KnowTuning: Knowledge-aware Fine-tuning for Large Language Models
Abstract
Despite their success at many natural language processing (NLP) tasks, large language models still struggle to effectively leverage knowledge for knowledge-intensive tasks, manifesting limitations such as generating incomplete, non-factual, or illogical answers. These limitations stem from inadequate knowledge awareness of LLMs during vanilla fine-tuning. To address these problems, we propose a knowledge-aware fine-tuning (KnowTuning) method to improve fine-grained and coarse-grained knowledge awareness of LLMs. We devise a fine-grained knowledge augmentation stage to train LLMs to identify difficult fine-grained knowledge in answers. We also propose a coarse-grained knowledge comparison stage to train LLMs to distinguish between reliable and unreliable knowledge, in three aspects: completeness, factuality, and logicality. Extensive experiments on both generic and medical question answering (QA) datasets confirm the effectiveness of KnowTuning, through automatic and human evaluations, across various sizes of LLMs. We further verify that KnowTuning generates more facts with less factual error rate under fine-grained facts evaluation.
KnowTuning: Knowledge-aware Fine-tuning for Large Language Models
Yougang Lyu1,3 Lingyong Yan2 Shuaiqiang Wang2 Haibo Shi2 Dawei Yin2 Pengjie Ren1 Zhumin Chen1 Maarten de Rijke3 Zhaochun Ren4††thanks: Corresponding author. 1Shandong University, Qingdao, China 2Baidu Inc., Beijing, China 3University of Amsterdam, Amsterdam, The Netherlands 4Leiden University, Leiden, The Netherlands [email protected], {yanlingyong, wangshuaiqiang}@baidu.com [email protected], [email protected], [email protected] [email protected], [email protected], [email protected]
1 Introduction
LLM have become a default solution for many natural language processing (NLP) scenarios, including the question answering (QA) task Brown et al. (2020); Ouyang et al. (2022); Qin et al. (2023). To achieve strong performance, most LLM first accumulate substantial knowledge by pre-training on extensive datasets Jiang et al. (2023); Touvron et al. (2023). Then, in the supervised fine-tuning (SFT) stage, these LLMs further learn downstream domain knowledge and how to exploit the corresponding knowledge to answer diverse questions Wei et al. (2022); Chung et al. (2022); Wang et al. (2023f); Peng et al. (2023); Kang et al. (2023); Wang et al. (2023c).


However, fine-tuned LLMs often struggle to effectively leverage knowledge for complex knowledge-intensive question-answering Yu et al. (2023a); Bai et al. (2023); Chen et al. (2023b); Chang et al. (2023). Concretely, many recent studies indicate that LLMs are susceptible to generating incomplete answers, offering incomprehensive and insufficient knowledge Singhal et al. (2022); Bian et al. (2024); Xu et al. (2023a); non-factual answers, delivering factually incorrect knowledge Wang et al. (2023a); Min et al. (2023); Wang et al. (2023b); or illogical answers, providing incoherent and poorly structured knowledge Chen et al. (2023b); Zhong et al. (2023); Kang et al. (2023). Although recent method FactTune Tian et al. (2023) improves the factuality of answers by increasing the proportion of correct facts, it ignores other critical aspects, such as completeness Min et al. (2023) and logicality Xu et al. (2023a).
We hypothesize that these limitations of LLMs arise from insufficient fine-grained and coarse-grained knowledge awareness during vanilla fine-tuning Bian et al. (2024); Ji et al. (2023); Dou et al. (2023); Hua et al. (2024). On the one hand, as illustrated in Figure 1, at the fine-grained level, vanilla fine-tuned LLMs face difficulties in identifying detailed atomic knowledge within the answer, leading to inadequate awareness of fine-grained knowledge. On the other hand, at the coarse-grained level, LLMs frequently fail to distinguish between reliable and unreliable knowledge in answers, indicating a lack of coarse-grained knowledge awareness. Consequently, there is a pressing need for designing knowledge-aware fine-tuning methods. This leads to our central research question: how can we effectively improve both the fine-grained and coarse-grained knowledge awareness of LLMs to address complex knowledge-intensive tasks?
To this end, we propose a novel knowledge-aware fine-tuning method, named KnowTuning, which aims to improve the fine-grained and coarse-grained knowledge awareness of LLMs. KnowTuning consists of two stages: (i) fine-grained knowledge augmentation, and (ii) coarse-grained knowledge comparison. In the first stage, we filter difficult atomic knowledge with high perplexity from original answers, and rewrite fine-grained QA pairs based on the filtered knowledge. After that, we subsequently use both the original and fine-gained QA pairs to train LLMs. In the second stage, we adopt several knowledge-disturbing techniques to construct coarse-grained knowledge comparison sets along three dimensions, completeness, factuality, and logicality. Specifically, we generate answers that are worse in terms of completeness, factuality, or logicality, by deleting, revising, and shuffling the atomic knowledge. Besides, we rephrase original answers based on the atomic knowledge to prevent overfitting. Finally, we combine the rephrased answers and answers with worse completeness, factuality, and logicality as our knowledge comparison sets. We adopt direct preference optimization (DPO) Rafailov et al. (2023) for optimizing LLMs on our coarse-grained knowledge comparison sets.
We conduct experiments on a generic QA dataset and a medical QA dataset using automatic and human evaluations. Experimental results demonstrate the effectiveness of our proposed method KnowTuning, assessing completeness, factuality, and logicality across various sizes of LLMs. Furthermore, we demonstrate that KnowTuning not only generates more facts but also reduces the factual error rate during fine-grained facts evaluation.
In summary, our main contributions are:
-
•
We focus on systematically enhancing the knowledge awareness of LLMs at both fine-grained and coarse-grained levels to address complex knowledge-intensive tasks.
-
•
We introduce KnowTuning, a novel method that fine-tunes LLMs to leverage fine-grained knowledge augmentation and coarse-grained knowledge comparison to improve fine-grained and coarse-grained knowledge awareness of LLMs.
-
•
We demonstrate the effectiveness of KnowTuning in the generic and medical domain QA datasets through automatic and human evaluations, across various sizes of LLMs. Furthermore, KnowTuning generates more facts with less factual error rate under fine-grained facts evaluation.111The code is available at https://github.com/youganglyu/KnowTuning
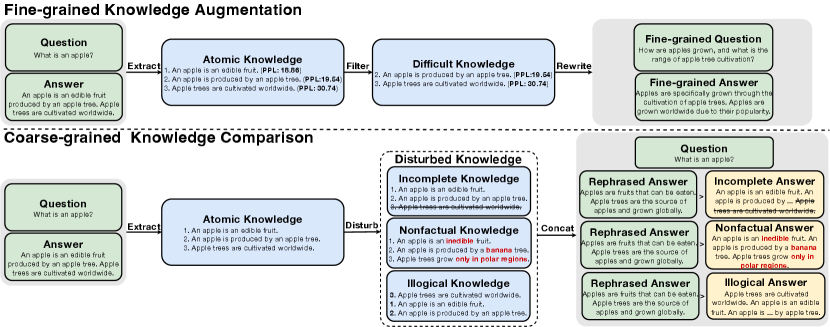
2 Related Work
2.1 LLMs for Knowledge-intensive Tasks
LLM have been applied to various knowledge-intensive tasks Moiseev et al. (2022); Yu et al. (2023b); Khattab et al. (2022); Tian et al. (2023); Zhang et al. (2023a); Xu et al. (2023b); Mishra et al. (2023); Nguyen et al. (2023); Zhang et al. (2024). Previous work mainly focus on knowledge-intensive tasks with short-form answers. Liu et al. (2022b) use few-shot demonstrations to elicit relevant knowledge statements from LLMs for QA tasks. Liu et al. (2022a) train a neural model to generate relevant knowledge through reinforcement learning for QA tasks. Liu et al. (2023a) propose a unified model for generating relevant knowledge and solving QA tasks.
However, these methods primarily address multiple-choice QA, rather than the more complex open-ended knowledge-intensive QA tasks Krishna et al. (2021); Kadavath et al. (2022); Liu et al. (2022a, 2023a); Kang et al. (2023), which aim to solve questions that require detailed explanations and extensive domain knowledge. Recent research indicates that LLMs face challenges in tackling complex knowledge-intensive QA tasks Yu et al. (2023a); Bai et al. (2023); Chang et al. (2023). In particular, they are prone to generating responses that are non-factual Lee et al. (2022); Sun et al. (2023); Su et al. (2022), incomplete Singhal et al. (2022); Bian et al. (2024), or illogical Chen et al. (2023b); Zhong et al. (2023). Recently, for open-ended knowledge-intensive tasks, Tian et al. (2023) propose a method FacTune to improve factuality. Specifically, they first automatically evaluate the proportion of correct facts in candidate answers as factuality scores, and fine-tuning LLMs to increase the likelihood of generating answers with higher factuality scores. In contrast, we focus on improving the knowledge awareness of LLMs at multiple essential aspects simultaneously, for solving complex knowledge-intensive QA tasks.
2.2 Fine-tuning for LLMs
Fine-tuning is a kind of method to optimize pre-trained LLMs for further learning downstream domain knowledge and how to exploit the corresponding knowledge to answer diverse questions Brown et al. (2020); Ouyang et al. (2022). Previously, fine-tuning is mainly focused on enhancing general-purpose QA abilities of LLMs Wang et al. (2022); Wei et al. (2022); Longpre et al. (2023). These approaches mainly adopt human-annotated datasets to build the QA dataset. Recently, an alternative strategy involves generating QA datasets through the utilization of advanced LLMs to create answers to a variety of questions Wang et al. (2023f); Shumailov et al. (2023).
Another line of fine-tuning methods fuse information about the quality of the generated answers into the supervision signals Zhao et al. (2023); Guo et al. (2023); Wang et al. (2023d); Dong et al. (2023); Chen et al. (2024); Zhao et al. (2024). Rafailov et al. (2023) propose direct preference optimization (DPO) to directly optimize LLMs on the pair-wise comparison set. Song et al. (2023) propose preference ranking optimization (PRO) to fine-tune LLMs on list-wise comparison sets. Yuan et al. (2023) propose a margin-rank loss to optimize the LLMs on comparison sets. Since collecting large-scale human judgment for the quality of generated answers is expensive, Bai et al. (2022) and Lee et al. (2023) propose reinforcement learning from AI feedback (RLAIF) methods to leverage off-the-shelf LLMs to annotate general helpfulness scores. In contrast, our work focuses on enhancing the fine-grained and coarse-grained knowledge-awareness of LLMs to improve performance in terms of completeness, factuality, and logicality simultaneously.
3 Method
In this section, we detail the KnowTuning method. First, we introduce the preliminaries. Then, we introduce the fine-grained knowledge augmentation. Next, we introduce coarse-grained knowledge comparison in detail. Finally, a training process for KnowTuning is explained.
3.1 Preliminaries
Supervised fine-tuning. Supervised fine-tuning (SFT) aims to train pre-trained LLMs to understand and answer natural language questions. Formally, given a QA dataset , where and denotes a question and a corresponding answer. The training objective of SFT is to minimize the following loss:
(1) |
where denotes the -th token of .
Atomic knowledge. Since individual facts can well cover the knowledge in answers Nenkova and Passonneau (2004); Zhang and Bansal (2021); Liu et al. (2023b); Min et al. (2023); Wei et al. (2024), we break an answer into individual facts as atomic knowledge. The atomic knowledge is a short statement conveying one piece of fact, which is a more fine-grained unit than a sentence. Specifically, we extract atomic knowledge set from the original answers as follows:
(2) |
where is implemented by prompting OpenAI models to extract atomic knowledge, following Min et al. (2023).
3.2 Fine-grained Knowledge Augmentation
As illustrated in Figure 2, to improve the fine-grained knowledge awareness of LLMs, we filter difficult atomic knowledge for LLMs, and rewrite fine-grained QA pairs based on the difficult knowledge. After that, we subsequently use both the original and fine-gained QA pairs to train LLMs. To filter the difficult atomic knowledge for LLMs, we first compute the generation perplexity of each atomic knowledge conditioned on as follows:
(3) |
Since high perplexity indicates the lack of knowledge awareness of LLMs on specific atomic knowledge, we select percent of the atomic knowledge set in descending order of perplexity to form the difficult knowledge set . Then, we rewrite the question as a fine-grained question relevant to difficult knowledge , as follows:
(4) |
where is implemented by prompting OpenAI models. In addition, we rewrite the answer based on the difficult knowledge set as the fine-grained answer:
(5) |
Finally, we combine the original QA dataset and the fine-grained QA pairs as the fine-grained knowledge augmentation dataset as:
(6) |
3.3 Coarse-grained Knowledge Comparison
To improve coarse-grained knowledge awareness of LLMs in terms of completeness, factuality and logicality, we construct three comparison sets by deleting, revising, and shuffling atomic knowledge.
Knowledge completeness comparison. To improve knowledge completeness awareness of LLMs, we construct the knowledge completeness comparison set by randomly deleting the atomic knowledge. Specifically, we first randomly delete atomic knowledge in the atomic knowledge set as incomplete knowledge set:
(7) |
where refers to randomly delete percent of atomic knowledge . Then, we concatenate leftover atomic knowledge of the incomplete knowledge set as an incomplete answer:
(8) |
In addition, to avoid overfitting on the original answers Jain et al. (2023), we rephrase the original answers based on the original atomic knowledge set as:
(9) |
Finally, we combine the rephrased answer and the incomplete answer into knowledge completeness comparison set as follows:
(10) |
Knowledge factuality comparison. To improve the knowledge factuality awareness of LLMs, we construct the knowledge factuality comparison set by revising the atomic knowledge as nonfactual atomic knowledge. Specifically, we first revise the atomic knowledge set as follows:
(11) |
where is implemented by prompting OpenAI models to revise the atomic knowledge to the wrong atomic knowledge. Then, we concatenate all atomic knowledge in the nonfactual knowledge set as:
(12) |
Finally, we combine the rephrased answer and the nonfactual answer into knowledge factuality comparison set as follows:
(13) |
Knowledge logicality comparison. To improve the knowledge logicality awareness of LLMs, we construct the knowledge logicality comparison set by randomly shuffling the atomic knowledge. Specifically, we first randomly shuffle all atomic knowledge in the atomic knowledge set as the illogical knowledge set:
(14) |
where is implemented by shuffling the order of all atomic knowledge in the atomic knowledge set . Then, we follow the shuffled order to concatenate all atomic knowledge in the illogical knowledge set as an illogical answer:
(15) |
Next, we combine the rephrased answer and the illogical answer into knowledge logicality comparison set as follows:
(16) |
Finally, we combine the knowledge completeness comparison set, the knowledge factuality comparison set, and the knowledge logicality comparison set as the coarse-grained knowledge comparison set:
(17) |
3.4 Training
To improve the knowledge awareness of LLMs for solving complex knowledge-intensive tasks, KnowTuning includes fine-grained knowledge augmentation training and coarse-grained knowledge comparison training. Specifically, we first train LLMs on fine-grained knowledge augmentation dataset , resulting in a model denoted as . To improve the coarse-grained knowledge awareness of the model , we rewrite the DPO Rafailov et al. (2023) loss as follows:
(18) |
where denotes the answer pair of the question , and is the better answer. To maintain coarse-grained knowledge awareness of better answers, we add SFT loss into the coarse-grained knowledge comparison loss:
(19) |
where is a term for better answers and is a scalar weighting hyperparameter.
4 Experiments
4.1 Research Questions
We aim to answer the following research questions in our experiments: RQ1: How does KnowTuning perform on generic and medical QA under automatic evaluation and human evaluation? RQ2: How does KnowTuning perform on generic and medical QA under fine-grained facts evaluation? RQ3: How do fine-grained knowledge augmentation and coarse-grained knowledge comparison affect the performance of KnowTuning?
Dolly | MedQuAD | NQ | ELI5 | |||||
---|---|---|---|---|---|---|---|---|
Method | METEOR | BERTScore | METEOR | BERTScore | METEOR | BERTScore | METEOR | BERTScore |
Backbone Language Model: Llama2-7b-base | ||||||||
Base | 12.29 | 78.07 | 12.79 | 78.44 | 5.10 | 72.70 | 9.09 | 76.05 |
SFT | 14.01 | 84.38 | 19.95 | 80.97 | 7.55 | 76.71 | 11.96 | 79.65 |
RLAIF | 17.60 | 85.31 | 20.60 | 83.82 | 10.77 | 79.62 | 13.66 | 80.41 |
FactTune | 16.84 | 85.16 | 21.82 | 82.99 | 10.08 | 79.09 | 14.19 | 80.83 |
KnowTuning | 19.56 | 86.37 | 24.71 | 84.28 | 12.22 | 80.54 | 16.32 | 81.74 |
Backbone Language Model: Llama2-13b-base | ||||||||
Base | 11.59 | 77.90 | 12.12 | 78.29 | 5.51 | 73.80 | 7.79 | 75.63 |
SFT | 15.31 | 84.39 | 19.66 | 82.34 | 8.70 | 78.18 | 12.00 | 81.21 |
RLAIF | 19.03 | 85.43 | 20.37 | 83.13 | 11.79 | 80.30 | 13.61 | 82.06 |
FactTune | 18.59 | 85.38 | 21.42 | 83.49 | 11.37 | 80.02 | 13.74 | 82.16 |
KnowTuning | 20.01 | 86.32 | 25.21 | 84.41 | 12.56 | 80.74 | 14.45 | 83.06 |
4.2 Datasets
We conduct experiments on general domain and domain-specific knowledge-intensive question-answering datasets:
-
•
Dolly Conover et al. (2023) is a general domain QA dataset carefully curated by thousands of human annotators. Since we focus on open-ended generic domain QA, we filter QA pairs of “open_qa” and “general_qa” categories.
- •
To evaluate the performance across a wider range of knowledge-intensive tasks, we further evaluate generic QA models on two representative test sets from knowledge intensive language tasks (KILT) benchmark Petroni et al. (2021):
-
•
NQ Kwiatkowski et al. (2019) consists of real questions directed to the Google search engine. Every question is paired with a corresponding Wikipedia page that includes a detailed long-form answer and a concise short answer. We filter questions and corresponding long answers as testing QA pairs.
-
•
ELI5 Fan et al. (2019) includes a set of question-answer-evidence triples. The questions are complex, and the responses are comprehensive, explanatory, and presented in a free-form style. We filter questions and corresponding answers as testing QA pairs.
More details of datasets are in Appendix A.
4.3 Baselines
We compare our model with the following baselines:
-
•
Base denotes that testing Llama2-base models Touvron et al. (2023) under zero-shot setting.
- •
- •
-
•
FactTune Tian et al. (2023) constructs factuality comparison sets by calculating the proportion of correct facts in candidate answers.
More details of baselines are in Appendix B.
Completeness | Factuality | Logicality | |||||||||
Method | Dataset | Win | Tie | Lose | Win | Tie | Lose | Win | Tie | Lose | Avg. gap |
Backbone Language Model: Llama2-7b-base | |||||||||||
KnowTuning vs Base | Dolly | 88.50∗ | 3.00 | 8.50 | 73.00∗ | 20.00 | 7.00 | 80.50∗ | 12.00 | 7.50 | +73.00 |
KnowTuning vs SFT | 78.50∗ | 5.50 | 16.00 | 37.00∗ | 46.50 | 16.50 | 50.50∗ | 34.00 | 15.50 | +39.33 | |
KnowTuning vs RLAIF | 69.50∗ | 5.00 | 25.50 | 32.00∗ | 49.00 | 19.00 | 46.50∗ | 39.00 | 14.50 | +29.67 | |
KnowTuning vs FactTune | 64.50∗ | 10.00 | 25.50 | 30.00∗ | 53.00 | 17.00 | 31.50∗ | 55.50 | 13.00 | +23.50 | |
KnowTuning vs Base | MedQuAD | 93.00∗ | 3.00 | 4.00 | 72.50∗ | 20.50 | 7.00 | 85.00∗ | 8.50 | 6.50 | +77.67 |
KnowTuning vs SFT | 81.00∗ | 3.50 | 15.50 | 46.50∗ | 37.50 | 16.00 | 64.50∗ | 21.50 | 14.00 | +48.83 | |
KnowTuning vs RLAIF | 85.00∗ | 2.50 | 12.50 | 41.00∗ | 38.50 | 20.50 | 50.50∗ | 30.00 | 19.50 | +41.33 | |
KnowTuning vs FactTune | 83.00∗ | 3.50 | 13.50 | 40.50∗ | 36.50 | 23.00 | 50.50∗ | 31.50 | 18.00 | +39.83 | |
Backbone Language Model: Llama2-13b-base | |||||||||||
KnowTuning vs Base | Dolly | 85.50∗ | 6.50 | 8.00 | 66.00∗ | 24.50 | 9.50 | 81.00∗ | 13.00 | 6.00 | +69.67 |
KnowTuning vs SFT | 77.00∗ | 5.00 | 18.00 | 35.50∗ | 49.50 | 15.00 | 45.00∗ | 40.00 | 15.00 | +36.50 | |
KnowTuning vs RLAIF | 73.50∗ | 4.00 | 22.50 | 33.50∗ | 52.50 | 14.00 | 46.50∗ | 40.50 | 13.00 | +34.67 | |
KnowTuning vs FactTune | 68.50∗ | 6.50 | 25.00 | 30.50∗ | 55.00 | 14.50 | 36.00∗ | 54.00 | 10.00 | +28.50 | |
KnowTuning vs Base | MedQuAD | 92.50∗ | 2.50 | 5.00 | 73.50∗ | 17.50 | 9.00 | 84.00∗ | 8.00 | 8.00 | +76.00 |
KnowTuning vs SFT | 86.50∗ | 3.50 | 10.00 | 45.50∗ | 41.00 | 13.50 | 60.00∗ | 31.00 | 9.00 | +53.16 | |
KnowTuning vs RLAIF | 82.50∗ | 5.00 | 12.50 | 38.50∗ | 48.00 | 13.50 | 54.00∗ | 38.50 | 7.50 | +47.17 | |
KnowTuning vs FactTune | 78.00∗ | 4.50 | 17.50 | 37.00∗ | 47.00 | 16.00 | 48.50∗ | 39.50 | 12.00 | +39.33 |
4.4 Evaluation Metrics
We present our experimental results using two evaluation metrics: automatic evaluation and human-based evaluation. Following previous studies Clinciu et al. (2021); Slobodkin et al. (2023), we employ two automatic metrics for absolute quality evaluation: the lexicon-based metric METEOR Banerjee and Lavie (2005) and the semantic-based metric BERTScore Zhang et al. (2019). Since recent studies propose that GPT-4 can effectively evaluate the quality of LLMs answers Zheng et al. (2024a); Dubois et al. (2023); Fu et al. (2023), we also conduct GPT-4 pairwise evaluation. Specifically, given the golden label as a reference, we employ GPT-4 to rate generated answers on three aspects: completeness, factuality, and logicality, on a range of 1 to 10. Following Singhal et al. (2022); Zheng et al. (2024a); Zhang et al. (2023b), we define completeness, factuality and logicality as: (i) Completeness: it examines whether the answers provide comprehensive and sufficient knowledge to the questions. (ii) Factuality: it examines whether the knowledge in the answers is factually correct. (iii) Logicality: it examines whether the knowledge in the answers is logically structured. Following Li et al. (2023); Chen et al. (2023a), we define “Win-Tie-Lose” as: (i) Win: KnowTuning wins twice, or wins once and ties once. (ii) Tie: KnowTuning ties twice, or wins once and loses once. (iii) Lose: KnowTuning loses twice, or loses once and ties once.
We also employ human judgments as the gold standard for assessing the quality of answers. Specifically, human evaluators perform pair-wise comparisons of the top-performing models identified in automatic evaluations. They are presented with a question with a golden answer, and asked to judge two generated answers on three aspects: completeness, factuality, and logicality.
To evaluate the capabilities of LLMs at a fine-grained level, we follow Min et al. (2023) to conduct fine-grained facts evaluation. Specifically, we first break candidate answers into individual facts, and use gpt-3.5-turbo to measure the correctness of each fact based on the golden answer as a reference. Following Tian et al. (2023), we report the number of correct facts ( Correct), the number of incorrect facts ( Incorrect), the number of total facts ( Total) and the proportion of correct facts out of the total number of extracted facts ( Correct). More details of the evaluation are in Appendix C.
4.5 Implementation Details
5 Experimental Results and Analysis
To answer our research questions, we conduct generic domain and medical domain QA experiments, fine-grained facts evaluation, and ablation studies. In addition, we conducted a case study to gain further understanding of the effectiveness of KnowTuning.
Completeness | Factuality | Logicality | |||||||||
---|---|---|---|---|---|---|---|---|---|---|---|
Method | Dataset | Win | Tie | Lose | Win | Tie | Lose | Win | Tie | Lose | Avg. gap |
Backbone Language Model: Llama2-7b-base | |||||||||||
KnowTuning vs FactTune | Dolly | 61.00∗ | 12.00 | 27.00 | 28.00∗ | 58.50 | 13.50 | 33.50∗ | 50.00 | 16.50 | +21.83 |
KnowTuning vs FactTune | MedQuAD | 73.00∗ | 9.00 | 18.00 | 40.00∗ | 43.00 | 17.00 | 45.50∗ | 36.00 | 18.50 | +35.00 |
Backbone Language Model: Llama2-13b-base | |||||||||||
KnowTuning vs FactTune | Dolly | 58.00∗ | 11.00 | 31.00 | 32.50∗ | 56.50 | 11.00 | 35.00∗ | 53.00 | 12.00 | +23.83 |
KnowTuning vs FactTune | MedQuAD | 78.00∗ | 6.50 | 15.50 | 43.00∗ | 45.50 | 11.50 | 39.00∗ | 45.50 | 15.50 | +39.17 |
Dolly | MedQuAD | |||||||
---|---|---|---|---|---|---|---|---|
Method | # Correct | # Incorrect | # Total | % Correct | # Correct | # Incorrect | # Total | % Correct |
Backbone Language Model: Llama2-7b-base | ||||||||
Base | 6.15 | 3.62 | 9.77 | 62.94 | 6.54 | 3.42 | 9.96 | 65.66 |
SFT | 7.77 | 1.85 | 9.62 | 80.77 | 16.11 | 1.73 | 17.84 | 90.30 |
RLAIF | 11.23 | 2.10 | 13.33 | 84.25 | 10.86 | 0.95 | 11.81 | 91.96 |
FactTune | 11.25 | 1.92 | 13.17 | 85.42 | 12.83 | 0.83 | 13.66 | 93.92 |
KnowTuning | 14.40 | 2.36 | 16.76 | 85.92 | 18.04 | 0.98 | 19.02 | 94.85 |
Backbone Language Model: Llama2-13b-base | ||||||||
Base | 9.57 | 4.28 | 13.85 | 69.10 | 7.96 | 3.50 | 11.46 | 69.46 |
SFT | 9.96 | 2.21 | 12.17 | 81.84 | 16.82 | 1.66 | 18.48 | 91.02 |
RLAIF | 10.72 | 2.16 | 12.88 | 83.23 | 13.01 | 1.16 | 14.17 | 91.81 |
FactTune | 12.73 | 2.12 | 14.85 | 85.72 | 13.02 | 1.01 | 14.03 | 92.80 |
KnowTuning | 15.44 | 2.20 | 17.64 | 87.53 | 19.01 | 1.11 | 20.12 | 94.48 |
5.1 Main Results (RQ1)
Automatic evaluation. Table 1 and Table 2 present the reference-based GPT-4 evaluation results and absolute quality evaluation results for both generic and medical domain QA datasets. Across all metrics, KnowTuning outperforms the baseline models in these domains. Based on the results, we have three main observations:
-
•
KnowTuning demonstrates effectiveness under lexicon-based and semantic-based evaluations. As shown in Table 1, our method consistently improves the absolute quality of answers for general and medical QA tasks. Furthermore, these results illustrate the ability of our method to generalize to a wider range of knowledge-intensive datasets, such as NQ and ELI5.
-
•
KnowTuning consistently outperforms baselines in terms of completeness, factuality and logicality, across generic and domain-specific QA datasets. Compared with Base and SFT, KnowTuning focuses on improving fine-grained and coarse-grained knowledge awareness of LLMs, which significantly improves the performance. Compared with RLAIF and FactTune, KnowTuning is more effective in improving the performance of LLMs on complex knowledge-intensive QA in multiple aspects. The reason is that RLAIF improves the performance by calculating overall helpfulness scores and FactTune focuses on improving the factuality, they ignore improving the knowledge awareness of LLMs in multiple essential aspects simultaneously.
-
•
KnowTuning demonstrates effectiveness on LLMs across different sizes. We observe that KnowTuning consistently improves the performance of QA tasks on different scales (7b and 13B) LLMs. This finding aligns with Bian et al. (2024) and Mecklenburg et al. (2024): LLMs learn a lot of generic knowledge during the pre-training stage but still need to learn downstream domain knowledge and explore how to effectively leverage knowledge for solving knowledge-intensive QA tasks.
Human evaluation. Human evaluations are crucial for accurately assessing the quality of answers. As shown in Table 3, to facilitate human annotation processes, we focus on comparing KnowTuning with the state-of-art baseline FactTune:
-
•
Our findings indicate that KnowTuning consistently surpasses FactTune in terms of completeness, factuality, and logicality performance across various sizes of LLMs under human evaluation.
-
•
KnowTuning demonstrates superior performance over QA in both generic and medical domain QA evaluated by human, in terms of completeness, factuality, and logicality.
Completeness | Factuality | Logicality | ||||||||
---|---|---|---|---|---|---|---|---|---|---|
Method | Win | Tie | Lose | Win | Tie | Lose | Win | Tie | Lose | Avg. gap |
-KA vs KnowTuning | 32.50 | 20.00 | 47.50 | 16.00 | 57.50 | 26.50 | 12.50 | 61.50 | 26.00 | -13.00 |
-KCC vs KnowTuning | 18.50 | 31.00 | 50.50 | 11.00 | 72.50 | 16.50 | 10.50 | 61.50 | 28.00 | -18.33 |
-KFC vs KnowTuning | 23.00 | 28.50 | 48.50 | 8.50 | 70.50 | 21.00 | 12.00 | 60.50 | 27.50 | -17.83 |
-KLC vs KnowTuning | 25.50 | 27.50 | 47.00 | 12.00 | 73.00 | 15.00 | 9.50 | 60.00 | 30.50 | -15.17 |
-KC vs KnowTuning | 11.50 | 6.00 | 82.50 | 16.00 | 52.00 | 32.00 | 15.50 | 40.50 | 44.00 | -38.50 |
5.2 Fine-grained Fact Evaluation (RQ2)
To evaluate the ability of methods to generate correct facts at the fine-grained level, we conduct fine-grained facts evaluation experiments. Based on the results in Table 4, we have two main observations:
-
•
Knowtuning generates answers with a higher proportion of correct facts across various sizes. Compared to baselines, KnowTuning can generate more facts with less factual error rate across different sizes of LLMs. Although RLAIF and FactTune improve the proportion of correct facts, they ignore fine-grained knowledge augmentation and coarse-grained knowledge completeness awareness. Note that even though FactTune generates fewer incorrect facts, KnowTuning outperforms FactTune on the more critical metric of the percentage of correct facts.
-
•
KnowTuning generates larger amounts of correct facts across generic and domain-specific QA datasets. Compared to SFT, we observe that KnowTuning consistently generates more correct facts across generic and domain-specific QA datasets. However, in the specific medical domain QA, RLAIF and FactTune generate fewer correct facts than SFT. This is because LLMs learn a large amount of generic knowledge during the pre-training stage, yet still lack domain-specific knowledge for downstream tasks Mecklenburg et al. (2024). This underscores the necessity for enhancing fine-grained knowledge awareness in domain-specific, knowledge-intensive QA tasks, as well as the need to improve coarse-grained knowledge awareness across key aspects of completeness, factuality, and logicality.
5.3 Ablation Studies (RQ3)
In Table 5, we compare KnowTuning with several ablative variants. The variants are as follows: (i) -KA: we remove the fine-grained knowledge augmentation. (ii) -KCC: we remove knowledge completeness comparison set. (iii) -KFC: we remove knowledge factuality comparison set. (iv) -KLC: we remove knowledge logicality comparison set. (v) -KC: we remove all coarse-grained knowledge comparison sets. Our findings are as follows:
-
•
Removing the fine-grained knowledge augmentation. We observe that removing fine-grained knowledge augmentation (-KA) decreases the performance of all three aspects. This indicates that fine-grained knowledge augmentation is effective for improving fine-grained knowledge awareness of LLMs.
-
•
Removing the coarse-grained knowledge comparison. The absence of coarse-grained knowledge comparisons results in substantial performance degradation in knowledge-intensive QA tasks. Specifically, removing the knowledge completeness comparison (-KCC) adversely affects completeness, the elimination of the knowledge factuality comparison (-KFC) undermines factuality, and the removal of the knowledge logicality comparison (-KLC) diminishes logicality. Although deleting and revising atomic knowledge can impact logicality, shuffling has been found more effective in improving coarse-grained logicality for LLMs. Furthermore, removing all coarse-grained knowledge comparison sets (-KC) results in a significant drop in performance across all aspects of the knowledge-intensive QA task.
5.4 Case Study
We conduct several case studies and find that KnowTuning is more effective at generating complete, factual and logical answers than baselines across various sizes of LLMs. More details of our case study results are in Appendix E.
6 Conclusions
In this paper, we focus on improving the knowledge awareness of LLMs via fine-tuning for complex knowledge-intensive tasks. We have proposed KnowTuning to fine-tune LLMs through fine-grained knowledge augmentation and coarse-grained knowledge comparison stages. We have conducted comprehensive experiments on generic and medical domain QA datasets, demonstrating the effectiveness of KnowTuning through automatic and human evaluations, across various sizes of LLMs. Moreover, KnowTuning generates more facts with less factual error rate under fine-grained facts evaluation.
Limitations
In this study, KnowTuning is mainly aimed at generic and medical knowledge-intensive tasks, we plan to adopt KnowTuning to other tasks such as legal domain QA Zhong et al. (2020); Lyu et al. (2022, 2023a) and mathematical reasoning Luo et al. (2023). Moreover, our efforts have been concentrated on enhancing the knowledge awareness of LLMs during the fine-tuning stage. Future studies will aim to explore improving knowledge awareness of LLMs in the pre-training stage Rosset et al. (2020).
Ethical Considerations
KnowTuning mainly focuses on completeness, factuality, and logicality, but not social bias Pitoura et al. (2017); Lyu et al. (2023b) or the potential for generating harmful or toxic content Song et al. (2024); Hewitt et al. (2024); Gao et al. (2024). We plan to adopt our method to reduce social bias and harmful content at fine-grained and coarse-grained levels in future work.
Acknowledgments
This work was supported by the Natural Science Foundation of China (62272274, 62372275, 62102234, 62202271, 62072279), the National Key R&D Program of China with grant No.2022YFC3303004, the Natural Science Foundation of Shandong Province (ZR2021QF129), the China Scholarship Council under grant number 202306220180, the Dutch Research Council (NWO), under project numbers 024.004.022, NWA.1389.20.183, and KICH3.LTP.20.006, and the European Union’s Horizon Europe program under grant agreement No 101070212. All content represents the opinion of the authors, which is not necessarily shared or endorsed by their respective employers and/or sponsors.
References
- Abacha and Demner-Fushman (2019) Asma Ben Abacha and Dina Demner-Fushman. 2019. A question-entailment approach to question answering. BMC Bioinform., 20(1):511:1–511:23.
- August et al. (2022) Tal August, Katharina Reinecke, and Noah A. Smith. 2022. Generating scientific definitions with controllable complexity. In Proceedings of ACL, pages 8298–8317.
- Bai et al. (2022) Yuntao Bai, Saurav Kadavath, Sandipan Kundu, Amanda Askell, Jackson Kernion, Andy Jones, Anna Chen, Anna Goldie, Azalia Mirhoseini, Cameron McKinnon, Carol Chen, Catherine Olsson, Christopher Olah, Danny Hernandez, Dawn Drain, Deep Ganguli, Dustin Li, Eli Tran-Johnson, Ethan Perez, Jamie Kerr, Jared Mueller, Jeffrey Ladish, Joshua Landau, Kamal Ndousse, Kamile Lukosiute, Liane Lovitt, Michael Sellitto, Nelson Elhage, Nicholas Schiefer, Noemí Mercado, Nova DasSarma, Robert Lasenby, Robin Larson, Sam Ringer, Scott Johnston, Shauna Kravec, Sheer El Showk, Stanislav Fort, Tamera Lanham, Timothy Telleen-Lawton, Tom Conerly, Tom Henighan, Tristan Hume, Samuel R. Bowman, Zac Hatfield-Dodds, Ben Mann, Dario Amodei, Nicholas Joseph, Sam McCandlish, Tom Brown, and Jared Kaplan. 2022. Constitutional AI: Harmlessness from AI feedback. CoRR, abs/2212.08073.
- Bai et al. (2023) Yuyang Bai, Shangbin Feng, Vidhisha Balachandran, Zhaoxuan Tan, Shiqi Lou, Tianxing He, and Yulia Tsvetkov. 2023. KGQuiz: Evaluating the generalization of encoded knowledge in large language models. CoRR, abs/2310.09725.
- Banerjee and Lavie (2005) Satanjeev Banerjee and Alon Lavie. 2005. METEOR: An automatic metric for MT evaluation with improved correlation with human judgments. In Proceedings of the acl workshop on intrinsic and extrinsic evaluation measures for machine translation and/or summarization, pages 65–72.
- Bian et al. (2024) Ning Bian, Xianpei Han, Le Sun, Hongyu Lin, Yaojie Lu, and Ben He. 2024. ChatGPT is a knowledgeable but inexperienced solver: An investigation of commonsense problem in large language models. In Proceedings of COLING.
- Brown et al. (2020) Tom B. Brown, Benjamin Mann, Nick Ryder, Melanie Subbiah, Jared Kaplan, Prafulla Dhariwal, Arvind Neelakantan, Pranav Shyam, Girish Sastry, Amanda Askell, Sandhini Agarwal, Ariel Herbert-Voss, Gretchen Krueger, Tom Henighan, Rewon Child, Aditya Ramesh, Daniel M. Ziegler, Jeffrey Wu, Clemens Winter, Christopher Hesse, Mark Chen, Eric Sigler, Mateusz Litwin, Scott Gray, Benjamin Chess, Jack Clark, Christopher Berner, Sam McCandlish, Alec Radford, Ilya Sutskever, and Dario Amodei. 2020. Language models are few-shot learners. In Proceedings of NeurIPS.
- Chang et al. (2023) Yupeng Chang, Xu Wang, Jindong Wang, Yuan Wu, Kaijie Zhu, Hao Chen, Linyi Yang, Xiaoyuan Yi, Cunxiang Wang, Yidong Wang, Wei Ye, Yue Zhang, Yi Chang, Philip S. Yu, Qiang Yang, and Xing Xie. 2023. A survey on evaluation of large language models. CoRR, abs/2307.03109.
- Chen et al. (2023a) Lichang Chen, Shiyang Li, Jun Yan, Hai Wang, Kalpa Gunaratna, Vikas Yadav, Zheng Tang, Vijay Srinivasan, Tianyi Zhou, Heng Huang, and Hongxia Jin. 2023a. AlpaGasus: Training a better Alpaca with fewer data. CoRR, abs/2307.08701.
- Chen et al. (2023b) Shiqi Chen, Yiran Zhao, Jinghan Zhang, I-Chun Chern, Siyang Gao, Pengfei Liu, and Junxian He. 2023b. FELM: benchmarking factuality evaluation of large language models. CoRR, abs/2310.00741.
- Chen et al. (2024) Zhipeng Chen, Kun Zhou, Wayne Xin Zhao, Junchen Wan, Fuzheng Zhang, Di Zhang, and Ji-Rong Wen. 2024. Improving large language models via fine-grained reinforcement learning with minimum editing constraint. CoRR, abs/2401.06081.
- Chung et al. (2022) Hyung Won Chung, Le Hou, Shayne Longpre, Barret Zoph, Yi Tay, William Fedus, Eric Li, Xuezhi Wang, Mostafa Dehghani, Siddhartha Brahma, Albert Webson, Shixiang Shane Gu, Zhuyun Dai, Mirac Suzgun, Xinyun Chen, Aakanksha Chowdhery, Sharan Narang, Gaurav Mishra, Adams Yu, Vincent Y. Zhao, Yanping Huang, Andrew M. Dai, Hongkun Yu, Slav Petrov, Ed H. Chi, Jeff Dean, Jacob Devlin, Adam Roberts, Denny Zhou, Quoc V. Le, and Jason Wei. 2022. Scaling instruction-finetuned language models. CoRR, abs/2210.11416.
- Clinciu et al. (2021) Miruna-Adriana Clinciu, Arash Eshghi, and Helen F. Hastie. 2021. A study of automatic metrics for the evaluation of natural language explanations. In Proceedings of EACL, pages 2376–2387. Association for Computational Linguistics.
- Conover et al. (2023) Mike Conover, Matt Hayes, Ankit Mathur, Jianwei Xie, Jun Wan, Sam Shah, Ali Ghodsi, Patrick Wendell, Matei Zaharia, and Reynold Xin. 2023. Free Dolly: Introducing the world’s first truly open instruction-tuned LLM.
- Dong et al. (2023) Hanze Dong, Wei Xiong, Deepanshu Goyal, Rui Pan, Shizhe Diao, Jipeng Zhang, Kashun Shum, and Tong Zhang. 2023. RAFT: Reward ranked finetuning for generative foundation model alignment. CoRR, abs/2304.06767.
- Dou et al. (2023) Shihan Dou, Enyu Zhou, Yan Liu, Songyang Gao, Jun Zhao, Wei Shen, Yuhao Zhou, Zhiheng Xi, Xiao Wang, Xiaoran Fan, Shiliang Pu, Jiang Zhu, Rui Zheng, Tao Gui, Qi Zhang, and Xuanjing Huang. 2023. LoRAMoE: Revolutionizing mixture of experts for maintaining world knowledge in language model alignment. CoRR, abs/2312.09979.
- Dubois et al. (2023) Yann Dubois, Xuechen Li, Rohan Taori, Tianyi Zhang, Ishaan Gulrajani, Jimmy Ba, Carlos Guestrin, Percy Liang, and Tatsunori B. Hashimoto. 2023. AlpacaFarm: A simulation framework for methods that learn from human feedback. CoRR, abs/2305.14387.
- Fan et al. (2019) Angela Fan, Yacine Jernite, Ethan Perez, David Grangier, Jason Weston, and Michael Auli. 2019. ELI5: long form question answering. In Proceedings of ACL, pages 3558–3567.
- Fu et al. (2023) Jinlan Fu, See-Kiong Ng, Zhengbao Jiang, and Pengfei Liu. 2023. GPTScore: Evaluate as you desire. CoRR, abs/2302.04166.
- Gao et al. (2024) Bofei Gao, Feifan Song, Yibo Miao, Zefan Cai, Zhe Yang, Liang Chen, Helan Hu, Runxin Xu, Qingxiu Dong, Ce Zheng, et al. 2024. Towards a unified view of preference learning for large language models: A survey. arXiv preprint arXiv:2409.02795.
- Guan et al. (2021) Jian Guan, Xiaoxi Mao, Changjie Fan, Zitao Liu, Wenbiao Ding, and Minlie Huang. 2021. Long text generation by modeling sentence-level and discourse-level coherence. In Proceedings of ACL, pages 6379–6393.
- Guo et al. (2023) Geyang Guo, Ranchi Zhao, Tianyi Tang, Wayne Xin Zhao, and Ji-Rong Wen. 2023. Beyond imitation: Leveraging fine-grained quality signals for alignment. CoRR, abs/2311.04072.
- Hewitt et al. (2024) John Hewitt, Sarah Chen, Lanruo Lora Xie, Edward Adams, Percy Liang, and Christopher D Manning. 2024. Model editing with canonical examples. arXiv preprint arXiv:2402.06155.
- Hu et al. (2022) Edward J. Hu, Yelong Shen, Phillip Wallis, Zeyuan Allen-Zhu, Yuanzhi Li, Shean Wang, Lu Wang, and Weizhu Chen. 2022. LoRA: Low-rank adaptation of large language models. In Proceedings of ICLR.
- Hua et al. (2024) Wenyue Hua, Jiang Guo, Mingwen Dong, Henghui Zhu, Patrick Ng, and Zhiguo Wang. 2024. Propagation and pitfalls: Reasoning-based assessment of knowledge editing through counterfactual tasks. CoRR, abs/2401.17585.
- Jain et al. (2023) Neel Jain, Ping-yeh Chiang, Yuxin Wen, John Kirchenbauer, Hong-Min Chu, Gowthami Somepalli, Brian R. Bartoldson, Bhavya Kailkhura, Avi Schwarzschild, Aniruddha Saha, Micah Goldblum, Jonas Geiping, and Tom Goldstein. 2023. NEFTune: Noisy embeddings improve instruction finetuning. CoRR, abs/2310.05914.
- Ji et al. (2023) Ziwei Ji, Tiezheng Yu, Yan Xu, Nayeon Lee, Etsuko Ishii, and Pascale Fung. 2023. Towards mitigating hallucination in large language models via self-reflection. CoRR, abs/2310.06271.
- Jiang et al. (2023) Albert Q. Jiang, Alexandre Sablayrolles, Arthur Mensch, Chris Bamford, Devendra Singh Chaplot, Diego de Las Casas, Florian Bressand, Gianna Lengyel, Guillaume Lample, Lucile Saulnier, Lélio Renard Lavaud, Marie-Anne Lachaux, Pierre Stock, Teven Le Scao, Thibaut Lavril, Thomas Wang, Timothée Lacroix, and William El Sayed. 2023. Mistral 7b. CoRR, abs/2310.06825.
- Kadavath et al. (2022) Saurav Kadavath, Tom Conerly, Amanda Askell, Tom Henighan, Dawn Drain, Ethan Perez, Nicholas Schiefer, Zac Hatfield-Dodds, Nova DasSarma, Eli Tran-Johnson, Scott Johnston, Sheer El Showk, Andy Jones, Nelson Elhage, Tristan Hume, Anna Chen, Yuntao Bai, Sam Bowman, Stanislav Fort, Deep Ganguli, Danny Hernandez, Josh Jacobson, Jackson Kernion, Shauna Kravec, Liane Lovitt, Kamal Ndousse, Catherine Olsson, Sam Ringer, Dario Amodei, Tom Brown, Jack Clark, Nicholas Joseph, Ben Mann, Sam McCandlish, Chris Olah, and Jared Kaplan. 2022. Language models (mostly) know what they know. CoRR, abs/2207.05221.
- Kang et al. (2023) Minki Kang, Seanie Lee, Jinheon Baek, Kenji Kawaguchi, and Sung Ju Hwang. 2023. Knowledge-augmented reasoning distillation for small language models in knowledge-intensive tasks. CoRR, abs/2305.18395.
- Khattab et al. (2022) Omar Khattab, Keshav Santhanam, Xiang Lisa Li, David Hall, Percy Liang, Christopher Potts, and Matei Zaharia. 2022. Demonstrate-search-predict: Composing retrieval and language models for knowledge-intensive NLP. CoRR, abs/2212.14024.
- Ko et al. (2020) Miyoung Ko, Jinhyuk Lee, Hyunjae Kim, Gangwoo Kim, and Jaewoo Kang. 2020. Look at the first sentence: Position bias in question answering. In Proceedings of EMNLP, pages 1109–1121.
- Krishna et al. (2021) Kalpesh Krishna, Aurko Roy, and Mohit Iyyer. 2021. Hurdles to progress in long-form question answering. In Proceedings of NAACL-HLT, pages 4940–4957.
- Kwiatkowski et al. (2019) Tom Kwiatkowski, Jennimaria Palomaki, Olivia Redfield, Michael Collins, Ankur P. Parikh, Chris Alberti, Danielle Epstein, Illia Polosukhin, Jacob Devlin, Kenton Lee, Kristina Toutanova, Llion Jones, Matthew Kelcey, Ming-Wei Chang, Andrew M. Dai, Jakob Uszkoreit, Quoc Le, and Slav Petrov. 2019. Natural questions: a benchmark for question answering research. Trans. Assoc. Comput. Linguistics, 7:452–466.
- Lee et al. (2023) Harrison Lee, Samrat Phatale, Hassan Mansoor, Kellie Lu, Thomas Mesnard, Colton Bishop, Victor Carbune, and Abhinav Rastogi. 2023. RLAIF: Scaling reinforcement learning from human feedback with AI feedback. CoRR, abs/2309.00267.
- Lee et al. (2022) Nayeon Lee, Wei Ping, Peng Xu, Mostofa Patwary, Pascale Fung, Mohammad Shoeybi, and Bryan Catanzaro. 2022. Factuality enhanced language models for open-ended text generation. In Proceedings of NeurIPS.
- Li et al. (2023) Ming Li, Yong Zhang, Zhitao Li, Jiuhai Chen, Lichang Chen, Ning Cheng, Jianzong Wang, Tianyi Zhou, and Jing Xiao. 2023. From quantity to quality: Boosting LLM performance with self-guided data selection for instruction tuning. CoRR, abs/2308.12032.
- Liu et al. (2022a) Jiacheng Liu, Skyler Hallinan, Ximing Lu, Pengfei He, Sean Welleck, Hannaneh Hajishirzi, and Yejin Choi. 2022a. Rainier: Reinforced knowledge introspector for commonsense question answering. In Proceedings of EMNLP, pages 8938–8958.
- Liu et al. (2022b) Jiacheng Liu, Alisa Liu, Ximing Lu, Sean Welleck, Peter West, Ronan Le Bras, Yejin Choi, and Hannaneh Hajishirzi. 2022b. Generated knowledge prompting for commonsense reasoning. In Proceedings of ACL, pages 3154–3169.
- Liu et al. (2023a) Jiacheng Liu, Ramakanth Pasunuru, Hannaneh Hajishirzi, Yejin Choi, and Asli Celikyilmaz. 2023a. Crystal: Introspective reasoners reinforced with self-feedback. In Proceedings of EMNLP, pages 11557–11572.
- Liu et al. (2023b) Yixin Liu, Alexander R. Fabbri, Pengfei Liu, Yilun Zhao, Linyong Nan, Ruilin Han, Simeng Han, Shafiq Joty, Chien-Sheng Wu, Caiming Xiong, and Dragomir Radev. 2023b. Revisiting the gold standard: Grounding summarization evaluation with robust human evaluation. In Proceedings of ACL, pages 4140–4170. Association for Computational Linguistics.
- Longpre et al. (2023) Shayne Longpre, Le Hou, Tu Vu, Albert Webson, Hyung Won Chung, Yi Tay, Denny Zhou, Quoc V. Le, Barret Zoph, Jason Wei, and Adam Roberts. 2023. The Flan collection: Designing data and methods for effective instruction tuning. In Proceedings of ICML, volume 202, pages 22631–22648.
- Loshchilov and Hutter (2019) Ilya Loshchilov and Frank Hutter. 2019. Decoupled weight decay regularization. In Proceedings of ICLR.
- Luo et al. (2023) Haipeng Luo, Qingfeng Sun, Can Xu, Pu Zhao, Jianguang Lou, Chongyang Tao, Xiubo Geng, Qingwei Lin, Shifeng Chen, and Dongmei Zhang. 2023. WizardMath: Empowering mathematical reasoning for large language models via reinforced evol-instruct. arXiv preprint arXiv:2308.09583.
- Lyu et al. (2023a) Yougang Lyu, Jitai Hao, Zihan Wang, Kai Zhao, Shen Gao, Pengjie Ren, Zhumin Chen, Fang Wang, and Zhaochun Ren. 2023a. Multi-defendant legal judgment prediction via hierarchical reasoning. In Findings of EMNLP, pages 2198–2209.
- Lyu et al. (2023b) Yougang Lyu, Piji Li, Yechang Yang, Maarten de Rijke, Pengjie Ren, Yukun Zhao, Dawei Yin, and Zhaochun Ren. 2023b. Feature-level debiased natural language understanding. In Proceedings of AAAI, pages 13353–13361.
- Lyu et al. (2022) Yougang Lyu, Zihan Wang, Zhaochun Ren, Pengjie Ren, Zhumin Chen, Xiaozhong Liu, Yujun Li, Hongsong Li, and Hongye Song. 2022. Improving legal judgment prediction through reinforced criminal element extraction. Inf. Process. Manag., 59(1):102780.
- Mangrulkar et al. (2022) Sourab Mangrulkar, Sylvain Gugger, Lysandre Debut, Younes Belkada, Sayak Paul, and Benjamin Bossan. 2022. PEFT: State-of-the-art parameter-efficient fine-tuning methods. https://github.com/huggingface/peft.
- Mecklenburg et al. (2024) Nick Mecklenburg, Yiyou Lin, Xiaoxiao Li, Daniel Holstein, Leonardo Nunes, Sara Malvar, Bruno Silva, Ranveer Chandra, Vijay Aski, Pavan Kumar Reddy Yannam, et al. 2024. Injecting new knowledge into large language models via supervised fine-tuning. arXiv preprint arXiv:2404.00213.
- Min et al. (2023) Sewon Min, Kalpesh Krishna, Xinxi Lyu, Mike Lewis, Wen-tau Yih, Pang Wei Koh, Mohit Iyyer, Luke Zettlemoyer, and Hannaneh Hajishirzi. 2023. FActScore: Fine-grained atomic evaluation of factual precision in long form text generation. In Proceedings of EMNLP, pages 12076–12100.
- Mishra et al. (2023) Aditi Mishra, Sajjadur Rahman, Hannah Kim, Kushan Mitra, and Estevam Hruschka. 2023. Characterizing large language models as rationalizers of knowledge-intensive tasks. CoRR, abs/2311.05085.
- Moiseev et al. (2022) Fedor Moiseev, Zhe Dong, Enrique Alfonseca, and Martin Jaggi. 2022. SKILL: Structured knowledge infusion for large language models. In Proceedings of NAACL, pages 1581–1588.
- Nenkova and Passonneau (2004) Ani Nenkova and Rebecca J. Passonneau. 2004. Evaluating content selection in summarization: The Pyramid method. In Proceedings of HLT-NAACL, pages 145–152.
- Nguyen et al. (2023) Minh Nguyen, Kishan K. C., Toan Nguyen, Ankit Chadha, and Thuy Vu. 2023. Efficient fine-tuning large language models for knowledge-aware response planning. In Machine Learning and Knowledge Discovery in Databases: Research Track - European Conference, ECML PKDD 2023, Turin, Italy, September 18-22, 2023, Proceedings, Part II, volume 14170 of Lecture Notes in Computer Science, pages 593–611.
- Ouyang et al. (2022) Long Ouyang, Jeffrey Wu, Xu Jiang, Diogo Almeida, Carroll L. Wainwright, Pamela Mishkin, Chong Zhang, Sandhini Agarwal, Katarina Slama, Alex Ray, John Schulman, Jacob Hilton, Fraser Kelton, Luke Miller, Maddie Simens, Amanda Askell, Peter Welinder, Paul F. Christiano, Jan Leike, and Ryan Lowe. 2022. Training language models to follow instructions with human feedback. In Proceedings of NeurIPS.
- Peng et al. (2023) Baolin Peng, Chunyuan Li, Pengcheng He, Michel Galley, and Jianfeng Gao. 2023. Instruction tuning with GPT-4. CoRR, abs/2304.03277.
- Petroni et al. (2021) Fabio Petroni, Aleksandra Piktus, Angela Fan, Patrick S. H. Lewis, Majid Yazdani, Nicola De Cao, James Thorne, Yacine Jernite, Vladimir Karpukhin, Jean Maillard, Vassilis Plachouras, Tim Rocktäschel, and Sebastian Riedel. 2021. KILT: a benchmark for knowledge intensive language tasks. In Proceedings of NAACL-HLT, pages 2523–2544.
- Pitoura et al. (2017) Evaggelia Pitoura, Panayiotis Tsaparas, Giorgos Flouris, Irini Fundulaki, Panagiotis Papadakos, Serge Abiteboul, and Gerhard Weikum. 2017. On measuring bias in online information. SIGMOD Rec., 46(4):16–21.
- Qin et al. (2023) Chengwei Qin, Aston Zhang, Zhuosheng Zhang, Jiaao Chen, Michihiro Yasunaga, and Diyi Yang. 2023. Is ChatGPT a general-purpose natural language processing task solver? In Proceedings of EMNLP, pages 1339–1384.
- Rafailov et al. (2023) Rafael Rafailov, Archit Sharma, Eric Mitchell, Stefano Ermon, Christopher D. Manning, and Chelsea Finn. 2023. Direct preference optimization: Your language model is secretly a reward model. CoRR, abs/2305.18290.
- Rosset et al. (2020) Corby Rosset, Chenyan Xiong, Minh Phan, Xia Song, Paul N. Bennett, and Saurabh Tiwary. 2020. Knowledge-aware language model pretraining. CoRR, abs/2007.00655.
- Shumailov et al. (2023) Ilia Shumailov, Zakhar Shumaylov, Yiren Zhao, Yarin Gal, Nicolas Papernot, and Ross J. Anderson. 2023. The curse of recursion: Training on generated data makes models forget. CoRR, abs/2305.17493.
- Singhal et al. (2022) Karan Singhal, Shekoofeh Azizi, Tao Tu, S. Sara Mahdavi, Jason Wei, Hyung Won Chung, Nathan Scales, Ajay Kumar Tanwani, Heather Cole-Lewis, Stephen Pfohl, Perry Payne, Martin Seneviratne, Paul Gamble, Chris Kelly, Nathaneal Schärli, Aakanksha Chowdhery, Philip Andrew Mansfield, Blaise Agüera y Arcas, Dale R. Webster, Gregory S. Corrado, Yossi Matias, Katherine Chou, Juraj Gottweis, Nenad Tomasev, Yun Liu, Alvin Rajkomar, Joelle K. Barral, Christopher Semturs, Alan Karthikesalingam, and Vivek Natarajan. 2022. Large language models encode clinical knowledge. CoRR, abs/2212.13138.
- Slobodkin et al. (2023) Aviv Slobodkin, Avi Caciularu, Eran Hirsch, and Ido Dagan. 2023. Don’t add, don’t miss: Effective content preserving generation from pre-selected text spans. In Findings of EMNLP, pages 12784–12800.
- Song et al. (2024) Feifan Song, Yuxuan Fan, Xin Zhang, Peiyi Wang, and Houfeng Wang. 2024. ICDPO: Effectively borrowing alignment capability of others via in-context direct preference optimization. CoRR, abs/2402.09320.
- Song et al. (2023) Feifan Song, Bowen Yu, Minghao Li, Haiyang Yu, Fei Huang, Yongbin Li, and Houfeng Wang. 2023. Preference ranking optimization for human alignment. CoRR, abs/2306.17492.
- Su et al. (2022) Dan Su, Xiaoguang Li, Jindi Zhang, Lifeng Shang, Xin Jiang, Qun Liu, and Pascale Fung. 2022. Read before generate! Faithful long form question answering with machine reading. In Findings of ACL, pages 744–756.
- Sun et al. (2023) Weiwei Sun, Zhengliang Shi, Shen Gao, Pengjie Ren, Maarten de Rijke, and Zhaochun Ren. 2023. Contrastive learning reduces hallucination in conversations. In Proceedings of AAAI, pages 13618–13626.
- Taori et al. (2023) Rohan Taori, Ishaan Gulrajani, Tianyi Zhang, Yann Dubois, Xuechen Li, Carlos Guestrin, Percy Liang, and Tatsunori B. Hashimoto. 2023. Stanford alpaca: An instruction-following llama model. https://github.com/tatsu-lab/stanford_alpaca.
- Tian et al. (2023) Katherine Tian, Eric Mitchell, Huaxiu Yao, Christopher D Manning, and Chelsea Finn. 2023. Fine-tuning language models for factuality. In Proceedings of NeurIPS Workshop on Instruction Tuning and Instruction Following.
- Touvron et al. (2023) Hugo Touvron, Louis Martin, Kevin Stone, Peter Albert, Amjad Almahairi, Yasmine Babaei, Nikolay Bashlykov, Soumya Batra, Prajjwal Bhargava, Shruti Bhosale, Dan Bikel, Lukas Blecher, Cristian Canton-Ferrer, Moya Chen, Guillem Cucurull, David Esiobu, Jude Fernandes, Jeremy Fu, Wenyin Fu, Brian Fuller, Cynthia Gao, Vedanuj Goswami, Naman Goyal, Anthony Hartshorn, Saghar Hosseini, Rui Hou, Hakan Inan, Marcin Kardas, Viktor Kerkez, Madian Khabsa, Isabel Kloumann, Artem Korenev, Punit Singh Koura, Marie-Anne Lachaux, Thibaut Lavril, Jenya Lee, Diana Liskovich, Yinghai Lu, Yuning Mao, Xavier Martinet, Todor Mihaylov, Pushkar Mishra, Igor Molybog, Yixin Nie, Andrew Poulton, Jeremy Reizenstein, Rashi Rungta, Kalyan Saladi, Alan Schelten, Ruan Silva, Eric Michael Smith, Ranjan Subramanian, Xiaoqing Ellen Tan, Binh Tang, Ross Taylor, Adina Williams, Jian Xiang Kuan, Puxin Xu, Zheng Yan, Iliyan Zarov, Yuchen Zhang, Angela Fan, Melanie Kambadur, Sharan Narang, Aurélien Rodriguez, Robert Stojnic, Sergey Edunov, and Thomas Scialom. 2023. Llama 2: Open foundation and fine-tuned chat models. CoRR, abs/2307.09288.
- Wang et al. (2023a) Cunxiang Wang, Sirui Cheng, Zhikun Xu, Bowen Ding, Yidong Wang, and Yue Zhang. 2023a. Evaluating open question answering evaluation. CoRR, abs/2305.12421.
- Wang et al. (2023b) Cunxiang Wang, Xiaoze Liu, Yuanhao Yue, Xiangru Tang, Tianhang Zhang, Jiayang Cheng, Yunzhi Yao, Wenyang Gao, Xuming Hu, Zehan Qi, Yidong Wang, Linyi Yang, Jindong Wang, Xing Xie, Zheng Zhang, and Yue Zhang. 2023b. Survey on factuality in large language models: Knowledge, retrieval and domain-specificity. CoRR, abs/2310.07521.
- Wang et al. (2023c) Keheng Wang, Feiyu Duan, Sirui Wang, Peiguang Li, Yunsen Xian, Chuantao Yin, Wenge Rong, and Zhang Xiong. 2023c. Knowledge-driven CoT: Exploring faithful reasoning in LLMs for knowledge-intensive question answering. CoRR, abs/2308.13259.
- Wang et al. (2023d) Peiyi Wang, Lei Li, Liang Chen, Feifan Song, Binghuai Lin, Yunbo Cao, Tianyu Liu, and Zhifang Sui. 2023d. Making large language models better reasoners with alignment. CoRR, abs/2309.02144.
- Wang et al. (2023e) Peiyi Wang, Lei Li, Liang Chen, Dawei Zhu, Binghuai Lin, Yunbo Cao, Qi Liu, Tianyu Liu, and Zhifang Sui. 2023e. Large language models are not fair evaluators. CoRR, abs/2305.17926.
- Wang et al. (2023f) Yizhong Wang, Yeganeh Kordi, Swaroop Mishra, Alisa Liu, Noah A. Smith, Daniel Khashabi, and Hannaneh Hajishirzi. 2023f. Self-instruct: Aligning language models with self-generated instructions. In Proceedings of ACL, pages 13484–13508.
- Wang et al. (2022) Yizhong Wang, Swaroop Mishra, Pegah Alipoormolabashi, Yeganeh Kordi, Amirreza Mirzaei, Atharva Naik, Arjun Ashok, Arut Selvan Dhanasekaran, Anjana Arunkumar, David Stap, Eshaan Pathak, Giannis Karamanolakis, Haizhi Gary Lai, Ishan Purohit, Ishani Mondal, Jacob Anderson, Kirby Kuznia, Krima Doshi, Kuntal Kumar Pal, Maitreya Patel, Mehrad Moradshahi, Mihir Parmar, Mirali Purohit, Neeraj Varshney, Phani Rohitha Kaza, Pulkit Verma, Ravsehaj Singh Puri, Rushang Karia, Savan Doshi, Shailaja Keyur Sampat, Siddhartha Mishra, Sujan Reddy A, Sumanta Patro, Tanay Dixit, and Xudong Shen. 2022. Super-naturalinstructions: Generalization via declarative instructions on 1600+ NLP tasks. In Proceedings of EMNLP, pages 5085–5109.
- Wei et al. (2022) Jason Wei, Maarten Bosma, Vincent Y. Zhao, Kelvin Guu, Adams Wei Yu, Brian Lester, Nan Du, Andrew M. Dai, and Quoc V. Le. 2022. Finetuned language models are zero-shot learners. In Proceedings of ICLR.
- Wei et al. (2024) Jerry Wei, Chengrun Yang, Xinying Song, Yifeng Lu, Nathan Hu, Dustin Tran, Daiyi Peng, Ruibo Liu, Da Huang, Cosmo Du, et al. 2024. Long-form factuality in large language models. arXiv preprint arXiv:2403.18802.
- Xu et al. (2023a) Fangyuan Xu, Yixiao Song, Mohit Iyyer, and Eunsol Choi. 2023a. A critical evaluation of evaluations for long-form question answering. In Proceedings of ACL, pages 3225–3245.
- Xu et al. (2023b) Shicheng Xu, Liang Pang, Huawei Shen, Xueqi Cheng, and Tat-Seng Chua. 2023b. Search-in-the-chain: Towards the accurate, credible and traceable content generation for complex knowledge-intensive tasks. CoRR, abs/2304.14732.
- Yu et al. (2023a) Jifan Yu, Xiaozhi Wang, Shangqing Tu, Shulin Cao, Daniel Zhang-li, Xin Lv, Hao Peng, Zijun Yao, Xiaohan Zhang, Hanming Li, Chunyang Li, Zheyuan Zhang, Yushi Bai, Yantao Liu, Amy Xin, Nianyi Lin, Kaifeng Yun, Linlu Gong, Jianhui Chen, Zhili Wu, Yunjia Qi, Weikai Li, Yong Guan, Kaisheng Zeng, Ji Qi, Hailong Jin, Jinxin Liu, Yu Gu, Yuan Yao, Ning Ding, Lei Hou, Zhiyuan Liu, Bin Xu, Jie Tang, and Juanzi Li. 2023a. KoLA: Carefully benchmarking world knowledge of large language models. CoRR, abs/2306.09296.
- Yu et al. (2023b) Wenhao Yu, Dan Iter, Shuohang Wang, Yichong Xu, Mingxuan Ju, Soumya Sanyal, Chenguang Zhu, Michael Zeng, and Meng Jiang. 2023b. Generate rather than retrieve: Large language models are strong context generators. In Proceedings of ICLR.
- Yuan et al. (2023) Zheng Yuan, Hongyi Yuan, Chuanqi Tan, Wei Wang, Songfang Huang, and Fei Huang. 2023. RRHF: Rank responses to align language models with human feedback without tears. CoRR, abs/2304.05302.
- Zhang and Bansal (2021) Shiyue Zhang and Mohit Bansal. 2021. Finding a balanced degree of automation for summary evaluation. In Proceedings of EMNLP, pages 6617–6632.
- Zhang et al. (2019) Tianyi Zhang, Varsha Kishore, Felix Wu, Kilian Q Weinberger, and Yoav Artzi. 2019. BERTScore: Evaluating text generation with BERT. arXiv preprint arXiv:1904.09675.
- Zhang et al. (2024) Xiaoyu Zhang, Ruobing Xie, Yougang Lyu, Xin Xin, Pengjie Ren, Mingfei Liang, Bo Zhang, Zhanhui Kang, Maarten de Rijke, and Zhaochun Ren. 2024. Towards empathetic conversational recommender systems. arXiv preprint arXiv:2409.10527.
- Zhang et al. (2023a) Yichi Zhang, Zhuo Chen, Yin Fang, Lei Cheng, Yanxi Lu, Fangming Li, Wen Zhang, and Huajun Chen. 2023a. Knowledgeable preference alignment for llms in domain-specific question answering. CoRR, abs/2311.06503.
- Zhang et al. (2023b) Yue Zhang, Ming Zhang, Haipeng Yuan, Shichun Liu, Yongyao Shi, Tao Gui, Qi Zhang, and Xuanjing Huang. 2023b. LLMEval: A preliminary study on how to evaluate large language models. CoRR, abs/2312.07398.
- Zhao et al. (2023) Yao Zhao, Rishabh Joshi, Tianqi Liu, Misha Khalman, Mohammad Saleh, and Peter J. Liu. 2023. SLiC-HF: Sequence likelihood calibration with human feedback. CoRR, abs/2305.10425.
- Zhao et al. (2024) Yukun Zhao, Lingyong Yan, Weiwei Sun, Guoliang Xing, Shuaiqiang Wang, Chong Meng, Zhicong Cheng, Zhaochun Ren, and Dawei Yin. 2024. Improving the robustness of large language models via consistency alignment. In Proceedings of the 2024 Joint International Conference on Computational Linguistics, Language Resources and Evaluation, LREC/COLING 2024, 20-25 May, 2024, Torino, Italy, pages 8931–8941.
- Zheng et al. (2024a) Lianmin Zheng, Wei-Lin Chiang, Ying Sheng, Siyuan Zhuang, Zhanghao Wu, Yonghao Zhuang, Zi Lin, Zhuohan Li, Dacheng Li, Eric P. Xing, Hao Zhang, Joseph E. Gonzalez, and Ion Stoica. 2024a. Judging LLM-as-a-judge with MT-Bench and Chatbot Arena. Proceedings of NeurIPS, 36.
- Zheng et al. (2024b) Yaowei Zheng, Richong Zhang, Junhao Zhang, Yanhan Ye, Zheyan Luo, and Yongqiang Ma. 2024b. LlamaFactory: Unified efficient fine-tuning of 100+ language models. arXiv preprint arXiv:2403.13372.
- Zhong et al. (2020) Haoxi Zhong, Chaojun Xiao, Cunchao Tu, Tianyang Zhang, Zhiyuan Liu, and Maosong Sun. 2020. JEC-QA: A legal-domain question answering dataset. In Proceedings of AAAI, volume 34, pages 9701–9708.
- Zhong et al. (2023) Qihuang Zhong, Liang Ding, Juhua Liu, Bo Du, and Dacheng Tao. 2023. Can ChatGPT understand too? A comparative study on ChatGPT and fine-tuned BERT. CoRR, abs/2302.10198.
Appendix
Appendix A Details of Datasets
-
•
Dolly Conover et al. (2023): Given our focus on open-ended generic domain QA, we selected QA pairs specifically categorized under "open_qa" and "general_qa" for our dataset. We filter 4,000 QA pairs for training, 200 QA pairs for validation, and 200 QA pairs for testing.
-
•
MedQuAD Abacha and Demner-Fushman (2019): The dataset covers 37 different question types. In this paper, following August et al. (2022), we filter QA pairs of the category “Information” for giving definitions and information about medical terms. We filter 4000 QA pairs for training, 200 QA pairs for validation and 200 QA pairs for testing.
-
•
NQ Kwiatkowski et al. (2019): We filter 200 questions and corresponding long answers as testing QA pairs from the development set. The length of these long answers ranges from 100 to 500.
-
•
ELI5 Fan et al. (2019): We filter 200 questions in the test set and the corresponding highest scoring answers as testing QA pairs.
Appendix B Details of Baselines
- •
-
•
SFT: We follow standard vanilla fine-tuning loss in Eq. 1 to train LLMs on original QA datasets.
- •
-
•
FactTune Tian et al. (2023): We follow Min et al. (2023) to first break each candidate answers into individual facts, and prompt LLMs to measure the correctness of each fact based on the golden answer as a reference.222https://github.com/shmsw25/FActScore Then, we construct factuality comparison sets by the percentage of correct facts. Finally, we adopt DPO Rafailov et al. (2023) for factuality comparison sets optimization.
Appendix C Details of Evaluation
C.1 GPT-4 Evaluation
This section provides specifics of the GPT-4 prompt utilized for reference-based evaluation, employing gpt4-turbo. Figure 3 illustrates the adapted prompt from Zheng et al. (2024a), aimed at assessing the completeness, factuality, and logicality of answers. To avoid positional bias Ko et al. (2020); Wang et al. (2023e), we evaluate each answer in both positions during two separate runs.
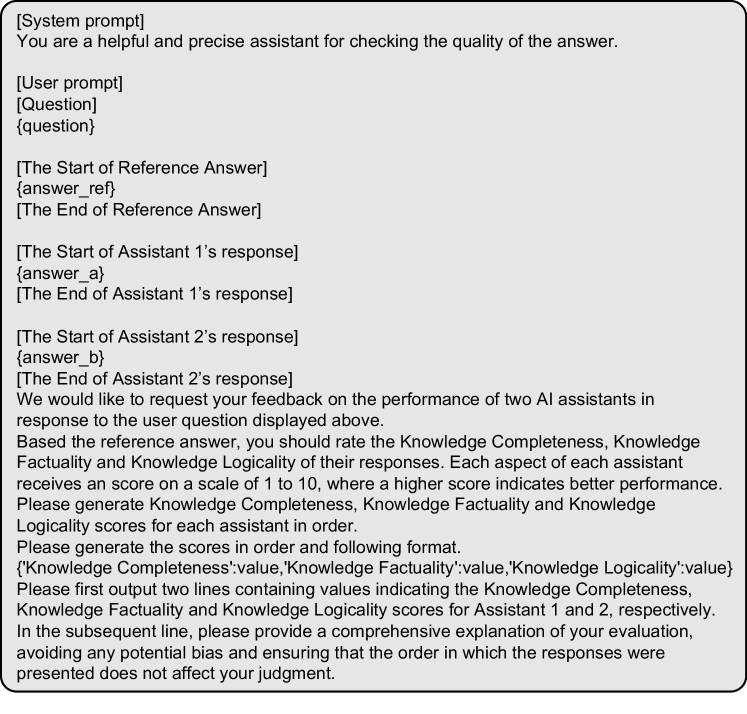
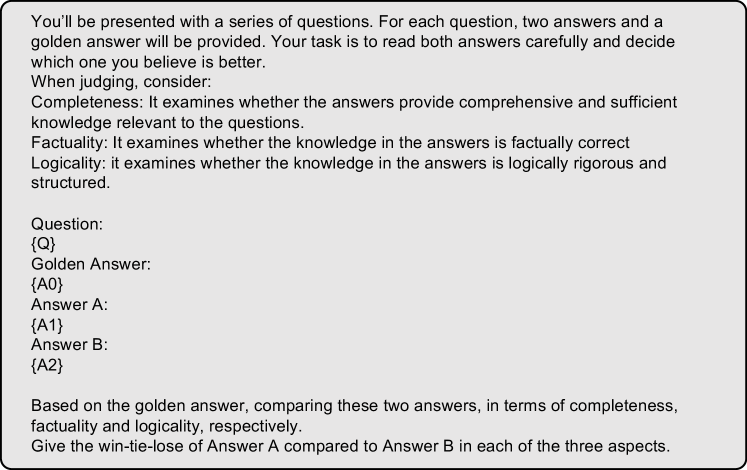
C.2 Human Evaluation
For the human evaluation, we hired people with undergraduate degrees and undergraduate medical degrees to annotate generic QA and medical QA test sets, respectively, to ensure the trustworthiness of the human evaluations, and we allowed the human evaluators to access Wikipedia to further validate the knowledge during the evaluation process. Instructions for human evaluation are depicted in Figure 4.
C.3 Fine-grained facts evaluation
Appendix D Details of Implementation
D.1 Prompts for Extracting, Rewriting, and Revising
Details for the prompts used in , , and are provided. Figures 5, 6, 7 and 8 display the prompts for extracting atomic knowledge, rewriting fine-grained questions, rewriting fine-grained answers, and revising atomic knowledge into nonfactual knowledge, respectively.
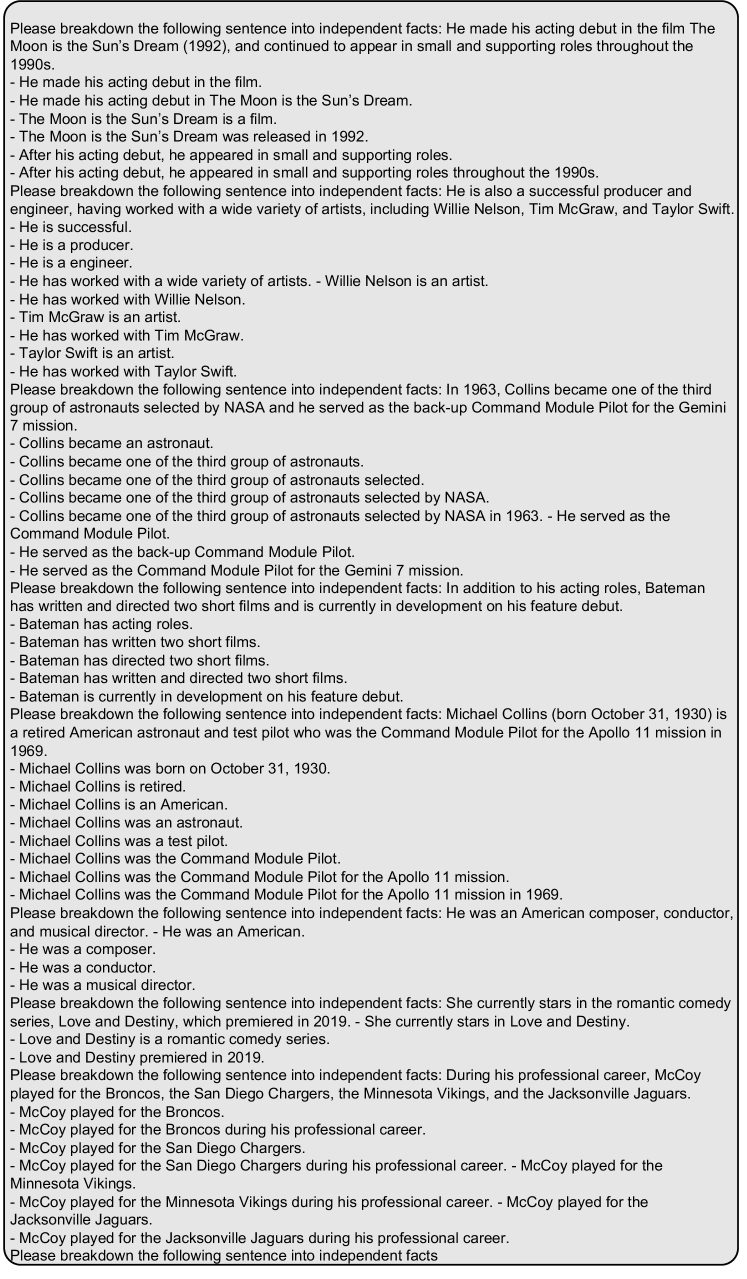
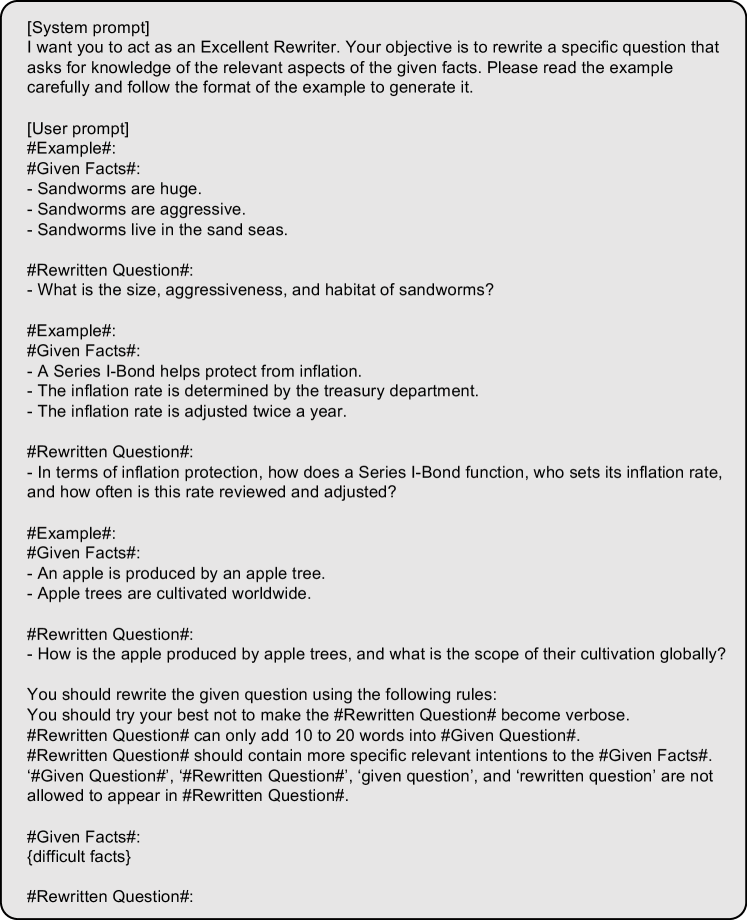
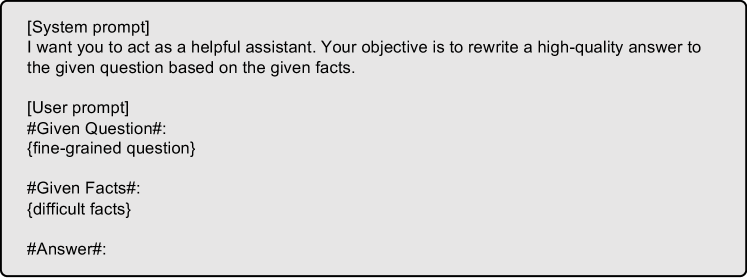
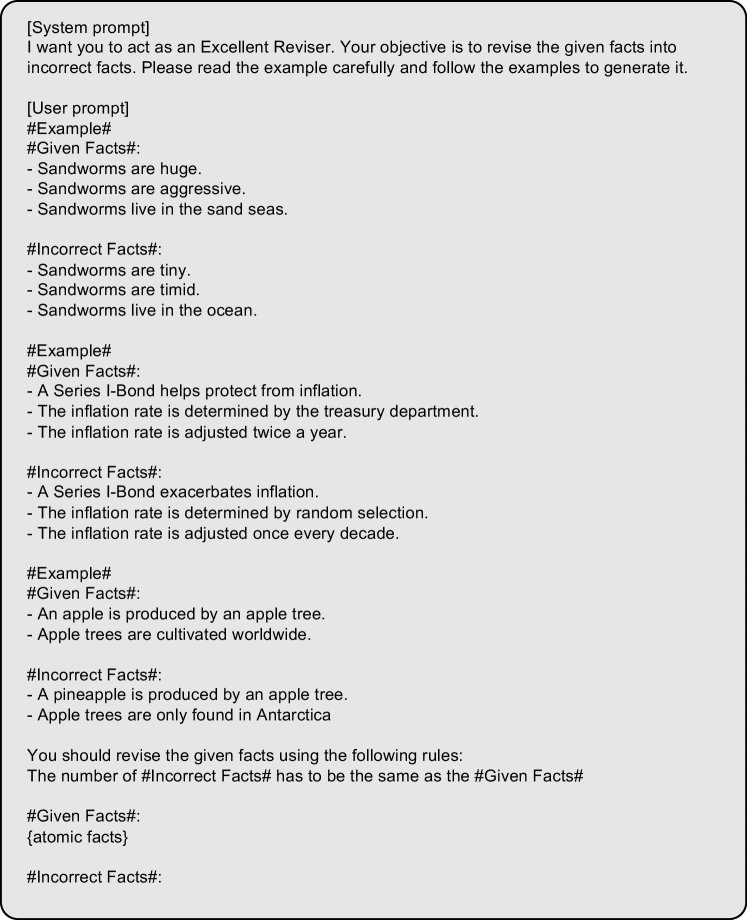
D.2 Reliability of atomic knowledge extraction
To evaluate the reliability of atomic knowledge extraction, we first sample 50 instances of genericQA dataset Dolly. We manually checked these data and find that only 3 instances required further separation or merging of atomic facts, illustrating the reliability of extracting atomic facts using gpt3.5-turbo.
D.3 Training
During the training phase, the AdamW optimizer Loshchilov and Hutter (2019) is utilized with initial learning rates of for SFT and for DPO. The batch sizes for SFT and DPO are set to 32 and 16, respectively, with SFT undergoing 3 epochs of training and DPO 1 epoch. The filtering and deleting percentages, and , are both fixed at 0.5. The scalar weighting hyperparameter is set to 0.2. We determine the hyperparameters through pilot experiments. Training leverages PEFT Mangrulkar et al. (2022), LLaMA-Factory Zheng et al. (2024b) and LoRA Hu et al. (2022).
D.4 Cost Analysis
The cost of KnowTuning is lower than that of the baseline methods RLAIF and FactTune. Specifically, in the generic domain QA dataset Dolly, the costs are as follows: KnowTuning is $8.45, RLAIF is $9.94, and FactTune is $10.53. This cost difference arises because RLAIF necessitates pairwise comparisons for assessing the overall helpfulness of all candidate answers, while FactTune requires a detailed factuality evaluation for each fact across all candidate answers, thereby increasing their dataset comparison construction costs.
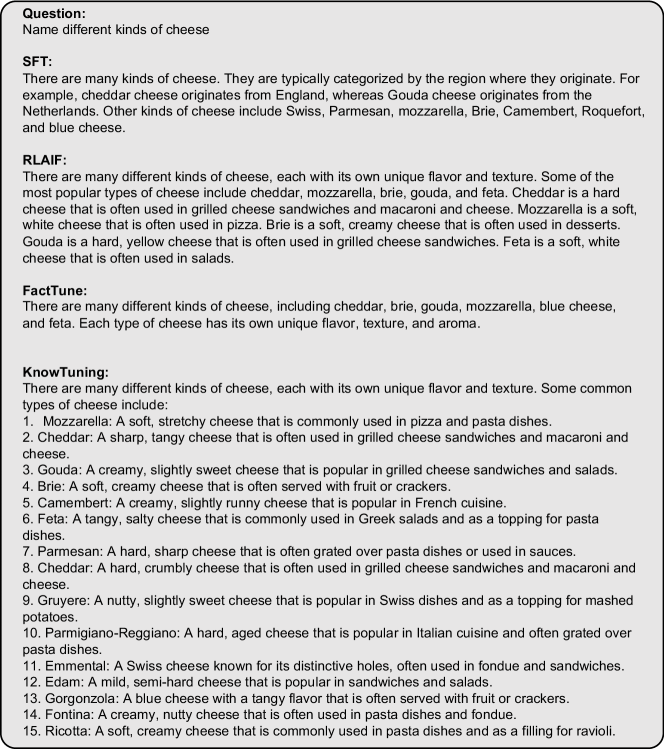
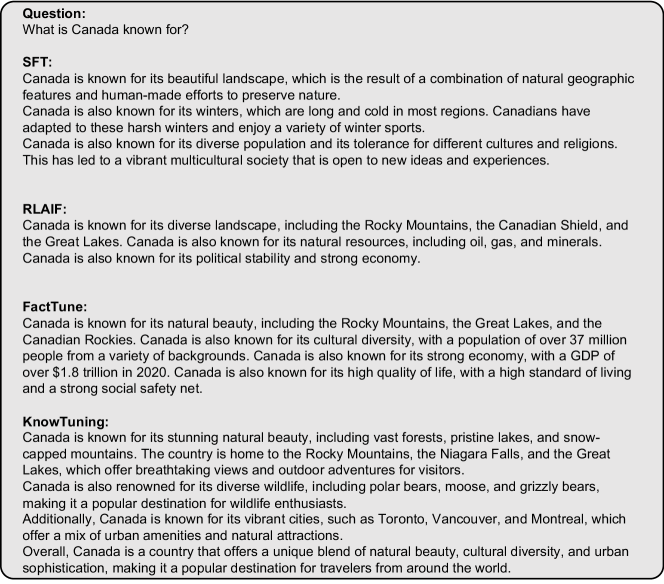
Appendix E Details of Case Study
As illustrated in Figures 9 and 10, the case studies evaluate answers generated by four methods: SFT, RLAIF, FactTune, and KnowTuning across various sizes. Our findings indicate that KnowTuning excels at producing answers that are more complete, factual, and logical across various sizes of LLMs, as detailed below:
-
•
As shown in Figure 9 for the case study based on backbone Llama2-7b-base, KnowTuning generates more complete and logical answers compared to all baselines. Although RLAIF produces more knowledge compared to SFT, it results in fewer logical answers because it does not explicitly focus on logicality optimization. FactTune, on the other hand, focuses on improving the percentage of factualness and performs poorly in terms of answer completeness and logic. This illustrates the need for multiple aspects of coarse-grained knowledge awareness.
-
•
As shown in Figure 10 for the case study based on backbone Llama2-13b-base, KnowTuning generates content that is more informative and factual, and the logic between the knowledge is more logical. Although RLAIF generates multiple aspects of knowledge, it does not provide fine-grained knowledge in the answer. FactTune generates detailed information such as Canada’s domestic population and GDP, but it provides factually incorrect information. This further underscores the critical need for enhanced fine-grained knowledge awareness.