Interpretable Visual Question Answering
Referring to Outside Knowledge
Abstract
We present a novel multimodal interpretable VQA model that can answer the question more accurately and generate diverse explanations. Although researchers have proposed several methods that can generate human-readable and fine-grained natural language sentences to explain a model’s decision, these methods have focused solely on the information in the image. Ideally, the model should refer to various information inside and outside the image to correctly generate explanations, just as we use background knowledge daily. The proposed method incorporates information from outside knowledge and multiple image captions to increase the diversity of information available to the model. The contribution of this paper is to construct an interpretable visual question answering model using multimodal inputs to improve the rationality of generated results. Experimental results show that our model can outperform state-of-the-art methods regarding answer accuracy and explanation rationality.
Index Terms— Visual question answering, interpretable machine learning, outside knowledge learning, natural language explanations.
1 Introduction
Visual Question Answering (VQA) [1] has been widely used in various scenarios such as defect detection [2] and medical diagnosis [3]. Since these applications are closely related to the safety and property of our life, there are high requirements for the reliability of the VQA methods. Although most present methods have demonstrated exemplary performance, they are considered black boxes, as their internal logic and workings are concealed from users, which cannot meet the reliability requirements. It is considered that providing a reasonable explanation for the prediction process can become one of the solutions to prove the model’s reliability. Therefore, new regulations have been created to establish standards for verifying mandatory decisions, which increases the demand for methods’ interpretability [4].
Most conventional interpretable VQA models use a heat map to represent the different attention parts of the model in an image. Although this representation can explain the prediction somewhat, it can still be abstract to users. To solve this problem, Park et al. [5] and Wu et al. [6] have proposed interpretable VQA models to generate explanations with human-readable and detailed natural language sentences. These methods require creating another model that generates explanations for the VQA model. Due to the generation of explanations and answers being independent, it is difficult to control whether the generated explanation conforms to the logic used by the VQA model. Recently, pre-trained large-scale models such as the Bidirectional Encoder Representations from Transformers (BERT) [7], GPT-2 [8], and the Contrastive Language-Image Pre-training (CLIP) [9] have been developed rapidly, which makes it possible to generate natural language more correctly. Focusing on the deficiency of the previous models, Sammani et al. [10], Marasovic et al. [11], and Kayser et al. [12] provided intrinsic interpretability models, which used a single model to generate answers and explanations simultaneously.
Although these methods ensure the underlying logic’s consistency, the generated results’ performance is still flawed.
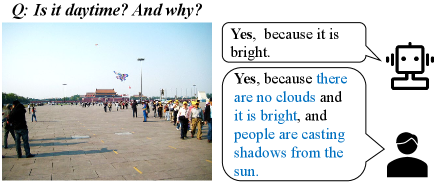
As shown in Fig. 1, human-provided explanations are more complex and informative than previous interpretable methods. The reason is that answering a question in our daily lives requires drawing on a wide range of knowledge and experience beyond just what we can see and recognize in an image. For example, people have a wealth of background knowledge in and outside the image and usually refer to them to explain an answer. Hence, simply referring to visual information to answer questions is still insufficient. As Zhu et al. [13] demonstrated, incorporating image captions as an additional modality is a valuable extension. However, their approach of introducing metadata to the model’s input does not align with the VQA task’s requirement of only image input. Additionally, the utilization of a single caption suffers from the limitation that many captions are irrelevant to the problem, and the provided information only pertains to the image’s interior. As a result, this strategy fails to address the issue of single-source information and leads to a monotonous interpretation. Outside knowledge is always considered to contain information outside the image. Thus, to solve the above informativeness problem, outside knowledge should be introduced to improve the model’s performance. Moreover, we need to use caption generation models to generate multiple captions to introduce more helpful information without changing the model inputs.
This paper proposes a novel multimodal interpretable VQA model that can refer to various information while generating results by introducing outside knowledge and multiple image captions. Concretely, we newly generate the image’s caption and use the generated content to obtain the most relevant outside knowledge from the wikidata [14]. In the proposed multimodal method, we use a joint vector consisting of the features of the image, its caption, and outside knowledge to resolve the conflict between different domains. By leveraging the novel joint vector, our model can draw on a variety of information sources to produce more rational explanations and more accurate answers. Finally, we summarize our contributions of this paper as follows.
-
•
We newly introduce the outside knowledge and multiple image captions to the multimodal interpretable VQA model to solve the informativeness problem.
-
•
Our method outperforms other state-of-the-art models, as evidenced by experimental results that demonstrate more accurate answers and more comprehensive and reasonable explanations.
2 NATURAL LANGUAGE EXPLANATION GENERATION VIA OUTSIDE-KNOWLEDGE AND MULTIPLE CAPTIONS
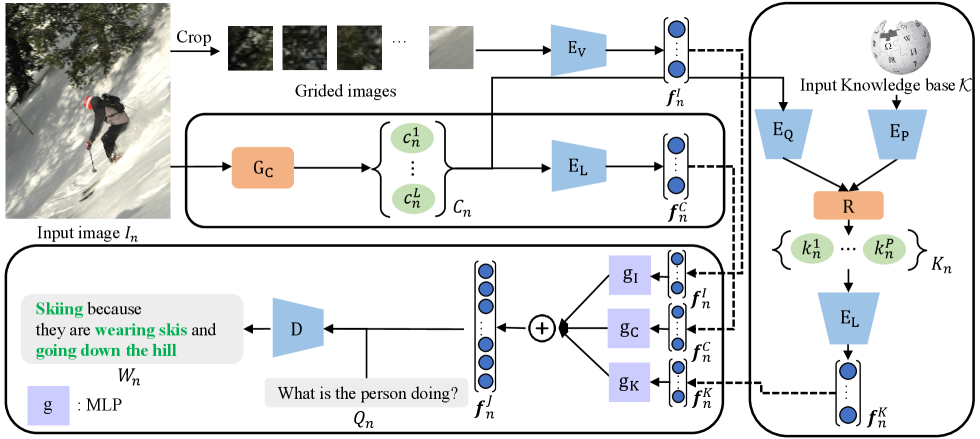
We provide an overview of our model in Fig. 2. Compared with the previous models with separate answering and explaining modules, we propose a VQA model that simultaneously answers questions and generates explanations. To improve the rationality of the generated explanations and the answer accuracy, instead of only using the input image, our model newly refers to the information contained in the retrieved outside knowledge and multiple image captions.
2.1 Image Feature Extraction
Explanation | Answer | ||||||||
BLEU-1 | BLEU-2 | BLEU-3 | BLEU-4 | ROUGE-L | METEOR | CIDEr | SPICE | Acc. | |
NLX-GPT [10] | 64.0 | 48.6 | 36.4 | 27.2 | 50.7 | 22.6 | 104.7 | 21.6 | 67.1 |
E-ViL [12] | 52.6 | 36.6 | 24.9 | 17.2 | 40.3 | 19.0 | 51.8 | 15.7 | 56.8 |
QA-only [11] | 59.3 | 44.0 | 32.3 | 23.9 | 47.4 | 20.6 | 91.8 | 17.9 | 63.4 |
RVT [11] | 59.4 | 43.4 | 31.1 | 22.3 | 46.6 | 20.1 | 84.4 | 17.3 | 62.7 |
Ours w/o OK | 64.0 | 49.1 | 37.2 | 28.3 | 51.3 | 23.1 | 109.8 | 21.3 | 68.5 |
Ours w/o C | 63.9 | 48.7 | 36.7 | 27.6 | 51.1 | 22.6 | 106.1 | 21.4 | 67.4 |
Ours (via BERT) | 64.3 | 49.0 | 37.1 | 28.1 | 51.3 | 23.2 | 108.0 | 21.3 | 67.3 |
Ours | 65.2 | 49.8 | 37.4 | 28.3 | 51.6 | 23.2 | 108.1 | 21.6 | 69.5 |
For each of training data instances, we denote it as -th instance (). Our method takes an image , a question , and a ground truth sentence . Then we generate an output sentence of length , where refers to -th word in the sentence, which contains both the answer and explanation.
We clip the image before extracting the feature as ( being the size of the grid) small pieces, which are represented as . Then we use a transformer-based vision encoder to extract the feature of the input image. The image feature can be calculated as follows:
(1) |
Through the encoder with an attention structure, we can extract image features and capture the relationship between regions in , which is the crucial information to answer a question and generate an explanation.
2.2 Introduction of Image Caption and Outside Knowledge
A knowledge base containing various types of knowledge is needed to retrieve the outside knowledge of images. We set our sights on Wikipedia, which collects a large amount of structured data and has become a resource of enormous value with potential applications. We finally chose a recently proposed subset from Wikidata [14] following KAT [15] as our outside knowledge set in this paper.
Since the image caption and the outside knowledge are natural language contents, we use a language encoder to extract their features in the model. We first use a pre-trained image captioning model to generate a caption set containing captions from the . The features of each caption in are extracted using , and then the obtained features are joined into a caption feature vector by summing. Next, we use a retrieval model and the query to retrieve the knowledge item set containing retrieved knowledge items from .
We use a query encoder and a passage encoder to encode and , respectively, and then use the extracted features to get the retrieved outside knowledge by using the retrieval model , which can be calculated as follows:
(2) |
To introduce the outside knowledge into the final prediction process, we extract the outside knowledge feature vector using the in the same way as extracting .
In this way, we extract the feature vectors of the image caption and outside knowledge containing the internal and external information of the image, which will then be joined with the vision vector in the final prediction process.
2.3 Generation of Answer and Explanation
We use a joint vector to expand the information because feature fusion is an essential step in multimodal tasks [16]. Because of the characteristics of our vision and language encoder, the image and language features are mapped to latent shared spaces. The concatenating operation allows us to use their features best and make our model contain much information. However, even if the extracted features are in a shared space, the simple concatenation between the different modalities will still lead to difficulties in model training. Thus, we use Multi-layer Perceptron (MLP) layers to better fuse the features with considering the combination effect and learning efficiency. First, three different MLPs, , and , are used to process different reference information separately. Then we use the concatenation operation to generate the joint vector as follows:
(3) |
Through this feature fusion process, we can join the feature vectors of the different modalities to preserve information as much as possible.
The output of our model is a sentence , which contains the answer to question and the corresponding explanation. We use a decoder to decode to generate the context: as follows:
(4) |
where is the input, and the decoder generates the answer and the explanation . To train the model, we employ the cross-entropy loss and minimize the negative log-likelihood, which can be computed as
(5) |
The probability mass function is represented by , where is a parameter of the model distribution. The term refers to the words preceding . By minimizing the loss , our model can produce accurate explanations that closely resemble the ground truth.
3 EXPERIMENTS
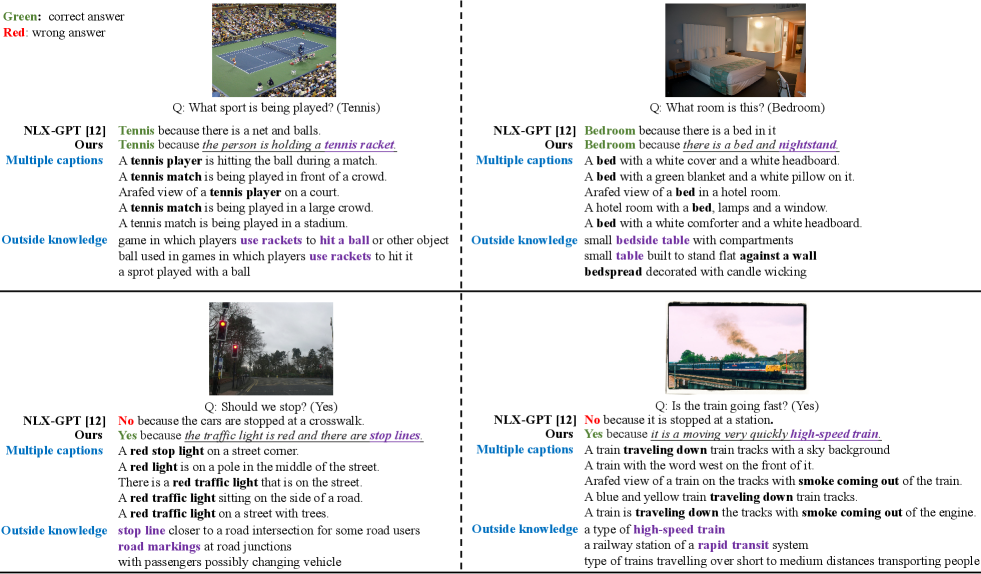
3.1 Experimental Settings
. For our experiments, we utilized the VQA-X dataset [5], an extension of the VQA-v2 dataset [1], which included explanations for each answer. The dataset comprises 33k QA pairs from 28k images sourced from the COCO2014 dataset [17]. For the dataset division, we used all the images in the COCO2014 training set with 29k QA pairs as our training set. We divided the COCO2014 validation set into our validation set and test set according to the proportion of 3:4, which contains 1.5k QA pairs and 2k QA pairs, respectively.
Compared to traditional vision models with specific tasks such as image classification and image segmentation, for the vision encoder, we only rely on their primary network function to output simple grid features rather than their time-consuming top-down features. Thus, to better adapt to the Vision & Language task, we used the CLIP based on the structure of the vision transformer as the vision encoder. The CLIP makes the fusion of vision and language features easier by encoding them in the same hidden space. After experimentally verifying two of the most widely used pre-trained language models, BERT and CLIP-text, for the language encoder, we selected the CLIP-text model, which can better match the vision and language features. We used five different models to generate multiple captions containing various information, including GIT-large [18] fine-tuned on COCO, GIT-large [18] fine-tuned on TextCaps, BLIP [19], CoCa [20] and BLIP-2 [21]. In the outside knowledge retrieval, we used the same query and passage encoder as Dense Passage Retrieval [22] and the Faiss [23] to realize the retrieval process.
All the MLPs in our model have three layers and a 128 hidden size, and we set the caption number to 5 and the outside knowledge item number to 3. During the training, we resized all images to 224 224 pixels and added random flips. We trained our models for 30 epochs using a batch size of 32 and a learning rate of , which was decreased to .
. The comparison methods answer the question and generate natural language explanations based only on the input image. The NLX-GPT [10] and E-ViL [12] have separate answering process and explanation process, and RVT and QA-only [11] combine the two processes.
. We used the accuracy to evaluate the generated answers and used the following common language modeling evaluation metrics: BLEU-n (n being 1 to 4) [24], METEOR [25], ROUGE [26], SPICE [27] and CIDEr [28]. All the scores of the language metrics were computed by the publicly available code provided by Chen et al. [29].
3.2 Experimental Results
As shown in Table 1, the obtained results prove that our model performs better than the comparison methods. Since introducing multiple references, we conducted ablation experiments to verify the validity of each reference information and the language encoder selection. Specifically, we experimented separately with models that only introduce outside knowledge (“Ours w/o C”) and caption (“Ours w/o OK”). Experimental results show that models that introduce captions or outside knowledge as additional references perform better than models that just refer to images. However, the model that uses captions and outside knowledge (“Ours”) performs best. These results prove that if the model has more information to refer to, it can generate answers and their corresponding explanations more correctly. The results of “Ours (via BERT)” also show that using the same language encoder CLIP-text model as the vision encoder can better align language and vision features and generate better results than the BERT.
We show the qualitative comparison between our model with the state-of-the-art method, NLX-GPT, in Fig. 3. We have bolded in black and purple some critical information in the caption and outside knowledge that is helpful for the results, respectively. We can see that this information is used for the generated answers and explanations. As shown in the example in the first row, with the same correct answer, we refer to more information. Thus, our approach yields a more detailed and reasonable explanation. Meanwhile, in the second row, our method correctly answers questions that the comparison model cannot answer because of the reference to the additional outside knowledge and image caption.
4 CONCLUSION
By incorporating multimodal reference information and advanced interpretability techniques, our VQA model generates highly precise explanations. The proposed method can solve the previous informativeness problem by additionally referring to the image caption and outside knowledge. The questions answered by our model were also more accurate. The qualitative and quantitative assessment results demonstrate that our approach for generating answers and explanations surpasses several state-of-the-art methods.
References
- [1] Stanislaw Antol, Aishwarya Agrawal, Jiasen Lu, Margaret Mitchell, Dhruv Batra, C Lawrence Zitnick, and Devi Parikh, “VQA: Visual question answering,” in Proceedings of the IEEE International Conference on Computer Vision, 2015, pp. 2425–2433.
- [2] Tamás Czimmermann and others., “Visual-based defect detection and classification approaches for industrial applications—a survey,” Sensors, p. 1459, 2020.
- [3] Zhihong Lin, Donghao Zhang, Qingyi Tac, Danli Shi, Gholamreza Haffari, Qi Wu, Mingguang He, and Zongyuan Ge, “Medical visual question answering: A survey,” arXiv preprint arXiv:2111.10056, 2021.
- [4] Christoph Molnar, Interpretable machine learning: A Guide for Making Black Box Models Explainable, Available online: https://christophm.github.io/interpretable-ml-book/, 2020.
- [5] Park et al., “Multimodal explanations: Justifying decisions and pointing to the evidence,” in Proceedings of the IEEE Conference on Conference Vision and Pattern Recognition, 2018, pp. 8779–8788.
- [6] Jialin Wu and Raymond Mooney, “Faithful multimodal explanation for visual question answering,” in Proceedings of the ACL Workshop Blackbox NLP: Analyzing and Interpreting Neural Networks for NLP, 2019, pp. 103–112.
- [7] Jacob Devlin, Ming-Wei Chang, Kenton Lee, and Kristina Toutanova, “Bert: Pre-training of deep bidirectional transformers for language understanding,” arXiv preprint arXiv:1810.04805, 2018.
- [8] Alec Radford, Jeffrey Wu, Rewon Child, David Luan, Dario Amodei, Ilya Sutskever, et al., “Language models are unsupervised multitask learners,” OpenAI Blog, vol. 1, no. 8, pp. 9, 2019.
- [9] Alec Radford, Jong Wook Kim, Chris Hallacy, Ramesh, et al., “Learning transferable visual models from natural language supervision,” in Proceedings of the International Conference on Machine Learning, 2021, pp. 8748–8763.
- [10] Fawaz Sammani, Tanmoy Mukherjee, and Nikos Deligiannis, “NLX-GPT: A model for natural language explanations in vision and vision-language tasks,” in Proceedings of the IEEE Conference on Computer Vision and Pattern Recognition, 2022, pp. 8322–8332.
- [11] Ana Marasović et al., “Natural language rationales with full-stack visual reasoning: From pixels to semantic frames to commonsense graphs,” in Proceedings of the Findings of the Association for Computational Linguistics: EMNLP, 2020, pp. 2810–2829.
- [12] Kayser et al., “E-ViL: A dataset and benchmark for natural language explanations in vision-language tasks,” in Proceedings of the IEEE International Conference on Computer Vision, 2021, pp. 1244–1254.
- [13] He Zhu, Ren Togo, Takahiro Ogawa, and Miki Haseyama, “A multimodal interpretable visual question answering model introducing image caption processor,” in Proceedings of the IEEE Global Conference on Consumer Electronics, 2022, pp. 805–806.
- [14] Denny Vrandečić and Markus Krötzsch, “Wikidata: a free collaborative knowledgebase,” Communications of the ACM, pp. 78–85, 2014.
- [15] Gui et al., “KAT: A knowledge augmented transformer for vision-and-language,” arXiv preprint arXiv:2112.08614, 2021.
- [16] Yimian Dai, Fabian Gieseke, Stefan Oehmcke, Yiquan Wu, and Kobus Barnard, “Attentional feature fusion,” in Proceedings of the IEEE Winter Conference on Applications of Computer Vision, 2021, pp. 3560–3569.
- [17] Tsung-Yi Lin, Michael Maire, Serge Belongie, Hays, et al., “Microsoft COCO: Common objects in context,” in Proceedings of the European Conference on Computer Vision, 2014, pp. 740–755.
- [18] Jianfeng Wang et al., “GIT: A generative image-to-text transformer for vision and language,” Transactions on Machine Learning Research, 2022.
- [19] Junnan Li, Dongxu Li, Caiming Xiong, and Steven Hoi, “Blip: Bootstrapping language-image pre-training for unified vision-language understanding and generation,” in Proceedings of the International Conference on Machine Learning. PMLR, 2022, pp. 12888–12900.
- [20] Jiahui Yu, Zirui Wang, Vijay Vasudevan, Legg Yeung, Mojtaba Seyedhosseini, and Yonghui Wu, “Coca: Contrastive captioners are image-text foundation models,” Transactions on Machine Learning Research, 2022.
- [21] Junnan Li, Dongxu Li, Silvio Savarese, and Steven Hoi, “Blip-2: Bootstrapping language-image pre-training with frozen image encoders and large language models,” arXiv preprint arXiv:2301.12597, 2023.
- [22] Vladimir Karpukhin, Barlas Oğuz, Sewon Min, Patrick Lewis, Ledell Wu, Sergey Edunov, Danqi Chen, and Wen-tau Yih, “Dense passage retrieval for open-domain question answering,” arXiv preprint arXiv:2004.04906, 2020.
- [23] Jeff Johnson, Matthijs Douze, and Hervé Jégou, “Billion-scale similarity search with GPUs,” IEEE Transactions on Big Data, pp. 535–547, 2019.
- [24] Papineni et al., “BLEU: a method for automatic evaluation of machine translation,” in Proceedings of the Annual Meeting of the Association for Computational Linguistics, 2002, pp. 311–318.
- [25] Satanjeev Banerjee and Alon Lavie, “METEOR: An automatic metric for mt evaluation with improved correlation with human judgments,” in Proceedings of the ACL workshop on Intrinsic and Extrinsic Evaluation Measures for Machine Translation and/or Summarization, 2005, pp. 65–72.
- [26] Chin-Yew Lin and Eduard Hovy, “Automatic evaluation of summaries using n-gram co-occurrence statistics,” in Proceedings of the Human Language Technology Conference of the North American Chapter of the Association for Computational linguistics, 2003, pp. 150–157.
- [27] Peter Anderson, Basura Fernando, Mark Johnson, and Stephen Gould, “SPICE: Semantic propositional image caption evaluation,” in Proceedings of the European Conference on Conference Vision, 2016, pp. 382–398.
- [28] Ramakrishna Vedantam, C Lawrence Zitnick, and Devi Parikh, “CIDEr: Consensus-based image description evaluation,” in Proceedings of the IEEE Conference on Computer Vision and Pattern Recognition, 2015, pp. 4566–4575.
- [29] Xinlei Chen, Hao Fang, Tsung-Yi Lin, Ramakrishna Vedantam, Saurabh Gupta, Piotr Dollár, and C Lawrence Zitnick, “Microsoft COCO captions: Data collection and evaluation server,” arXiv preprint arXiv:1504.00325, 2015.