the prominent low-level visual patterns, such as edges, line segments, and junctions, as shown in Fig. 1. To this end, this paper particularly focuses on learning to reconstruct these visual patterns for image inpainting, and proposes a method of the best merits in repairing the masked regions of man-made scene images, such as images with indoor and outdoor buildings.
Both traditional approaches [2, 8, 19] and deep learning methods [24, 44, 40, 17, 43, 21] had made great efforts on reconstructing the structures of images in producing visually realistic results. However, these methods are still challenged by producing structurally coherent results, especially in the inpainting of man-made scenes. Typically, inpainting approaches may suffer from the following problems. (1) Missing critical structures. Traditional synthesis-based approaches are normally unable to model the critical structures as in Fig. 1(b). On the other hand, recent learning-based inpainting methods utilize auxiliary information to support the inpainting, e.g., edges [28, 20], and segmentation [32, 22], predominantly inpainting local visual cues, rather than holistic structures of man-made scenes. For example, the results of EdgeConnect [28] with canny edges [5] in Fig. 1(d) suffer from broken and blurry line segments and lose connectivity of building structures. (2) Unreliable pattern transfer. The learning-based auxiliary detectors will transfer and magnify the unreliable image priors or patterns to the masked image regions, which causes degraded inpainting results [22]. (3) Trading off performance and efficiency. The auxiliary-based inpainting methods usually consume more computation, due to additional components or training stages [28, 20, 39]. But these methods still have artifacts in junction regions as framed with red dotted lines in Fig. 1(c)(d). Therefore, the design of a more effective network is expected to efficiently enhance the inpainting performance.
To address these issues, our key idea is to learn a Sketch Tensor (ST) space by an encoder-decoder model. (1) The encoder learns to infer both local and holistic critical structures of input images, including canny edges and compositional lines. The image is encoded as the binarized ‘sketch’ style feature maps, dubbed as sketch tensor. The decoder takes the restored structure to fill in holes of images. (2) For the first time, the idea of parsing wireframes [14] is re-purposed to facilitate inpainting by strengthening the holistic structures of man-made scenes with more effective and flexible holistic structures. We propose a Line Segment Masking (LSM) algorithm to effectively train the wireframe parser, which alleviates unreliable structure guidance from corrupted images and the heavy computation of auxiliary detectors during the training phase. Besides, LSM also leverage the separability of line segments to extend the proposed model to obtain better object removal results. (3) Most importantly, we significantly boost the training and inference process of previous inpainting architectures. Thus, a series of efficient modules are proposed, which include partially gated convolutions, efficient attention module, and Pyramid Decomposing Separable (PDS) blocks. Critically, we present PDS blocks to help better learn binary line and edge maps. Our proposed modules make a good balance of model performance and training efficiency.
Formally, this paper proposes a novel Multi-scale Sketch Tensor inpainting (MST) network with an encoder-decoder structure. The encoder employs LSM algorithm to train an hourglass wireframe parser [38] and a canny detector to extract line and edge maps. These maps concatenated with input images are projected into ST space by Pyramid Structure Sub-encoder (PSS), which is sequentially constructed by 3 partially gated convolution layers, 8 dilated residual block layers with an efficient attention module, and 3 pyramid decomposing block layers as in Fig. 2. The image is encoded as a third-order sketch tensor in ST space, representing local and holistic structures. Finally, the decoder is stacked by two groups of 3 partially gated convolution layers for both ends with 8 residual block layers, which will re-project the sketch tensor into the restored image.
We highlight several contributions here. (1) We propose learning a novel sketch tensor space for inpainting tasks. Such a space is learned to to restore the critical missed structures and visual patterns, and makes reliable predictions of the holistic image structures. Essentially, the skech tensor has good interpretability of input images, as empirically shown in experiments. (2) For the first time, the wireframe parsing has been re-purposed to extract lines and junctions for inpainting. A novel line segment masking algorithm is proposed to facilitate training our inpainting wireframe parser. (3) We introduce the novel partially gated convolution and efficient attention module to significantly improve model performance without incurring additional expensive computational cost than competitors, such as EdgeConnect. (4) A novel pyramid decomposing separable block is proposed to address the issue of effectively learning sparse binary edge and line maps. (5) Extensive experiments on the dataset of both man-made and natural scenes, including ShanghaiTech [14], Places2 [48], and York Urban [4]. show the efficacy of our MST-net, over the competitors.
2 Related work
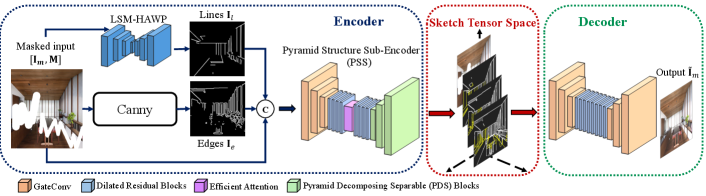
Image Inpainting by Auxiliaries. The auxiliary information of semantics and structures have been utilized to help inpainting tasks in traditional methods, such as lines, structures [13, 1], and approximation images [10]. Recently, deep learning based approaches take auxiliary information as the important prior, such as canny edges [28, 20], smoothed edges [29, 12], edge and gradient information [39], and semantic segmentation [32, 22]. Auxiliary information has been also utilized in image editing [47, 30, 15, 18]. Particularly, EdgeConnect [28] and GateConv [43] both leverage edges to inpaint masked areas with specific structural results. However, there is no holistic structure information for man-made scenes that has been explicitly modeled and utilized as auxiliary information in previous work. Such information is crucial to inpaint the images of complex structures, such as buildings, or indoor furniture. To this end, our method utilizes the separability and connectivity of line segments to help improve the performance of inpainting.
Line Detection Approaches. Line detection enjoys immense value in many real-world problems. Therefore, it has been widely researched and developed in computer vision. Many classic graphics algorithms are proposed to extract line segments from raw images [9, 34, 26]. However, these traditional methods suffer from intermittent and spurious line segments. Moreover, the extracted lines lack positions of junctions which causes poor connectivity. Huang et al. [14] propose a deep learning based wireframe parsing to improve the line detection task, which uses DNNs to predict heatmaps of junctions and line segments as the wireframe representation. LCNN proposed in [49] leverages heuristic sampling strategies and a line verification network to improve the performance of wireframe parsing. Furthermore, Xue et al. [38] utilize the holistic attraction field to enhance both efficiency and accuracy for the wireframe parser. Although wireframe parsing based models enjoy distinctive strengths, to the best of our knowledge, no previous work has considiered utilizing wireframe parser to help preserve line structures for downstreaming inpainting task. Critically, for the first time, our method first repurposes the wireframe parser to facilitate image inpainting, which leverages connectivity and structural information of wireframes to achieve better inpainting performance.
3 Multi-scale Sketch Tensor Inpainting
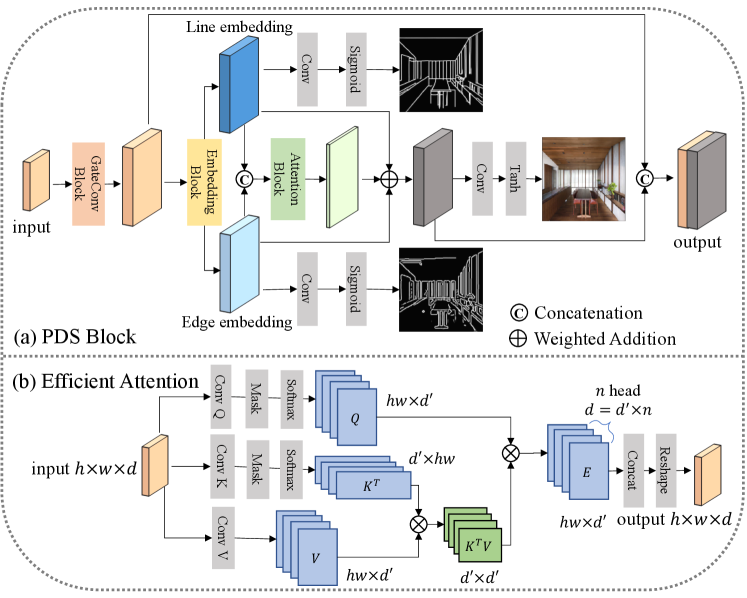
Overview. The MST network is shown in Fig. 2. Given the input masked image and corresponding binary mask , MST has three key components, i.e., encoder , decoder , and Sketch Tensor (ST) space of a third-order tensor denoted by . Particularly, the encoder firstly employs the improved wireframe parser LSM-HAWP and canny detector [5] to extract line and edge maps ; then the concatenated image and maps is processed by Pyramid Structure Sub-Encoder (PSS) to produce the ST space . The decoder predicts inpainted image , closer to ground-truth image .
In this section, Sec. 3.1 will introduce the LSM algorithm. Details about the PSS and ST space are specified in Sec. 3.2. Finally, the decoder will be discussed in Sec. 3.4.
3.1 Line Segment Masking Algorithm
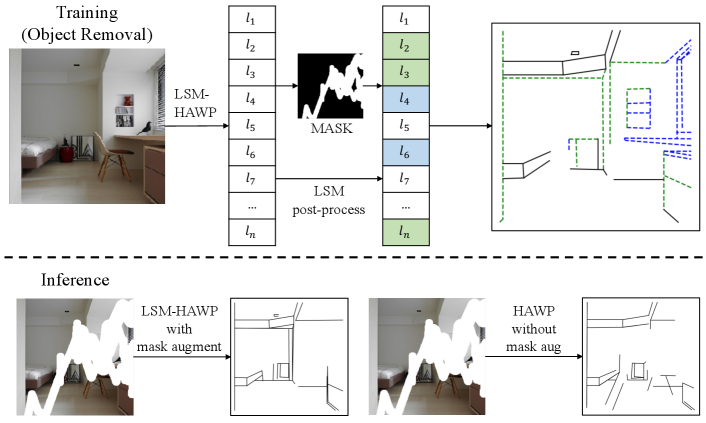
The wireframe parser HAWP [38] is adopted to extract lines from images. Specifically, it extracts the junction set , and line set paired by junctions in . Unfortunately, if lines are corrupted by the mask , the results of naive HAWP [38] will be largely degraded as shown in the inference stage of Fig. 4 with broken structures.
To this end, we propose an LSM algorithm to infer missed line segments with the flexible wireframe parsing, which is composed of two parts. 1) Learning an LSM-HAWP network by retraining HAWP with the irregular [43] and object segmentation [45] masks. This not only improves the stability for the corrupted images, but also achieves superior results in trivial wireframe parsing tasks (Sec. 5). 2) Introducing an indicator function to denote the masking probability of each line segment according to the mask as the post-processing for LSM-HAWP,
(1) |
where is a hyper-parameter between 0 and 1, and junction means that is masked or not. Fig. 4 illustrates the LSM post-process in training and inference stages. In training, we set to train the first half epoches, and for training in the rest epoches. In the object removal task, we use the unmasked image with to retain necessary structures.
Thus as one practical strategy of speedup the learning process, we extract all wireframes beforehand and filtering them by the post-process of LSM, rather than an end-to-end training with LSM-HAWP, which dramatically improves the training efficiency. Note that in the testing stage for inpainting, corrupted images are used as the input to make our results fair comparable to the competitors.
3.2 Pyramid Structure Sub-Encoder
The LSM-HAWP and canny detector extract the line and edge maps respectively. Essentially, is binary map, and is got from connecting junction pairs from LSM-HAWP with anti-aliased lines. The input to PSS is the concatenation of the masked image and structure maps . As shown in Fig. 2, the PSS is composed of partially gated convolutions, dilated [41] residual blocks, efficient attention, and pyramid decomposing separable blocks, which will be explained next. For the detailed structures, please refer to our supplementary.
Partially Gated Convolutions. We adopt the Gated Convolution (GC) layers to process the masked input features, as it works well for the irregular mask inpainting tasks in [43]. Unfortunately, GC demands much more trainable parameters than vanilla convolutions. To this end, as shown in Fig. 2 and Fig. 3(a), we propose a partially GC strategy of only utilizing three GC layers for the input and output features in both encoder and decoder models. Essentially, this is motivated by our finding that the outputs of GC mostly devoting to filtering features of masked and unmasked regions only in the encoder layers of the coarse network and the decoder layers of the refinement network in [43]. In contrast, we do not observe significantly performance improvement of using GC in the middle layers of backbones. Thus, we maintain vanilla convolutions (i.e., residual blocks) in the middle layers, to save parameters and improve the performance as empirically validated in Tab. 4.
Efficient Attention Block. Intuitively, attention is important to learn patterns crossing spatial locations in image inpainting [42, 25, 21, 39]. However, attention modules are expensive to be computed, and non-trivial to be parallelized in image inpainting [42]. To this end, we leverage Efficient Attention (EA) module among middle blocks of Fig. 2 and detailed in Fig. 3(b). Particularly, for the input feature , and mask , we first reshape them to and as the vector data respectively. Then, the process of efficient attention can be written as
(2) |
where indicates different learned parameter matrices; means masking the corrupted inputs before the softmax operation. Critically, and means the softmax operations on the row and column individually. Then, we achieve the output , which will be reshaped back to . Note that Eq. (2) is an approximation to vanilla attention operation as in [31]. Typically, this strategy should in principle, reduce significantly computational cost. Since is computed by , rather than standard . In practice, the dimension is much smaller than in computer vision tasks. Furthermore, as in Fig. 3(b), we introduce the multi-head attention [33] to further reduce the dimension from to . Thus, could aggregate the query in feature level and obtain the global context . Note that EA module is inspired but different from [31], as the attention scores of corrupted regions are masked to aggregate features from uncorrupted regions.
Pyramid Decomposing Separable (PDS) Block. We have found that GAN-based edge inpainting [35] suffers from generating meaningless edges or unreasonable blanks for masked areas as shown in Fig. 6, due to the nature of sparsity in binary edge maps. Thus, we propose a novel PDS block in PSS as in Fig. 3(a). PDS leverages dilated lower-scale structures to make up the sparse problem for ST space. Particularly, assume the image feature , PDS firstly decouples it as
(3) |
where is sigmoid activation. We project the feature into two separated embedding spaces called line embedding and edge embedding with the function , which is composed with . is the operation of splitting the features into two respective tensors with equal channels. Then, and are utilized to learn to roughly reconstruct the image without corruption as
(4) |
where is . denotes the element-wise product. The prediction of coarse gives a stronger constraint to the conflict of and , and we expect the attention map can be learned adaptively according to a challenging target. Furthermore, a multi-scale strategy is introduced in the PDS. For the given feature from dilated residual blocks of PSS, PDS predicts lines, edges, and coarse images with different resolutions as follow
(5) |
where indicate that the output maps with 6464, 128128, and 256256 resolutions respectively. Various scales of images can be in favor of reconstructing different types of structure information.
Loss Function of PSS. We minimize the objectives of PPS with two spectral norm [27] based SN-PathGAN [43] discriminators and for lines and edges respectively. And the and are indicated as
(6) |
where , are the multi-scale outputs (Eq. 5) and ground-truth images varying image size to respectively. is the feature matching loss as in [28] to restrict the loss between discriminator features from the concatenated coarse-to-fine real and fake sketches discussed below. And the adversarial loss can be further specified as
(7) |
where , are got from the multi-scale PSS outputs of lines and edges, which are upsampled and concatenated with and . To unify these outputs into the same scale, the nearest upsampling is utilized here. Accordingly, , and are the concatenation of multi-scale ground-truth edge and line maps, respectively. Note that, we firstly dilate the ground-truth edges and lines with the kernel, and then subsample them to produce the low resolution maps at the scale of 6464, 128128. The hyper-parameters are set as , .
3.3 Sketch Tensor (ST) Space
The last outputs of Eq. 5 is used to compose the ST space as
(8) |
Generally, lines represent holistic structures, while edges indicate some local details. They provide priors of structures in different manners to the inpainting model. We also combine and clip them within 0 and 1 to emphasize overlaps and make an intuitive expression of the whole structure.
3.4 Decoder of MST Network
The structure of the decoder is the same as PSS except that the decoder has no attention blocks and PSD blocks. Because we find that the generator in [28] is sufficient to generate fine results with reasonable structure information. But we still add gated mechanism to the leading and trailing three convolutions to improve the performance for the irregular mask. As the encoder has provided the ST space in Eq. 8, we can get the inpainted image with the masked input and mask as
(9) |
We use SN-PathGAN [43] based discriminator for the decoder, and the objectives to be minimized are
(10) |
(11) |
where is the origin undamanged image for training. We adopt the loss , VGG-19 based perceptual loss [16] , and style-loss [7] to train our model, with the empirically setted hyperparameters , [28, 12]. We propose using the balanced loss form [3] to implement reconstruction losses and , normalizing by the weights of masked region and unmasked region . The balanced loss form can settle the inpainting task with imbalanced irregular masks properly.
Note that we learn the encoder and the decoder jointly in the forward pass. The gradients of the decoder will not be propagated to update the encoder, which not only saves the memory but also maintains the interpretability of the sketch tensor . Generally, the total parameter number and calculation costs of our model are comparable to [28].
4 Experiments and Results
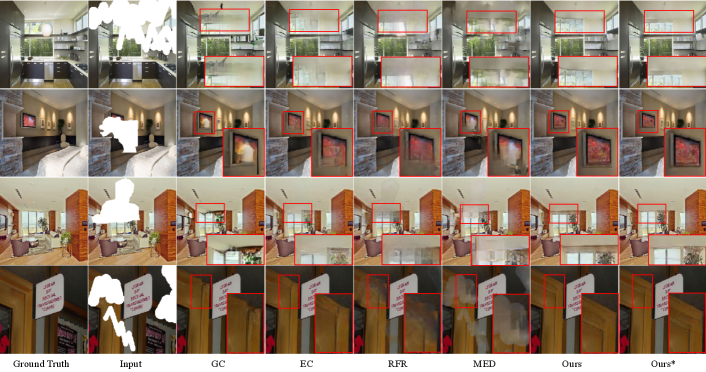
|
Datasets. The proposed approach is evaluated on three datasets: ShanghaiTech [14], Places2 [48], and York Urban [4]. ShanghaiTech contains 5000 training images and 462 test images consisted of buildings and indoor scenes with wireframe labels. The LSM-HAWP wireframe detection model is trained on this dataset with the mask augmentation. For Places2, we select 10 categories images according to the number of line segments detected by HAWP as man-made scenes (P2M). Moreover, we randomly select 10 Places2 classes with both natural and urban scenes as comprehensive scenes (P2C)111Details about Places2 are illustrated in the supplementary.. For York Urban, it has 102 city street view images for testing models trained with P2M.
Implementation Details. Our method is implemented with PyTorch in image size. All models are trained with Adam optimizer of and , and the initial learning rates are and for generators and discriminators respectively. We train the model with 400k steps in ShanghaiTech and 1000k steps in Places2. Besides, is trained with 1/3 of the total steps, and then be fixed. We decay the learning rate with for each 100k steps. For the structure information, line segments are extracted by HAWP, and Canny edges are got with . Our model is trained in Pytorch v1.3.1, and costs about 2 days training in ShanghaiTech and about 5 days in Places2 with a single NVIDIA(R) Tesla(R) V100 16GB GPU.
Comparison Methods. We compare the proposed MST with some state-of-the-art methods, which include Gated Convolution (GC) [43], Edge Connect (EC) [28], Recurrent Feature Reasoning (RFR) [21], and Mutual Encoder-Decoder with Feature Equalizations (MED) [12]. These methods are all retrained with similar settings and costs compared with ours.
Settings of Masks. To handle the real-world image inpainting and editing tasks, such as object removal, the random irregular mask generation in [43, 37] is adopted in this paper. Besides, as discussed in [45], real-world inpainting tasks usually remove regions with typical objects or scenes segments. So we collect 91707 diverse semantic segmentation masks with various objects and scenes with the coverage rates in from the COCO dataset [23]. Overall, the final mask will be chosen from irregular masks and COCO segment masks randomly with in both training and test set. To be fair, all comparison methods are retrained with the same masking strategy.
4.1 Image Inpainting Results
For fair comparisons in image inpainting, we do not leak any line segments of the uncorrupted images for the image inpainting task and related discussions. The wireframe parser LSM-HAWP is trained in ShanghaiTech, and it predicts the line segments for the other two datasets. Besides, results from the object removal with in Eq. (1) are offered for reference only in this section.
Quantitative Comparisons. In this section, we evaluate results with PSNR, SSIM [36], and FID [11]. As discussed in [46], we find that based metrics such as PSNR and SSIM often contradict human judgment. For example, some meaningless blurring areas will cause large perceptual deviations but small loss [46]. Therefore, we pay more attention to the perceptual metric FID in quantitative comparisons. The results on ShanghaiTech, P2M, and York Urban are shown in Tab. 1. From the quantitative results, our method outperforms other approaches in PSNR and FID. Especially for the FID, which accords with the human perception, our method achieves considerable advantages. Besides, our method enjoys good generalization, as it also works properly in P2M, York Urban, and even P2C.
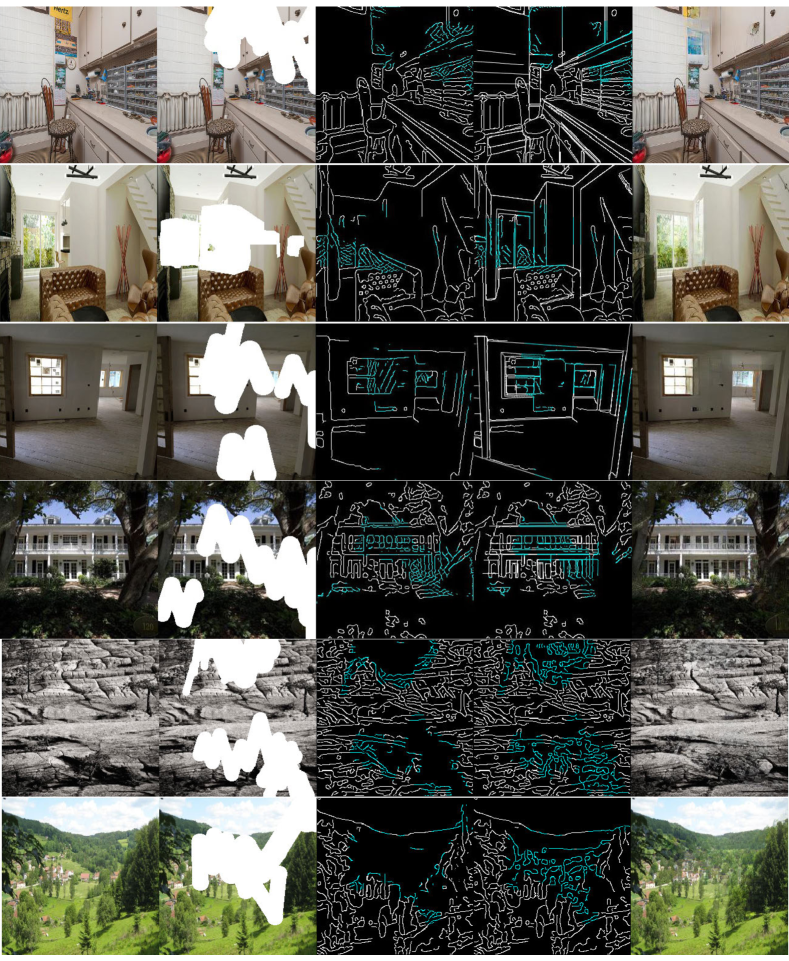
Qualitative Comparisons. Qualitative results among our method and other state-of-the-art methods are shown in Fig. 5. Compared with other methods, our approach achieves more semantically coherent results. From the enlarged regions, our schema significantly outperforms other approaches in preserving the perspectivity and the structures of man-made scenes. Moreover, we show the edge results of EC [28] and partial sketch tensor space (edges and lines) results of our method in ShanghaiTech, P2M, and P2C in Fig. 6. Results in Fig. 6 demonstrate that line segments can supply compositional information to avoid indecisive and meaningless generations in edges. Furthermore, line segments can also provide clear and specific structures in masked regions to reconstruct more definitive results in man-made scenes. Besides, for the natural scene images in P2C without any lines, our method can still outperform EC with reasonable generated edges for the masked regions. As discussed in Sec. 3.2, the proposed PDS works properly to handle the sparse generative problem.
Results of Natural Scenes. In the last three rows of Tab. 1, we present the results of ours method on the comprehensive Places2 dataset (P2C) to confirm the generalization. They are compared with GC and EC, which have achieved fine scores in man-made Places2 (P2M). Note that all metrics are improved in P2C compared with ones in P2M of Tab. 1, which demonstrates man-made scenes are more difficult to tackle. Our methods still get the best results among all competitors, and the object removal results achieve superior performance. Besides, the last two rows of Fig. 6 show that our method can generate reliable edge sketches even without lines in natural scenes. These phenomenons are largely due to two reasons: 1) There is still a considerable quantity of line segments in the comprehensive scenes, and these instances are usually more difficult than others. 2) The proposed partially gated convolutions, efficient attention, and PDS blocks can work properly for various scenes.
GC | EC | Ours | |
---|---|---|---|
S.-T. | 3.00 | 7.67 | 33.67 |
P2M | 6.67 | 8.67 | 32.33 |
Human Judgements. For more comprehensive comparisons, 50 inpainted images from GC [43], EC [28], and ours are chosen from ShanghaiTech and P2M randomly. And these samples are compared by 3 uncorrelated volunteers. Particularly, volunteers need to choose the best one from the mixed pool of the inpainted images with different methods, and give one score to the respective approach. If all methods work roughly the same for a certain sample, it will be ignored. The average scores are shown in Tab. 2. Ours method is significantly better than other competitors.
4.2 Other Applications and Ablation Study
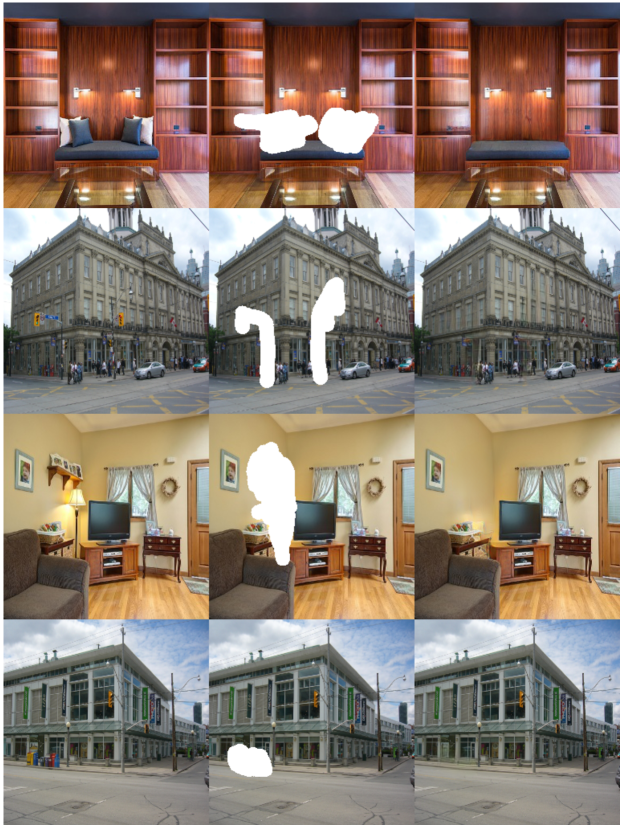
Object Removal. From the last column in Fig. 5, we show the inpainting results of our MST working in the post-process of LSM with for the object removal. These refined images with more reasonable structures indicate that the our model can strengthen the capability of image reconstruction without redundant residues. From Fig. 7, some object removal cases are shown to confirm the practicability of the proposed method. Numerical results are presented in the last columns of Tab. 1. So, our method can achieve further improvements in all metrics compared with the vanilla image inpainting schema for the object removal, and it can correctly mask undesired lines. Therefore, the post-process of LSM can significantly improve the performance in the object removal task with the separability of line segments .
|
PSNR | SSIM | FID | Param | |
---|---|---|---|---|
w/o GC | 26.51 | 0.871 | 24.84 | 10.8M |
Partially GC | 26.78 | 0.874 | 22.68 | 12.1M |
All GC | 26.71 | 0.874 | 22.62 | 25.1M |
|
Masked Wireframe Detection. As discussed in Sec. 3.1, we retrain the HAWP with mask augmentation to ensure the robustness for the corrupted data as LSM-HAWP in ShanghaiTech dataset [14]. To confirm the effect of the augmentation, we further prepare another masked ShanghaiTech testset with the same images. The results are shown in Tab. 3. The structural average precision (sAP) metric is proposed in [49], which is defined as the area under the PR curve of detected lines with different thresholds. From Tab. 3, LSM-HAWP performs better than vanilla HAWP significantly for the masked testset. Moreover, HAWP can also gain improvements in the uncorropted testset with more than 1% in sAP, which indicates the great generality of the mask augmentation for the wireframe detection task.
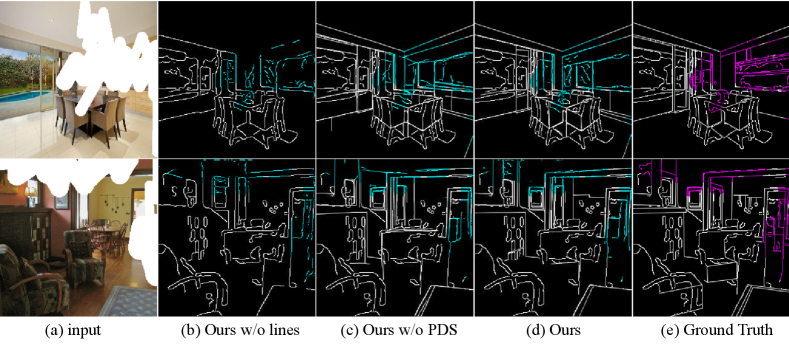
Simplification of Gated Convolutions. The related ablation study of GC are shown in Tab. 4. Only replacing vanilla convolutions for input and output stages of the model with partially GC gains satisfactory improvements. But replacing all convolution layers with GC fails to achieve a further large advance, while the parameters are doubled222The visualization results of GC are shown in the supplementary..
Contributions of Lines, PDS, and EA. We explored the effects of lines, Pyramid Decomposing Separable (PDS) blocks and the Efficient Attention (EA) module in Tab. 5 in ShanghaiTech. Specifically, line segments, PDS blocks and the EA module are removed respectively, while other settings unchanged. As shown in Fig. 8, our method without line segments causes severe structural loss, and the one without PDS suffers from contradictory perspectivity. From Tab. 5, we can see all line segments, PDS blocks and the EA module can improve the performance in image inpainting.
5 Conclutions
This paper studies an encoder-decoder MST-net for inpainting. It learns a sketch tensor space restoring edges, lines, and junctions. Specifically, the encoder extracts and refines line and edge structures into a sketch tensor space. The decoder recover the image from it. Moreover, the LSM algorithm is proposed to update the line extractor HAWP to fit the inpainting and object removal tasks. Several effective network modules are proposed to improve the MST for tough man-made scenes inpainting. Extensive experiments validate the efficacy of our MST-net for inpainting.
Acknowledgment Yanwei Fu is the corresponding authour. This project is supported by Huawei, and released on https://ewrfcas.github.io/MST_inpainting.
References
- [1] Connelly Barnes, Eli Shechtman, Adam Finkelstein, and Dan B Goldman. Patchmatch: A randomized correspondence algorithm for structural image editing. ACM Trans. Graph., 28(3):24, 2009.
- [2] Antonio Criminisi, Patrick Perez, and Kentaro Toyama. Object removal by exemplar-based inpainting. In 2003 IEEE Computer Society Conference on Computer Vision and Pattern Recognition, 2003. Proceedings., volume 2, pages II–II. IEEE, 2003.
- [3] Yin Cui, Menglin Jia, Tsung-Yi Lin, Yang Song, and Serge Belongie. Class-balanced loss based on effective number of samples. In Proceedings of the IEEE/CVF Conference on Computer Vision and Pattern Recognition, pages 9268–9277, 2019.
- [4] Patrick Denis, James H Elder, and Francisco J Estrada. Efficient edge-based methods for estimating manhattan frames in urban imagery. In European conference on computer vision, pages 197–210. Springer, 2008.
- [5] Lijun Ding and Ardeshir Goshtasby. On the canny edge detector. Pattern Recognition, 34(3):721–725, 2001.
- [6] Omar Elharrouss, Noor Almaadeed, Somaya Al-Maadeed, and Younes Akbari. Image inpainting: A review. Neural Processing Letters, pages 1–22, 2019.
- [7] Leon A Gatys, Alexander S Ecker, and Matthias Bethge. Image style transfer using convolutional neural networks. In Proceedings of the IEEE conference on computer vision and pattern recognition, pages 2414–2423, 2016.
- [8] Qiang Guo, Shanshan Gao, Xiaofeng Zhang, Yilong Yin, and Caiming Zhang. Patch-based image inpainting via two-stage low rank approximation. IEEE transactions on visualization and computer graphics, 24(6):2023–2036, 2017.
- [9] Peter E Hart and RO Duda. Use of the hough transformation to detect lines and curves in pictures. Communications of the ACM, 15(1):11–15, 1972.
- [10] James Hays and Alexei A Efros. Scene completion using millions of photographs. Communications of the ACM, 51(10):87–94, 2008.
- [11] Martin Heusel, Hubert Ramsauer, Thomas Unterthiner, Bernhard Nessler, and Sepp Hochreiter. Gans trained by a two time-scale update rule converge to a local nash equilibrium. In Advances in neural information processing systems, pages 6626–6637, 2017.
- [12] Bin Jiang Hongyu Liu, Yibing Song, Wei Huang, and Chao Yang. Rethinking image inpainting via a mutual encoder-decoder with feature equalizations. In Proceedings of the European Conference on Computer Vision, 2020.
- [13] Jia-Bin Huang, Sing Bing Kang, Narendra Ahuja, and Johannes Kopf. Image completion using planar structure guidance. ACM Transactions on graphics (TOG), 33(4):1–10, 2014.
- [14] Kun Huang, Yifan Wang, Zihan Zhou, Tianjiao Ding, Shenghua Gao, and Yi Ma. Learning to parse wireframes in images of man-made environments. In Proceedings of the IEEE Conference on Computer Vision and Pattern Recognition, pages 626–635, 2018.
- [15] Youngjoo Jo and Jongyoul Park. Sc-fegan: Face editing generative adversarial network with user’s sketch and color. In Proceedings of the IEEE International Conference on Computer Vision, pages 1745–1753, 2019.
- [16] Justin Johnson, Alexandre Alahi, and Li Fei-Fei. Perceptual losses for real-time style transfer and super-resolution. In European conference on computer vision, pages 694–711. Springer, 2016.
- [17] Avisek Lahiri, Arnav Kumar Jain, Sanskar Agrawal, Pabitra Mitra, and Prabir Kumar Biswas. Prior guided gan based semantic inpainting. In IEEE/CVF Conference on Computer Vision and Pattern Recognition (CVPR), June 2020.
- [18] Cheng-Han Lee, Ziwei Liu, Lingyun Wu, and Ping Luo. Maskgan: Towards diverse and interactive facial image manipulation. In Proceedings of the IEEE/CVF Conference on Computer Vision and Pattern Recognition, pages 5549–5558, 2020.
- [19] Haodong Li, Weiqi Luo, and Jiwu Huang. Localization of diffusion-based inpainting in digital images. IEEE Transactions on Information Forensics and Security, 12(12):3050–3064, 2017.
- [20] Jingyuan Li, Fengxiang He, Lefei Zhang, Bo Du, and Dacheng Tao. Progressive reconstruction of visual structure for image inpainting. In Proceedings of the IEEE International Conference on Computer Vision, pages 5962–5971, 2019.
- [21] Jingyuan Li, Ning Wang, Lefei Zhang, Bo Du, and Dacheng Tao. Recurrent feature reasoning for image inpainting. In Proceedings of the IEEE/CVF Conference on Computer Vision and Pattern Recognition, pages 7760–7768, 2020.
- [22] Liang Liao, Jing Xiao, Zheng Wang, Chia-Wen Lin, and Shin’ichi Satoh. Guidance and evaluation: Semantic-aware image inpainting for mixed scenes. In Computer Vision–ECCV 2020: 16th European Conference, Glasgow, UK, August 23–28, 2020, Proceedings, Part XXVII 16, pages 683–700. Springer, 2020.
- [23] Tsung-Yi Lin, Michael Maire, Serge Belongie, James Hays, Pietro Perona, Deva Ramanan, Piotr Dollár, and C Lawrence Zitnick. Microsoft coco: Common objects in context. In European conference on computer vision, pages 740–755. Springer, 2014.
- [24] Guilin Liu, Fitsum A Reda, Kevin J Shih, Ting-Chun Wang, Andrew Tao, and Bryan Catanzaro. Image inpainting for irregular holes using partial convolutions. In Proceedings of the European Conference on Computer Vision (ECCV), pages 85–100, 2018.
- [25] Hongyu Liu, Bin Jiang, Yi Xiao, and Chao Yang. Coherent semantic attention for image inpainting. In Proceedings of the IEEE International Conference on Computer Vision, pages 4170–4179, 2019.
- [26] Xiaohu Lu, Jian Yao, Kai Li, and Li Li. Cannylines: A parameter-free line segment detector. In 2015 IEEE International Conference on Image Processing (ICIP), pages 507–511. IEEE, 2015.
- [27] Takeru Miyato, Toshiki Kataoka, Masanori Koyama, and Yuichi Yoshida. Spectral normalization for generative adversarial networks. In International Conference on Learning Representations, 2018.
- [28] Kamyar Nazeri, Eric Ng, Tony Joseph, Faisal Qureshi, and Mehran Ebrahimi. Edgeconnect: Structure guided image inpainting using edge prediction. In Proceedings of the IEEE/CVF International Conference on Computer Vision (ICCV) Workshops, Oct 2019.
- [29] Yurui Ren, Xiaoming Yu, Ruonan Zhang, Thomas H Li, Shan Liu, and Ge Li. Structureflow: Image inpainting via structure-aware appearance flow. In Proceedings of the IEEE International Conference on Computer Vision, pages 181–190, 2019.
- [30] Patsorn Sangkloy, Jingwan Lu, Chen Fang, Fisher Yu, and James Hays. Scribbler: Controlling deep image synthesis with sketch and color. In Proceedings of the IEEE Conference on Computer Vision and Pattern Recognition, pages 5400–5409, 2017.
- [31] Zhuoran Shen, Mingyuan Zhang, Haiyu Zhao, Shuai Yi, and Hongsheng Li. Efficient attention: Attention with linear complexities. In Proceedings of the IEEE/CVF Winter Conference on Applications of Computer Vision, pages 3531–3539, 2021.
- [32] Yuhang Song, Chao Yang, Yeji Shen, Peng Wang, Qin Huang, and C-C Jay Kuo. Spg-net: Segmentation prediction and guidance network for image inpainting. arXiv preprint arXiv:1805.03356, 2018.
- [33] Ashish Vaswani, Noam Shazeer, Niki Parmar, Jakob Uszkoreit, Llion Jones, Aidan N Gomez, Łukasz Kaiser, and Illia Polosukhin. Attention is all you need. In Advances in neural information processing systems, pages 5998–6008, 2017.
- [34] Rafael Grompone Von Gioi, Jeremie Jakubowicz, Jean-Michel Morel, and Gregory Randall. Lsd: A fast line segment detector with a false detection control. IEEE transactions on pattern analysis and machine intelligence, 32(4):722–732, 2008.
- [35] Ting-Chun Wang, Ming-Yu Liu, Jun-Yan Zhu, Andrew Tao, Jan Kautz, and Bryan Catanzaro. High-resolution image synthesis and semantic manipulation with conditional gans. In Proceedings of the IEEE conference on computer vision and pattern recognition, pages 8798–8807, 2018.
- [36] Zhou Wang, Alan C Bovik, Hamid R Sheikh, and Eero P Simoncelli. Image quality assessment: from error visibility to structural similarity. IEEE transactions on image processing, 13(4):600–612, 2004.
- [37] Wei Xiong, Jiahui Yu, Zhe Lin, Jimei Yang, Xin Lu, Connelly Barnes, and Jiebo Luo. Foreground-aware image inpainting. In Proceedings of the IEEE conference on computer vision and pattern recognition, pages 5840–5848, 2019.
- [38] Nan Xue, Tianfu Wu, Song Bai, Fudong Wang, Gui-Song Xia, Liangpei Zhang, and Philip HS Torr. Holistically-attracted wireframe parsing. In Proceedings of the IEEE/CVF Conference on Computer Vision and Pattern Recognition, pages 2788–2797, 2020.
- [39] Jie Yang, Zhiquan Qi, and Yong Shi. Learning to incorporate structure knowledge for image inpainting. In AAAI, pages 12605–12612, 2020.
- [40] Zili Yi, Qiang Tang, Shekoofeh Azizi, Daesik Jang, and Zhan Xu. Contextual residual aggregation for ultra high-resolution image inpainting. In IEEE/CVF Conference on Computer Vision and Pattern Recognition (CVPR), June 2020.
- [41] Fisher Yu and Vladlen Koltun. Multi-scale context aggregation by dilated convolutions. arXiv preprint arXiv:1511.07122, 2015.
- [42] Jiahui Yu, Zhe Lin, Jimei Yang, Xiaohui Shen, Xin Lu, and Thomas S Huang. Generative image inpainting with contextual attention. In Proceedings of the IEEE conference on computer vision and pattern recognition, pages 5505–5514, 2018.
- [43] Jiahui Yu, Zhe Lin, Jimei Yang, Xiaohui Shen, Xin Lu, and Thomas S Huang. Free-form image inpainting with gated convolution. In Proceedings of the IEEE International Conference on Computer Vision, pages 4471–4480, 2019.
- [44] Yanhong Zeng, Jianlong Fu, Hongyang Chao, and Baining Guo. Learning pyramid-context encoder network for high-quality image inpainting. In Proceedings of the IEEE conference on computer vision and pattern recognition, pages 1486–1494, 2019.
- [45] Yu Zeng, Zhe Lin, Jimei Yang, Jianming Zhang, Eli Shechtman, and Huchuan Lu. High-resolution image inpainting with iterative confidence feedback and guided upsampling. In European Conference on Computer Vision, pages 1–17. Springer, 2020.
- [46] Richard Zhang, Phillip Isola, Alexei A Efros, Eli Shechtman, and Oliver Wang. The unreasonable effectiveness of deep features as a perceptual metric. In Proceedings of the IEEE conference on computer vision and pattern recognition, pages 586–595, 2018.
- [47] Richard Zhang, Jun-Yan Zhu, Phillip Isola, Xinyang Geng, Angela S Lin, Tianhe Yu, and Alexei A Efros. Real-time user-guided image colorization with learned deep priors. ACM Transactions on Graphics (TOG), 9(4), 2017.
- [48] Bolei Zhou, Agata Lapedriza, Aditya Khosla, Aude Oliva, and Antonio Torralba. Places: A 10 million image database for scene recognition. IEEE transactions on pattern analysis and machine intelligence, 40(6):1452–1464, 2017.
- [49] Yichao Zhou, Haozhi Qi, and Yi Ma. End-to-end wireframe parsing. In Proceedings of the IEEE International Conference on Computer Vision, pages 962–971, 2019.
6 Appendix
6.1 Network Architectures
The network architectures are illustrated as follows.
Partially Gated Convolution (GC) Block. Both encoder and decoder contain two groups of partially GC blocks for the input (3 blocks) and output (2 blocks) features, and the GC is consist of GateConv2D [43] InstanceNorm ReLU. We also add the spectral normalization [27] to the GC used in the generator.
Dilated Residual Block. The dilated residual blocks are the same as the ones used in EdgeConnect [28] with Conv2D InstanceNorm ReLU Conv2D InstanceNorm, where the first convolution is based on .
Efficient Attention. The input feature of the Efficient Attention is channels, and we use the multi-head attention with . So, the dimension of each group is .
6.2 Details of the Experiments
6.2.1 Preprocessing
For our method, the input images are resized to 256256 at first. Then they are normalized to , and values of the masked regions are set to 1. For the line detection, we set the thresholds of LSM-HAWP for unmasked and masked with (0.95, 0.925) in ShanghaiTech [14], and (0.85, 0.8) in Places2 [48] and York Urban [4].
6.2.2 Places2 Dataset Selection
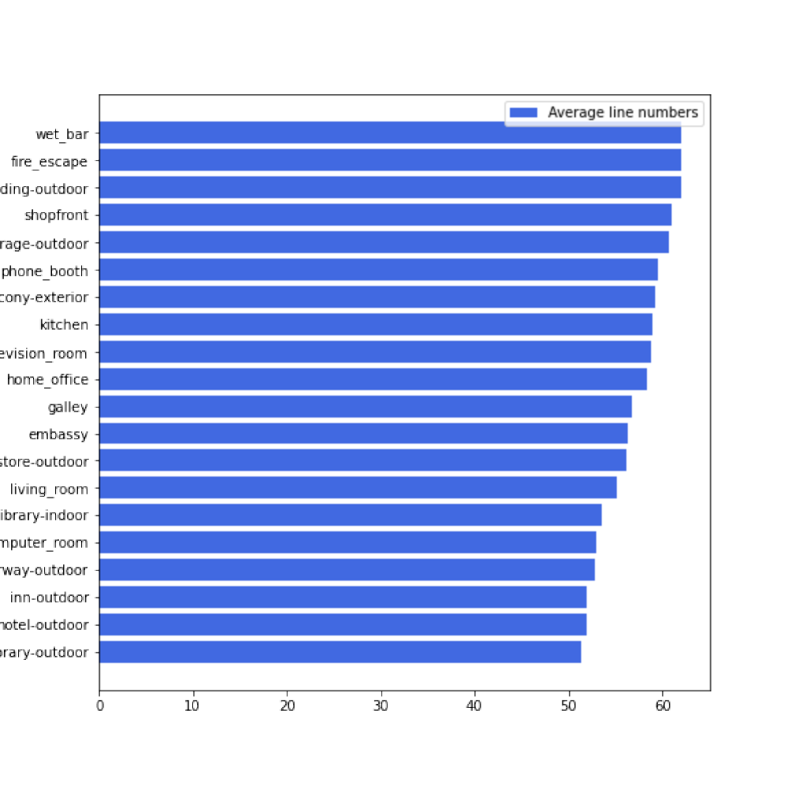
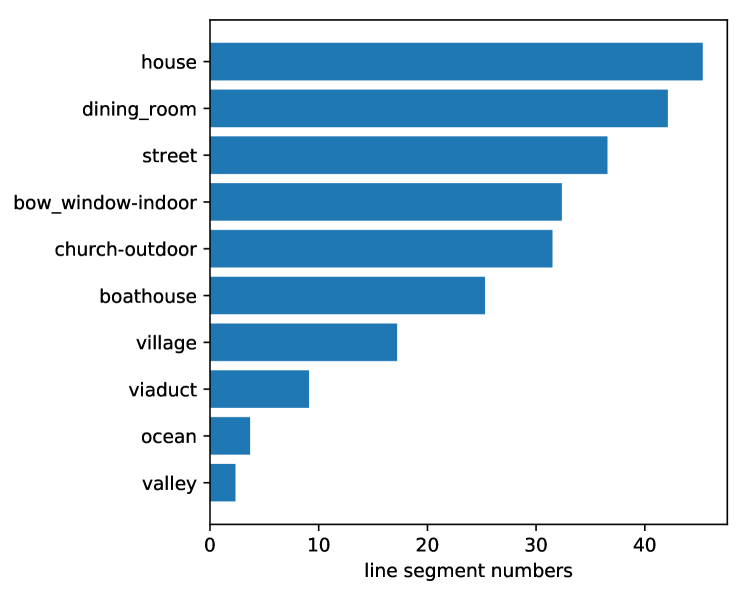
Places2 dataset [48] is consisted of 10 million+ images from 365 various scenes. Since the proposed method is devoted to reconstructing the images with man-made structures, we need to select some representative scenes whose images contain enough structure information for man-made Places2 (P2M). Therefore, we leverage the retrained LSM-HAWP to predict the number of extracted line segmentations with scores larger than 0.925 in the validation of Places2. The scenes with top20 average line segment numbers are shown in Fig. 9. For the generalization, we randomly select 10 of them which is consist of ‘balcony-exterior’, ‘computer-room’, ‘embassy’, ‘galley’, ‘general-store-outdoor’, ‘home-office’, ‘kitchen’, ‘library-outdoor’, ‘parking-garage-outdoor’, and ‘shopfront’. And there are 47949 training images and 1000 test images at all, which include buildings, indoor scenes, and outdoor scenes with various structures.
Besides, we also provide the results of comprehensive Places2 (P2C) in the main paper, which is consist of 10 randomly selected scenes: ‘valley’, ‘church-outdoor’, ‘village’, ‘house’, ‘dining-room’, ‘street’, ‘ocean’, ‘bow-window-indoor’, ‘boathouse’, and ‘viaduct’. And the related line segments are shown in Fig. 10.
6.2.3 Implements of Compared Methods
All of the compared methods are based on the official implements with the new mask strategy mentioned in the paper. The hyper-parameter settings and learning rate settings refer to the official settings too. Besides, other settings are listed as follows. Note that code addresses listed in the footnote are the official implements of other compared methods.
Gated Convolution (GC) [43]333https://github.com/JiahuiYu/generative_inpainting GC is trained with 1500 epochs (about 468k steps) for ShanghaiTech, and 350 epochs (1049k steps) for Places2 with batch size 16.
Edge Connect (EC) [28]444https://github.com/knazeri/edge-connect EC is trained with 400k (150k for the edge refinement network) steps for ShanghaiTech, and 1000k (300k steps for the edge refinement network) for Places2 with batch size 16.
Recurrent Feature Reasoning (RFR) [21]555https://github.com/jingyuanli001/RFR-Inpainting RFR is trained with 400k (150k for finetune) steps for ShanghaiTech, and 1200k (200k for finetune) for Places2 with batch size 6.
Mutual Encoder Decoder with Feature Equalizations (MED) [12]666https://github.com/KumapowerLIU/Rethinking-Inpainting-MEDFE Note that the official implement of MED only supports training with batch=1 due to its special CSA attention design [25]. So, the training is very inefficient and it takes us more than three weeks to train the MED on Places2. However, MED still works badly on Places2, which dues to the instable training process with batch=1 in our opinions. As the result, MED is trained with 200 epochs (1000k steps) for ShanghaiTech, and 60 epochs (about 2877k steps) for Places2 with batch size 1.
6.3 Supplementary Experimental Results
6.3.1 Visualization of Gated Convolutions
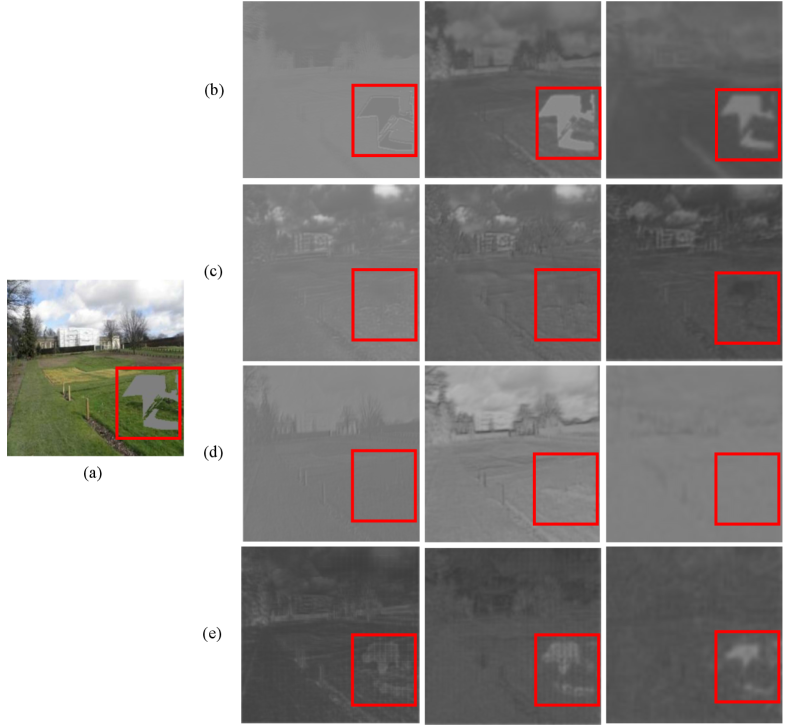
We show the visualization results of the origin GC in Fig. 11, which are collected by averaging the outputs from different GateConvs. for the masked regions are active in Fig. 11(b) and Fig. 11(e), which are the encoder of the coarse network and the decoder of the refinement network. And they can also be seen as the encoder and decoder of the whole GC pipeline model.
6.3.2 Efficiency Comparisons of Efficient Attention
Attention | Batchsize | Image/sec |
---|---|---|
CA | 16 | 19.42 |
EA | 16 | 36.37 |
CA | 32 | 20.70 |
EA | 32 | 40.81 |
In this section, we pay attention to compare the efficiency of Contextual Attention (CA) [42] and Efficient Attention (EA) [31]. All comparisons are based on our structure refinement network, and only one attention layer is added to the middle of the residual blocks with the resolution 6464. Then, we train the model with two attention strategies and batch sizes on the ShanghaiTech dataset for one epoch. The results are shown in Tab. 6. Since CA can only be implemented without parallel computing for the batch, we just compare the training speed with images per second between CA and EA. From Tab. 6, EA can be trained more efficiently than CA. Moreover, benefited from the parallelism, EA enjoys the speedup by enlarging the training batch size.
6.3.3 Ablation Study with Only Edge and PSS
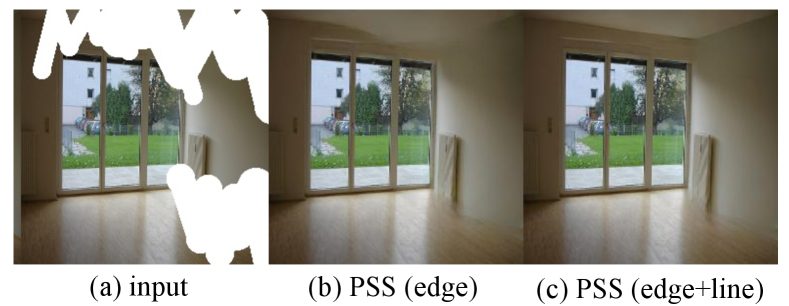
PSNR | SSIM | FID | |
---|---|---|---|
PSS (edge) | 26.78 | 0.875 | 21.40 |
PSS (edge+line) | 26.90 | 0.876 | 21.16 |
6.3.4 Comparisons with ProFill

6.3.5 Additional Visual Results
In this section we provide more experiment results for image inpainting and object removal on different datasets. Furthermore, we provided more comparisons in P2C compared with EC, which show the superior performance of the proposed sketch tensor space reconstructing.
6.3.6 Object Removal Video
We also provide a video that displays the object removal in ShanghaiTech, P2M, and P2C. The video is played in a triple-speed to compress the storage size. This video shows the good generalization and the fast interactive experience of the proposed method in the GPU environment.
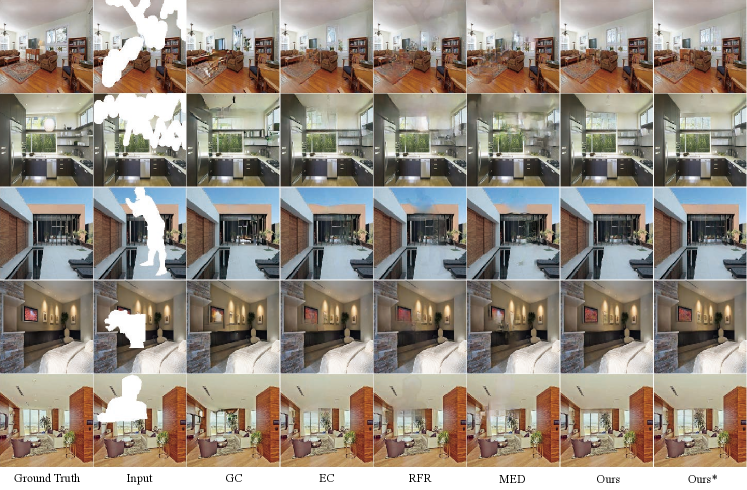
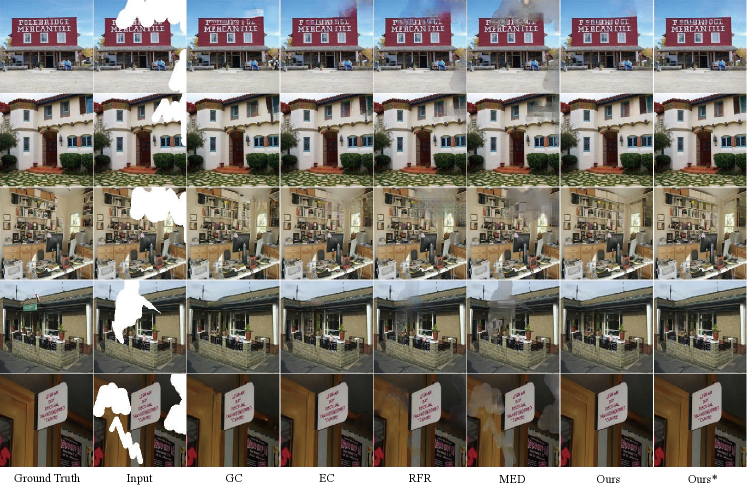
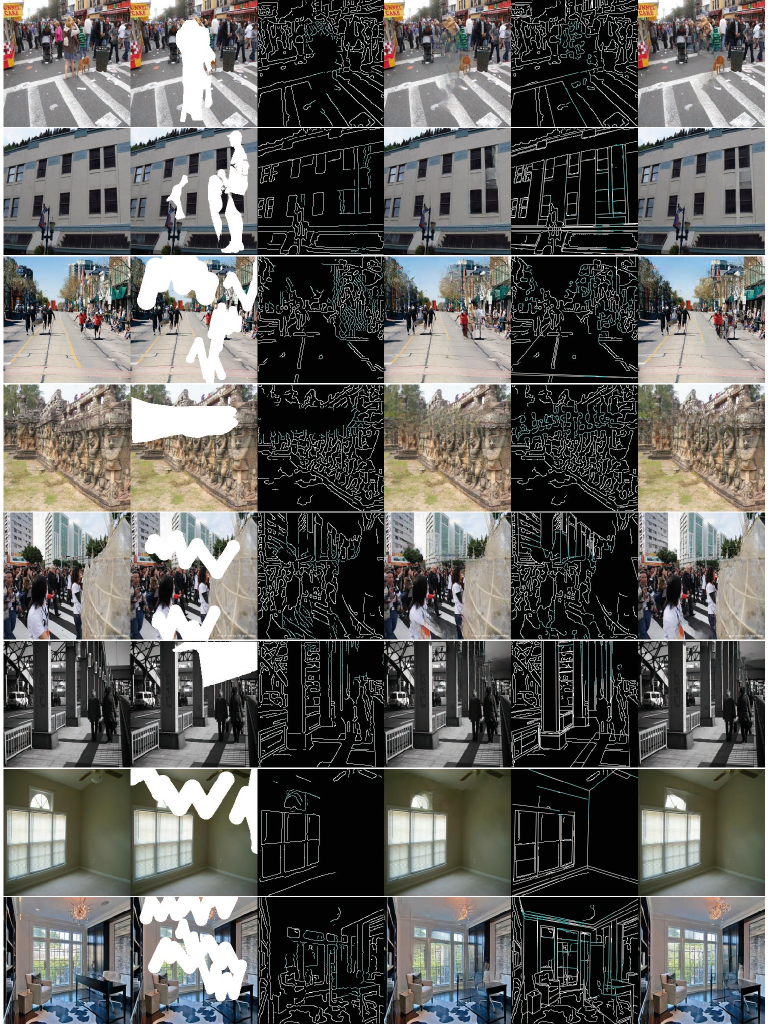
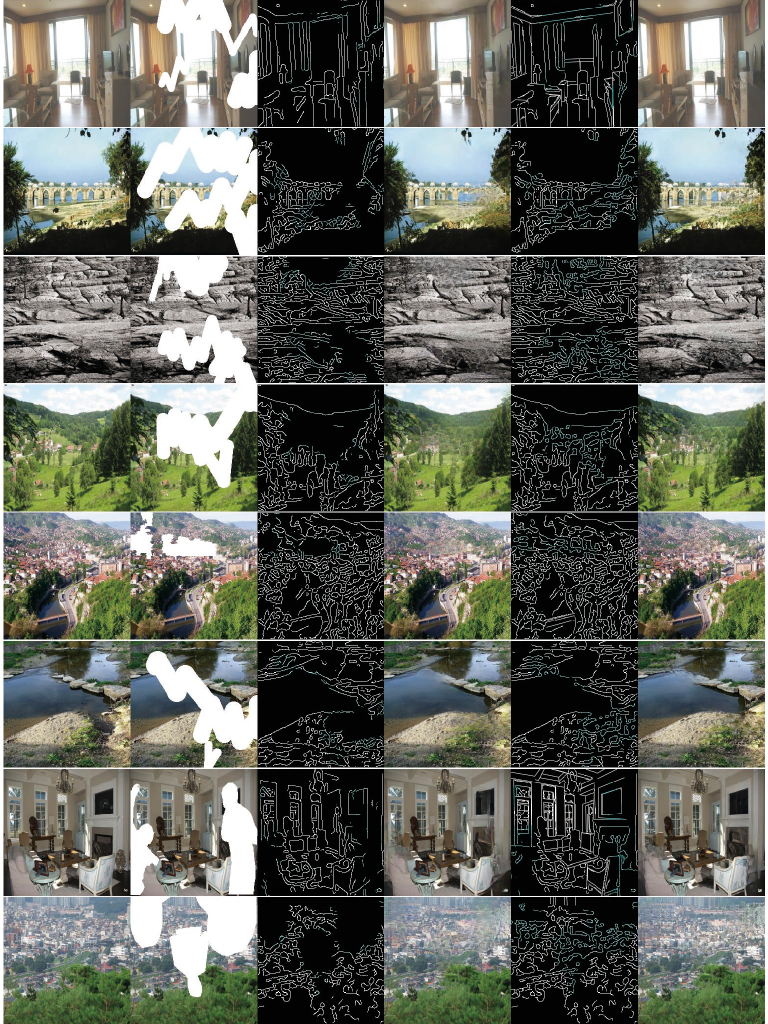
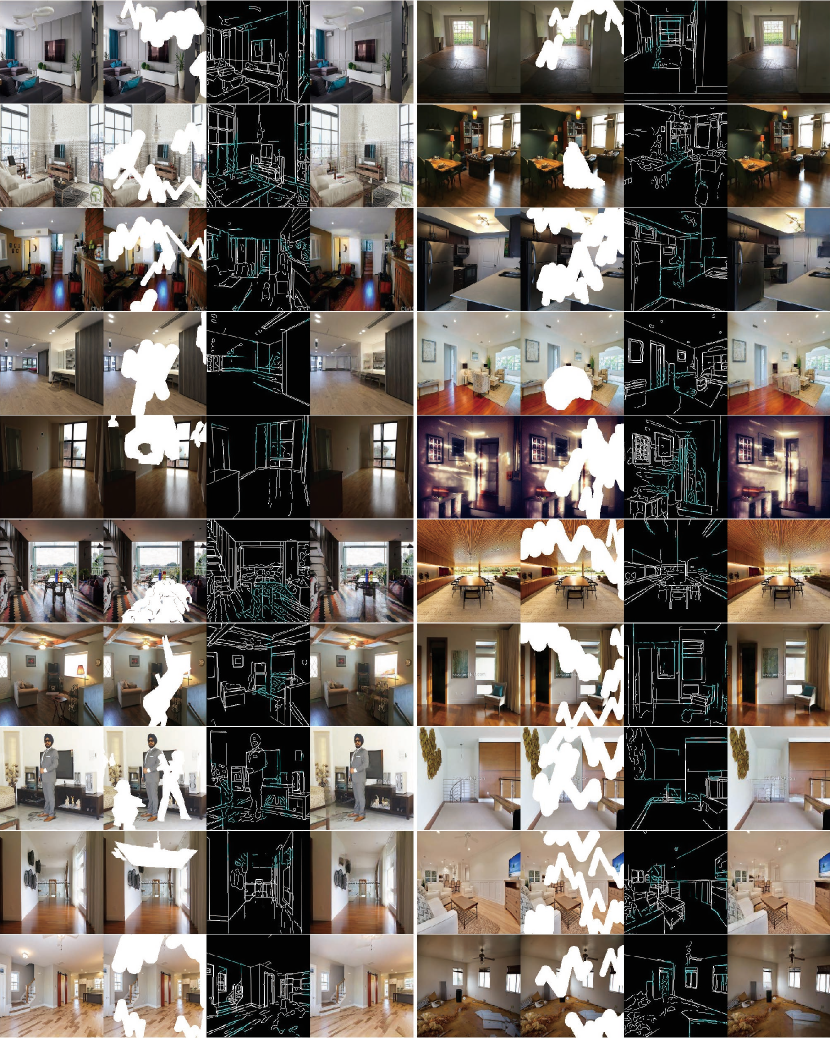
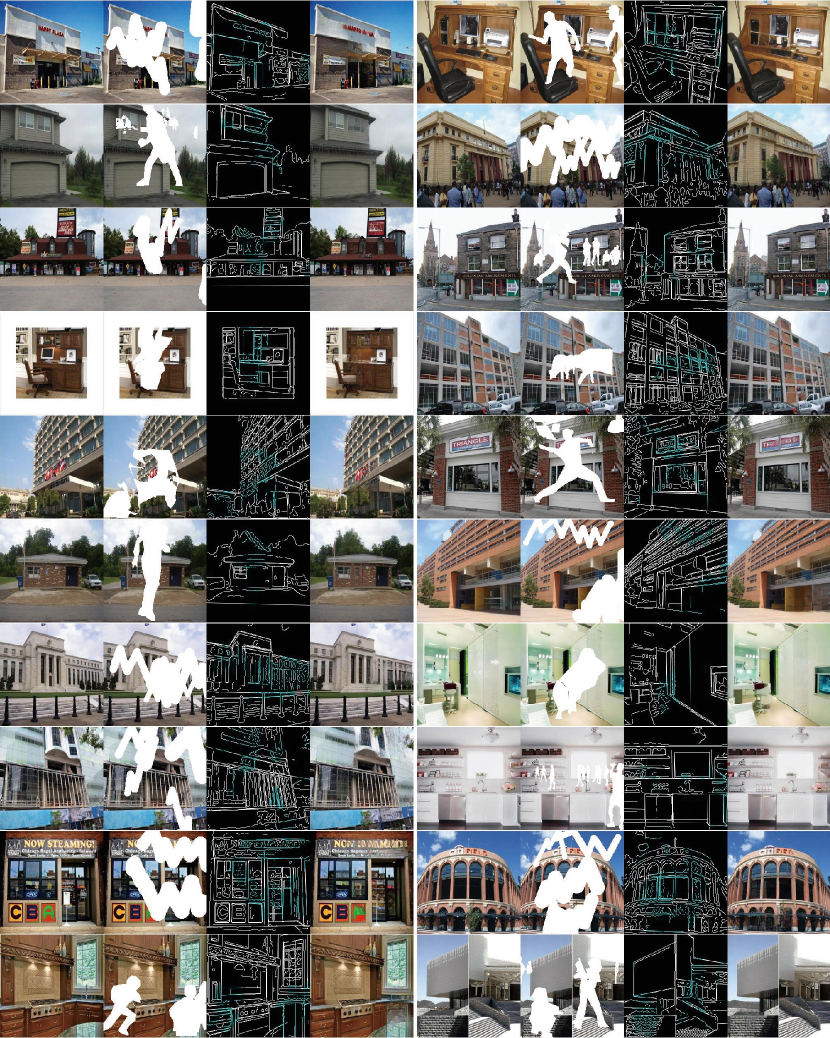
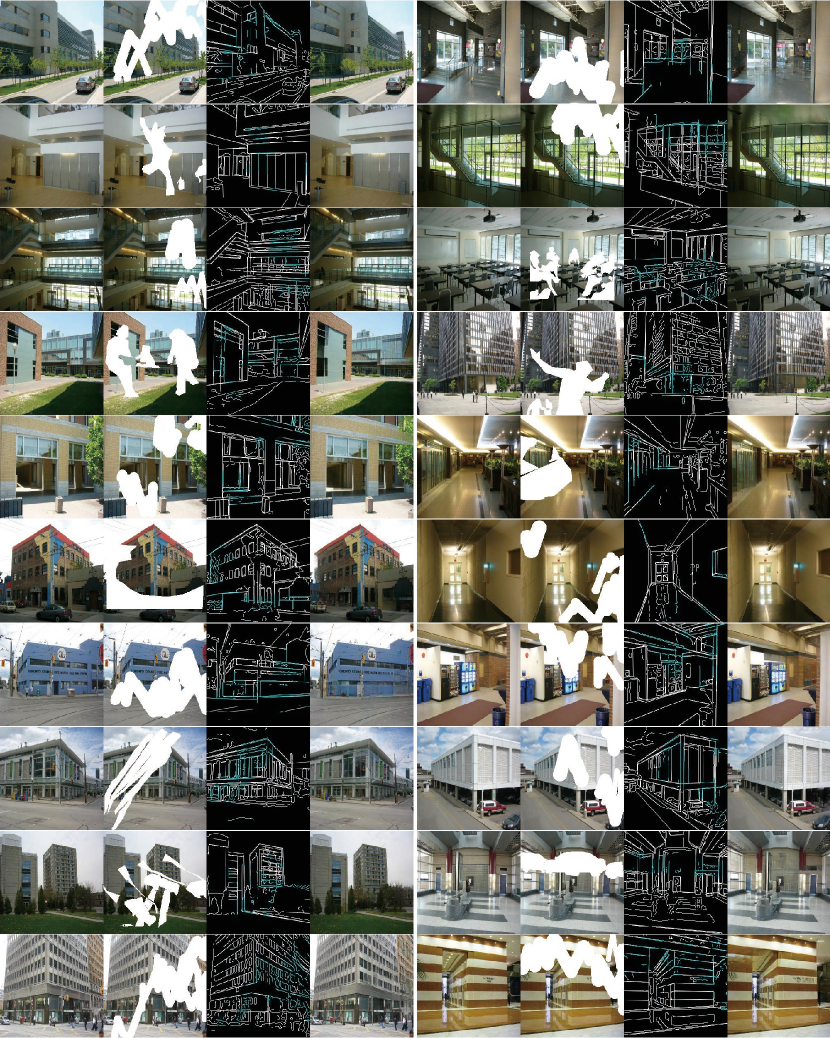
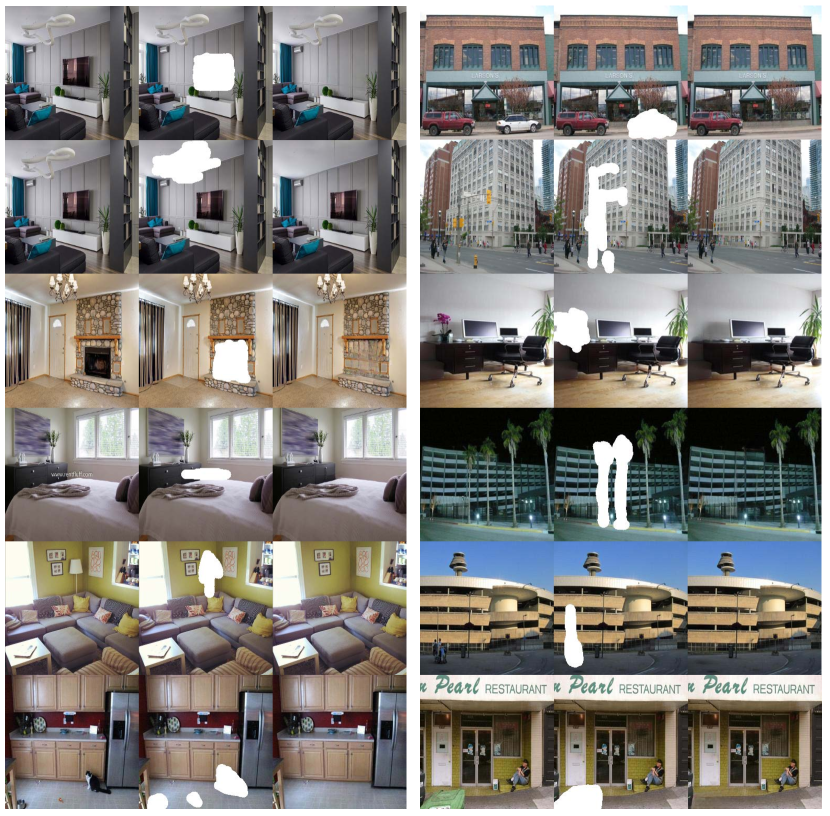