Interpretable Embedding for Ad-Hoc Video Search
Abstract.
Answering query with semantic concepts has long been the mainstream approach for video search. Until recently, its performance is surpassed by concept-free approach, which embeds queries in a joint space as videos. Nevertheless, the embedded features as well as search results are not interpretable, hindering subsequent steps in video browsing and query reformulation. This paper integrates feature embedding and concept interpretation into a neural network for unified dual-task learning. In this way, an embedding is associated with a list of semantic concepts as an interpretation of video content. This paper empirically demonstrates that, by using either the embedding features or concepts, considerable search improvement is attainable on TRECVid benchmarked datasets. Concepts are not only effective in pruning false positive videos, but also highly complementary to concept-free search, leading to large margin of improvement compared to state-of-the-art approaches.
1. Introduction
Ad-hoc video search (AVS) is to retrieve video segments for textual queries. As no visual example is provided along with the textual query, AVS is also known as zero-example video retrieval. The task is challenging due to the semantic gap between the high-level semantics expressed in terms of textual description and the low-level signals embedded in videos. Furthermore, AVS is ad-hoc for assuming the scenario of open vocabulary, where the composition of a query using words or concepts new to a search engine is allowed. In the literature, TRECVid AVS is the most known activity that conducts benchmark evaluation for this task (Awad et al., 2016). The evaluation is conducted on a large video collection, e.g., V3C1 corpus (Berns et al., 2019) composed of one million video segments, with no training data or labels being provided. The testing query is non-verbose, e.g., Find shots of a woman wearing a red dress outside in the daytime. Achieving satisfactory performance in face of large datasets, short query information and open vocabulary problem is certainly difficult, as evidenced from the evaluation results of TRECVid on automatic search (Awad et al., 2016, 2018b, 2019, 2018a).
The mainstream approaches are devoted to query understanding, either relying on concept classifiers (i.e., concept-based (Ueki et al., 2017; Snoek et al., 2017; Avgerinakis et al., 2018; Wei and Ngo, 2007)) or learning embedding features common to text and video (i.e., concept-free (Li et al., 2019a; Dong et al., 2019; Wu et al., 2019)) for search. Concept-based approach explicitly maps a user query to concept tokens. Capitalizing on the pre-trained convolutional neural network (CNN), a variety of concepts (e.g., object, action, scene) in videos are indexed and then matched against the tokens extracted from query description. As similarity is semantically enumerated based on their common concepts, the search result is explainable. The progress of concept-based search is bottlenecked by a number of issues, including the selection of concepts for query (Ueki et al., 2017) and the reliability of concept classifiers (Snoek et al., 2017). Concept-free approach, in contrast, bypasses these issues and performs matching between query and video by their embedded features. The features are learnt in a black box manner by minimizing the distances of video-text pairs. As features are not interpretable, the result of matching cannot be unrolled to recount the commonality between video-text pairs as in concept-based search.
The success of concept-based approach for AVS has been evidenced in (Snoek et al., 2017; Ueki et al., 2017), by building a large visual vocabulary to accommodate for tens of thousands of semantic concepts. Screening these concepts to represent user queries, however, is not a trivial issue. Human intervention is often required. As studied in interactive search (Lokoč et al., 2018), human is excellent in picking the right concepts for video search. Concept-free approach, which is relieved from the burden of concept screening, has recently surpassed the retrieval performance of concept-based approach in automatic search (Awad et al., 2018b, 2019; Li et al., 2019a). Nevertheless, being not interpretable, its utility for browsing is questionable when user inspects the search result. Unlike concept-based search, there is also no explicit way to identify the mismatch of a video from the query when the performance is not satisfying.
While both concept-based and concept-free approaches have their respective merit and limitation, there is no research studying the complementarity between them. This paper proposes a new network architecture to equip concept-free approach with the capability of inferring concepts from an embedded feature. The network performs dual tasks, i.e., learning cross-modal embedding features while enforcing the features to explicitly recount the concepts underlying a video. The network is trained end-to-end to force the consistency between features and concepts. This results in each video being associated with an embedding feature for search and a concept list for interpretation. Empirically, this paper shows that, by combining both features and concepts for search, superior performance is attainable on the TRECVid benchmarked datasets. The main contribution of this paper is the proposal of a new architecture along with its novel loss function for concept decoding. This paper also paves new insights of how concept-free and concept-based approaches can possibly see eye-to-eye for a number of issues in AVS. These issues include using the decoded concepts to verify the result of concept-free search, and the ability of handling Boolean queries.
2. Related Work
Ad-hoc video search, being a long-lasting task annually evaluated in TRECVid, can date back to as early as year 2003 (Smeaton et al., 2006). The task is, presented with a topic (i.e., textual descriptions of information need), a search system formulates query and then returns a ranked list of video shots. Since its beginning, the utilization of concepts for search has become the main focus of study (Snoek and Worring, 2009). The progress evolves from the early efforts of concept bank development and ontology reasoning (Naphade et al., 2006; Jiang et al., 2010; Snoek et al., 2006) to the recent advances in concept screening, representation and combination (Nguyen et al., 2016; Avgerinakis et al., 2018; Lu et al., 2016; Ueki et al., 2018; Huang et al., 2018). This branch of approaches is generally referred to as concept-based search. The most recent approaches mostly focus on the linguistic analysis for concept selection on large vocabulary (Ueki et al., 2018; Huang et al., 2018). To deal with out-of-vocabulary (OOV) problem, query expansion with ontology influencing (Ueki et al., 2018) and webly-label learning by crawling online visual data (Huang et al., 2018) are commonly adopted. Despite numerous progress (Boer et al., 2017; Jiang et al., 2015; Nguyen et al., 2019), automatic selection of concepts to capture query semantics as well as context remains highly difficult. Human intervention is often required in practice, for example, by manually removing undesired concepts after automatic matching (Nguyen et al., 2018) or by hand-picking query phrases that should be matched with concepts (Ueki et al., 2019).
Concept-based search is superior in finding videos when the concepts required for a query topic can be precisely identified for search. The associated problem, however, is the inherent expression ambiguity when using a sparse list of concepts to describe a complex information need. For example, a topic of Find shots of a person holding a tool and cutting something is hard to be expressed precisely using the concepts like “holding”, “cutting”, and “tool”, especially if these concepts are treated independently during search. To this end, concept-free search, which embeds the entire video shot and information need as high-dimensional features, is recently shown to be more effective in capturing compositional concepts (Li et al., 2019a; Dong et al., 2019; Huang et al., 2018). Nevertheless, the performance of concept-free search is not always predictable. For example, the queries Find shots of people queuing and Find shots of people standing in line, despite being similar in meaning, can end up in considerably different retrieval performances.
With the availability of image and video captioning datasets (e.g., MSCOCO (Chen et al., 2015), MSVD (Chen and Dolan, 2011), MSR-VTT (Xu et al., 2016)), the training of visual-text embedding models is greatly facilitated. Various models have been attempted for AVS, including VideoStory (Habibian et al., 2014), visual semantic embedding (VSE++) (Faghri et al., 2018), intra-modal and inter-modal attention networks (Huang et al., 2018), Word2VisualVec (W2VV) (Dong et al., 2018) and dual encoding (Dong et al., 2019). These models differ mainly in ways of how query topics are represented and encoded. For instance, VSE++ employs a recurrent network to encode the word sequence (Faghri et al., 2018), while attention networks weight the text and visual elements for embedding (Huang et al., 2018). More sophisticated approach is W2VV (Dong et al., 2018), which encodes bag-of-words, word2vec and word sequence altogether. The extension of W2VV (W2VV++) is the first model that shows significant improvement in AVS over the concept-based approaches (Awad et al., 2018b). Building on top of W2VV++, the most recent dual encoding network (Dong et al., 2019) reports state-of-the-art performance by multi-level encoding. Three different levels of information are considered, including the short-term pooling of local features and mean pooling of word and sequence features respectively. Different from other models, the network treats a video frame as a word and processes video shot in a similar fashion as a text sequence. Similar approach is adopted in (Wu et al., 2019), with the use of graph convolutional network and VLAD for encoding.
A hybrid of concept-based and concept-free approaches has also been studied (Nguyen et al., 2017; Huang et al., 2018; Ueki et al., 2018, 2019; Francis et al., 2019; Shirahama et al., 2019; Snoek et al., 2017). The early works include the fusion of VideoStory embedding and concept features (Snoek et al., 2017), which leads to improvement over individual features. In the recent TRECVid benchmarking, late fusion of concept-based and concept-free approaches has become a norm (Nguyen et al., 2017; Huang et al., 2018; Ueki et al., 2018, 2019; Francis et al., 2019; Shirahama et al., 2019). Although being shown to be complementary, the fact that both features are generated using two separate models trained using different forms of data has tremendously increased the system complexity. Different from these works, the proposed dual-task model is end-to-end trained using the same set of data for generating both embedding and concept features. This results in significant save of effort in training and housekeeping models, while maintaining the consistency between two different natures of features.
3. Dual-Task Network
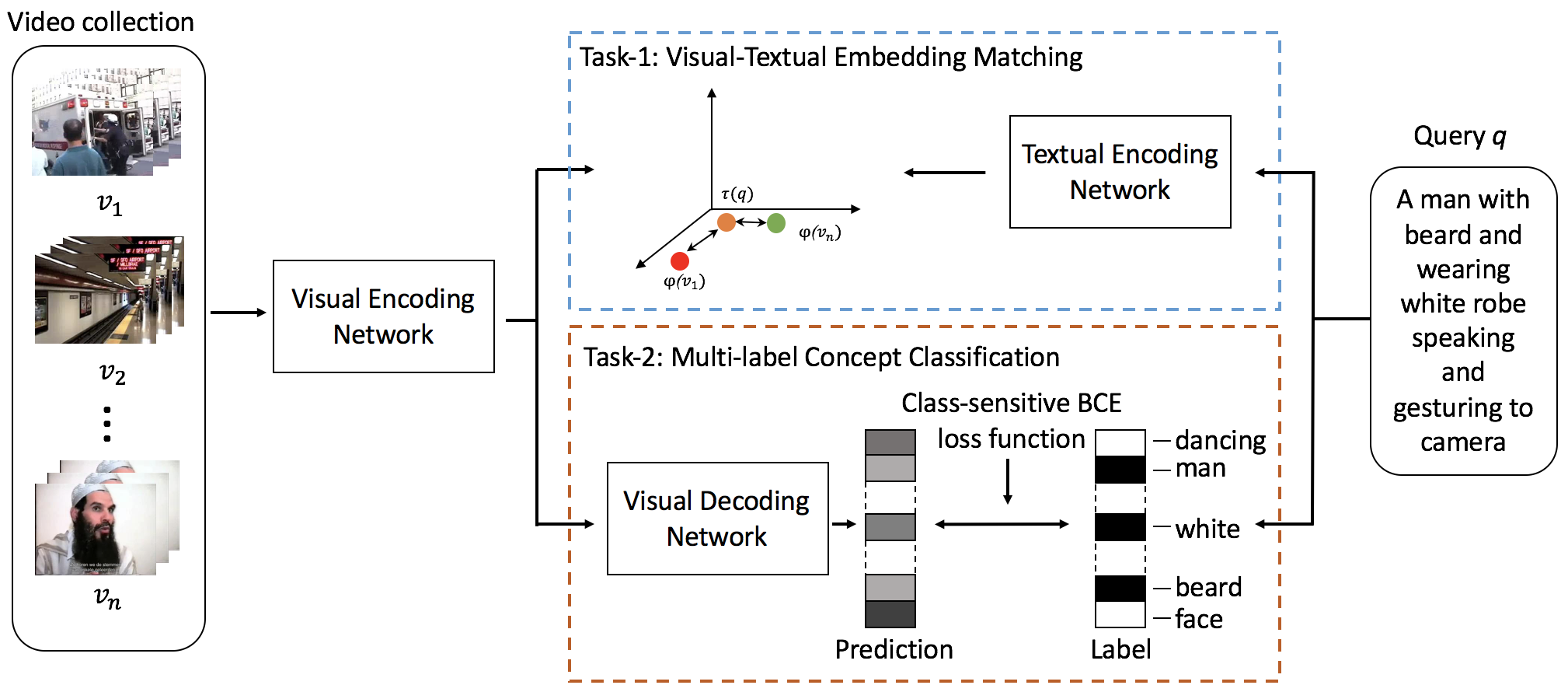
Figure 1 illustrates the architecture of dual-task network, which is composed of visual-textual embedding (Task-1) and multi-label concept classification (Task-2). Both tasks share visual encoding network for video feature extraction. Taking user query as input, Task-1 trains a textual encoding network to project the query into the same latent space as video features. Task-2, on the other hand, trains a visual decoding network to predict the concepts underlying a video feature. Given a video-text pair, Task-1 aims to minimize the pairwise distance, while Task-2 is to recover the query words from the video embedding. It is worth noting that a query usually describes only one perspective of video content. Hence, the loss function of decoder is novelly designed to emphasize more on missing query words than the words outside of a query. To this end, as both tasks are learnt simultaneously, the video features are learnt iteratively to accommodate both tasks.
3.1. Visual-text Embedding
We use the same architecture as dual encoding network (Dong et al., 2019) to extract multi-level video and text features. The visual encoding network has three sub-networks. The first sub-network captures the global information of a video segment by mean pooling its CNN features over the video frames. The second sub-network extracts the temporal information of video by mean pooling the features of the bidirectional GRU (Cho et al., 2014) (biGRU) across all time steps. The final sub-network further extracts the local information in the temporal sequence of video by performing 1-d CNN on the top of the biGRU features from the second sub-network. A multi-level video feature is formed by concatenating the global, temporal and local information extracted from these sub-networks. The visual embedding is denoted as
(1) |
where denotes the feature extracted from the first, second and third sub-networks for a video . The video feature is further mapped to the common space of dimensions by using a fully connected layer (FC) and a batch normalization layer (BN) as following
(2) |
where BN() denotes the batch normalized layer. The matrix and the vector are weight and bias of the fully connected layer in the visual encoding network.
The query is encoded similarly using the texture encoding network. Each word in is represented as a one-hot vector and then concatenated as a matrix to represent the sentence . The first level feature is obtained by taking the mean value of the matrix over all the vectors of sentence words. A denser vector is further generated for every word by multiplying its one-hot vector with a pre-trained Word2Vec matrix. The second-level feature of the query is obtained by mean pooling of the features output by each stage of biGRU (Cho et al., 2014) over the sequence of dense vectors. The third-level feature is extracted by conducting 1-d CNN over the biGRU features obtained from the second level. Finally, a multi-level feature for the query is formed by concatenating all of the features as
(3) |
The feature is subsequently mapped to the common space as the video feature by a fully connected layer and a batch normalize layer
(4) |
where and are the weight and bias of the fully connected layer in the textual encoding network. The textual embedding has the same output dimension as visual embedding.
Finally, the similarity of the input query and the video is computed as . The visual-textual embedding matching task is trained in an end-to-end manner by using improved marginal ranking loss (Faghri et al., 2018). The loss in this matching task is defined as
(5) | |||
where is the margin. The visual-text pair is indicated by the notations and respectively. The query is associated with a negative sample , and similarly is associated with a negative query . The visual-textual embedding matching task is trained to minimize this loss.
3.2. Multi-label Concept Decoding
Video content is multi-perspective. The content can be narrated from different perspectives depending on the subjects or contexts of interest in the topic of discussion. Even for one perspective, there exists a variety of ways expressing the same semantics using different words. Hence, given a video, Task-2 is to train a decoder such that all the words in its descriptions are activated with high probability. The activated words that are absent from the descriptions do not necessarily belong to false positives. Instead, these words may be just not mentioned than being classified incorrectly. In general, the challenge of training such a decoder is beyond concept prediction as in the conventional CNN. The novelty of Task-2 is the design of a loss function to maximize the intersection between the classified concepts of a video embedding and the words mentioned in the captions.
The visual decoding network is comprised of a fully connected layer and a batch normalization layer. The output is denoted as
(6) |
where and are weight and bias of the network. The sigmoid function is performed over the output to obtain the probability distribution for a video embedding
(7) |
where for numbers of concept classes. The value of is compiled from the training set, by including the words that appear in at least five descriptions and removing the words in the NLTK stopword list. For a video , the words that appear in its captions are labeled as ground-truth , where indicates whether a word is present in the captions of .
Binary cross entropy (BCE) loss is widely adopted for multi-label classification task. However, BCE cannot be directly applied for treating each class equally by penalizing the predictions different from the ground-truth labels. In general, the number of words used to describe a video is much smaller than the total number of concepts. Using BCE will cause the amount of loss being dominated by the words not mentioned in video captions. The decoder trained in this way will play trick to predict almost all classes as zero in order to get an overall low BCE loss. Thus, a class-sensitive loss is proposed, by computing the loss of mentioned words separately from those that do not appear in the captions, as following
(8) | |||
(9) |
Eq. (9) is the BCE loss function that computes the binary cross-entropy for a class . The proposed function in Eq. (8) is the summation of two BCE terms. The first term computes the losses of those classes mentioned in the captions, i.e., in the ground truth of a video. The second term computes the losses of the remaining classes, i.e., . Note that the proportion of concepts involved in the first and second terms is highly imbalanced. Eq. (8) effectively assigns higher weights to the mentioned concepts. In this way, the decoder is trained to play a more active role in maximizing the overlap between the predicted and ground-truth concepts. Nevertheless, to avoid an excessive number of concepts being predicted as positive, a hyper parameter is required to keep a balance between activating mentioned words and suppressing irrelevant concepts. When equals to 1 or 0, it represents the extreme case of whether to penalize only the concepts being mentioned (i.e., ) or vice versa (i.e., ). In practice, the value of should bias towards the second term to restrict the number of activated concepts. This is also justified in the experiment, where the value is learnt as , when verified using validation set. The multi-class classification is trained in an end-to-end manner to minimize the .
3.3. Dual-task Learning and Inference
We have designed one loss for each task and they are and . The dual-task loss is set as
(10) |
In the training stage, we compute the average dual-task loss over all input video-text pairs in a batch and update all the parameters of the model simultaneously by using this loss.
In the inference stage, we have two trained models for video search. One model is from the visual-textual embedding matching task (denoted as ) and the other model comes from the multi-label video concept classification task (denoted as ). The embedding model and the concept model have the same parameters in the visual encoding network. For all videos in the collection, we first use the trained visual encoding network to extract their visual embeddings and apply the trained visual decoding network of to get their predicted concepts offline. As a result, each video in the dataset is indexed with an embedding and a predicted concept vector . Given a test query, the embedding model applies its textual encoding network to encode the test query as a textual embedding . Then, will give each video a score () according to the similarity of its embedding with the textual embedding of the query
(11) |
will output a list of videos for the query ranked according to the scores. The top-1 in the list has the highest score which represents the video best matches the input query. Meanwhile, the concept model will generate a one-hot vector for the given test query. It maps the query sentence over the constructed concept list from the classification task to get a one-hot query vector . If , it means that th concept of the concept list is wanted by the query. Then, will also give each video a score ( based on the similarity of its predicted concept vector and the query vector .
(12) |
Another ranked list is output by the concept model based on this score. A linear function is used to combine two models together. We give a combined score () for each video with respect to the input query as
(13) | |||
where is a hyper parameter to control the contributions of the embedding model and the concept model to the final retrieval score. We denote the model which uses the for video search as . Normally, we use the combined model to retrieve videos for an input query in the experiments.
4. Experiments
We begin by describing the datasets, followed by ablation studies to justify the proposed class-sensitive loss function and parameter choices. Performance comparison with state-of-the-art techniques is then presented. Finally, we provide insights on the potential of dual-task model in interpreting search result and answering Boolean queries.
4.1. Experimental Settings
. Table 1 lists the datasets used in the experiments. The proposed model is trained and validated on the captioning datasets. These datasets are also used by other approaches such as W2VV++ (Li et al., 2019a) and dual coding (Dong et al., 2019). The number of captions per video varies from 2 in TV2016TRAIN (Awad et al., 2016) to as many as 40 in MSVD (Chen and Dolan, 2011). The performance of AVS is evaluated on two large video collections. Both are TRECVid benchmarked datasets, where IACC.3 (Awad et al., 2016) is used during the years 2016 to 2018 and V3C1(Berns et al., 2019) in the year 2019. As TRECVid evaluates 30 query topics per year, a total of 120 queries are involved in the evaluation. For convenience, we name the query sets as tv16, tv17, tv18 and tv19, and each set contains the 30 queries being evaluated on that year. The full set of queries is listed in the supplementary document.
name | #video | #caption | #AVS test query |
---|---|---|---|
Training set: | |||
MSR-VTT (Xu et al., 2016) | 10,000 | 200,000 | |
TGIF (Li et al., 2016) | 100,855 | 124,534 | |
Validation set: | |||
TV2016TRAIN (Awad et al., 2016) | 200 | 400 | |
Classification test set: | |||
MSVD (Chen and Dolan, 2011) | 1,907 | 80,837 | |
AVS test set: | |||
IACC.3 (Awad et al., 2016) | 335,944 | 90 | |
V3C1 (Berns et al., 2019) | 1,082,659 | 30 |
. We use extended inferred average precision (xinfAP) to evaluate ad-hoc video search results (Awad et al., 2019). For the overall performance of a search system, we report the mean xinfAP of all test queries as results.
. We use the PyTorch code provided by the dual coding model (Dong et al., 2019) to set up the basic architecture of visual encoding network and textual encoding network. Following (Dong et al., 2019), we set the output dimension of both networks as , and the margin in the triplet loss function (Eq. (5)) as . We use the pre-trained ResNet-152 (Dong et al., 2017) and ResNext-101 (Snoek et al., 2017) to extract a feature of 4,096 dimensions for each video frame. For the visual decoding network, the concept list is composed of words, compiling from the captions of TGIF and MSR-VTT. We use a learning rate of 0.0001 and Adam optimizer to train the model. The batch size is 128. The hyper-parameter is set as (Eq. (8)) based on validation set, and we report (Eq. (13)) as our combined model.
4.2. Ablation Studies
BCE loss. We first compare the proposed class-sensitive loss (Eq. (8)) to the normal BCE in the design of multi-label concept decoder. The evaluation is conducted by counting the number of classified concepts that are mentioned in video captions. On MSVD dataset (Chen and Dolan, 2011) with 1,907 video clips, the proposed BCE loss attains recall@10=0.532. In other words, on average there are more than 50% of concepts in the top-10 list are mentioned by the video captions. This performance is significantly better than the normal BCE loss which only manages to reach recall@10=0.293.
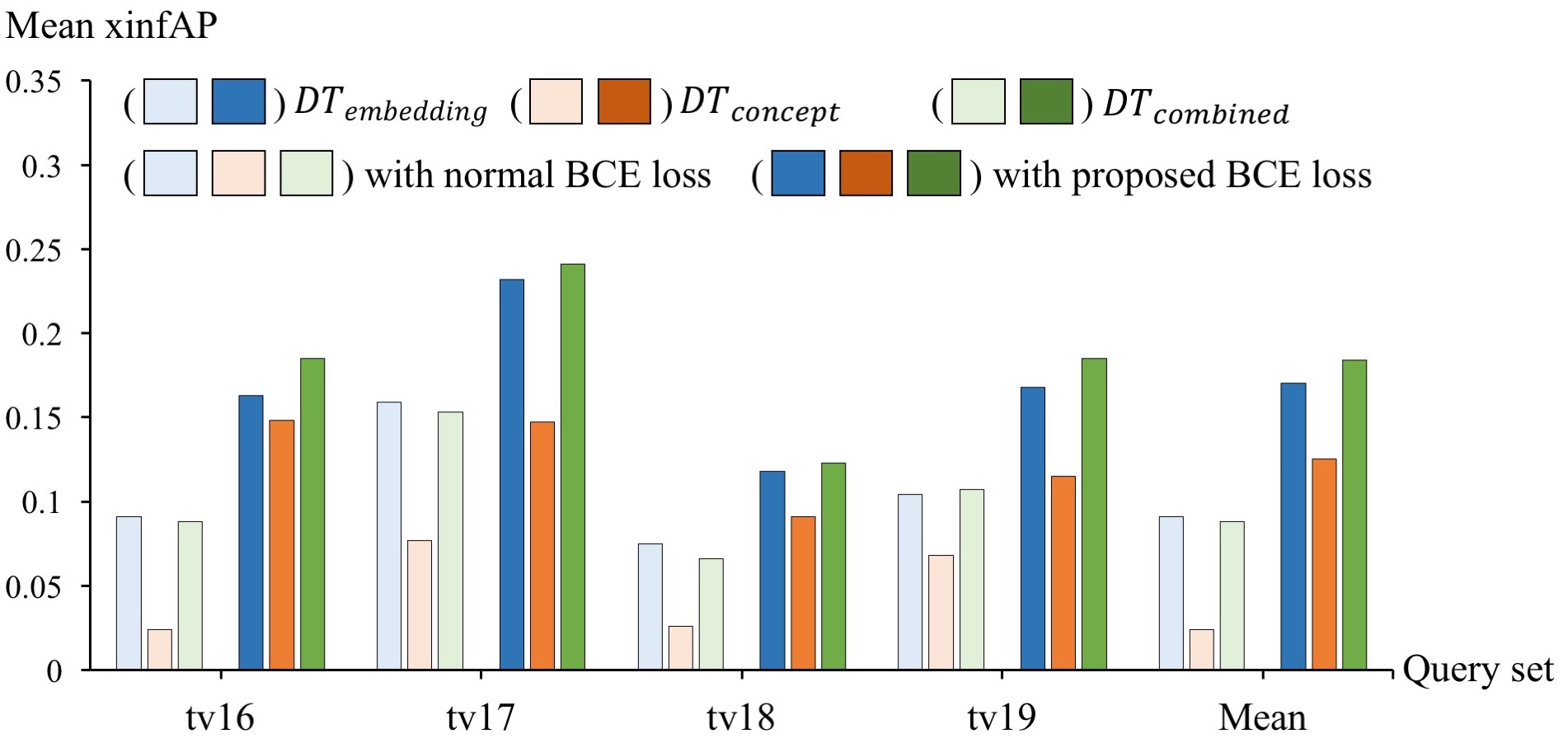
Figure 2 further contrasts the retrieval performances between the proposed class-sensitive and normal loss functions on four sets of TRECVid queries. Note that in the figure, two groups of performances are shown for each query set. Each group separately lists three performances for using embedding feature only (i.e., ), concept only (i.e., ) and late fusion of them (i.e., ). The performances based on the models trained with normal and proposed BCE losses are visualized with bars of lighter and darker colors respectively. As shown in the figure, the models trained with the proposed class-sensitive loss function show consistently better retrieval rate across all the four query sets. For concept-only retrieval, the average performance over 120 queries is five times higher than the model trained with normal BCE. We attribute the performance gain due to higher accuracy in concept prediction. The result indicates the merit of the new BCE loss function for dual-task learning. With class-sensitive loss, the performance of also improves. The performance difference is almost double (xinfAP =0.170) when comparing to the model trained by the normal BCE (xinfAP = 0.091). Furthermore, by using the proposed BCE loss, the late fusion of concept-only and embedding-only features always leads to performance boost across the four query sets. In contrast, the model trained with normal BCE only manages to show improvement on tv19.
Datasets | IACC.3 | V3C1 | |||
---|---|---|---|---|---|
Query sets | tv16 | tv17 | tv18 | tv19 | Mean |
Single-task models: | |||||
0.134 | 0.137 | 0.068 | 0.104 | 0.111 | |
0.156 | 0.222 | 0.115 | 0.160 | 0.163 | |
Dual-task models: | |||||
0.148 | 0.147 | 0.091 | 0.115 | 0.125 | |
0.163 | 0.232 | 0.118 | 0.168 | 0.170 |
Dual-task learning. Next, we verify the merit of dual-task learning versus training two single tasks independently. Specifically, the encoders for Task-1 (visual-text embedding) and the encoder-decoder for Task-2 (multi-level concept classification) are trained separately. For notation convenience, we call the two single-task models as and respectively. As shown in Table 2, consistent improvement is noticeable for dual-task model when performing retrieval using either concept-only or embedding-only feature. improves 69 out of 120 queries over . Using query-556 Find shots of a person wearing a blue shirt as an example, xinfAP is significantly boosted from 0.144 to 0.198. Benefited from dual-task learning, true positives are ranked at higher positions in . At xinfAP@100, the average improvement of over is 18.3%. Similar trend of improvement is also observed in , where xinfAP is boosted for 66 out of 120 queries. Benefited from , some true positives originally out of the search depth of 1,000 are ranked high. Examples include query-577 Find shots of two or more people wearing coats, where brings forward more than 100 positive videos. As a consequence, xinfAP is boosted for 173% from 0.1793 to 0.4898 for query-577. Nevertheless, there are 19 queries drop for both and . These are the queries where either or performs poorly. As training is conducted using video captioning datasets, dual-task learning may have been confused if the results of both tasks are in conflict with each other.
Late fusion. In general, concept-only search performs better when a query topic can be uniquely described by a few concepts independently. In contrast, embedding-only search is superior for complex queries, such as query-543 Find shots of a person communicating using sign language, where collective understanding of query terms is required. As both search methodologies are complementary, late fusion is employed. Figure 3 shows the sensitivity of hyper-parameter (Eq. (13)) that linearly combines the rank lists of both methods. The extreme values represents retrieval by embedding-only (i.e., ) and concept-only (i.e., ). As observed, the retrieval performance varies slightly when is set in the range of . Considering the capability of answering complex queries, we set to bias embedding-only more than concept-only retrieval in the late fusion. The detailed performances of our models on every query are list in the supplementary material.
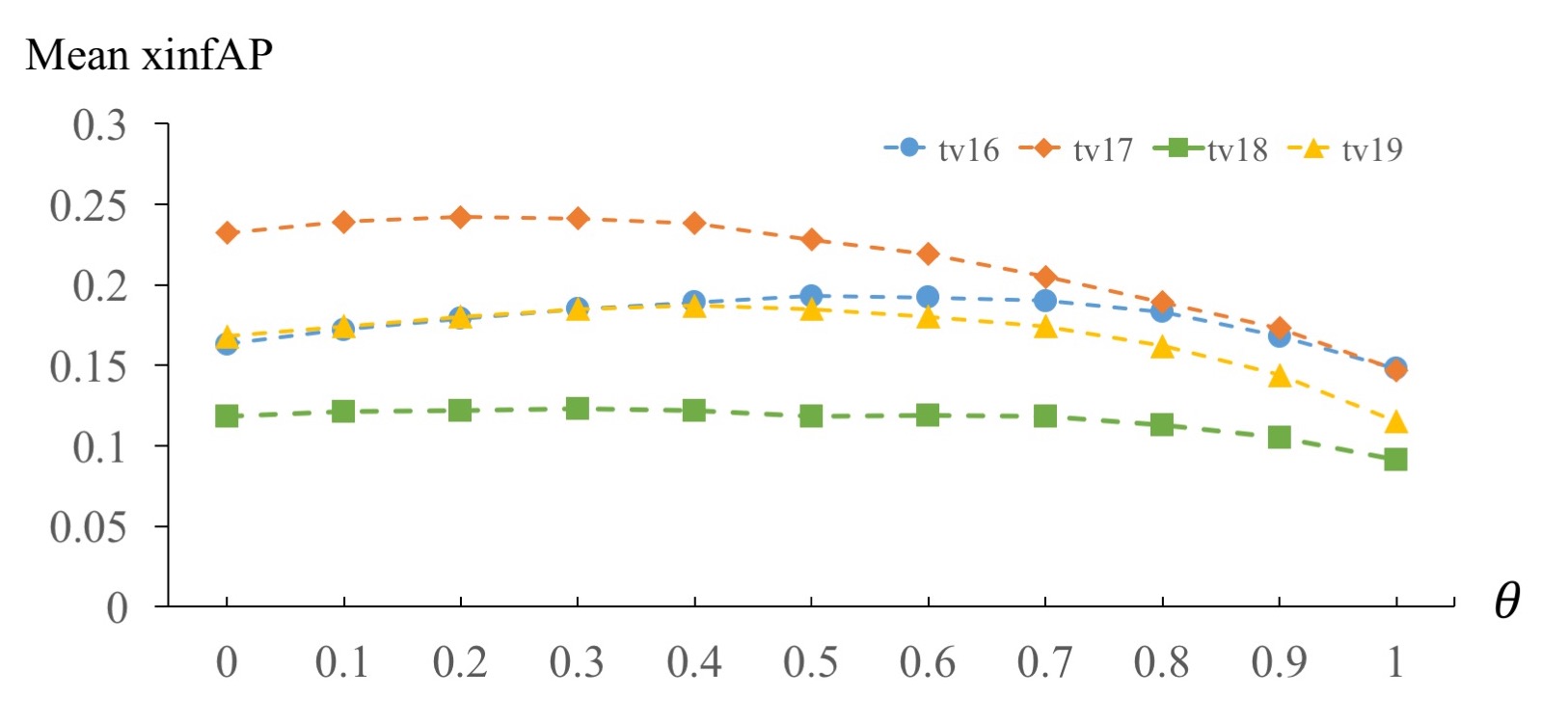
4.3. AVS Performance Comparison
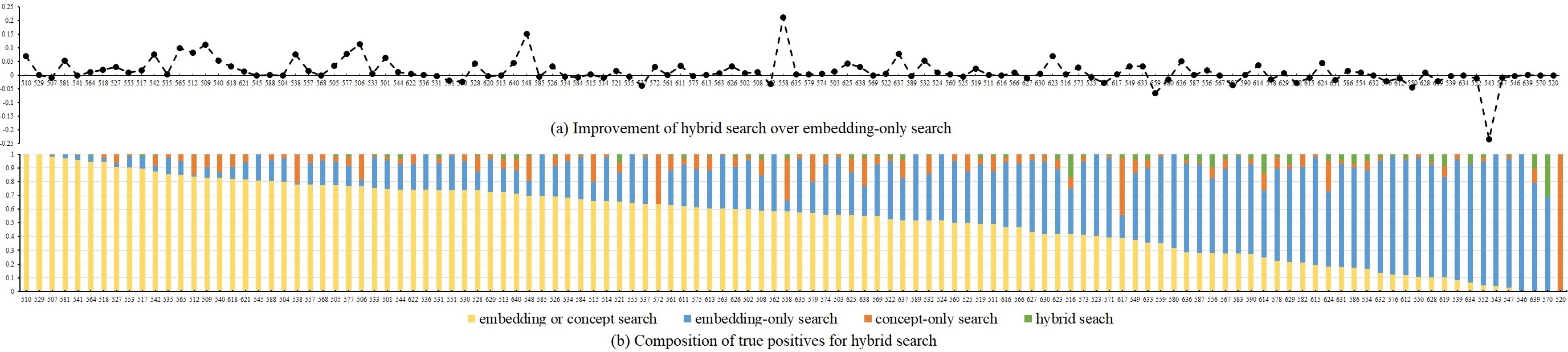
We compare dual-task model to the existing methods based on embedding-only, concept-only and hybrid search. Embedding-only search includes W2VV++ (Li et al., 2019a) and dual coding (Dong et al., 2019), which report the state-of-the-art performance on TRECVid datasets. Concept-based search includes mainly (Markatopoulou et al., 2017; Ueki et al., 2016, 2017, 2018, 2019). QKR (query and keyframe representation) (Markatopoulou et al., 2017) performs linguistic analysis to select concepts for queries. Through word2vec (Mikolov et al., 2013), the concept lists of queries and keyframes are projected into an embedding space for similarity comparison. Large concept bank (ConBank) composed of about 55,000 words is leveraged in (Ueki et al., 2017, 2018, 2019). Multi-level query analysis from words, phrases to sentences is conducted for concept mapping. As this process is error-prone, manual selection of query words and phrases for mapping with concepts is also performed in (Ueki et al., 2016, 2017, 2018, 2019) to contrast the performances between automatic and manual searches. The performances of hybrid approaches are mostly reported in TRECVid benchmarking only. For reference, the top-3 performances benchmarked in TRECVid are listed for comparison.
Datasets | IACC.3 | V3C1 | ||
---|---|---|---|---|
Query sets | tv16 | tv17 | tv18 | tv19 |
TRECVid top results: | ||||
Rank 1 | 0.054 (Nguyen et al., 2016) | 0.206* (Snoek et al., 2017) | 0.121 (Li et al., 2019a) | 0.163 (Wu et al., 2019) |
Rank 2 | 0.051 (Markatopoulou et al., 2016) | 0.159 (Ueki et al., 2017) | 0.087* (Huang et al., 2018) | 0.160 (Li et al., 2019b) |
Rank 3 | 0.040 (Liang et al., 2016) | 0.120* (Nguyen et al., 2017) | 0.082 (Bastan et al., shop) | 0.123 (Ueki et al., 2019) |
Embedding only: | ||||
VideoStory (Habibian et al., 2014) | 0.087 | 0.150 | / | / |
VSE++ (Faghri et al., 2018) | 0.123 | 0.154 | 0.074 | / |
W2VV (Dong et al., 2018) | 0.050 | 0.081 | 0.013 | / |
W2VV++ (Li et al., 2019a) | 0.163 | 0.196 | 0.115 | 0.127 |
Dual coding (Dong et al., 2019) | 0.165 | 0.228 | 0.117 | 0.152 |
Concept only: | ||||
QKR (Markatopoulou et al., 2017) | 0.064 | / | / | / |
ConBank (auto) | / | 0.159 (Ueki et al., 2017) | 0.060 (Ueki et al., 2018) | / |
ConBank (manual) | 0.177 (Ueki et al., 2016) | 0.216 (Ueki et al., 2017) | 0.106 (Ueki et al., 2018) | 0.114 (Ueki et al., 2019) |
Dual-task: | ||||
0.148 | 0.147 | 0.091 | 0.115 | |
0.163 | 0.232 | 0.118 | 0.168 | |
0.185* | 0.241* | 0.123* | 0.185* |
Table 3 lists the performance comparison. Except on tv16, the proposed shows better performances than its counterparts including W2VV++ and dual coding. Among the embedding-only approaches, shows the highest xinfAP in 43 out of 120 queries. Through dual-task training, shows higher ability in answering queries with unique concepts such as “scarf” in query-558 and “coats” in query-577. For concept-only search, outperforms most of its counterparts including QKR. Despite that the number of concepts is almost five times smaller than the large concept bank (Ueki et al., 2017, 2018, 2019), still shows competitive performance. Nevertheless, is not competent with (Ueki et al., 2016, 2017, 2018) when human intervention is allowed for concept selection. , as a hybrid model, outperforms all the existing approaches, including the top performers benchmarked in TRECVid. The performance also surpasses (Ueki et al., 2016, 2017, 2018, 2019) which is based on manual search. Compared to the best reported result by dual coding (Dong et al., 2019), the xinfAP gain is as large as 12.1% and 21.7% on tv16 and tv19 respectively. To verify the performance is not by chance, we conduct randomization test (Smucker et al., 2007). At the -value 0.01, the test shows that the dual-task model is significantly better than other approaches including dual coding, W2VV++ and the best reported performance in TRECVid.
In TRECVid (Awad et al., 2016), the 120 query topics are classified into 12 different types of complexities based on the composition of object, scene, action and location. Comparing to dual coding, is better in answering person-related queries, especially, the combination of person, object and location. The performance of exceeds dual coding on all queries in this kind, e.g., query-624 Find shots of a person in front of a curtain indoor. Compared with dual coding and W2VV++, has higher xinfAP on detecting object-only queries. For some queries such as query-512 Find shots of palm trees, xinfAP is as much as 167.8% better than W2VV++ and 82.7% than dual coding. The hybrid model, outperforms dual coding in 76 out of 120 queries, and the xinfAP gain ranges from 0.3% to 158.4%. These queries correspond to person-related or object-related queries, e.g., 121.8% gain on query-509 Find shots of a crowd demonstrating in a city street at night, and 100.0% gain on query-572 Find shots of two or more cats both visible simultaneously. The remaining queries suffering from xinfAP degradation mostly are those queries that fails miserably. The drop ranges from 0.2% to 87.5%. For example, dual coding has a xinfAP of 0.194 on query-514 Find shots of soldiers performing training or other military maneuvers, while only manages to attain xinfAP = 0.181. For this particular query, the performance gap between (xinfAP=0.191) and (xinfAP=0.079) is relative large. Further late fusion hurts the overall retrieval rate.
Type | Concept-free | Concept-based | ||||
Single query | Multiple sub-queries | |||||
Dual coding | Dual coding | Nguyen et al. (Nguyen et al., 2018) | ||||
AND | 0.249 | 0.260 | 0.073 | 0.089 | 0.293 | 0.454 |
OR | 0.714 | 0.686 | 0.737 | 0.775 | 0.356 | 0.864 |
NOT | 0.045 | 0.049 | 0.353 | 0.406 | 0.067 | 0.133 |
To provide further insights, Figure 4 visualizes the composition of true positives in the rank lists of . As observed, 72 out of 120 queries share more than 50% of true positives common between and . Among them, 50 queries show improvement in xinfAP due to elevation of ranking positions for true positions after score combination. Overall, performance improvement is more apparent for queries where true positives are separately contributed by embedding-only and concept-only searches. Finally, manages to pull true positives outside of 1,000 search depth from both and for 76 queries, and the number ranges from 1 to 20. However, as these positives are still ranked low in the list, their contributions to overall xinfAP are not significant.
4.4. Interpretability of Concept-free Search
Concept-free search suffers from the black-box retrieval of results. With dual-task model, nevertheless, interpretation of search result is feasible by listing out the decoded concepts alongside the retrieved videos. Here, we conduct an experiment to verify the top-10 retrieved videos of by the decoded concepts. A video is pruned from search list if the required keywords are not present among the list of decoded concepts. For example, the required keyword for query-625 Find shots of a person wearing a backpack is “backpack”. Any retrieved videos without the “backpack” concept will be eliminated from the search result.
The experiment is conducted as follows. A total of 30 queries, where exhibits higher xinfAP values, are selected for experiment. The top-10 retrieval accuracy averaged over queries is 82%. Among these query topics, 1-3 keywords are manually specified as the required concepts to present in a retrieved video. Any videos without all the required keywords present within the list of top-30 decoded concepts will be marked as negative videos. The result shows that, by dual-task model, 44.27% of false positives retrieved by can be successfully eliminated from the search list. Nevertheless, 10.67% of true positives are also being erroneously pruned. Generally, for queries involving unique concepts such as “helmet”, “plane”, “ballon”, the accuracy of pruning is high. Query-572 Find shots of two or more cats both visible simultaneously, by listing “two” and “cat” as keywords, more than 60% of false positives are removed. The method is relatively weak in interpreting queries with compositional concepts such as “bird in the tree” (query-638), only 20% of false positives can be pruned.
Note that the setting is realistic in the scenario of interactive search. Concretely, a searcher can specify a few keywords to quickly locate a small number of true positives among the search list. These true positives identified in a short period of time can serve multiple purposes. For example, the positives are leveraged for example-based video search (Rossetto et al., 2019), online training of classifier (Lu et al., 2017) and discovery of contextually relevant concepts for query refinement (Lokoč et al., 2019). We repeat the experiment by randomly sampling another 30 queries from the remaining 90 queries, where the performance of is generally better than . Among the top-10 retrieved videos, there are 168 true positives and 132 false positives, implying accuracy of 56.0%. By dual task model, 90 false positives are successfully pruned and 123 true positives are retained. The accuracy is boosted from 54% to 74.5%. With this, a searcher can potentially speed up the time, by skipping more than 50% of false positives, to identify a small number of true positives for subsequent search actions.
4.5. Boolean Queries
Boolean expression of query terms has been exploited in manual (Ueki et al., 2016) and interactive search (Nguyen et al., 2018). An example of query is Find shots of drinking beverage but not wine or beer. The performance of concept-free search are generally unpredictable when the entire Boolean query is embedded as a single vector (Li et al., 2019b). A feasible way is by decomposition of query into multiple sub-queries, such as “drinking beverage”, “wine” and “beer” as three separate sub-queries for retrieval. The results are then merged with Boolean combination. The experiment here aims to study the limit of dual-task model in answering Boolean queries by and .
As TRECVid queries are non-Boolean, we compile 15 Boolean queries for experiment, and conduct retrieval on V3C1 dataset (Berns et al., 2019). The list of queries is listed in supplementary document. For and dual coding (Dong et al., 2019), we contrast their performances when a query is treated as a single vector and multiple vectors by query splitting. We also compare with the recent work in (Nguyen et al., 2018) which performs Boolean based concept search using a vocabulary size of 16,263 that is similar to dual-task model. We use the measures in (Nguyen et al., 2018) for late fusion of search lists from multiple sub-queries for all the compared methods. As shown in Table 4, both and generally exhibit better performances than their respective counterparts. Concept-based search by shows higher xinfAP not only than (Nguyen et al., 2018) but also concept-free search when query is not split.
An interesting note is that, when query decomposition is performed, the performance of concept-free search is boosted significantly for queries with NOT operators. Query splitting is also helpful for queries with OR operator, but is likely to hurt the performance for queries with AND operator. Nevertheless, when a query involves not only NOT but also AND or OR, answering with multiple sub-queries will bring significant improvement in retrieval. In other words, concept-free approach fails to embed the semantics of NOT in the feature space. Explicit handling of NOT operator by multiple sub-queries processing is required. The performance trend is consistent for both and dual coding.
The result gives clues of how to exploit concept-free and concept-based search for Boolean queries. For example, when the query terms are logically rather than linguistically compositional, concept-based is likely a better choice than concept-free search. When NOT operator is involved, however, concept-free with query splitting strategy should be adopted. As dual-task model is interpretable with the result of being verified by (as in Section 4.4), appropriate search strategies can be flexibly implemented. For example, the result of concept-free search can be pruned by manually expressing the required concepts of a query with boolean expression. In general, these strategies are expected to be practical for interactive search.
5. Conclusion
We have presented a dual-task model to enable interpretability of concept-free (or embedding-only) search. The proposed class-sensitive BCE loss is essentially critical in guaranteeing the proper decoding of concepts. Empirical studies verify the merit of this loss function in mutually boosting the performances of both concept-free and concept-based searches under the dual-task learning strategy. A significant margin of improvement is attained when lately fusing both search lists, leading to the new state-of-the-art retrieval performances on the TRECVid datasets.
The experimental results reveal three main findings towards answering some research questions in AVS. First, the complement in search comes from different abilities in modeling the complexity of semantics. Concept-based search is limited by its ability to interpret compositional semantics that is built up from phrasal or sentence meaning. Concept-free search, on the other hand, appears less sensitive to lexical semantics that deals with individual word meaning. Late fusion of both search paradigms can lead to considerable boost in performance. Second, as proven in the empirical studies, using decoded concepts to interpret the result of concept-free search is potentially an effective strategy to eliminate false positives that rank high in the list. Such functionality will be highly practical for interactive search, where user can rapidly make sense of search result for query refinement. The final remark is that both search paradigms react differently to Boolean queries, and there is no clear way of fusion, such as by late fusion, to take advantage of each other. NOT statement, especially, remains highly difficult to be embedded and interpreted. Explicit query splitting is required to answer such queries.
Acknowledgements.
This work was supported by the National Natural Science Foundation of China (No. 61872256)References
- (1)
- Avgerinakis et al. (2018) Konstantinos Avgerinakis, Anastasia Moumtzidou, Damianos Galanopoulos, Georgios Orfanidis, Stelios Andreadis, Foteini Markatopoulou, Elissavet Batziou, Konstantinos Ioannidis, Stefanos Vrochidis, Vasileios Mezaris, and Ioannis Kompatsiaris. 2018. ITI-CERTH participation in TRECVID 2018. In Proceedings of the TRECVID 2018 Workshop.
- Awad et al. (2019) George Awad, Asad Butt, Keith Curtis, Yooyoung Lee, Jonathan Fiscus, Godil Afzal, Andrew Delgado, Zhang Jesse, Eliot Godard, Lukas Diduch, Alan F. Smeaton, Yvette Graham, Wessel Kraaij, and Georges Quenot. 2019. TRECVID 2019: An evaluation campaign to benchmark Video Activity Detection, Video Captioning and Matching, and Video Search and retrieval. In Proceedings of the TRECVID 2019 Workshop.
- Awad et al. (2018a) George Awad, Asad Butt, Jonathan Fiscus, David Joy, Andrew Delgado, Willie McClinton, Martial Michel, Alan Smeaton, Yvette Graham, Wessel Kraaij, Georges Quenot, Maria Eskevich, Roeland Ordelman, Gareth Jones, and Benoit Huet. 2018a. Trecvid 2017: Evaluating ad-hoc and instance video search, events detection, video captioning and hyperlinking. In Proceedings of the TRECVID 2017 Workshop.
- Awad et al. (2018b) George Awad, Asad Gov, Asad Butt, Keith Curtis, Yooyoung Lee, yooyoung@nist Gov, Jonathan Fiscus, David Joy, Andrew Delgado, Alan Smeaton, Yvette Graham, Wessel Kraaij, Georges Quenot, Joao Magalhaes, and Saverio Blasi. 2018b. TRECVID 2018: Benchmarking Video Activity Detection, Video Captioning and Matching, Video Storytelling Linking and Video Search. In Proceedings of the TRECVID 2018 Workshop.
- Awad et al. (2016) George Awad, Fiscus Jonathan, Joy David, Michel Martial, Smeaton Alan, Kraaij Wessel, Quenot Georges, Eskevich Maria, Aly Robin, Ordelman Roeland, Jones Gareth, Huet Benoit, and LarsonMartha. 2016. TRECVID 2016: Evaluating Video Search, Video Event Detection, Localization, and Hyperlinking. In Proceedings of the TRECVID 2016 Workshop.
- Bastan et al. (shop) Muhammet Bastan, Xiangxi Shi, Jiuxiang Gu, Zhao Heng, Chen Zhuo, Dennis Sng, and Alex Kot. Proceedings of the TRECVID 2018 Workshop. NTU ROSE Lab at TRECVID 2018: Ad-hoc Video Search and Video to Text. In Proceedings of the TRECVID 2018 Workshop.
- Berns et al. (2019) Fabian Berns, Luca Rossetto, Klaus Schoeffmann, Christian Beecks, and George Awad. 2019. V3C1 Dataset: An Evaluation of Content Characteristics. In Proceedings of the International Conference on Multimedia Retrieval. 334–338.
- Boer et al. (2017) Maaike H. T. De Boer, Yi-Jie Lu, Hao Zhang, Klamer Schutte, Chong-Wah Ngo, and Wessel Kraaij. 2017. Semantic Reasoning in Zero Example Video Event Retrieval. ACM Transactions on Multimedia Computing, Communications, and Applications 13, 4 (2017).
- Chen and Dolan (2011) David L. Chen and William B. Dolan. 2011. Collecting Highly Parallel Data for Paraphrase Evaluation. In Proceedings of the Annual Meeting of the Association for Computational Linguistics.
- Chen et al. (2015) Xinlei Chen, Hao Fang, Tsung-Yi Lin, Ramakrishna Vedantam, Saurabh Gupta, Piotr Dollar, and C. Zitnick. 2015. Microsoft COCO Captions: Data Collection and Evaluation Server. arxiv (2015).
- Cho et al. (2014) Kyunghyun Cho, Bart van Merriënboer, Caglar Gulcehre, Dzmitry Bahdanau, Fethi Bougares, Holger Schwenk, and Yoshua Bengio. 2014. Learning Phrase Representations using RNN Encoder–Decoder for Statistical Machine Translation. In Proceedings of the Conference on Empirical Methods in Natural Language Processing. 1724–1734.
- Dong et al. (2017) Jianfeng Dong, Shaoli Huang, Duanqing Xu, and Dacheng Tao1. 2017. DL-61-86 at TRECVID 2017: Video-to-Text Description. In Proceedings of the TRECVID 2017 Workshop.
- Dong et al. (2018) Jianfeng Dong, Xirong Li, and Cees G. M. Snoek. 2018. Predicting Visual Features From Text for Image and Video Caption Retrieval. IEEE Transactions on Multimedia 20, 12 (2018), 3377–3388.
- Dong et al. (2019) Jianfeng Dong, Xirong Li, Chaoxi Xu, Shouling Ji, Yuan He, Gang Yang, and Xun Wang. 2019. Dual Encoding for Zero-Example Video Retrieval. In Proceedings of the IEEE Conference on Computer Vision and Pattern Recognition.
- Faghri et al. (2018) Fartash Faghri, David J. Fleet, Jamie Ryan Kiros, and Sanja Fidlere. 2018. VSE++: Improving Visual-Semantic Embeddings with Hard Negatives. In Proceedings of the British Machine Vision Conference.
- Francis et al. (2019) Danny Francis, Phuong Anh Nguyen, Benoit Huet, and Chong-Wah Ngo. 2019. EURECOM at TRECVid AVS 2019. In Proceedings of the TRECVID 2019 Workshop.
- Habibian et al. (2014) Amirhossein Habibian, Thomas Mensink, and Cees G. M. Snoek. 2014. VideoStory: A New Multimedia Embedding for Few-Example Recognition and Translation of Events. In Proceedings of the ACM Conference on Multimedia.
- Huang et al. (2018) Po-Yao Huang, Junwei Liang, Vaibhav, Xiaojun Chang, and Alexander Hauptmann. 2018. Informedia@TRECVID 2018:Ad-hoc Video Search with Discrete and Continuous Representations. In Proceedings of the TRECVID 2018 Workshop.
- Jiang et al. (2015) Lu Jiang, Shoou-I Yu, Deyu Meng, Teruko Mitamura, and Alexander Hauptmann. 2015. Bridging the Ultimate Semantic Gap: A Semantic Search Engine for Internet Videos. In Proceedings of the ACM International Conference on Multimedia Retrieval.
- Jiang et al. (2010) Yu-Gang Jiang, Jun Yang, Chong-Wah Ngo, and Alexander Hauptmann. 2010. Representations of Keypoint-Based Semantic Concept Detection: A Comprehensive Study. IEEE Transactions on Multimedia 12 (02 2010), 42–53.
- Li et al. (2019a) Xirong Li, Chaoxi Xu, Gang Yang, Zhineng Chen, and Jianfeng Dong. 2019a. W2VV++: Fully Deep Learning for Ad-hoc Video Search. In Proceedings of the ACM International Conference on Multimedia.
- Li et al. (2019b) Xirong Li, Jinde Ye, Chaoxi Xu, Shanjinwen Yun, Leimin Zhang, Xun Wang, Rui Qian, and Jianfeng Dong. 2019b. Renmin University of China and Zhejiang Gongshang University at TRECVID 2019: Learn to Search and Describe Videos. In Proceedings of the TRECVID 2019 Workshop.
- Li et al. (2016) Yuncheng Li, Yale Song, Liangliang Cao, Joel Tetreault, Larry Goldberg, Alejandro Jaimes, and Jiebo Luo. 2016. TGIF: A New Dataset and Benchmark on Animated GIF Description. In Proceedings of the IEEE Conference on Computer Vision and Pattern Recognition.
- Liang et al. (2016) Junwei Liang, Poyao Huang, Lu Jiang, Zhenzhong Lan, Jia Chen, and Alexander Hauptmann. 2016. Informedia @ TRECVID 2016 MED and AVS. In Proceedings of the TRECVID 2016 Workshop.
- Lokoč et al. (2018) Jakub Lokoč, Werner Bailer, Klaus Schoeffmann, Bernd Munzer, and George Awad. 2018. On Influential Trends in Interactive Video Retrieval: Video Browser Showdown 2015–2017. IEEE Transactions on Multimedia 20, 12 (2018), 3361–3376.
- Lokoč et al. (2019) Jakub Lokoč, Gregor Kovalčík, Bernd Münzer, Klaus Schöffmann, Werner Bailer, Ralph Gasser, Stefanos Vrochidis, Phuong Anh Nguyen, Sitapa Rujikietgumjorn, and Kai Uwe Barthel. 2019. Interactive Search or Sequential Browsing? A Detailed Analysis of the Video Browser Showdown 2018. ACM Trans. Multimedia Comput. Commun. Appl. 15, 1 (2019).
- Lu et al. (2017) Yi-Jie Lu, Phuong Anh Nguyen, Hao Zhang, and Chong-Wah Ngo. 2017. Concept-Based Interactive Search System. In Proceedings of the MultiMedia Modeling. 463–468.
- Lu et al. (2016) Yi-Jie Lu, Hao Zhang, Maaike de Boer, and Chong-Wah Ngo. 2016. Event Detection with Zero Example: Select the Right and Suppress the Wrong Concepts. In Proceedings of the ACM on International Conference on Multimedia Retrieval. 127–134.
- Markatopoulou et al. (2017) Foteini Markatopoulou, Damianos Galanopoulos, Vasileios Mezaris, and Ioannis Patras. 2017. Query and Keyframe Representations for Ad-Hoc Video Search. In Proceedings of the ACM on International Conference on Multimedia Retrieval. 407–411.
- Markatopoulou et al. (2016) Foteini Markatopoulou, Anastasia Moumtzidou, Damianos Galanopoulos, Theodoros Mironidis, Vagia Kaltsa, Anastasia Ioannidou, Spyridon Symeonidis, Konstantinos Avgerinakis, Stelios Andreadis, Ilias Gialampoukidis, Stefanos, Vrochidis, Alexia Briassouli, Vasileios Mezaris, Ioannis Kompatsiaris, Ioannis, and Patravs. 2016. ITI-CERTH participation in TRECVID 2016. In Proceedings of the TRECVID 2016 Workshop.
- Mikolov et al. (2013) Tomas Mikolov, Ilya Sutskever, Kai Chen, Greg Corrado, and Jeffrey Dean. 2013. Distributed Representations of Words and Phrases and Their Compositionality. In Proceedings of the International Conference on Neural Information Processing. 3111–3119.
- Naphade et al. (2006) Milind Naphade, J.R. Smith, Jelena Tesic, S. Chang, Winston Hsu, Lyndon Kennedy, Alexander Hauptmann, and Jon Curtis. 2006. Large-Scale Concept Ontology for Multimedia. IEEE Transactions on Multimedia 13 (08 2006), 86–91.
- Nguyen et al. (2017) Phuong Anh Nguyen, Qing Li, Zhi-Qi Cheng, Yi-Jie Lu, Hao Zhang, Xiao Wu, and Chong-Wah Ngo. 2017. VIREO @ TRECVID 2017: Video-to-Text, Ad-hoc Video Search and Video Hyperlinking. In Proceedings of the TRECVID 2017 Workshop.
- Nguyen et al. (2018) Phuong Anh Nguyen, Yi-Jie Lu, Hao Zhang, and Chong-Wah Ngo. 2018. Enhanced VIREO KIS at VBS 2018. In Proceedings of the International conference on MultiMedia Modeling. 407–412.
- Nguyen et al. (2019) Phuong Anh Nguyen, Jiaxin Wu, Chong-Wah Ngo, Francis Danny, and Huet Benoit. 2019. VIREO-EURECOM @ TRECVID 2019: Ad-hoc Video Search. In Proceedings of the TRECVID 2019 Workshop.
- Nguyen et al. (2016) Vinh-Tiep Nguyen, Duy-Dinh Le, Benjamin Renoust, Thanh Duc Ngo, Minh-Triet Tran, Duc Anh Duong, and Shinichi Satoh. 2016. NII-HITACHI-UIT at TRECVID 2016 Ad-hoc Video Search: Enriching Semantic Features using Multiple Neural Networks. In Proceedings of the TRECVID 2016 Workshop.
- Rossetto et al. (2019) Luca Rossetto, Mahnaz Amiri Parian, Ralph Gasser, Ivan Giangreco, Silvan Heller, and Heiko Schuldt. 2019. Deep Learning-Based Concept Detection in vitrivr. In Proceedings of the MultiMedia Modeling, Ioannis Kompatsiaris, Benoit Huet, Vasileios Mezaris, Cathal Gurrin, Wen-Huang Cheng, and Stefanos Vrochidis (Eds.). 616–621.
- Shirahama et al. (2019) Kimiaki Shirahama, Daichi Sakurai, Takashi Matsubara, and Kuniaki Uehara. 2019. Kindai University and Kobe University at TRECVID 2019 AVS Task. In Proceedings of the TRECVID 2019 Workshop.
- Smeaton et al. (2006) Alan F. Smeaton, Paul Over, and Wessel Kraaij. 2006. Evaluation Campaigns and TRECVid. In Proceedings of the ACM International Workshop on Multimedia Information Retrieval. 321–330.
- Smucker et al. (2007) Mark D. Smucker, James Allan, and Ben Carterette. 2007. A Comparison of Statistical Significance Tests for Information Retrieval Evaluation. In Proceedings of the ACM Conference on Conference on Information and Knowledge Management. 623–632.
- Snoek et al. (2017) Cees G. M. Snoek, Xirong Li, Chaoxi Xu, and Dennis C. Koelma. 2017. University of Amsterdam and Renmin University at TRECVID 2017: Searching Video, Detecting Events and Describing Video. In Proceedings of the TRECVID 2017 Workshop.
- Snoek and Worring (2009) Cees G. M. Snoek and Marcel Worring. 2009. Concept-Based Video Retrieval. Foundations and Trends in Information Retrieval 2 (2009), 215–322.
- Snoek et al. (2006) Cees G. M. Snoek, Marcel Worring, Jan C. van Gemert, Jan-Mark Geusebroek, and Arnold W. M. Smeulders. 2006. The Challenge Problem for Automated Detection of 101 Semantic Concepts in Multimedia. In Proceedings of the 14th ACM International Conference on Multimedia. 421–430.
- Ueki et al. (2017) Kazuya Ueki, Koji Hirakawa, Kotaro Kikuchi, Tetsuji Ogawa, and Tetsunori Kobayashi. 2017. Waseda Meisei at TRECVID 2017:Ad-hoc Video Search. In Proceedings of the TRECVID 2017 Workshop.
- Ueki et al. (2019) Kazuya Ueki, Takayuki Hori, and Tetsunori Kobayashi. 2019. Waseda Meisei SoftBank at TRECVID 2019: Ad-hoc Video Search. In Proceedings of the TRECVID 2019 Workshopp.
- Ueki et al. (2016) Kazuya Ueki, Kotaro Kikuchi, Susumu Saito, and Tetsunori Kobayashi. 2016. Waseda at TRECVID 2016: Ad-hoc Video Search. In Proceedings of the TRECVID 2016 Workshop.
- Ueki et al. (2018) Kazuya Ueki, Yu Nakagome, Koji Hirakawa, Kotaro Kikuchi, Yoshihiko Hayashi, Tetsuji Ogawa, and Tetsunori Kobayashi. 2018. Waseda Meisei at TRECVID 2018:Ad-hoc Video Search. In Proceedings of the TRECVID 2018 Workshop.
- Wei and Ngo (2007) Xiao-Yong Wei and Chong-Wah Ngo. 2007. Ontology-Enriched Semantic Space for Video Search. In Proceedings of the ACM International Conference on Multimedia. 981–990.
- Wu et al. (2019) Xiang Wu, Da Chen, Yuan He, Hui Xue, Mingli Song, and Feng Mao. 2019. Hybrid Sequence Encoder for Text based Video Retrieval. In Proceedings of the TRECVID 2019 Workshop.
- Xu et al. (2016) Jun Xu, Tao Mei, Ting Yao, and Yong Rui. 2016. MSR-VTT: A Large Video Description Dataset for Bridging Video and Language. In Proceedings of the IEEE International Conference on Computer Vision and Pattern Recognition.