Identifying Untrustworthy Samples: Data Filtering for Open-domain Dialogues with Bayesian Optimization
Abstract.
Being able to reply with a related, fluent, and informative response is an indispensable requirement for building high-quality conversational agents. In order to generate better responses, some approaches have been proposed, such as feeding extra information by collecting large-scale datasets with human annotations, designing neural conversational models (NCMs) with complex architecture and loss functions, or filtering out untrustworthy samples based on a dialogue attribute, e.g., Relatedness or Genericness. In this paper, we follow the third research branch and present a data filtering method for open-domain dialogues, which identifies untrustworthy samples from training data with a quality measure that linearly combines seven dialogue attributes. The attribute weights are obtained via Bayesian Optimization (BayesOpt) that aims to optimize an objective function for dialogue generation iteratively on the validation set. Then we score training samples with the quality measure, sort them in descending order, and filter out those at the bottom. Furthermore, to accelerate the “filter-train-evaluate” iterations involved in BayesOpt on large-scale datasets, we propose a training framework that integrates maximum likelihood estimation (MLE) and negative training method (NEG). The training method updates parameters of a trained NCMs on two small sets with newly maintained and removed samples, respectively. Specifically, MLE is applied to maximize the log-likelihood of newly maintained samples, while NEG is used to minimize the log-likelihood of newly removed ones. Experimental results on two datasets show that our method can effectively identify untrustworthy samples, and NCMs trained on the filtered datasets achieve better performance.
1. Introduction
With the availability of large-scale data, such as posts on social media or forums, scripts of movies or TV series, and datasets from collection or crowdsourcing (Lison and Tiedemann, 2016; Henderson et al., 2019; Chen et al., 2020), neural conversational models (NCMs) (Shen et al., 2019, 2021a) have developed rapidly and their performance has been improved steadily. Current NCMs are mainly trained on context-response pairs111A pair of context and response is a training sample in the dialogue generation task. with maximum likelihood estimation (MLE). However, the generated responses may suffer from some notorious problems, such as being generic, inconsistent, or unrelated to the given contexts. Previous studies tried to solve these issues by feeding extra information, e.g., topics (Liu et al., 2020), sentence types (Reed et al., 2018), personas (Li et al., 2016b), emotions (Zhou et al., 2018a; Shen and Feng, 2020), documents (Meng et al., 2020), multi-modal (Shen et al., 2021b) or knowledge (Ke et al., 2018; Zhan et al., 2021a; Zhou et al., 2018b; Liu et al., 2019a; Zhan et al., 2021b), augmenting the model itself (Zhao et al., 2017; Xu et al., 2018), or modifying the loss function (Li et al., 2016a).
1 | A big nail should be put in your head. | |
---|---|---|
Who are they? (An unrelated response) | ||
2 | We met yesterday. Oh, you’re Thomas. Yes. | |
We haven’t met. (An inconsistent response) | ||
3 | There! You can see a window there. | |
It’s about an hour. | ||
You can buy a bus schedule in a news stand. | ||
I see. Thank you. (A generic response) |
In addition to the above mentioned approaches, some researchers pay attention to data filtering methods that remove untrustworthy training samples and further improve the performance of open-domain dialogue generation. Take Table 1 as an example. The response in Case 1 is unrelated to the given context, while the response in Case 2 is inconsistent to the previous utterances. In Case 3, the response can be used to respond three different contexts, and is considered as a generic response. When it comes to the question “how to identify untrustworthy samples?”, each work firstly defines a measure based on an individual dialogue attribute, including Coherence (Xu et al., 2018), Genericness (Csáky et al., 2019), Relatedness and Connectivity (Akama et al., 2020)222The definition of Relatedness from Akama et al. (2020) is the same as that of Coherence from Xu et al. (2018). Though Akama et al. (2020) used both Relatedness and Connectivity, these two attributes are combined simply with a weighted sum, where the weights are calculated by overall scores on the training set.. Then samples with low (or high) scores333For metrics like Coherence, lower is worse; while for metrics like Genericness, higher is worse. are regarded as untrustworthy ones. However, due to the subjectivity and open-ended nature of human conversations, the quality of dialogue data varies greatly (Cai et al., 2020). Moreover, it is hard to evaluate the dialogue quality just grounding on a single metric (Mehri and Eskenazi, 2020a, b; Pang et al., 2020). In general, a reasonable assessment should contain multiple aspects of attributes (See et al., 2019), such as the fluency and specificity of the response, the topical relatedness and factual consistency between the context and response, etc.
In this paper, we propose a data filtering method to identify untrustworthy samples in training data with the consideration of seven dialogue attributes. Instead of using these attributes in isolation, we gather them together to learn a measure of data quality based on Bayesian Optimization (BayesOpt) (Brochu et al., 2010). Specifically, the measure is a combination of seven attributes and the weights are learnt via BayesOpt. BayesOpt aims to iteratively find a group of weights that optimizes an objective function on the validation set for dialogue generation. After obtaining the optimal weights and calculating the measure score for each sample, we can filter out data with low scores and use the maintained (filtered) dataset444In this paper, “maintained dataset”, “filtered dataset”, and have the same meaning. to train an NCM. Nevertheless, simply applying BayesOpt for data filtering on large-scale dialogue datasets is inappropriate, since an iteration of “filter-train-evaluate” based on lots of samples is time-consuming. To accelerate the process, we design a training framework, named Diff-MLE-NEG, which combines maximum likelihood estimation (MLE) and negative training method (NEG) (He and Glass, 2020). Given a model trained on a randomly filtered dataset, Bayesian Optimization with Diff-MLE-NEG is performed to update the model parameters iteratively. In each iteration, we keep track of a small size of newly maintained or removed sample set. For newly maintained samples, denoted as desirable ones, MLE is applied to maximize their log-likelihood, while for newly removed samples, denoted as undesirable ones, NEG is used to minimize their log-likelihood.
Experimental results on two open-domain dialogue datasets, OpenSubtitles and DailyDialog, show that our data filtering method can effectively detect untrustworthy samples. Furthermore, after training several NCMs on our filtered datasets, the performance of dialogue generation can be improved.
Our main contributions can be summarized as: (1) We propose a measure based on seven dialogue attributes for estimating the quality of dialogue samples in an unsupervised manner. (2) We frame the dialogue data filtering task as an optimization problem, and propose a new method to accelerate the iterations of “filter-train-evaluate” involved in Bayesian Optimization on large-scale datasets. (3) Automatic and human evaluations show that our method can identify untrustworthy dialogue samples and further improve the performance of several NCMs for dialogue generation on different datasets.
2. Data filtering Method
In this section, we introduce our proposed data filtering method for open-domain dialogues in detail. We firstly give a description of the task, and then illustrate the Bayesian Optimization approach used for data filtering as well as the definitions of two important components, i.e., dialogue attributes and the objective function. Finally, we design a training method that combines maximum likelihood optimization (MLE) and negative training method (NEG) to accelerate the optimization iterations performed on large-scale datasets.
2.1. Task Definition
Given a dialogue corpus with pairs of context and response , i.e., , data filtering aims to remove untrustworthy utterance pairs from the training data, and further improve the performance of NCMs for dialogue generation (Akama et al., 2020).
To score each sample (- pair), we propose a measure as a linear combination of several dialogue attributes:
(1) |
where is the dialogue attributes further described in Section 2.3 for each training sample, is the number of attributes, and denotes the weights we want to obtain for these attributes. After each sample is scored according to the measure , we consider pairs with low scores as untrustworthy samples and filter them out for the dialogue generation task. We define the objective function as the automatic evaluation metric for dialogue generation performed on the validation set. Then we convert the dialogue data filtering task into an optimization problem, and our goal is to define and find the weights that optimizes .
2.2. Bayesian Optimization for Data Filtering
The defined measure is agnostic of the objective function , thus we cannot apply gradient-based methods for optimization. Other search methods such as random search or grid search requires exponential traverses regarding the number of parameterization of , which is time-consuming and not suitable for our task.
Inspired by Tsvetkov et al. (2016) and Ruder and Plank (2017), we use Bayesian Optimization (Brochu et al., 2010) here, which is a framework used to globally optimize any black-box function (Shahriari et al., 2015). Bayesian Optimization can be considered as a sequential approach to performing a regression from high-level model parameters (e.g., hidden state dimension, the number of layers in a neural network, or in our method) to the loss function or the objective function (Tsvetkov et al., 2016).
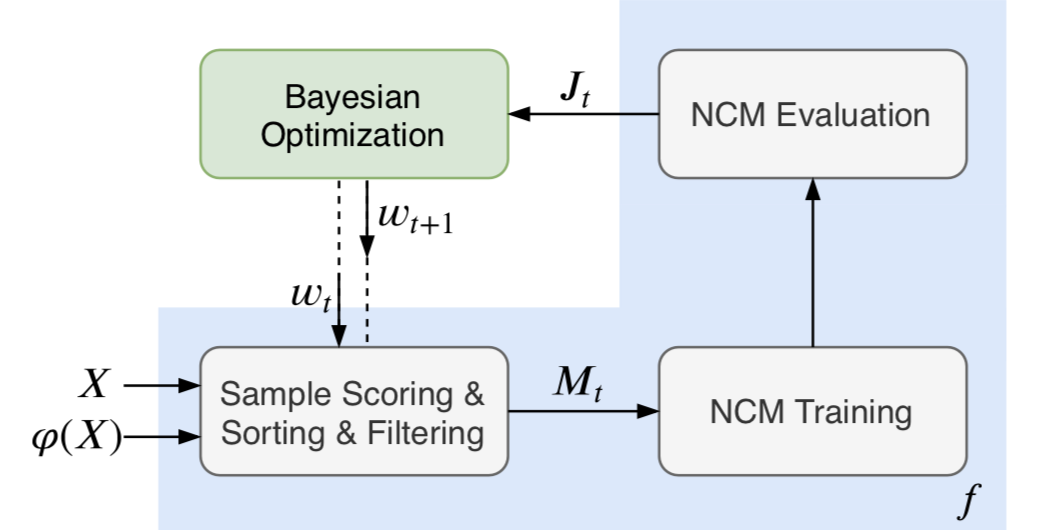
In general, given a black-box function , Bayesian Optimization tries to find an input that globally minimize . To fulfill this, it requires a prior over the function and an acquisition function that calculates the utility of any evaluation at any . Bayesian Optimization then proceeds in an iterative manner. At iteration step , (1) it obtains the most promising input via numerical optimization; (2) then, it evaluates the surrogate function on input , and adds the resulting data point (, ) to the set of observations ; (3) based on , it updates the prior and the acquisition function . Following Ruder and Plank (2017), Gaussian Processes (GP) is chosen for , and GP with Monte Carlo acquisition and Expected Improvement (EI) (Mockus, 1975) is used as the acquisition function. Note that the Robust Bayesian Optimization framework, RoBO555http://automl.github.io/RoBO/, implements several details in Bayesian Optimization, and can be applied directly.
As for utilizing Bayesian Optimization in our task, the data filtering process described in Figure 1 can be treated as the black-box function: (1) it takes that should be evaluated as input; (2) all training samples are sorted according to the measure defined in Equation 1; (3) we remove the lowest samples and get a subset of , denoted as ; (4) a specific NCM is trained on ; (5) the model is evaluated on the validation set according to the evaluation metric and the value of is returned.
2.3. and
in Equation 1 denotes the values of some dialogue attributes. There exists several work focusing on dialogue attributes, and some use these features to influence the training and inference processes of dialogue models (See et al., 2019; Zhang et al., 2018; Cai et al., 2020), while others apply them as metrics for evaluation (Tao et al., 2018; Zhao et al., 2020; Pang et al., 2020; Mehri and Eskenazi, 2020a, b). Our paper follows the former branch, and we use seven dialogue attributes to calculate the measure .
Specificity. Generic and unspecific responses are common in open-domain datasets, e.g., “I don’t know” or “That’s great”. Following See et al. (2019), we apply Normalized Inverse Document Frequency (NIDF) to measure word rareness:
(2) |
where . is the number of responses in the dataset, and is the number of responses that contain word . and are the minimum and maximum , taken over all words in the vocabulary. Then, the specificity of a response , , is the mean of all words in .
Repetitiveness. Specificity cares more about inter-utterance difference, for intra-utterance diversity, we use (Cai et al., 2020) to measure the repetitiveness of a response :
(3) |
where is an indicator function that takes the value 1 when is true and 0 otherwise. is the length of .
Relatedness. Cosine similarity between the vectors of two utterances, 666For contexts with multiple utterances, we concatenate them into a long string. and , has been widely used to calculate their topical relatedness (Zhang et al., 2018; Xu et al., 2018; Akama et al., 2020):
(4) |
where is the utterance embedding, and is computed as the weighted sum of word embeddings: (Arora et al., 2017), where and are the embedding and probability777Probability is calculated based on the maximum likelihood estimation on the training data. of word , respectively.
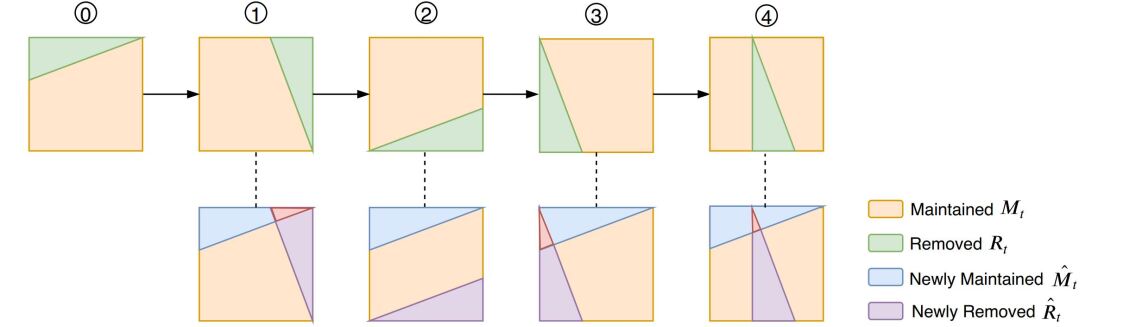
Continuity. A good open-domain conversational agent should be able to interact with users in more turns, that is, a response is also responsible to encourage the next utterance. Following Cai et al. (2020), is introduced as the cosine similarity between vectors of and the subsequent utterance with the same calculation as Relatedness.
Coherence. Coherence evaluates whether a response can be considered as an appropriate and natural reply to the context, and it is also described as Connectivity (Akama et al., 2020). Coherence between and can be either measured as next sentence prediction (Tao et al., 2018) or conditional language modeling task (Pang et al., 2020). With the support of large-scale pre-trained language models, e.g., GPT-2 (Radford et al., 2019), the latter one could be more reliable to capture the coherence between and after we further fine-tune GPT-2 on dialogue datasets. Following Pang et al. (2020), Coherence is defined as follows (ranging from 0 to 1):
(5) |
where represents the fine-tuned GPT-2 model, and . Since is a negative unbounded number, a lower bound used to normalize Coherence is defined as 5th percentile of the score distribution. Please refer to Pang et al. (2020) for more details.
Fluency. Fluency assesses the grammatical correctness and readability of an utterance. Similar to Coherence, the fluency score is computed as:
(6) |
where , and denotes 5th percentile of the score distribution.
Consistency. Factual consistency is also an important attribute for a dialogue, and there should not be logic contradictions. Natural Language Inference (NLI) (Bowman et al., 2015) tries to map a sentence pair to an entailment category including ”Entailment”, “Neutral”, and “Contradiction” (abbreviated as “Contra” below). Besides, it has been used in dialogue systems to measure the logic consistency (Welleck et al., 2019; Song et al., 2020; Pang et al., 2020). We apply the pre-trained model RoBERTa888We use RoBERTa released by Huggingface: https://huggingface.co/roberta-large-mnli. (Liu et al., 2019b), , to compute the consistency score between and as follows:
(7) |
Choices of . For the objective function , we mainly try two simple options: perplexity (Serban et al., 2015) and the negative value999Bayesian Optimization needs to minimize , but most metrics we used for evaluating dialogue generation should be larger when indicating a better response. of a sum of 13 widely-used automatic metrics, including BLEU, Dist-1/2/3, Intra-1/2/3, Ent-1/2, Average, Greedy, Extrema, and Coherence (Xu et al., 2018). For the latter one, please refer to Cai et al. (2020) for more details. For simplicity, we denote these two types of as “+ppl” and “-metric”, respectively. Some automatic metrics (Tao et al., 2018; Zhao et al., 2020; Sinha et al., 2020; Pang et al., 2020; Mehri and Eskenazi, 2020a, b) that correlate more with human judgment have been proposed recently, but they are not accepted thoroughly by the entire community. Therefore, we leave applying these metrics as for future work.
2.4. Training Acceleration
As we can see in Figure 1, in each iteration of Bayesian Optimization, we need to repeat filtering, training and evaluation. Since the maintained dataset is still large, training an NCM with it is extremely time-consuming. To accelerate the process, we design a training method, Diff-MLE-NEG, which combines maximum likelihood estimation (MLE) and negative training method (NEG) (He and Glass, 2020) together.
Following He and Glass (2020), MLE is used to maximize the log-likelihood of desirable samples, while NEG aims to minimize the log-likelihood of undesirable ones. Here the desirable samples are defined as newly maintained ones in iteration , i.e., , while the undesirable samples are defined as newly removed ones in iteration , i.e., , which is illustrated in Figure 2101010We use the difference set of (or ) and (or ), rather than (or ) and (or ) (the difference set of two consecutive iterations), to achieve a stable update process.. and are obtained by randomly removing bottom samples in . An NCM is trained to convergence with at first, and then Diff-MLE-NEG (shown in Algorithm 1) updates the model parameter on and iteratively, where is the number of optimization iterations.
3. Experiments
In this section, we introduce some empirical settings of our experiments, including research questions, datasets, models and comparable methods, implementation details, and evaluation measures.
3.1. Research Questions
We aim at answering the following research questions:
(RQ1): What is the performance of our approach on automatic and human evaluations? Does our model outperform other comparable methods?
(RQ2): Is our method able to identify untrustworthy samples, and even to sort training samples properly?
(RQ3): Does our data filtering method improve the generation quality and generate better response?
(RQ4): What are the relations among chosen attributes? Are they reasonable to be included in measure ?
(RQ5): Where does the improvements of our method come from? What is the difference between using only one attribute and our proposed measure ?
(RQ6): How does Bayesian Optimization work in this method?
OpenSubtitles | DailyDialog | |
---|---|---|
Training size | 298,780 | 54,889 |
Validation size | 29,948 | 6,005 |
Test size | 29,940 | 5,700 |
Vocabulary size | 116,611 | 17,875 |
Avg. of word per utterance | 12.89 | 14.87 |
3.2. Datasets
Following Csáky et al. (2019) and Akama et al. (2020), we conduct experiments on two widely-used open-domain dialogue datasets: OpenSubtitles (Lison and Tiedemann, 2016) and DailyDialog (Li et al., 2017b). OpenSubtitles is a collection of movie subtitles and originally contains over 2 billion utterances. DailyDialog consists of 90,000 utterances in 13,000 daily conversations. After data preprocessing111111We use the preprocessed datasets provided by Cai et al. (2020)., including tokenization and utterance concatenation, the number of - pairs in training/validation/test set is 298,780/29,948/29,940 for OpenSubtitles, and 54,889/6,005/5,700 for DailyDialog. The detailed statistics of two datasets we used in experiments are shown in Table 2. As we can see in Table 1, both of these datasets include some untrustworthy - pairs.
3.3. Models and Comparable Methods
We perform experiments using four representative NCMs for open-domain dialogue generation:
-
•
S2S (Bahdanau et al., 2015): a sequence-to-sequence model with cross-attention mechanism.
-
•
CVAE (Zhao et al., 2017): a conditional variational auto-encoder model with KL-annealing and a bag-of-word (BOW) loss.
-
•
TRS (Vaswani et al., 2017): the Transformer model, which is an encoder-decoder architecture with self-attention mechanism.
-
•
GVT (Lin et al., 2020): a Transformer model with a global latent variable.
We also compare our method with an entropy-based filtering approach (Csáky et al., 2019), which aims to remove generic utterances from the training data for promoting less-safe response generation. In our experiments, we apply IDENTITY-BOTH121212IDENTITY means the entropy is calculated on all utterances, while BOTH means the filtering is conducted on both context and response sides. method of Csáky et al. (2019) and denote it as “-Ent” to show the results.
3.4. Implementation Details
We employ a 2-layer bidirectional GRU (Cho et al., 2014) as the encoder and a unidirectional one as the decoder for S2S and CVAE. The hidden size is set to 300, and the size of latent variable is set to 64 for CVAE. For TRS and GVT, the hidden size, number of attention heads and number of hidden layers are set to 300, 2, and 2, respectively. The size of latent variable for GVT equals to 300. The word embedding is initialized with the 300-dimensional pre-trained GloVe embeddings (Pennington et al., 2014) for both encoder and decoder. KL annealing and the BOW loss are applied as in Zhao et al. (2017). , the number of Bayesian Optimization iteration, is set to 100. The proportion (%) of training samples we need to filter out is 26% and 12% for OpenSubtitles and DailyDialog, respectively131313In order to make fair comparisons, the proportion number is determined by IDENTITY-BOTH method in the work of Csáky et al. (2019).. In the test time, we use greedy decoding strategy for all models. Each model is trained on two kinds of datasets: the original dataset and the filtered dataset obtained from our data filtering method, keeping other configurations the same. To restrict the NEG training method and avoid the loss being too large, we utilize gradient penalty following Gulrajani et al. (2017). All the models are implemented with Pytorch141414https://pytorch.org/ and are trained on four Titan Xp GPUs.
Model | BLEU | Perplexity | Dist-1 | Dist-2 | Dist-3 | Intra-1 | Intra-2 | Intra-3 | Average | Greedy | Extrema | Coherence |
---|---|---|---|---|---|---|---|---|---|---|---|---|
S2S-Orig | 0.55 | 46.59 | 0.13 | 0.54 | 1.31 | 98.65 | 99.42 | 99.59 | 84.95 | 73.63 | 54.80 | 89.29 |
S2S-Ent | 0.41 | 57.79 | 0.07 | 0.27 | 0.52 | 97.76 | 98.23 | 98.62 | 87.87 | 75.59 | 54.45 | 92.41 |
S2S () | 0.52 | 47.96 | 0.14 | 0.59 | 1.26 | 99.05 | 99.47 | 99.68 | 85.04 | 73.69 | 55.08 | 88.25 |
S2S () | 0.54 | 47.88 | 0.17 | 0.62 | 1.36 | 99.12 | 99.52 | 99.73 | 85.13 | 73.71 | 55.14 | 90.75 |
CVAE-Orig | 0.21 | 37.78 | 0.07 | 0.28 | 0.62 | 99.28 | 99.46 | 99.79 | 86.97 | 73.72 | 53.41 | 91.02 |
CVAE-Ent | 0.19 | 41.51 | 0.06 | 0.31 | 0.70 | 97.90 | 98.60 | 98.80 | 87.12 | 72.30 | 51.60 | 91.27 |
CVAE () | 0.20 | 40.36 | 0.09 | 0.32 | 0.68 | 98.45 | 99.32 | 99.67 | 87.35 | 73.56 | 52.89 | 91.23 |
CVAE () | 0.23 | 38.27 | 0.12 | 0.34 | 0.72 | 99.33 | 99.67 | 99.84 | 87.73 | 73.97 | 53.45 | 91.18 |
TRS-Orig | 0.46 | 48.98 | 0.05 | 0.16 | 0.27 | 99.03 | 99.58 | 99.72 | 87.57 | 73.81 | 52.67 | 91.07 |
TRS-Ent | 0.47 | 54.10 | 0.10 | 0.45 | 0.94 | 97.26 | 98.97 | 99.51 | 87.69 | 73.99 | 50.88 | 91.24 |
TRS () | 0.63 | 46.13 | 0.14 | 0.66 | 1.50 | 95.25 | 97.88 | 99.21 | 86.68 | 73.52 | 51.44 | 91.14 |
TRS () | 0.80 | 46.06 | 0.17 | 0.78 | 1.70 | 98.57 | 99.61 | 99.76 | 86.00 | 74.40 | 53.57 | 91.55 |
GVT-Orig | 0.32 | 26.70 | 0.11 | 0.95 | 3.74 | 98.50 | 99.55 | 99.84 | 86.82 | 74.04 | 53.03 | 90.89 |
GVT-Ent | 0.32 | 30.76 | 0.08 | 0.65 | 2.48 | 98.62 | 99.56 | 99.81 | 87.32 | 73.07 | 50.70 | 91.52 |
GVT () | 0.33 | 30.46 | 0.10 | 0.73 | 3.56 | 98.42 | 99.34 | 99.62 | 87.03 | 74.12 | 53.28 | 91.04 |
GVT () | 0.35 | 29.78 | 0.16 | 1.06 | 4.27 | 98.55 | 99.63 | 99.91 | 87.25 | 75.10 | 54.12 | 91.63 |
Model | BLEU | Perplexity | Dist-1 | Dist-2 | Dist-3 | Intra-1 | Intra-2 | Intra-3 | Average | Greedy | Extrema | Coherence |
---|---|---|---|---|---|---|---|---|---|---|---|---|
S2S-Orig | 1.13 | 33.43 | 1.69 | 6.10 | 11.04 | 94.22 | 97.29 | 98.15 | 90.33 | 76.73 | 52.50 | 89.37 |
S2S-Ent | 1.49 | 34.75 | 1.20 | 4.44 | 8.08 | 93.64 | 96.70 | 97.52 | 90.72 | 76.25 | 51.52 | 90.22 |
S2S () | 1.35 | 34.04 | 1.57 | 5.79 | 10.27 | 95.84 | 97.92 | 98.83 | 90.31 | 76.43 | 52.37 | 89.45 |
S2S () | 1.22 | 33.74 | 1.66 | 6.18 | 11.31 | 96.19 | 98.50 | 99.04 | 90.27 | 76.77 | 52.46 | 89.38 |
CVAE-Orig | 1.23 | 29.36 | 2.77 | 10.53 | 19.98 | 94.64 | 98.00 | 98.59 | 90.74 | 76.15 | 51.08 | 90.38 |
CVAE-Ent | 1.28 | 32.40 | 2.66 | 9.60 | 17.74 | 94.28 | 97.86 | 98.57 | 90.67 | 76.00 | 51.13 | 90.55 |
CVAE () | 1.25 | 30.15 | 2.73 | 10.55 | 19.87 | 94.66 | 98.17 | 98.61 | 91.02 | 76.33 | 51.10 | 90.43 |
CVAE () | 1.26 | 29.43 | 2.81 | 10.57 | 20.13 | 94.71 | 98.13 | 98.62 | 91.35 | 76.54 | 51.12 | 90.47 |
TRS-Orig | 2.42 | 31.13 | 1.21 | 4.62 | 9.22 | 83.10 | 91.77 | 94.25 | 91.04 | 76.58 | 50.59 | 91.50 |
TRS-Ent | 2.49 | 31.79 | 0.89 | 3.48 | 6.90 | 79.85 | 89.26 | 92.46 | 91.20 | 76.58 | 49.73 | 91.43 |
TRS () | 2.40 | 31.70 | 0.93 | 4.20 | 8.07 | 83.21 | 91.29 | 93.63 | 91.26 | 76.51 | 49.90 | 91.11 |
TRS () | 2.44 | 31.01 | 1.25 | 5.07 | 10.32 | 83.31 | 92.12 | 94.75 | 91.43 | 76.85 | 50.14 | 91.15 |
GVT-Orig | 1.14 | 30.04 | 0.30 | 1.37 | 3.76 | 92.22 | 97.09 | 98.06 | 91.63 | 76.84 | 50.33 | 91.30 |
GVT-Ent | 1.29 | 30.63 | 0.26 | 1.23 | 3.23 | 90.71 | 95.48 | 96.59 | 91.56 | 76.41 | 50.28 | 91.36 |
GVT () | 1.25 | 30.25 | 0.29 | 1.34 | 3.72 | 92.08 | 96.45 | 97.86 | 91.52 | 76.53 | 50.34 | 91.34 |
GVT () | 1.32 | 30.13 | 0.36 | 1.45 | 3.84 | 92.56 | 97.43 | 98.21 | 91.48 | 77.43 | 50.62 | 91.31 |
3.5. Evaluation Measures
We use both automatic metrics and human judgement for evaluation in our experiments.
Automatic Metrics. We adopt several automatic metrics in existing work to measure the performance of dialogue generation models, including: (1) BLEU (Papineni et al., 2002) measures how much a generated response containing n-gram overlaps with the ground-truth response151515We use the script provided by Lin et al. (2020) to calculate the BLEU score.. (2) Perplexity (Serban et al., 2015) measures the high-level general quality of the generation model, and usually a relatively lower Perplexity value indicates a more fluent response. (3) Distinct (Li et al., 2016a) measures the degree of diversity. Specifically, we leverage Dist-1/2/3 (Gu et al., 2019) to compute the average of distinct values within each generated response. (4) Embedding-based metrics (Serban et al., 2017; Liu et al., 2016; Xu et al., 2018) contain three metrics to compute the word embedding similarity between the generated response and the ground truth (Liu et al., 2016): 1. Greedy: greedily matching words in two utterances based on the cosine similarities between their embeddings; 2. Average: cosine similarity between the averaged word embeddings in the two utterances; 3. Extrema: cosine similarity between the largest extreme values among the word embeddings in the two utterances161616We employ a popular NLG evaluation project available at https://github.com/Maluuba/nlg-eval for automatic evaluation.. Inspired by Xu et al. (2018), we use Coherence to calculate the cosine distance of two semantic vectors of a context and the generated response171717The definition of Coherence in Xu et al. (2018) is similar to that of Relatedness in Section 2.3.. We use GloVe vectors pre-trained on Twitter as the word embeddings (Pennington et al., 2014).
Human Evaluation. A human evaluation is also conducted to validate the effectiveness of our proposed method. Firstly, we randomly sample 100 contexts from the test set and get the generated responses of NCMs trained on either filtered dataset or original dataset. Next, we send pairs of the context and generated response to three professional annotators without orders. Annotators are then required to evaluate among “Win” (response1 is better), “Loss” (response2 is better) and “Tie” (they are equally good or bad) independently, considering four aspects: Relatedness, Consistency, Fluency and Informativeness. Relatedness evaluates whether the generated responses are relevant on topic with its contexts; Consistency measures whether the generated responses contain factual contradictions with respect to its contexts; Fluency assesses the grammatical correctness and readability of the generated responses; Informativeness indicates whether the generated responses are informative and not generic. The result on each sample is determined by majority voting. Finally, we calculate the percentage of samples where an NCM trained on filtered or original dataset generates the better response and where an NCM performs similarly on filtered and original datasets.
4. Experimental Results
In this section, we demonstrate our experimental results on two datasets: OpenSubtitles and DailyDialog, including automatic evaluation results, human evaluation results, and case study on data filtering and dialogue generation.
Filtered vs. Original | Win | Loss | Tie | Kappa | Filtered vs. Original | Win | Loss | Tie | Kappa | ||
---|---|---|---|---|---|---|---|---|---|---|---|
(1) | S2S | 43% | 15% | 42% | 0.514 | (2) | S2S | 43% | 11% | 46% | 0.462 |
CVAE | 48% | 7% | 45% | 0.493 | CVAE | 51% | 9% | 40% | 0.584 | ||
TRS | 52% | 8% | 40% | 0.596 | TRS | 45% | 12% | 43% | 0.497 | ||
GVT | 47% | 10% | 43% | 0.565 | GVT | 56% | 8% | 36% | 0.515 |
Context | Response | |||
---|---|---|---|---|
(1) | 1 | Get on with it. | No, No, No, No, No, No, No, No. | -1.2063 |
2 | Does he roll? Does he talk in his sleep? I don’t know. | There’s a spider! | 0.0010 | |
3 | Well, what do you want me to do? | Go back to harrow alley. Talk to Mrs.Goldstein. | 0.0748 | |
4 | What? The favorite flower for your bouquet. Oh, well! | Red roses. | 1.6667 | |
(2) | 5 | You can get it from the student business office. | I didn’t know that. | -0.6359 |
6 | That’s all right. I understand. | I’m so sorry. | -0.4400 | |
7 | I’m feeling hungry. | Isn’t it time for dinner? | 0.7346 | |
8 | How many rooms are there? | Eight rooms, …, three sweet bedrooms. | 0.9264 |
Context | Response-O | Response-F | ||
---|---|---|---|---|
(1) | 1 | Where were you? On the toilet. | I’m not going to get it. | I was just trying to get you. |
2 | She’s in shock! Okay? | She’s a good idea. | I’m sorry about that. | |
3 | They’re changing the guard over. | They ’re not going to be a good idea. | Then they’re not going to be here. | |
4 | I totally need my board. I gotta go find it. | I’m not going to get it. | OK, I’ll see you later. | |
(2) | 5 | So, Paula, where are you from? | I’m in the middle of the city. | I’m from Beijing. |
6 | Hey man, you wanna buy some weed? | I’ m sorry. I don’t know what to do. | I’ m sorry. I’m going to buy a new car. | |
7 | … I have a terrible headache. | I’m sorry, Sir. | I’m sorry to hear that. | |
8 | Excuse me, may I help you ? | Yes, I’d like to buy a new one. | Yes, I’d like to book a book. |
4.1. Automatic Evaluation Results (RQ1)
We list the results of automatic evaluation on both the OpenSubtitles and DailyDialog datasets in Table 3 and 4, respectively. From the results, we have three main observations.
First, compared with training on original datasets, our data filtering method can not only bring improvements for all the four NCMs on almost all the evaluation metrics (t-test, p-value 0.05), but also achieve competitive performance across two datasets. This also affirms the generalization ability of our proposed method.
Second, when it comes to the comparison between our method and a comparable data filtering approach (“-Ent”) (Csáky et al., 2019), our method can also achieve better performance. The work of Csáky et al. (2019) aims to solve the “general response” problem and generate more diverse responses in open-domain conversations. Therefore, the higher Diversity scores, i.e., Dist-1/2/3 and Intra-1/2/3, further reflect the effectiveness of our proposed method.
Third, we also notice that the absolute improvement of Dist-1/2/3 value on OpenSubtitles is large (e.g., up to 0.12%/0.62%/1.32% based on the TRS model), but for DailyDialog, the improvement is relatively small. We conjecture that OpenSubtitles benefits more from the data filtering method than DailyDialog, as DailyDialog is manually-collected and many samples of it are in high quality, while OpenSubtitles is more complex and contains a larger amount of low-quality samples.
4.2. Human Evaluation Results (RQ1)
We also conduct pairwise human evaluation to confirm the improvement of our method, and the results on two datasets are shown in Table 5.
We observe that training on filtered datasets outperforms training on original datasets for all the four NCMs as the percentage of “Win” is much larger than that of “Loss”. The kappa scores indicate that the annotators came to a moderate agreement in the judgment. Meanwhile, as the DailyDialog dataset contains less low-quality training samples, the percentage of “Tie” is also high, showing that training on original or filtered dataset has similar performance. The human evaluation here is based on the overall sample quality, and we leave the fine-grained comparisons for the future work.
Opponent | Kendall | Opponent | Kendall | Opponent | Kendall |
---|---|---|---|---|---|
Specificity vs. Repetitiveness | 0.039 | Specificity vs. Relatedness | -0.044 | Specificity vs. Continuity | -0.057 |
Specificity vs. Coherence | -0.333 | Specificity vs. Fluency | -0.451 | Specificity vs. Consistency | 0.005 |
Repetitiveness vs. Relatedness | 0.011 | Repetitiveness vs. Continuity | 0.011 | Repetitiveness vs. Coherence | -0.274 |
Repetitiveness vs. Fluency | 0.011 | Repetitiveness vs. Consistency | 0.025 | Relatedness vs. Continuity | 0.102 |
Relatedness vs. Coherence | 0.104 | Relatedness vs. Fluency | 0.005 | Relatedness vs. Consistency | 0.090 |
Continuity vs. Coherence | 0.047 | Continuity vs. Fluency | 0.027 | Continuity vs. Consistency | 0.035 |
Coherence vs. Fluency | 0.281 | Coherence vs. Consistency | -0.002 | Fluency vs. Consistency | 0.009 |
4.3. Case Study on Data Filtering (RQ2)
To better understand whether our method can identify untrustworthy samples, and even sort training samples based on data quality, we conduct some case studies on both OpenSubtitles and DailyDialog datasets.
As shown in Table 6, we can see that cases with repetitive words, unrelated contents, or generic expressions, named as untrustworthy samples in our work, are scored with low scores. In contrast, informative and related cases are likely to have high scores. The results here are correlated with human judgement, which indicates the effectiveness of our designed measure that takes several attributes into consideration to assess the quality of a training sample.
Filtered by | BLEU | Perplexity | Dist-1 | Dist-2 | Dist-3 | Intra-1 | Intra-2 | Intra-3 | Avg. | Gre. | Ext. | Coh. |
---|---|---|---|---|---|---|---|---|---|---|---|---|
None | 0.46 | 48.98 | 0.05 | 0.16 | 0.27 | 99.03 | 99.58 | 99.72 | 87.57 | 73.81 | 52.67 | 91.07 |
Specificity | 0.42 | 51.65 | 0.05 | 0.15 | 0.27 | 98.33 | 99.31 | 98.91 | 87.97 | 73.84 | 50.98 | 91.50 |
Repetitiveness | 0.61 | 47.24 | 0.09 | 0.34 | 0.63 | 99.26 | 99.76 | 99.86 | 86.00 | 72.30 | 50.47 | 90.28 |
Relatedness | 0.55 | 47.48 | 0.08 | 0.27 | 0.51 | 92.15 | 94.81 | 97.16 | 86.01 | 72.41 | 51.21 | 90.37 |
Continuity | 0.83 | 47.12 | 0.09 | 0.36 | 0.69 | 97.80 | 98.55 | 98.90 | 87.34 | 74.15 | 53.31 | 91.34 |
Coherence | 0.65 | 47.07 | 0.11 | 0.46 | 0.93 | 97.79 | 98.91 | 99.35 | 86.58 | 73.29 | 51.92 | 91.37 |
Fluency | 0.68 | 47.16 | 0.12 | 0.51 | 1.08 | 98.16 | 98.81 | 99.01 | 85.34 | 73.01 | 52.97 | 89.70 |
Consistency | 0.71 | 47.18 | 0.11 | 0.47 | 0.93 | 97.77 | 98.69 | 98.92 | 86.33 | 72.79 | 50.90 | 91.20 |
0.80 | 46.06 | 0.17 | 0.78 | 1.70 | 98.57 | 99.61 | 99.76 | 86.00 | 74.40 | 53.57 | 91.55 |
4.4. Case Study on Dialogue Generation (RQ3)
The ultimate goal of our work is to improve the open-domain dialogue generation with data filtering method. To further check the performance of NCMs trained on filtered datasets, we show some responses generated by the TRS model on both OpenSubtitles and DailyDialog datasets in Table 7.
“Response-O” and “Response-F” denote responses generated by a TRS model that is trained on original datasets and filtered datasets, respectively. In general, responses under “Response-F” are more informative and related to the corresponding contexts. Take Case 1 as an example. The context contains a question “Where were you?”, and the response is “I was just trying to get you”. The past tenses and contents in these two utterances make them highly coherent with each other. However, “I’m not going to get it” is irrelevant to the context, especially with a strange “it” that confuses people a lot.
5. Further Analysis
We conduct some further analyses to validate the effectiveness of our method.
5.1. Correlation among Attributes (RQ4)
Seven dialogue attributes are combined together when we define in Section 2.3. To show their relations, we calculate the Kendall correlations of any two attributes inspired by Cai et al. (2020). Table 8 illustrates the results on OpenSubtitles.
We see that the maximum absolute value of correlations is 0.451 between Specificity and Fluency, and other correlation values are around 0.1. This demonstrates that these attributes, in general, do not show strong correlations with each other. At the same time, it partially validates that dialogue quality is reflected in multiple facets. Besides, the negative correlation of Specificity and Fluency is also consistent with people’s cognition, as a generic response has a higher probability to be fluent.
5.2. Single vs. Multiple Attributes (RQ5)
To gain some insights into the effects of seven dialogue attributes on our proposed filtering method, we conduct the ablation study using TRS by only exploiting a single attribute as the measure to score each training sample. Table 9 reports the results on Opensubtitles.
We observe that data filtering can lead to better performance, even with only one attribute. When applying the measure that combines seven attributes, the performance is the best on most of automatic metrics, including Perplexity, Diversity metrics, and Embedding-based metrics representing fluency, diversity, and relatedness, respectively,
5.3. -value Curves of Optimization (RQ6)
To get some intuitive feelings of the optimization procedure, Figure 3 shows the “metric” value, the sum of 13 open-domain automatic metrics, for model TRS on OpenSubtitles. The red line denotes the hypothesis space exploration of Bayesian Optimization for the “metric” value181818Bayesian Optimization aims to minimize “-metric” value, while here we plot the increase of “metric” value., while the blue line displays the overall best value on the validation set.
We find that Bayesian Optimization here results in a large explored space (more variance), and the “metric” value keeps increasing as the iteration continues. This plot can not only illustrate the optimization produce, but also implicitly validate the effectiveness of DIFF-MLE-NEG, as Bayesian Optimization can work normally.
6. Related Work
Our work is related to two research branches: Data Filtering for Noisy Corpora, and Metrics for Automatic Dialogue Evaluation.
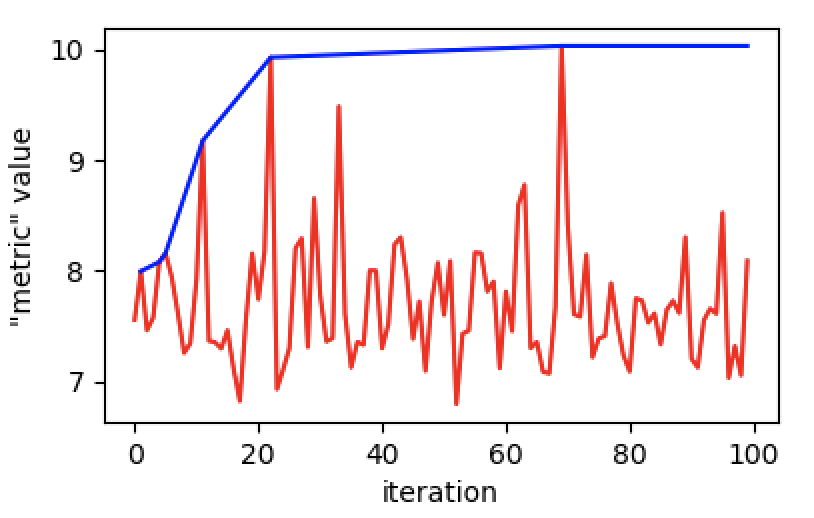
6.1. Data Filtering for Noisy Corpora.
To better utilize noisy dialogue corpora, model- and data-based approaches have been proposed. The former one use models to handle noise during the training process. Shang et al. (2018) integrated a calibration network into a generation network. The calibration network is trained to measure the quality of the context-response pairs, and the generation network takes the scores produced by the calibration network to weight the training samples, such that the high-quality samples have more impacts on the generation model while the low-quality ones are less influential. The latter one aims to improve the data quality by pre-processing before feeding it into a model. Xu et al. (2018) introduced Coherence with cosine similarity to measure the semantic relationship between contexts and responses, and then filtered out pairs with low coherence score. Csáky et al. (2019) used entropy to find out the most generic contexts or responses, and then filtered out pairs with high entropy. Akama et al. (2020) defined Connectivity (C) and Relatedness (R) with normalized pointwise mutual information (Bouma, 2009) and cosine similarity to remove noisy pairs with low C+R score. Li et al. (2017a) proposed an iteration-based way to distill data, which operates as: a neural sequence-to-sequence (S2S) model is first trained and used to generate responses to inputs in a dataset. Then a list of the most common responses is constructed, and training samples with outputs that are semantically close to these common responses are removed. As the process iterates, responses that are generic are gradually distilled, and the trained models gradually increase in specificity.
The differences between our method and the above listed ones are: (1) The proposed measure does not rely on only one or two attributes, it integrates seven dialogue attributes to estimate the quality of dialogue samples. (2) We frame the dialogue data filtering task as an optimization problem, and use Bayesian Optimization to find the optimal weights in the linear combination of .
6.2. Metrics for Automatic Dialogue Evaluation.
The evaluation of open-domain dialogue generation generally consists of both automatic metrics and human judgement. Since human evaluation has high variance, high cost, and is difficult to replicate, some researchers aim to propose automatic metrics that correlate more with human intuition for this task. Dialogue quality is inherently multi-faceted (Walker et al., 1997; See et al., 2019; Cai et al., 2020), including fluency, coherence, diversity, consistency, etc. Inspired by the automatic evaluation conducted in other fields, referenced automatic metrics are applied (Papineni et al., 2002; Ghazarian et al., 2019; Tao et al., 2018). Recently, unreferenced metrics are also proposed to assess different dialogue attributes, such as Distinct (Li et al., 2016a), Entropy (Pang et al., 2020), MAUDE (Sinha et al., 2020), USR (Mehri and Eskenazi, 2020b), and FED (Mehri and Eskenazi, 2020a). The last three ones also rely on pre-trained language models, and have higher correlation with human judgement. However, compared with some simple metrics, these new metrics are not widely used and thoroughly accepted by this community.
Our work does not aim to propose a new automatic metric for open-domain dialogue generation, we only utilize some of them to help define the measure or objective function . In addition to the options we choose in Section 2.3, other metrics like FED can also be used to compute or , and we leave this for the future work.
7. Conclusion and Future Work
In this paper, we present a data filtering method for open-domain dialogues, which aims to identify untrustworthy training samples with a quality measure that linearly combines seven dialogue attributes. The attribute weights are obtained via Bayesian Optimization. Besides, to accelerate the optimization iterations on large-scale datasets, we propose a training method that integrates the maximum likelihood estimation (MLE) and negative training method (NEG). Experimental results on two widely-used datasets show that our method can effectively identify untrustworthy samples, and NCMs trained on the filtered datasets can further generate fluent, related, and informative responses. In the future, we will try to utilize some newly proposed automatic metrics for open-domain dialogue generation, and combine them in the definition of the quality measure and the choice of the objective function.
References
- (1)
- Akama et al. (2020) Reina Akama, Sho Yokoi, Jun Suzuki, and Kentaro Inui. 2020. Filtering Noisy Dialogue Corpora by Connectivity and Content Relatedness. In Proceedings of the 2020 Conference on Empirical Methods in Natural Language Processing (EMNLP). Association for Computational Linguistics, Online, 941–958.
- Arora et al. (2017) Sanjeev Arora, Yingyu Liang, and Tengyu Ma. 2017. A Simple but Tough-to-Beat Baseline for Sentence Embeddings. In 5th International Conference on Learning Representations, ICLR 2017, Toulon, France, April 24-26, 2017, Conference Track Proceedings. OpenReview.net.
- Bahdanau et al. (2015) Dzmitry Bahdanau, Kyunghyun Cho, and Yoshua Bengio. 2015. Neural Machine Translation by Jointly Learning to Align and Translate. In 3rd International Conference on Learning Representations, ICLR 2015, San Diego, CA, USA, May 7-9, 2015, Conference Track Proceedings, Yoshua Bengio and Yann LeCun (Eds.).
- Bouma (2009) Gerlof Bouma. 2009. Normalized (pointwise) mutual information in collocation extraction. (2009).
- Bowman et al. (2015) Samuel R. Bowman, Gabor Angeli, Christopher Potts, and Christopher D. Manning. 2015. A large annotated corpus for learning natural language inference. In Proceedings of the 2015 Conference on Empirical Methods in Natural Language Processing. Association for Computational Linguistics, Lisbon, Portugal, 632–642.
- Brochu et al. (2010) Eric Brochu, Vlad M Cora, and Nando De Freitas. 2010. A tutorial on Bayesian optimization of expensive cost functions, with application to active user modeling and hierarchical reinforcement learning. arXiv preprint arXiv:1012.2599 (2010).
- Cai et al. (2020) Hengyi Cai, Hongshen Chen, Cheng Zhang, Yonghao Song, Xiaofang Zhao, Yangxi Li, Dongsheng Duan, and Dawei Yin. 2020. Learning from Easy to Complex: Adaptive Multi-Curricula Learning for Neural Dialogue Generation. In The Thirty-Fourth AAAI Conference on Artificial Intelligence, AAAI 2020, The Thirty-Second Innovative Applications of Artificial Intelligence Conference, IAAI 2020, The Tenth AAAI Symposium on Educational Advances in Artificial Intelligence, EAAI 2020, New York, NY, USA, February 7-12, 2020. AAAI Press, 7472–7479.
- Chen et al. (2020) Meng Chen, Ruixue Liu, Lei Shen, Shaozu Yuan, Jingyan Zhou, Youzheng Wu, Xiaodong He, and Bowen Zhou. 2020. The JDDC Corpus: A Large-Scale Multi-Turn Chinese Dialogue Dataset for E-commerce Customer Service. In Proceedings of the 12th Language Resources and Evaluation Conference. European Language Resources Association, Marseille, France, 459–466.
- Cho et al. (2014) Kyunghyun Cho, Bart van Merriënboer Caglar Gulcehre, Dzmitry Bahdanau, Fethi Bougares Holger Schwenk, and Yoshua Bengio. 2014. Learning Phrase Representations using RNN Encoder–Decoder for Statistical Machine Translation. In Proceedings of the 2014 Conference on Empirical Methods in Natural Language Processing. 1724–1734.
- Csáky et al. (2019) Richárd Csáky, Patrik Purgai, and Gábor Recski. 2019. Improving Neural Conversational Models with Entropy-Based Data Filtering. In Proceedings of the 57th Annual Meeting of the Association for Computational Linguistics. Association for Computational Linguistics, Florence, Italy, 5650–5669.
- Fleiss and Cohen (1973) Joseph L Fleiss and Jacob Cohen. 1973. The equivalence of weighted kappa and the intraclass correlation coefficient as measures of reliability. Educational and psychological measurement 33, 3 (1973), 613–619.
- Ghazarian et al. (2019) Sarik Ghazarian, Johnny Tian-Zheng Wei, Aram Galstyan, and Nanyun Peng. 2019. Better Automatic Evaluation of Open-Domain Dialogue Systems with Contextualized Embeddings. NAACL HLT 2019 (2019), 82.
- Gu et al. (2019) Xiaodong Gu, Kyunghyun Cho, Jung-Woo Ha, and Sunghun Kim. 2019. DialogWAE: Multimodal Response Generation with Conditional Wasserstein Auto-Encoder. In 7th International Conference on Learning Representations, ICLR 2019, New Orleans, LA, USA, May 6-9, 2019. OpenReview.net.
- Gulrajani et al. (2017) Ishaan Gulrajani, Faruk Ahmed, Martin Arjovsky, Vincent Dumoulin, and Aaron Courville. 2017. Improved training of wasserstein GANs. In Proceedings of the 31st International Conference on Neural Information Processing Systems. 5769–5779.
- He and Glass (2020) Tianxing He and James Glass. 2020. Negative Training for Neural Dialogue Response Generation. In Proceedings of the 58th Annual Meeting of the Association for Computational Linguistics. Association for Computational Linguistics, Online, 2044–2058.
- Henderson et al. (2019) Matthew Henderson, Paweł Budzianowski, Iñigo Casanueva, Sam Coope, Daniela Gerz, Girish Kumar, Nikola Mrkšić, Georgios Spithourakis, Pei-Hao Su, Ivan Vulić, and Tsung-Hsien Wen. 2019. A Repository of Conversational Datasets. In Proceedings of the First Workshop on NLP for Conversational AI. Association for Computational Linguistics, Florence, Italy, 1–10.
- Ke et al. (2018) Pei Ke, Jian Guan, Minlie Huang, and Xiaoyan Zhu. 2018. Generating Informative Responses with Controlled Sentence Function. In Proceedings of the 56th Annual Meeting of the Association for Computational Linguistics (Volume 1: Long Papers). Association for Computational Linguistics, Melbourne, Australia, 1499–1508.
- Li et al. (2016a) Jiwei Li, Michel Galley, Chris Brockett, Jianfeng Gao, and Bill Dolan. 2016a. A Diversity-Promoting Objective Function for Neural Conversation Models. In Proceedings of the 2016 Conference of the North American Chapter of the Association for Computational Linguistics: Human Language Technologies. Association for Computational Linguistics, San Diego, California, 110–119.
- Li et al. (2016b) Jiwei Li, Michel Galley, Chris Brockett, Georgios Spithourakis, Jianfeng Gao, and Bill Dolan. 2016b. A Persona-Based Neural Conversation Model. In Proceedings of the 54th Annual Meeting of the Association for Computational Linguistics (Volume 1: Long Papers). Association for Computational Linguistics, Berlin, Germany, 994–1003.
- Li et al. (2017a) Jiwei Li, Will Monroe, and Dan Jurafsky. 2017a. Data distillation for controlling specificity in dialogue generation. arXiv preprint arXiv:1702.06703 (2017).
- Li et al. (2017b) Yanran Li, Hui Su, Xiaoyu Shen, Wenjie Li, Ziqiang Cao, and Shuzi Niu. 2017b. DailyDialog: A Manually Labelled Multi-turn Dialogue Dataset. In Proceedings of the Eighth International Joint Conference on Natural Language Processing (Volume 1: Long Papers). Asian Federation of Natural Language Processing, Taipei, Taiwan, 986–995.
- Lin et al. (2020) Zhaojiang Lin, Genta Indra Winata, Peng Xu, Zihan Liu, and Pascale Fung. 2020. Variational transformers for diverse response generation. arXiv preprint arXiv:2003.12738 (2020).
- Lison and Tiedemann (2016) Pierre Lison and Jörg Tiedemann. 2016. OpenSubtitles2016: Extracting Large Parallel Corpora from Movie and TV Subtitles. In Proceedings of the Tenth International Conference on Language Resources and Evaluation (LREC’16). European Language Resources Association (ELRA), Portorož, Slovenia, 923–929.
- Liu et al. (2016) Chia-Wei Liu, Ryan Lowe, Iulian Serban, Mike Noseworthy, Laurent Charlin, and Joelle Pineau. 2016. How NOT To Evaluate Your Dialogue System: An Empirical Study of Unsupervised Evaluation Metrics for Dialogue Response Generation. In Proceedings of the 2016 Conference on Empirical Methods in Natural Language Processing. Association for Computational Linguistics, Austin, Texas, 2122–2132.
- Liu et al. (2020) Ruixue Liu, Meng Chen, Hang Liu, Lei Shen, Yang Song, and Xiaodong He. 2020. Enhancing Multi-turn Dialogue Modeling with Intent Information for E-Commerce Customer Service. In CCF International Conference on Natural Language Processing and Chinese Computing. Springer, 65–77.
- Liu et al. (2019b) Yinhan Liu, Myle Ott, Naman Goyal, Jingfei Du, Mandar Joshi, Danqi Chen, Omer Levy, Mike Lewis, Luke Zettlemoyer, and Veselin Stoyanov. 2019b. RoBERTa: A Robustly Optimized BERT Pretraining Approach. (2019).
- Liu et al. (2019a) Zhibin Liu, Zheng-Yu Niu, Hua Wu, and Haifeng Wang. 2019a. Knowledge Aware Conversation Generation with Explainable Reasoning over Augmented Graphs. In Proceedings of the 2019 Conference on Empirical Methods in Natural Language Processing and the 9th International Joint Conference on Natural Language Processing (EMNLP-IJCNLP). Association for Computational Linguistics, Hong Kong, China, 1782–1792.
- Mehri and Eskenazi (2020a) Shikib Mehri and Maxine Eskenazi. 2020a. Unsupervised Evaluation of Interactive Dialog with DialoGPT. In Proceedings of the 21th Annual Meeting of the Special Interest Group on Discourse and Dialogue. Association for Computational Linguistics, 1st virtual meeting, 225–235.
- Mehri and Eskenazi (2020b) Shikib Mehri and Maxine Eskenazi. 2020b. USR: An Unsupervised and Reference Free Evaluation Metric for Dialog Generation. In Proceedings of the 58th Annual Meeting of the Association for Computational Linguistics. Association for Computational Linguistics, Online, 681–707.
- Meng et al. (2020) Chuan Meng, Pengjie Ren, Zhumin Chen, Christof Monz, Jun Ma, and Maarten de Rijke. 2020. Refnet: A reference-aware network for background based conversation. In Proceedings of the AAAI Conference on Artificial Intelligence, Vol. 34. 8496–8503.
- Mockus (1975) J Mockus. 1975. On the Bayes methods for seeking the extremal point. IFAC Proceedings Volumes 8, 1 (1975), 428–431.
- Pang et al. (2020) Bo Pang, Erik Nijkamp, Wenjuan Han, Linqi Zhou, Yixian Liu, and Kewei Tu. 2020. Towards Holistic and Automatic Evaluation of Open-Domain Dialogue Generation. In Proceedings of the 58th Annual Meeting of the Association for Computational Linguistics. Association for Computational Linguistics, Online, 3619–3629.
- Papineni et al. (2002) Kishore Papineni, Salim Roukos, Todd Ward, and Wei-Jing Zhu. 2002. Bleu: a Method for Automatic Evaluation of Machine Translation. In Proceedings of the 40th Annual Meeting of the Association for Computational Linguistics. Association for Computational Linguistics, Philadelphia, Pennsylvania, USA, 311–318.
- Pennington et al. (2014) Jeffrey Pennington, Richard Socher, and Christopher Manning. 2014. GloVe: Global Vectors for Word Representation. In Proceedings of the 2014 Conference on Empirical Methods in Natural Language Processing (EMNLP). Association for Computational Linguistics, Doha, Qatar, 1532–1543.
- Radford et al. (2019) Alec Radford, Jeffrey Wu, Rewon Child, David Luan, Dario Amodei, and Ilya Sutskever. 2019. Language models are unsupervised multitask learners. (2019).
- Reed et al. (2018) Lena Reed, Shereen Oraby, and Marilyn Walker. 2018. Can Neural Generators for Dialogue Learn Sentence Planning and Discourse Structuring?. In Proceedings of the 11th International Conference on Natural Language Generation. 284–295.
- Ruder and Plank (2017) Sebastian Ruder and Barbara Plank. 2017. Learning to select data for transfer learning with Bayesian Optimization. In Proceedings of the 2017 Conference on Empirical Methods in Natural Language Processing. Association for Computational Linguistics, Copenhagen, Denmark, 372–382.
- See et al. (2019) Abigail See, Stephen Roller, Douwe Kiela, and Jason Weston. 2019. What makes a good conversation? How controllable attributes affect human judgments. In Proceedings of the 2019 Conference of the North American Chapter of the Association for Computational Linguistics: Human Language Technologies, Volume 1 (Long and Short Papers). Association for Computational Linguistics, Minneapolis, Minnesota, 1702–1723.
- Serban et al. (2015) Iulian V Serban, Alessandro Sordoni, Yoshua Bengio, Aaron Courville, and Joelle Pineau. 2015. Hierarchical neural network generative models for movie dialogues. arXiv preprint arXiv:1507.04808 7, 8 (2015), 434–441.
- Serban et al. (2017) Iulian Vlad Serban, Alessandro Sordoni, Ryan Lowe, Laurent Charlin, Joelle Pineau, Aaron C. Courville, and Yoshua Bengio. 2017. A Hierarchical Latent Variable Encoder-Decoder Model for Generating Dialogues. In Proceedings of the Thirty-First AAAI Conference on Artificial Intelligence, February 4-9, 2017, San Francisco, California, USA, Satinder P. Singh and Shaul Markovitch (Eds.). AAAI Press, 3295–3301.
- Shahriari et al. (2015) Bobak Shahriari, Kevin Swersky, Ziyu Wang, Ryan P Adams, and Nando De Freitas. 2015. Taking the human out of the loop: A review of Bayesian optimization. Proc. IEEE 104, 1 (2015), 148–175.
- Shang et al. (2018) Mingyue Shang, Zhenxin Fu, Nanyun Peng, Yansong Feng, Dongyan Zhao, and Rui Yan. 2018. Learning to Converse with Noisy Data: Generation with Calibration. In Proceedings of the Twenty-Seventh International Joint Conference on Artificial Intelligence, IJCAI 2018, July 13-19, 2018, Stockholm, Sweden, Jérôme Lang (Ed.). ijcai.org, 4338–4344.
- Shen and Feng (2020) Lei Shen and Yang Feng. 2020. CDL: Curriculum Dual Learning for Emotion-Controllable Response Generation. In Proceedings of the 58th Annual Meeting of the Association for Computational Linguistics. 556–566.
- Shen et al. (2019) Lei Shen, Yang Feng, and Haolan Zhan. 2019. Modeling Semantic Relationship in Multi-turn Conversations with Hierarchical Latent Variables. In Proceedings of the 57th Annual Meeting of the Association for Computational Linguistics. 5497–5502.
- Shen et al. (2021a) Lei Shen, Haolan Zhan, Xin Shen, and Yang Feng. 2021a. Learning to select context in a hierarchical and global perspective for open-domain dialogue generation. In ICASSP 2021-2021 IEEE International Conference on Acoustics, Speech and Signal Processing (ICASSP). IEEE, 7438–7442.
- Shen et al. (2021b) Lei Shen, Haolan Zhan, Xin Shen, Yonghao Song, and Xiaofang Zhao. 2021b. Text is NOT Enough: Integrating Visual Impressions into Open-domain Dialogue Generation. arXiv preprint arXiv:2109.05778 (2021).
- Sinha et al. (2020) Koustuv Sinha, Prasanna Parthasarathi, Jasmine Wang, Ryan Lowe, William L. Hamilton, and Joelle Pineau. 2020. Learning an Unreferenced Metric for Online Dialogue Evaluation. In Proceedings of the 58th Annual Meeting of the Association for Computational Linguistics. Association for Computational Linguistics, Online, 2430–2441.
- Song et al. (2020) Haoyu Song, Wei-Nan Zhang, Jingwen Hu, and Ting Liu. 2020. Generating persona consistent dialogues by exploiting natural language inference. In Proceedings of the AAAI Conference on Artificial Intelligence, Vol. 34. 8878–8885.
- Tao et al. (2018) Chongyang Tao, Lili Mou, Dongyan Zhao, and Rui Yan. 2018. RUBER: An Unsupervised Method for Automatic Evaluation of Open-Domain Dialog Systems. In Proceedings of the Thirty-Second AAAI Conference on Artificial Intelligence, (AAAI-18), the 30th innovative Applications of Artificial Intelligence (IAAI-18), and the 8th AAAI Symposium on Educational Advances in Artificial Intelligence (EAAI-18), New Orleans, Louisiana, USA, February 2-7, 2018, Sheila A. McIlraith and Kilian Q. Weinberger (Eds.). AAAI Press, 722–729.
- Tsvetkov et al. (2016) Yulia Tsvetkov, Manaal Faruqui, Wang Ling, Brian MacWhinney, and Chris Dyer. 2016. Learning the Curriculum with Bayesian Optimization for Task-Specific Word Representation Learning. In Proceedings of the 54th Annual Meeting of the Association for Computational Linguistics (Volume 1: Long Papers). Association for Computational Linguistics, Berlin, Germany, 130–139.
- Vaswani et al. (2017) Ashish Vaswani, Noam Shazeer, Niki Parmar, Jakob Uszkoreit, Llion Jones, Aidan N. Gomez, Lukasz Kaiser, and Illia Polosukhin. 2017. Attention is All you Need. In Advances in Neural Information Processing Systems 30: Annual Conference on Neural Information Processing Systems 2017, December 4-9, 2017, Long Beach, CA, USA, Isabelle Guyon, Ulrike von Luxburg, Samy Bengio, Hanna M. Wallach, Rob Fergus, S. V. N. Vishwanathan, and Roman Garnett (Eds.). 5998–6008.
- Walker et al. (1997) Marilyn Walker, Diane Litman, Candace A Kamm, and Alicia Abella. 1997. PARADISE: A Framework for Evaluating Spoken Dialogue Agents. In 35th Annual Meeting of the Association for Computational Linguistics and 8th Conference of the European Chapter of the Association for Computational Linguistics. 271–280.
- Welleck et al. (2019) Sean Welleck, Jason Weston, Arthur Szlam, and Kyunghyun Cho. 2019. Dialogue Natural Language Inference. In Proceedings of the 57th Annual Meeting of the Association for Computational Linguistics. Association for Computational Linguistics, Florence, Italy, 3731–3741.
- Xu et al. (2018) Xinnuo Xu, Ondřej Dušek, Ioannis Konstas, and Verena Rieser. 2018. Better Conversations by Modeling, Filtering, and Optimizing for Coherence and Diversity. In Proceedings of the 2018 Conference on Empirical Methods in Natural Language Processing. Association for Computational Linguistics, Brussels, Belgium, 3981–3991.
- Zhan et al. (2021a) Haolan Zhan, Hainan Zhang, Hongshen Chen, Zhuoye Ding, Yongjun Bao, and Yanyan Lan. 2021a. Augmenting knowledge-grounded conversations with sequential knowledge transition. In Proceedings of the 2021 Conference of the North American Chapter of the Association for Computational Linguistics: Human Language Technologies. 5621–5630.
- Zhan et al. (2021b) Haolan Zhan, Hainan Zhang, Hongshen Chen, Zhuoye Ding, Yongjun Bao, and Yanyan Lan. 2021b. Augmenting knowledge-grounded conversations with sequential knowledge transition. In Proceedings of the 2021 Conference of the North American Chapter of the Association for Computational Linguistics: Human Language Technologies. 5621–5630.
- Zhang et al. (2018) Yizhe Zhang, Michel Galley, Jianfeng Gao, Zhe Gan, Xiujun Li, Chris Brockett, and Bill Dolan. 2018. Generating Informative and Diverse Conversational Responses via Adversarial Information Maximization. In Advances in Neural Information Processing Systems 31: Annual Conference on Neural Information Processing Systems 2018, NeurIPS 2018, December 3-8, 2018, Montréal, Canada, Samy Bengio, Hanna M. Wallach, Hugo Larochelle, Kristen Grauman, Nicolò Cesa-Bianchi, and Roman Garnett (Eds.). 1815–1825.
- Zhao et al. (2020) Tianyu Zhao, Divesh Lala, and Tatsuya Kawahara. 2020. Designing Precise and Robust Dialogue Response Evaluators. In Proceedings of the 58th Annual Meeting of the Association for Computational Linguistics. Association for Computational Linguistics, Online, 26–33.
- Zhao et al. (2017) Tiancheng Zhao, Ran Zhao, and Maxine Eskenazi. 2017. Learning Discourse-level Diversity for Neural Dialog Models using Conditional Variational Autoencoders. In Proceedings of the 55th Annual Meeting of the Association for Computational Linguistics (Volume 1: Long Papers). Association for Computational Linguistics, Vancouver, Canada, 654–664.
- Zhou et al. (2018a) Hao Zhou, Minlie Huang, Tianyang Zhang, Xiaoyan Zhu, and Bing Liu. 2018a. Emotional Chatting Machine: Emotional Conversation Generation with Internal and External Memory. In Proceedings of the Thirty-Second AAAI Conference on Artificial Intelligence, (AAAI-18), the 30th innovative Applications of Artificial Intelligence (IAAI-18), and the 8th AAAI Symposium on Educational Advances in Artificial Intelligence (EAAI-18), New Orleans, Louisiana, USA, February 2-7, 2018, Sheila A. McIlraith and Kilian Q. Weinberger (Eds.). AAAI Press, 730–739.
- Zhou et al. (2018b) Hao Zhou, Tom Young, Minlie Huang, Haizhou Zhao, Jingfang Xu, and Xiaoyan Zhu. 2018b. Commonsense Knowledge Aware Conversation Generation with Graph Attention. In Proceedings of the Twenty-Seventh International Joint Conference on Artificial Intelligence, IJCAI 2018, July 13-19, 2018, Stockholm, Sweden, Jérôme Lang (Ed.). ijcai.org, 4623–4629.