Largest eigenvalue of the configuration model
and breaking of ensemble equivalence
Abstract.
We analyse the largest eigenvalue of the adjacency matrix of the configuration model with large degrees, where the latter are treated as hard constraints. In particular, we compute the expectation of the largest eigenvalue for degrees that diverge as the number of vertices tends to infinity, uniformly on a scale between and , and show that a weak law of large numbers holds. We compare with what was derived in our earlier paper [14] for the Chung-Lu model, which in the regime considered represents the corresponding configuration model with soft constraints, and show that the expectation is shifted down by asymptotically. This shift is a signature of breaking of ensemble equivalence between the hard and soft (also known as micro-canonical and canonical) versions of the configuration model. The latter result generalizes a previous finding [13] obtained in the case when all degrees are equal.
Key words and phrases:
Constrained random graphs; micro-canonical and canonical ensembles; adjacency matrix; largest eigenvalue; breaking of ensemble equivalence.2000 Mathematics Subject Classification:
60B20,60C05, 60K351. Introduction and main results
Motivation.
Spectral properties of adjacency matrices in random graphs play a crucial role in various areas of network science. The largest eigenvalue is particularly sensitive to the graph architecture, making it a key focus. In this paper we focus on a random graph with a hard constraint on the degrees of nodes. In the homogeneous case (all degrees equal to ), it reduces to a random -regular graph. In the heterogeneous case (different degrees), it is known as the configuration model. Our interest is characterizing the expected largest eigenvalue of the configuration model and comparing it with the same quantity for a corresponding random graph model where the degrees are treated as soft constraints.
The set of -regular graphs on vertices, with , is non-empty when and is even. Selecting a graph uniformly at random from this set results in what is known as the random -regular graph, denoted by . The spectral properties of are well-studied for (for , the graph is trivially a set of disconnected edges). For instance, all eigenvalues of its adjacency matrix fall in the interval , with the largest eigenvalue being . The computation of , where and are the second-largest and the smallest eigenvalue, respectively, has been challenging. It is well known that for fixed the empirical distribution function of the eigenvalues of converges to the so-called Kesten-McKay law [22], the density of which is given by
A lower bound of the type was expected from this, and was indeed proven in the celebrated Alon-Boppana theorem in [1], stating that , where the constant in the error term only depends on . In the same paper an upper bound of the type was conjectured . This conjecture was later proven in the pioneering work [17] (for a shorter proof, see [6]). When , the spectral distribution converges to the semi-circular law [26]. It was proven in [10] that with high probability when . This result was extended in [3] to . For recent results and open problems, we refer the reader to [30].
There has not been much work on the inhomogeneous setting where not all the degrees of the graph are the same. The natural extension of the regular random graph is the configuration model, where a degree sequence is prescribed. Unless otherwise specified, we assume the degrees to be hard constraints, i.e. realized exactly on each configuration of the model (this is sometimes called the ‘microcanonical’ configuration model, as opposed to the ‘canonical’ one where the degrees are soft constraints realized only as ensemble averages [24, 20]). The empirical spectral distribution of the configuration model is known under some assumptions on the growth of the sum of the degrees. When , the graph locally looks like a tree. It has been shown that the empirical spectral distribution exists and that the limiting measure is a functional of a size-biased Galton-Watson tree [7]. When grows polynomially with , the local geometry is no longer a tree. This case was recently studied in [12], where it is shown that the appropriately scaled empirical spectral distribution of the configuration model converges to the free multiplicative convolution of the semi-circle law with a measure that depends on the empirical degree sequence. The scaling of the largest and the second-largest eigenvalues has not yet been studied in full generality.
For the configuration model the behaviour of the largest eigenvalue is non-trivial. In the present paper, we consider the configuration model with large degrees, compute the expectation of the largest eigenvalue of its adjacency matrix, and prove a weak law of large numbers. When , the empirical distribution of (appropriately scaled) converges to the semi-circle law. Also, if we look at Erdős-Rényi random graphs on vertices with connection probability , then the appropriately scaled empirical distribution converges to the semi-circle law. It is known that the latter two random graphs exhibit different behaviour for the expected largest eigenvalue [13]. This can be understood in the broader perspective of breaking ensemble equivalence [24, 20], which we discuss later. In the inhomogeneous setting, the natural graph to compare the configuration model with is the Chung-Lu model. For the case where the sum of the degrees grows with , it was shown in [11] that the empirical spectral distribution converges to the free multiplicative product of the semi-circle law with a measure that depends on the degree sequence, similar to [12]. In [14], we investigated the largest eigenvalue and its expectation for the Chung-Lu model (and even derived a central limit theorem). In the present paper we complete the picture by showing that, when the degrees are large, there is a gap between the expected largest eigenvalue of the Chung-Lu model and the configuration model. We refer the reader to the references listed in [13, 14] for more background.
Main theorem.
For , let be the random graph on vertices generated according to the configuration model with degree sequence [27, Chapter 7.2]. Define
Throughout the paper we need the following assumptions on as .
Assumption 1.1.
(D1) Bounded inhomogeneity: .
(D2) Connectivity and sparsity: .
Under these assumptions, is with high probability non-simple [27, Chapter 7.4]. We write and to denote probability and expectation with respect to the law of conditional on being simple, suppressing the dependence on the underlying parameters.
Let be the adjacency matrix of . Let be the eigenvalues of , ordered such that . We are interested in the behaviour of as . Our main theorem reads as follows.
Theorem 1.2.
In [14] we looked at an alternative version of the configuration model, called the Chung-Lu model , where the average degrees, rather than the degrees themselves, are fixed at . This is an ensemble with soft constraints; in the considered regime for the degrees, it coincides with a maximum-entropy ensemble, also called ‘canonical’ configuration model [24]. For this model we showed that, subject to Assumption 1.1,
(1.2) |
and in -probability, where and denote expectation with respect to the law of and is the largest eigenvalue of the . The notable difference between (1.1) and (1.2) is the shift by .
For the special case where all the degrees are equal to , we have and , and so and . In fact, . Since in this model the degrees can fluctuate with the same law (soft constraint in the physics literature), this case reduces to the Erdős-Rényi random graph for which results on were already well known in [21, 19] and further analyzed in [15].
Breaking of ensemble equivalence.
The shift by was proven in [13] for the homogeneous case with equal degrees and is a spectral signature of breaking of ensemble equivalence [24]. Indeed, a -regular graph is the ‘micro-canonical’ version of a random graph where all degrees are equal and ‘hard’, and the Erdős-Rényi random graph is the corresponding ‘canonical’ version where all degrees are equal and ‘soft’. More in general, is the micro-canonical configuration model where the constraint on the degrees is ‘hard’, while is the canonical version where the constraint is ‘soft’. We refer the reader to [20] for the precise definition of these two configuration model ensembles and for the proof that they are not asymptotically equivalent in the measure sense [25]. This means that the relative entropy per node of with respect to has a strictly positive limit as . This shows that the choice of constraint matters, not only on a microscopic scale but also on a macroscopic scale. Indeed, for non-equivalent ensembles one expects the existence of certain macroscopic properties that have different expectation values in the two ensembles (macrostate (in)equivalence [25]). The fact that the largest eigenvalue picks up this discrepancy is interesting. What is remarkable is that the shift by , under the hypotheses considered, holds true also in the case of heterogeneous degrees and remains the same irrespective of the scale of the degrees and of the distribution of the degrees on this scale.
Outline.
The remainder of this paper is organised as follows. In Section 2 we look at the issue of simplicity of the graph. In Section 3 we bound the spectral norm of the matrix
(1.3) |
We use the proof of [10] to show that with high probability. Using the latter we show that
with high probability. In Section 4 we use the estimates in Section 3 to prove Theorem 1.2.
2. Configuration model and simple graphs with a given degree sequence
In the context of random graphs with hard constraints on the degrees, we work with both the configuration model (a random multi-graph with a prescribed degree sequence) and with the conditional configuration model (a random simple graph with a prescribed degree sequence). We view the second as a special case of the first.
Configuration model.
The configuration model with degree sequence , , generates a graph through a perfect matching of the set of half-edges , i.e., such that is an isomorphism and an involution, and for all .
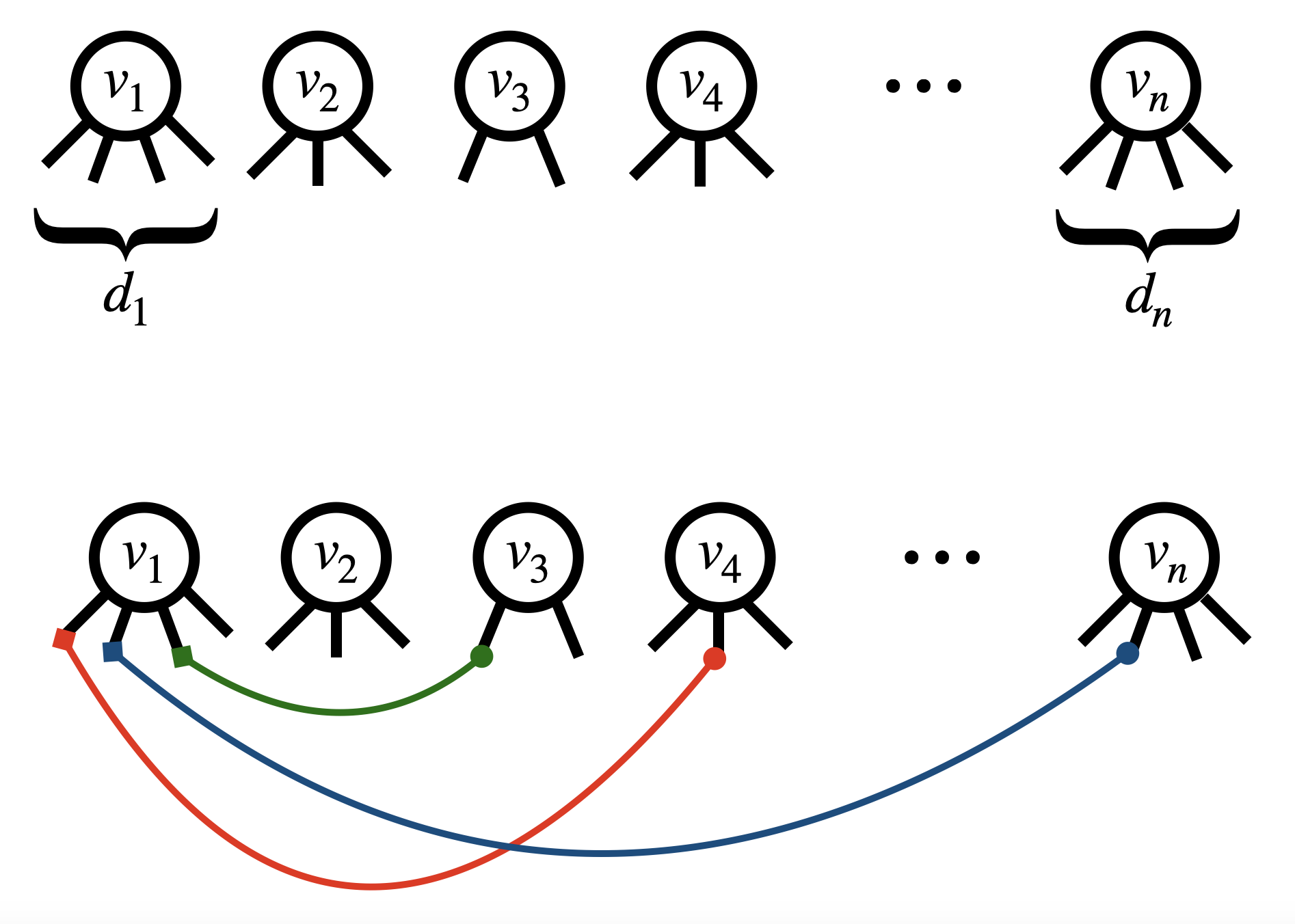
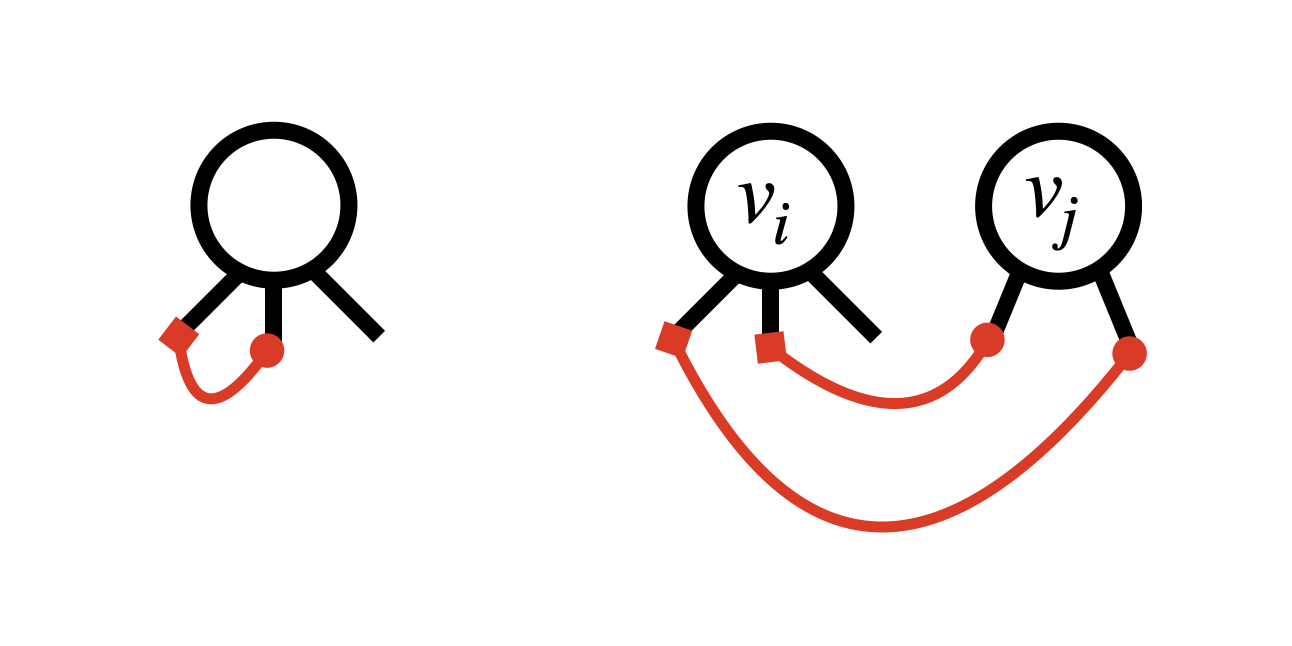
It turns out that a pairing scheme, such as matching half-edges from left to right or selecting pairs uniformly at random, is considered admissible as long as it yields the correct probability for a perfect matching (see, for example, [27, Lemma 7.6]). An important property of each admissible pairing scheme is that
(2.1) |
We can therefore endow with the lexicographic order. An element , , is associated with a vertex through the first component of . An edge is an element of given by the pairing . We define the configuration as the set of all such . Given the lexicographic order, we may assume that and identify arbitrarily the head and the tail of the edge: , . We can then order the edges in their order of appearance via , forming a list . These properties of the configuration model will be used in Subsection 3.1. For the configuration model it is easy to check that111We adopt the convention that a self-loop contributes to the degree, i.e., is twice the number of self-loops attached to vertex . This convention is useful because it yields .
(2.2) |
Indeed every matrix element , can be expressed as
where is the indicator function of the event . Taking expectations and using (2.1), we get 2.2.
Simple graphs with a given degree sequence.
A linked but different model is the one that samples uniformly at random a simple graph with a given degree sequence , 222With a little abuse of notation we permit our simple graphs to have self-loops. We maintain the convention explained in the previous footnote.. An immediate question is whether there is any relation between and . It turns out that the following is true (see [27] for reference):
Lemma 2.1.
Let be a graph generated via the configuration model with degree sequence . Then
(2.3) |
and
(2.4) |
Thus, we can identify the law of the uniform random graph with a given degree sequence with the law of the configuration model conditional on simplicity, i.e.,
It follows that the expectation under the uniform random graph with a given degree sequence can be expressed as a the expectation of the configuration model conditional on simplicity. As explained in [28, Remark 1.14], we have
(2.5) |
3. Bound on
In this section we derive the following bound on the spectral norm of the matrix defined in (1.3). This bound will play a crucial role in the proof of Theorem 1.2 in Section 4.
Theorem 3.1.
For every , there exists a constant such that
where the constant depends on and only.
The proof is given in Section 3.1 and we follow the proof of Lemma 16 of [10]. In Sections 3.2–3.3 we derive some estimates that are needed in Section 3.1.
We have
Recall (1.3). In Lemma 3.3 below we will see that is asymptotically rank (more precisely, the other eigenvalues are of order . This means that , . Therefore, by Weyl’s inequality, it easy to see that and are bounded above and below by and with error , while is in a window of size around . We therefore have the following Corollary, which will be needed in Section 4:
Corollary 3.2.
For every ,
3.1. Spectral estimates
Notation.
Abbreviate . Let be uniformly distributed on , let be the degree of a vertex that is picked uniformly at random, and let
be the average of the empirical degree distribution. Under Assumption 1.1, and . We define the normalised degree sequence as
(3.1) |
and the normalised adjacency matrix as
In the following we will need multiples of the vector
(3.2) |
which is the eigenvector corresponding to the rank- approximation of , as it easy to check using (2.2).
Two lemmas.
The matrix is asymptotically rank 1:
Lemma 3.3.
Define
and
Then
Proof.
It is shown in [12] that the empirical spectral distribution of has a deterministic limit given by , where is the standard Wigner semicircle law, is the distribution of , and is the free product defined in [29, 4, 2].
Lemma 3.4.
Let be the normalised degree sequence, and suppose that Assumption 1.1 holds and
(3.3) |
Then is compactly supported.
Key estimates.
From [12, Theorem 1] we know that, under Assumption 1.1, the empirical spectral distribution of , written
converges weakly to . The fact that and are compactly supported implies that is compactly supported too. However, this still allows that has outliers, which possibly control . It is hard to analyse for sparse graphs, because it is related to expansion properties of the graph, mixing times of random walks on the graph, and more. To prove that , we will use the argument described in [10], which is an adaptation of the argument in [18]. We are only after the order of , not sharp estimates.
For random regular graphs with fixed degree, the problem of computing was solved in [16]. We are not aware of any proof concerning the second largest eigenvalue of configuration models with bounded degrees, but any sharp bound must come from techniques of the type employed in [16, 5]. In what follows we prove Theorem 3.1 based on [18] and its adaptation to the inhomogeneous setting in [10]. In the following, we present the proof as outlined in the latter paper, adapting it to our case to make our paper self-contained. This adjustment is necessary because the result we need is somewhat obscured in the context in which it appears in [10].
Let . The transition kernel of a random walk on the graph is given by . The random matrix has as principal normalised eigenvector with eigenvalue 1. Define
Note that the matrices and have the same spectrum by a similarity transformation. Hence we can write the Rayleigh formula
where . Define . Let be the second largest eigenvalue of . Then
Since
putting we see that
(3.4) |
which gives a bound of the type . Note that the matrix elements of can be expressed as
(3.5) |
where counts the number of edges between vertices and , with the convention for the diagonal elements stated earlier. In view of (3.4), in order to obtain a bound on we must focus on . In fact, we must show that
(3.6) |
in order to obtain the desired bound . To achieve this we proceed in steps:
-
–
We reduce the computation of to the analysis of two terms.
-
–
In (3.7) below we identify the leading order of from these two terms, which turns out to be .
-
–
We show that the other terms are of higher order and therefore are negligible.
-
–
We prove concentration around the mean through concentration of the leading order term in Lemma 3.8 below.
-
–
Recalling the denominator of (3.5), we get a bound on after multiplying by .
To prove (3.6), we reduce the problem to a maximisation problem in a simpler space. Namely, let , and
Then, using the formula for the volume of the -dimensional ball, we have
The maximisation over can be reduced to over and this leads to an error that depends on .
Lemma 3.5.
Let
Then
Proof.
Let be . We want to show that for every there is a vector such that and . Let us write the components of as
where and is an error term. Because , we choose such that , where is a non-negative integer smaller than . We relabel the indices in such a way that when . Now consider the vector given by
It follows that and , and therefore . Furthermore, because by construction, we have the required property . Iterating the previous argument, we can express every vector in terms of a sequence of vectors in such that
Therefore, because (3.4) is maximized on , we have
from which the claim follows. ∎
Our next goal is to show that for all with a suitably high probability. This can be done in the following way. Fix and define the random variable
Define the set of indices
Then we can rewrite with
In Section 3.2 we show that is of the correct order and that is well concentrated around its mean. In Section 3.3 we analyse , which is of a different nature and requires that we exclude subgraphs in the configuration model that are too dense.
3.2. Estimate of first contribution
1. Use (2.2) and (3.5) to write out . This gives
In view of the bound on , the last term gives a contribution
Since , we have that and , and therefore . Hence
where we can bound the right-hand side as
We can therefore conclude that
(3.7) |
2. To prove that is concentrated around its mean, we use an argument originally developed in [9, 23] and used for the configuration model in [18, 10]. This argument employs the martingale structure of the configuration model conditional on partial pairings. Define
We can then express as
We divide the set of pairings into three sets
and write
We can do a similar decomposition for .
3. The following martingale lemma from [18, 10] is the core estimate that we want to apply to each of the ’s.
Lemma 3.6.
Let be and two graphs generated via the configuration model through perfect matchings and . Let be the edges of and be the edges of , ordered as above. Define an equivalence relation on the probability space by setting when , i.e., the first pairings match. Let be the set of equivalence classes, and the corresponding -algebra with . Consider a bounded measurable , and set . Note that
-
is a Doob martingale with
and , .
Define , and suppose that there exist functions such that
Then, for all and ,
(3.8) |
Remark 3.7.
The condition on the existence can be rephrased as the existence of a random variable that stochastically dominates on (see [18]).
Lemma 3.8.
[10, Lemma 15] There exist constants , , depending only on the ratio , such that
(3.9) |
Proof.
While the properties in allow us to apply standard martingale arguments to capture (see below), the properties in and force us to use Lemma 3.6 to capture and .
i. From the definition of and it follows that a bound on implies by symmetry a bound on (with, possibly, different constants). We will therefore focus on , the result for carrying through trivially. Without loss of generality we may reorder the indices in such a way that and for each use the lexicographic order and (i.e., we perform the pairing sequentially from left to right; see [27, Lemma 7.6]). It follows that and . Define
For each in the configuration we have
with .
ii. Next, take , with and . Then is a Doob martingale and, by the definition of the configuration model, we can write as
Now that we have an expression for , we can use the method of switching (see, for example, [31]). Indeed, given a , we can define a quantity as follows. Given the first pairings of , let be the set of points already paired, and let be the -th pair. Put and . Then is the pairing obtained from by mapping
Is easy to see that and that is a partition of . We can therefore rewrite
Because , there are at most indices of such that . By the definition of , the lexicographic ordering of and the ordering of , there exists a such that . Take . For , we have that
where the free parameter has to be fixed such that the last inequality holds. (Note that, because there exists a constant such that , we can always choose an small enough so that this holds). Hence we can bound
iii. Define
Because , we can bound
A similar bound holds for for the same reason. For the other two edges, and , we need to upper bound with a symmetric term, because we do not know whether or . Thus, we have the upper bound
(the same bound holds for ). Moreover, by the lexicographic order, bounds all the other components, and therefore . Now note that (because ), and so
By the previous considerations, substituting into the expression for , we have
iv. From the above bounds we are able to obtain an upper bound for and then use lemma 3.6. Indeed,
Looking at (3.8), we see that we have to fix in order to achieve the required bound. Using that (because and ), the exponent in the previous display is bounded by . Using that , , and putting , we have
Next, let us pick an index such that, for all ,
One possible choice is to take . We finally get
which proves what was said at the beginning of the proof (3.9) for .
v. Finally, consider . In view of the bounds in , this case can be dealt with via classical martingale arguments (see, for example, the McDiarmid inequality and its generalizations in [8]). Considering the variables , we have that . Thus, given our choice of being constant, we have
where . ∎
4. Combining the results for in the above lemma, we get an exponential bound on of the type
where is a suitable constant, possibly different from any of the . By symmetry, the same holds for , which proves the concentration around of .
Remark 3.9.
Up to now we have worked with multi-graphs, so we have to pass to simple graphs with a prescribed degree sequence. Is easy to see that, because of (2.3), we can express the final result as saying that, conditional on the event that the graph is simple, there exist two constants in and a constant such that
3.3. Estimate of second contribution
It remains to show that the pairs with give a bounded contribution of the order with sufficiently high probability. For this it suffices to show that a simple random graph with a prescribed degree sequence cannot have too dense subgraphs (see [18, Lemma 2.5] and [10, Lemma 16]):
Lemma 3.10.
Let be a simple random graph of size drawn uniformly at random with a given degree sequence . Let be two subsets of the vertex set, and let be the set of edges such that either or . Since with a sufficiently large constant, for any there exist a constant such that with probability any pair with satisfies at least one of the following:
(3.10) | ||||
(3.11) |
The above lemma has the following corollary.
Corollary 3.11.
Proof.
Fix and define
where ( is defined similarly). Then, for , write
In order to apply Lemma 3.10, define and , and let and be their cardinality, respectively. Divide the set of indices into two groups:
By the definition of and the set , we have
It suffices to show that either of the contributions coming from or are (the other will follow by symmetry). Focus on . Putting and , we see that the bound can be rewritten as
Divide into the union of and , where satisfies (3.10) and satisfies (3.11). Clearly and
If we are able to show that both contributions from and are , then the theorem follows. It is easy to see that gives a bounded contribution. Indeed,
where in the last step we use that, because ,
It remains to show that
In order to do so, we divide into subsets , , having the following properties:
For we have that and . Thus it suffices to show a bound of for each of the quantities .
For we note that, since ,
For we obtain
For , because the pairs have property (3.11), it follows easily that . Furthermore, because , we have that , . It follows that
For we take advantage of the fact that do not belong to and . This implies that
Hence we have and, by (3.11), also . We can therefore conclude that
where in the last equality we use that .
For , using the property in (5) and (3.11), we have
Also, using that , we have , from which we conclude that
This completes the proof. ∎
4. Proof of the main theorem
Expansion.
Throughout this section we abbreviate and condition on the event that is simple. Recall Lemma 3.3. Compute
Rewriting the equation we have,
Therefore componentwise we have the following inequality,
If , and are non-negative vector (the non-negativity of follows from Perron-Frobenius theory) with , then , we can use Corollary 3.2 and invert the matrix multiplying . Indeed, given that and on the event with probability of Corollary 3.2 and Theorem 3.1, we can invert and expand
and similarly for . Thus,
Our final goal is to determine the expectation , which splits as
(4.1) |
The event has probability at most , where is a large arbitrary constant. Thus, given the deterministic bound , we may focus on . In order to do this, we need to be able to handle terms of the type
Since
the last sum is , which is an error term. It therefore remains to study , . The study of these moments for the configuration model is more involved than for random regular graphs.
Case .
Compute
Since
where the last term comes from the presence of selfloops. It follows that .
Case .
Compute
Write
(by symmetry the third term is equal) and
Indeed in , the summand appears exactly times, because the node has exactly neighbours, and so . Putting the terms together, we get
Taking expectations, we get
Note that is a sum over the wedges centered at vertex , summed all . We can swap the summation over pairs of vertices, and choose a third neighbour to form a wedge, which gives
Compute
Hence
Given the event , by (4.1) and Corollary 3.2, we have that concentrates around with an error, and so we can write
Weak law of large numbers.
References
- [1] N. Alon. Eigenvalues and expanders. Combinatorica, 6(2):83–96, June 1986.
- [2] O. Arizmendi E. and V. Pérez-Abreu. The $S$-transform of symmetric probability measures with unbounded supports. Proceedings of the American Mathematical Society, 137(09):3057–3057, Sept. 2009.
- [3] R. Bauerschmidt, J. Huang, A. Knowles, and H.-T. Yau. Edge rigidity and universality of random regular graphs of intermediate degree. Geom. Funct. Anal., 30(3):693–769, 2020.
- [4] H. Bercovici and D. Voiculescu. Free Convolution of Measures with Unbounded Support. Indiana University Mathematics Journal, 42(3), 1993.
- [5] C. Bordenave. A new proof of Friedman’s second eigenvalue Theorem and its extension to random lifts, Mar. 2019. arXiv:1502.04482 [math].
- [6] C. Bordenave. A new proof of Friedman’s second eigenvalue theorem and its extension to random lifts. Ann. Sci. Éc. Norm. Supér. (4), 53(6):1393–1439, 2020.
- [7] C. Bordenave and M. Lelarge. Resolvent of large random graphs. Random Structures Algorithms, 37(3):332–352, 2010.
- [8] S. Boucheron, G. Lugosi, and P. Massart. Concentration inequalities: a nonasymptotic theory of independence. Oxford University Press, Oxford, 1st ed edition, 2013. OCLC: ocn818449985.
- [9] A. Broder and E. Shamir. On the second eigenvalue of random regular graphs. In 28th Annual Symposium on Foundations of Computer Science (sfcs 1987), pages 286–294, Los Angeles, CA, USA, Oct. 1987. IEEE.
- [10] A. Z. Broder, A. M. Frieze, S. Suen, and E. Upfal. Optimal Construction of Edge-Disjoint Paths in Random Graphs. SIAM Journal on Computing, 28(2):541–573, Jan. 1998.
- [11] A. Chakrabarty, R. S. Hazra, F. den Hollander, and M. Sfragara. Spectra of adjacency and Laplacian matrices of inhomogeneous Erdos-Renyi random graphs. Random Matrices Theory Appl., 10(1):Paper No. 2150009, 34, 2021.
- [12] A. Dembo, E. Lubetzky, and Y. Zhang. Empirical Spectral Distributions of Sparse Random Graphs. In M. E. Vares, R. Fernández, L. R. Fontes, and C. M. Newman, editors, In and Out of Equilibrium 3: Celebrating Vladas Sidoravicius, volume 77, pages 319–345. Springer International Publishing, Cham, 2021. Series Title: Progress in Probability.
- [13] P. Dionigi, D. Garlaschelli, F. d. Hollander, and M. Mandjes. A spectral signature of breaking of ensemble equivalence for constrained random graphs. Electronic Communications in Probability, 26(none), Jan. 2021. arXiv:2009.05155 [math-ph].
- [14] P. Dionigi, D. Garlaschelli, R. Subhra Hazra, F. den Hollander, and M. Mandjes. Central limit theorem for the principal eigenvalue and eigenvector of Chung–Lu random graphs. Journal of Physics: Complexity, 4(1):015008, Mar. 2023.
- [15] L. Erdős, A. Knowles, H.-T. Yau, and J. Yin. Spectral statistics of Erdős–Rényi graphs I: Local semicircle law. The Annals of Probability, 41(3B), May 2013.
- [16] J. Friedman. A proof of Alon’s second eigenvalue conjecture and related problems, May 2004. arXiv:cs/0405020.
- [17] J. Friedman. A proof of Alon’s second eigenvalue conjecture and related problems. Mem. Amer. Math. Soc., 195(910):viii+100, 2008.
- [18] J. Friedman, J. Kahn, and E. Szemerédi. On the second eigenvalue of random regular graphs. In Proceedings of the twenty-first annual ACM symposium on theory of computing, STOC ’89, pages 587–598, New York, NY, USA, 1989. Association for Computing Machinery. Number of pages: 12 Place: Seattle, Washington, USA.
- [19] Z. Füredi and J. Komlós. The eigenvalues of random symmetric matrices. Combinatorica, 1:233–241, 1981.
- [20] D. Garlaschelli, F. den Hollander, and A. Roccaverde. Covariance Structure Behind Breaking of Ensemble Equivalence in Random Graphs. Journal of Statistical Physics, 173(3-4):644–662, Nov. 2018.
- [21] F. Juhász. On the spectrum of a random graph. Algebraic methods in graph theory, 1, 1981.
- [22] B. D. McKay. The expected eigenvalue distribution of a large regular graph. Linear Algebra and its applications, 40:203–216, 1981.
- [23] E. Shamir and J. Spencer. Sharp concentration of the chromatic number on random graphs G(n, p). Combinatorica, 7(1):121–129, Mar. 1987.
- [24] T. Squartini, J. de Mol, F. den Hollander, and D. Garlaschelli. Breaking of ensemble equivalence in networks. Physical review letters, 115(26):268701, 2015.
- [25] H. Touchette. Equivalence and nonequivalence of ensembles: Thermodynamic, macrostate, and measure levels. Journal of Statistical Physics, 159(5):987–1016, 2015.
- [26] L. V. Tran, V. H. Vu, and K. Wang. Sparse random graphs: eigenvalues and eigenvectors. Random Structures Algorithms, 42(1):110–134, 2013.
- [27] R. v. d. van der Hofstad. Random Graphs and Complex Networks. Cambridge University Press, 1 edition, Nov. 2016.
- [28] R. v. d. van der Hofstad. Random Graphs and Complex Networks vol 2, volume 2. 2023.
- [29] D. Voiculescu. Multiplication of Certain Non-Commuting Random Variables. Journal of Operator Theory, 18(2):223–235, 1987.
- [30] V. H. Vu. Recent progress in combinatorial random matrix theory. Probab. Surv., 18:179–200, 2021.
- [31] N. C. Wormald. Models of Random Regular Graphs. In J. D. Lamb and D. A. Preece, editors, Surveys in Combinatorics, 1999, pages 239–298. Cambridge University Press, 1 edition, July 1999.