High-fidelity Facial Avatar Reconstruction from Monocular Video with Generative Priors
Abstract
High-fidelity facial avatar reconstruction from a monocular video is a significant research problem in computer graphics and computer vision. Recently, Neural Radiance Field (NeRF) has shown impressive novel view rendering results and has been considered for facial avatar reconstruction. However, the complex facial dynamics and missing 3D information in monocular videos raise significant challenges for faithful facial reconstruction. In this work, we propose a new method for NeRF-based facial avatar reconstruction that utilizes 3D-aware generative prior. Different from existing works that depend on a conditional deformation field for dynamic modeling, we propose to learn a personalized generative prior, which is formulated as a local and low dimensional subspace in the latent space of 3D-GAN. We propose an efficient method to construct the personalized generative prior based on a small set of facial images of a given individual. After learning, it allows for photo-realistic rendering with novel views and the face reenactment can be realized by performing navigation in the latent space. Our proposed method is applicable for different driven signals, including RGB images, 3DMM coefficients, and audios. Compared with existing works, we obtain superior novel view synthesis results and faithfully face reenactment performance.
![[Uncaptioned image]](https://cdn.awesomepapers.org/papers/402b742f-a96a-410d-9112-94e690d4a17c/x1.png)
1 Introduction
Reconstructing high-fidelity controllable 3D faces from a monocular video is significant in computer graphics and computer vision and has great potential in digital human, video conferencing and AR/VR applications. Yet it is very challenging, due to the complex facial dynamics and missing 3D information in monocular videos.
Recently, Neural Radiance Field (NeRF) [29] has shown impressive quality for novel view synthesis. The key idea of NeRF is to encode color and density as a function of spatial location and viewing direction by a neural network and adopt volume rendering technique for novel view synthesis. Its photo-realistic rendering ability has sparked great interest in facial avatar reconstruction. Starting with static face reconstruction, deformable neural radiance field has been proposed to handle the non-rigidly deforming faces captured in monocular videos. For example, the works of [33, 34] proposed to learn a conditional deformation field to capture the non-rigidly deformation of each frame. After training, they can provide novel view synthesis for the training frames. However, they don’t support facial editing and cannot be used for face reenactment.
The controllability of facial avatar is indispensable for many down-stream applications, such as talking head synthesis. To facilitate this, the core idea of existing works is to learn a dynamic neural radiance field conditioned on specific driven signals. For example, 3D morphable face model (3DMM) [3] is introduced as a guidance in NeRF-based facial avatar reconstruction [11, 2, 13]. The work of [11] learns a dynamic NeRF that directly conditioned on the pose and expression coefficients estimated by 3DMM. In RigNeRF [2], the deformation field is a combination of a pre-calculated 3DMM deformation field prior and a learned residual conditioned on the pose and expression coefficients. After modeling, one can use 3DMM coefficients for face reenactment. In addition to the explicit 3DMM coefficients, audio-driven dynamic NeRF has also been studied [17, 40]. Recently, AD-NeRF [17] is proposed to optimize a dynamic neural radiance field by augmenting the input with audio features. DFRF [40] further considers the few-shot audio-driven talking head synthesis scenario. These works directly learn a conditional deformation field and scene representation in the continuous 5D space. However, recovering 3D information from monocular videos is an ill-posed problem, it is very challenging to obtain high-fidelity facial avatar.
To alleviate the aforementioned challenges, we propose to adopt 3D generative prior. Recently, 3D-aware generative adversarial networks (3D-GAN) [6, 5, 16, 32, 42] are proposed for unsupervised generation of 3D scenes. By leveraging the state-of-the-art 2D CNN generator [21] and neural volume rendering, the work of [5] can generate high-quality multi-view-consistent images. The latent space of 3D-GAN constitutes a rich 3D-aware generative prior, which motivates us to explore latent space inversion and navigation for 3D facial avatar reconstruction from monocular videos. However, 3D-GAN is usually trained on the dataset with a large number of identities, such as FFHQ [20], resulting in a generic generative prior. It is inefficient for personalized facial reconstruction and reenactment, which requires faithfully maintenance of personalized characteristics.
In this work, we propose to learn a personalized 3D-aware generative prior to reconstruct multi-view-consistent facial images of that individual faithfully. Considering that facial variations share common characteristics, we learn a local and low-dimensional personalized subspace in the latent space of 3D-GAN. Specifically, we assign learnable basis vectors for the individual, where is the dimension of the 3D-GAN latent space. Each frame is sent to an encoder to regress a weight coefficient , which is used to form a linear combination of the basis. The resulting latent code is sent to a 3D-aware generator for multi-view-consistent rendering. We show that both the personalized basis and encoder can be well modeled given a small set of personalized facial images. After training, one can directly project the testing frames with different facial expressions onto the learned personalized latent space to obtain a high-quality 3D consistent reconstruction. It is worth noting that the input modality is not limited to RGB frames. We demonstrate with a simple modification. The encoder can be trained with different signals, such as 3DMM expression coefficients or audio features, enabling 3DMM or audio-driven face reenactment. To verify its effectiveness, we conduct experiments with different input modalities, including monocular RGB videos, 3DMM coefficients, and audios. The comparison to state-of-the-art methods demonstrates our superior 3D consistent reconstruction and faithfully face reenactment performance.
Our main contributions are four-fold: 1) we propose to utilize 3D-aware generative prior for facial avatar reconstruction; 2) we propose an efficient method to learn a local and low-dimensional subspace to maintain personalized characteristics faithfully; 3) we develop 3DMM and audio-driven face reenactment by latent space navigation; 4) we conduct complementary experimental studies and obtain superior facial reconstruction and reenactment performance.
2 Related Work
We introduce recent works that are closely related to our method, including neural volume rendering, controllable face generation, and generative 3D-aware neural networks.
Neural scene representation and rendering.
Recently, Neural Radiance Field (NeRF) [29, 8, 11, 12, 25, 26, 28, 37, 48, 50, 35, 43, 27, 7] obtains impressive performance for novel view synthesis of complex scenes. Instead of explicitly modeling the geometry and appearance, NeRF represents a scene using a neural network (e.g., MLP) to encode color and density as a function of a continuous 5D coordinate (including spatial location and viewing direction). It then use classic volume rendering techniques for novel view synthesis. The volume rendering is differentiable and the neural representation can be optimized given a set of images of a scene.
The photo-realistic 3D consistent rendering ability of NeRF has sparked great interest in facial avatar reconstruction. However, the standard formulation in [29] is proposed for static scene representation. And it requires multi-view input images for faithfully reconstruction. To handle the non-rigid dynamics in facial images captured by a monocular camera, the work of [33] proposed to learn a continuous deformation field, which learns a per-frame latent code and maps each observation coordinate into a canonical template canonical coordinate space. Furthermore, HyperNeRF [34] proposed to learn a higher-dimensional deformation field to better model the topology variations. After learning, they can be used for novel view synthesis. However, they don’t support facial editing.
Controllable face generation.
Controllable face generation is a key building-block for many applications in computer graphics and computer vision. The explicit 3D Morphable Face Model (3DMM) [3, 4, 24] uses linear subspace to control pose, expression and appearance independently. It provides fine-grained control over poses and expressions. However, it only models the face region and lacks personalized attributes, including hairs, eyes and accessories such as glasses. It suffers from artifacts when used for photo-realistic rendering. Apart from the explicit 3D based models, there have been several works that directly animate images in 2D space for face reenactment [41, 38, 30, 47, 23, 49, 36, 44, 45, 52, 46, 18]. They are usually realized by learning a warping field from driven information (e.g., image or audio) or training an encoder-decoder-based image translation networks. These methods, however, have to learn 3D deformation from 2D input. They couldn’t provide free view synthesis and suffer from artifacts, especially for large driven poses or expressions.
Recently, some works are proposed for controllable NeRF-based facial avatar reconstruction. They are realized by optimizing a conditional deformation field and scene representation based on either 3DMM coefficients or audio signals. For example, the work of [11] first transforms the camera space point into canonical space by the estimated pose parameters and then regresses its color and density conditioned on the 3DMM expression coefficients. In RigNeRF [2], the deformation field is realized as a combination of a explicit 3DMM deformation field and a predicted residual. The deformed point, as well as the 3DMM expression and pose coefficients, are send to an MLP to predict the color and density. To enable semantic control over facial expression, the work of [13] learns a series of neural radiance fields as basis, and associate them with expression coefficients extracted by mesh-based face models. As for audio-driven facial avatar, AD-NeRF [17] augments the 5D input with an audio feature for neural scene representation. DFRF [40] proposed an audio-driven few-shot talking head synthesis method. It learns a dynamic NeRF conditions on both audio features and 2D appearance images. These methods, however, directly construct dynamic facial neural radiance field from a monocular video. Considering the non-rigidly facial dynamics and missing 3D information in monocular videos, it is challenging to obtain high-fidelity multi-view-consistent results. Instead of directly learning the dynamic radiance field, we propose to utilize the rich generative prior of 3D-GAN and learn facial avatar by latent space inversion and navigation.
3D-aware Generative Neural Networks.
Generative adversarial networks have achieved great success in image generation. While most existing works focus on 2D images [14, 19, 20, 21, 31], recently 3D-aware generation has attracted more and more attention [6, 5, 53, 16, 32, 42]. The representative method, EG3D [5] significant improves the quality of 3D-aware generation. EG3D inherits the high-fidelity 2D image generation ability of StyleGAN [21] and the multi-view-consistent geometry of neural volume rendering. It is shown that after trained on FFHQ [20], a real world large-scale face dataset, EG3D obtains state-of-the-art 3D face synthesis. And its latent space constitutes a generative prior for multi-view-consistent images and 3D geometry. Inspired by these, we propose to learn a personalized 3D generative prior to faithfully reconstruct the specific characteristic of a given individual.
3 Proposed Method
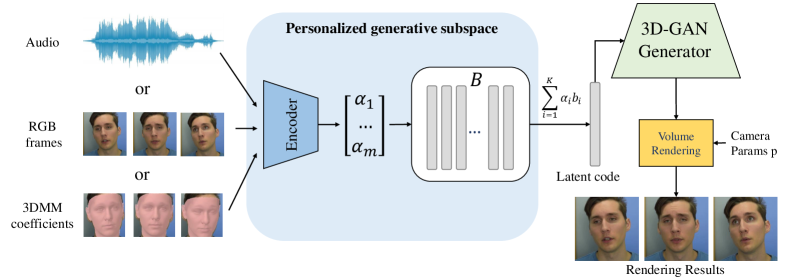
3.1 Preliminary of 3D-GAN
Our work builds on the multi-view-consistent image synthesis ability of 3D-GAN. The state-of-the-art method, EG3D [5], proposes an expressive hybrid explicit-implicit network based on a 2D CNN generator and neural rendering. For a 3D-aware generation, each random sampled latent code is first sent to a pose-conditioned StyleGAN2 generator to learn a tri-plane 3D representation. It then learns a neural radiance field based on it and generates a low-dimensional raw image by volume rendering. Finally, a super resolution module is adopted to generate high-resolution result. After being trained on FFHQ [20], EG3D can be used for unsupervised generation of multi-view-consistent facial images. Its latent space constitutes a generative prior for facial images with consistent 3D geometry.
A closer look at EG3D latent space. EG3D [5] is an unconditioned generation network and doesn’t provide any controls over identity or expression. For generation w.r.t. to a specific facial image, one can invert the input image back into the latent space. Given the inverted latent code, novel view synthesis can be realized by changing the camera pose during generation. However, recovering the 3D geometry from a single image is an ill-posed problem. Directly inverting facial images to the generic latent space of EG3D cannot faithfully reconstruct the specific characteristics of that individual, an example is given in Figure 3. In addition, it doesn’t support face reenactment.
3.2 Learning A Personalized Generative Prior
We aim to reconstruct 3D-aware animatable facial avatar based on a monocular video. To take advantage of the rich generative prior of 3D-GAN as well as maintain the personalized characteristics, we propose to learn a personalized generative prior. Our overall framework is given in Figure 2. Specifically, we define the personalized generative prior as a local, low-dimensional, and smooth subspace in the latent space. The low-dimensional property is expected as the facial images of a specific identity share common properties.
We consider space of 3D-GAN and assign a learnable personalized basis with vectors as in the space. The subspace that spanned by is defined by
(1) |
where represents the coefficient w.r.t. basis vectors. Rather than directly inversing each facial image into the high-dimensional space, we project into the low-dimensional subspace , by learning an encoder to regress the coefficient of . Finally, is sent to 3D-GAN generator for free view synthesis, as , where is the 3D-GAN generator and is the camera pose used for rendering.
3.3 Training Objective
Given a monocular face video of a individual with frames, each frame of which contains different expressions and poses. The encoder and the basis are jointly optimized for a faithfully reconstruction of . Let and is the camera pose extracted from , we calculate the loss and LPIPS loss [51] between and ,
(2) |
During training, we further constraint the basis vectors to be orthogonal to each other. The orthogonal constraint can largely boost the disentanglement of the basis. We provide an visualization of the basis in Figure 6. For the generator , we adopt the pretrain model [5] that learned on FFHQ. Similar to PTI [39], during training we slightly modify the generator to better maintain the personalized characteristics. We use a two-stage training strategy: 1) freezing the parameters of the generator and updating the encoder and the basis , and 2) turning on the gradient of the generator to adapt it to the personalized subspace.
Generalize to testing frames. The local and low-dimensional personalized subspace provides a good generalization to facial variations beyond the training frames. After training, the encoder can be directly applied to testing frames with different facial expressions to generate high-fidelity facial avatar reconstruction.
3.4 Face Reenactment with Various Signals
In the above section, we learn an encoder to project each facial image into the personalized latent space, to provide faithful 3D-aware reconstruction. Indeed, the input signal is not limited to facial images. Here, we provide two realizations with 3DMM coefficients and audio signals as input information, respectively. After training, they can be used for 3DMM or audio driven 3D-aware face reenactment.
3DMM-driven face reenactment: we extract 3DMM expression coefficient from each image, forming training pairs of . The coefficient is learned by . Audio-driven face reenactment: following [17], we use DeepSpeech [1] to extract a 29-dimensional feature for each 20ms audio clip. To eliminate the noisy signals from raw input, we concatenate the features of sixteen neighboring audio clips, resulting in for the audio feature of the current frame. We then project into the latent space by .
4 Experiments
Our method performs 3D facial reconstruction with a monocular video sequence, and the modeled face can be driven by various input signals. In this section, we first introduce the experimental settings. Then, we perform the reconstruction with RGB images, 3DMM coefficients, and audio signals as inputs, respectively. We also compared our proposed method with several baseline models both qualitatively and quantitatively. Finally, we performed several ablation studies to analyze the key elements of our approach.
4.1 Implementation Details
Data preprocessing. The training video needs to be processed before face modeling. For each frame of the video, we use an off-the-shelf pose estimator [10] to estimate its corresponding camera intrinsic and extrinsic matrices as the input to EG3D generator. The flattened camera extrinsic matrix and flattened camera intrinsic matrix are concatenated into a 25-dimensional vector as the camera input to EG3D generator. Then, we extract the appropriately-sized crops from each frame and resize each cropped image to the resolution of .
Training details. For each video, we train the network 200k iterations to obtain a personalized face model. The parameters of the generator are not optimized in the first 50k rounds. We train our model on a single Nvidia Telsa V100 GPU. We use the Adam optimizer [22] to train the network, and the learning rate is set to , and set to and , respectively. The batch size is and . The number of basis vectors in our method is set to .
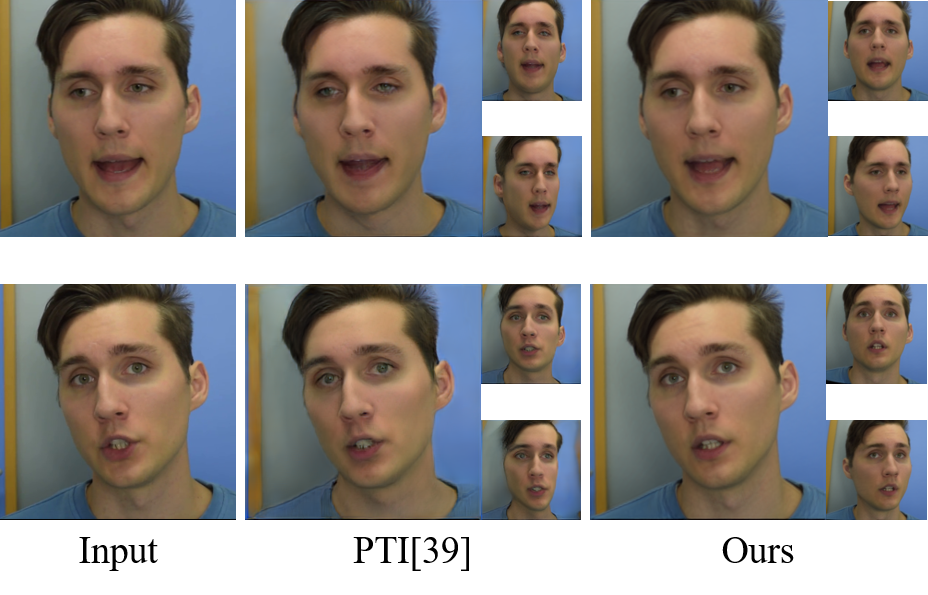
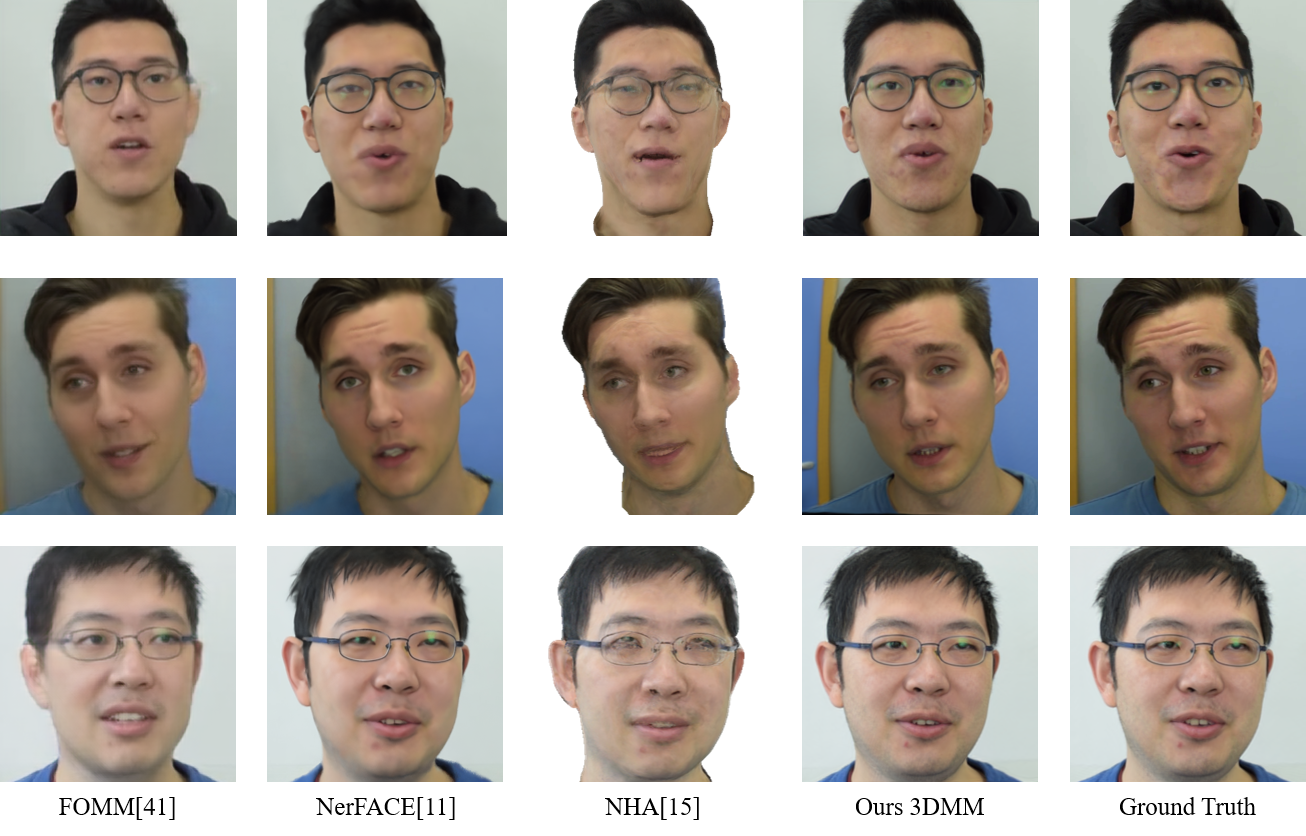
Methods | Metrics | ||
PSNR | SSIM | LPIPS | |
PTI [39] | 32.62 | 0.959 | 0.037 |
Ours RGB | 34.70 | 0.979 | 0.024 |
4.2 Results of RGB-based Face Reconstruction
We first conduct experiments with RGB frames as input. We adopt the three monocular videos used in NerFACE [11] for evaluation. For each video, we extract the first 2 minutes ( 6000 frames) for training and the left 20 seconds ( 1000 frames) for testing. After training, we directly send each testing frame into the learned encoder to obtain its latent code, which is then sent to the generator for novel view synthesis. To better verify the face reconstruction performance, we also present the performance of PTI [39], which is an optimization-based GAN inversion method. For each testing frame, PTI optimizes both the latent code and the EG3D generator for a faithfully reconstruction.
To measure their quality, we conduct a quantitative evaluation using several common metrics, including Peak Signal-to-Noise Ratio (PSNR), Structure Similarity Index (SSIM), and the Learned Perceptual Image Patch Similarity (LPIPS) [51]. As we don’t have novel view ground truth images, we calculate these metrics under the same views of the testing frames. The numerical results are given in Table 1. Compared to PTI, we obtain superior performance under all three metrics. In Figure 3, we show some rendered images with the camera views of testing frames and two randomly picked novel views. The complete novel view comparisons are given in the supplementary materials. Our method can better maintain the personalized characteristics, such as the mouth area. More importantly, for novel view synthesis, PTI shows significant artifacts for face shapes and cannot preserve the ID, while we obtain superior multi-view-consistent results. These demonstrate that the learned personalized generative prior enables faithful face reconstruction.
4.3 Results of 3DMM-driven Face Reenactment
We then evaluate the performance of 3DMM-driven face reenactment. We compare to the 3D-aware methods, Neural Head Avatar (NHA) [15] and NerFACE [11]. We also provide the results of FOMM [41], a 2D-based face animation method. Note that FOMM doesn’t support novel view synthesis. As in the previous section, we adopt the three monocular videos used in NerFACE. We extract the 3DMM expression coefficients from each frame for training and testing.
The average results in terms of PSNR, SSIM and LPIPS are listed in Table 2. The 3D-aware methods NerFACE, NHA and ours obtain better performance than FOMM. With the help of the high-quality prior of the generative model, our method significantly boosts the performance of 3DMM-driven face reenactment.
We also show a qualitative comparison in Figure 4, where all results are rendered under the same view of the ground truth image. It can be seen that the results of FOMM have obvious artifacts, and the identity of the animated face is altered a lot from the ground truth. The results of NerFACE are too smooth, and the details of the facial textures are not well reconstructed. NHA cannot faithfully reconstruct facial characteristics, including eyeglasses and mouth areas. In comparison, our method can better maintain the facial characteristics and generate faithful face reenactment. In Figure High-fidelity Facial Avatar Reconstruction from Monocular Video with Generative Priors, we present some novel view rendered images of NerFACE and ours, a complete comparison is given in the supplementary materials. The results of NerFACE are blurred and distorted. It fails to generate high-quality renderings under novel views, while our method obtains faithfully multi-view-consistent images.
Methods | Metrics | ||
PSNR | SSIM | LPIPS | |
FOMM [41] | 27.75 | 0.919 | 0.059 |
NerFACE [11] | 29.76 | 0.931 | 0.053 |
NHA [15] | 31.52 | 0.954 | 0.039 |
Ours 3DMM | 34.38 | 0.972 | 0.027 |
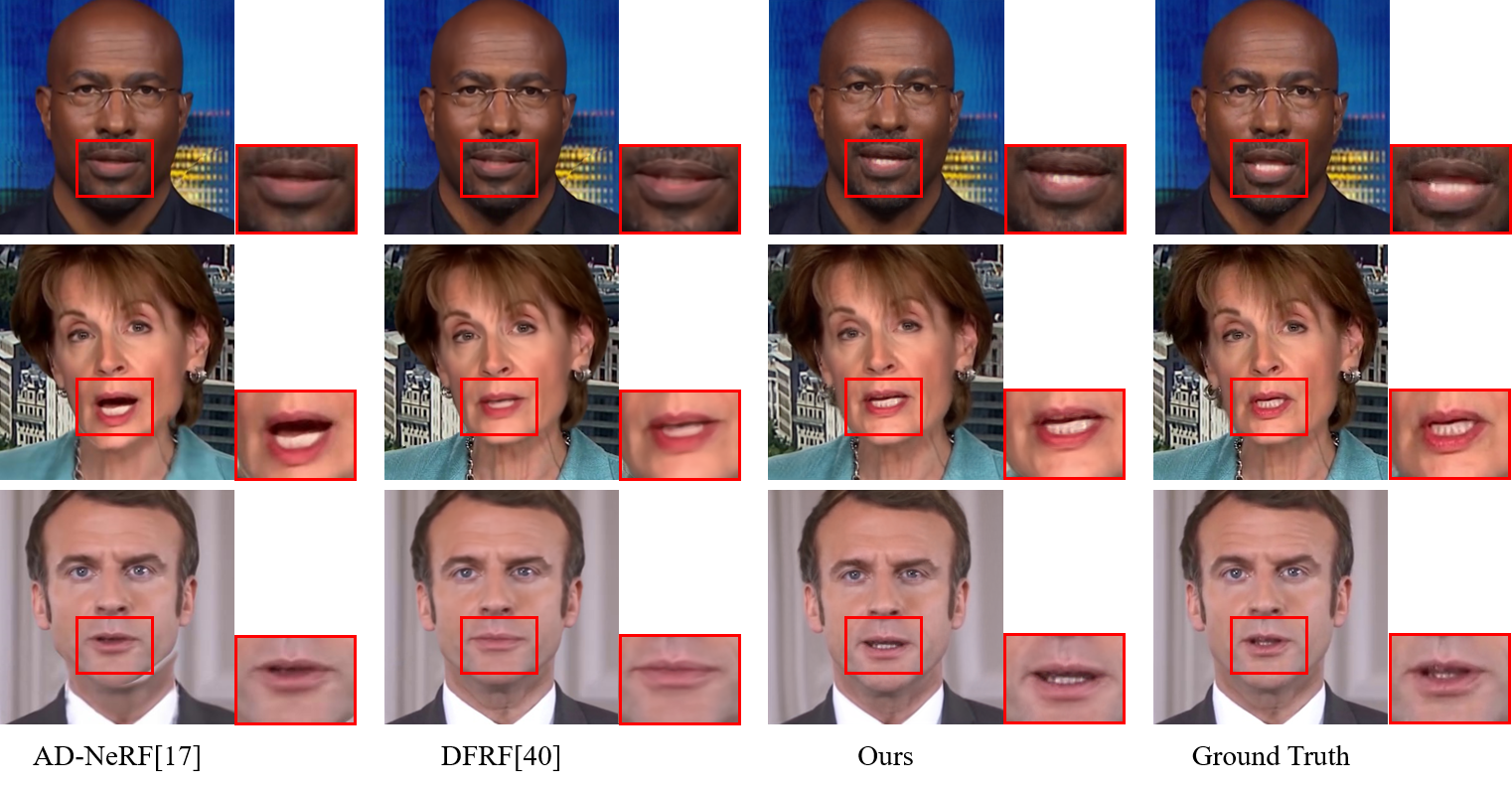
4.4 Results of Audio-driven Face Reenactment
Following the practice of [17], we perform audio-driven experiments on three public videos collected from YouTube. The position of the camera is fixed and the resolution of the videos is . Each video is divided into two segments of the training set and testing set, with no overlap between them. We extract their audio features for training and testing.
Methods | Metrics | |||
PSNR | SSIM | LPIPS | SyncNet | |
Ground Truth | - | - | - | 7.653 |
AD-NeRF [17] | 29.69 | 0.934 | 0.057 | 1.238 |
DFRF [40] | 30.23 | 0.939 | 0.042 | 4.142 |
Ours Audio | 32.57 | 0.957 | 0.035 | 4.866 |
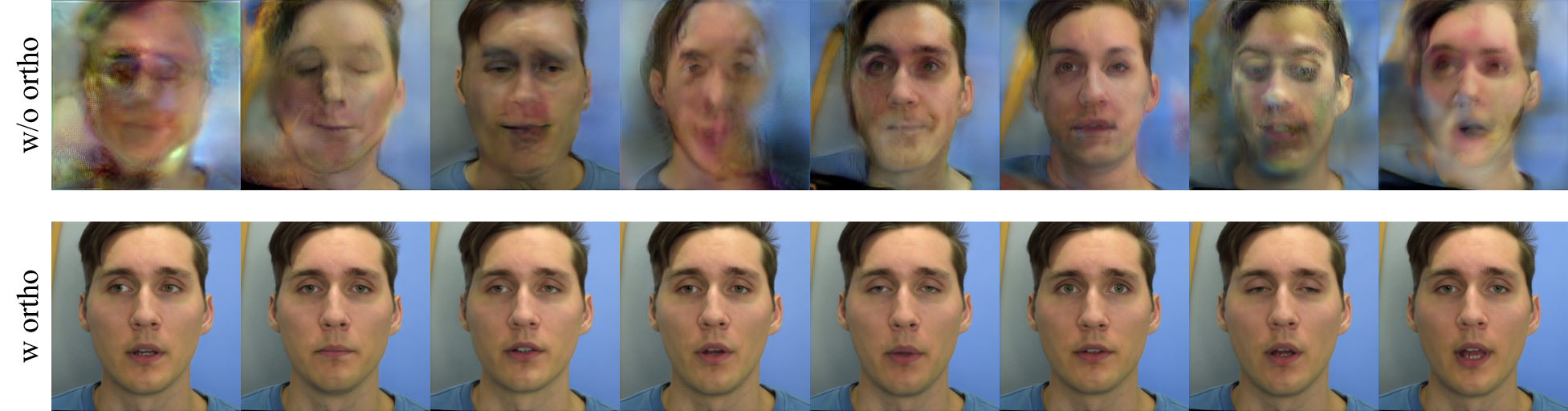
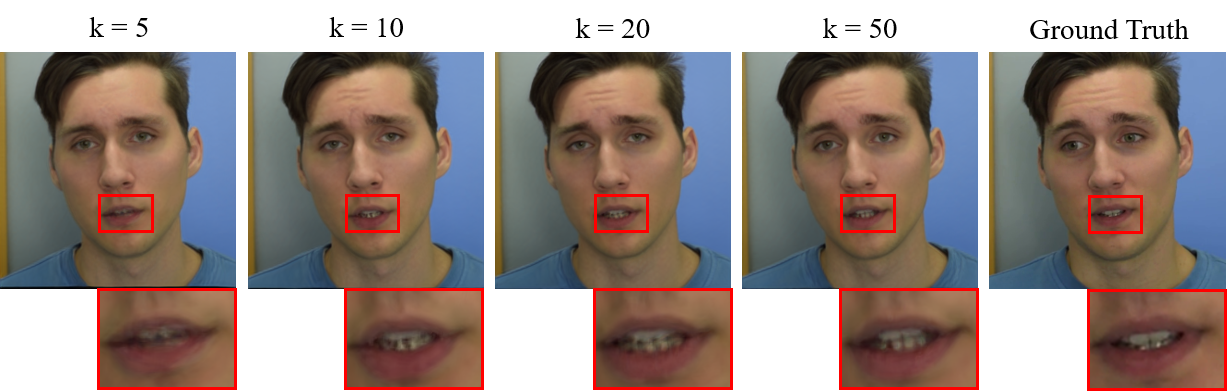
We compare our method with two audio-driven NeRF methods: AD-NeRF [17] and DFRF [40]. DFRF is a few-shot method. To make a fair comparison to it, we use a short 20 seconds video clip for training for all methods. In addition to the image quality metrics of PSNR, SSIM and LPIPS, SyncNet [9] is further used to measure audio-visual synchronization. The average metrics of three videos are given in Table 3. In the audio-driven scenario, our method also outperforms the previous methods with a significant margin for all four evaluation metrics. Besides, we provide some rendered images in Figure 5. We highlight the mouth areas that are most significant to audio-driven face reenactment. Compared to AD-NeRF and DFRF, we obtain much better mouth shapes and teeth. We also provide some novel view results of DFRF and ours in Figure High-fidelity Facial Avatar Reconstruction from Monocular Video with Generative Priors. Under novel views, the distortion of DFRF is even worse.
4.5 Ablation Studies
The key to our approach is to learn a basis to represent the personalized generative prior. We conduct ablation studies based on RGB-based face reconstruction to analyze the properties of the basis vectors.
Visualization of the basis vectors. We require the set of basis vectors to be orthogonal to each other during training. In Figure 6 we make a visualization of the learned basis. We also show the visualization results for a basis learned without the orthogonal constraints. It can be seen that the basis vectors are coupled together when there is no orthogonal constraint. With the orthogonal constraint, the basis vectors are disentangled and show better semantic meanings. The quantitative comparison results are shown in Table 4, which demonstrates that the reconstruction quality is much better with the orthogonal constraint.
Number of the basis vectors. We further explore the effect of the number of basis vectors. We vary the number of basis vectors by 5, 10, 20 and 50. The quantitative comparisons in terms of PSNR, SSIM and LPIPS are given in Table 4. And Figure 7 shows some rendered images under the different value of . We highlight the mouth areas for better visualization. As the number of basis vectors increases, the model is more capable of representing facial details and obtains better rendered quality.
Schemes | Metrics | ||
PSNR | SSIM | LPIPS | |
k = 50 (w/o ortho) | 33.36 | 0.962 | 0.037 |
k = 5 (w ortho) | 28.64 | 0.927 | 0.055 |
k = 10 (w ortho) | 30.83 | 0.946 | 0.042 |
k = 20 (w ortho) | 33.98 | 0.965 | 0.033 |
k = 50 (w ortho) | 34.70 | 0.979 | 0.024 |
Supplementary materials.
Due to space limitation, we only provide some rendered images and novel views in the main paper. We highly recommend checking the supplementary materials for more novel view synthesis results.
5 Conclusions
In this work, we propose to utilize 3D-aware generative prior for facial avatar reconstruction and reenactment from monocular videos. We propose an efficient method to learn a local and low-dimensional subspace in the latent space of 3D-GAN, for a better maintenance of personalized characteristics. The learned personalized generative prior provides a good constraint for 3D-aware generation, which is helpful for modeling the complex facial dynamics and missing 3D information in monocular videos. We conduct extensive experiments, including RGB-based face reconstruction and 3DMM and audio-driven face reenactment. Compared to existing works, we obtain superior performance both quantitatively and qualitatively.
Discussions. In the current formulation, we aim to optimize a personalized subspace that can be used for faithful reconstruction and self-reenactment driven by different signals. The learned personalized basis has shown some good properties of disentanglement and semantic meanings. We will continue our study on the properties of 3D-aware personalized generative prior and investigate more strategies to control the basis vectors. Besides, it is also very interesting and helpful to explore cross-identity face reenactment based on personalized 3D-aware generative prior.
References
- [1] Dario Amodei, Sundaram Ananthanarayanan, Rishita Anubhai, Jingliang Bai, Eric Battenberg, Carl Case, Jared Casper, Bryan Catanzaro, Qiang Cheng, Guoliang Chen, et al. Deep speech 2: End-to-end speech recognition in english and mandarin. In International conference on machine learning, pages 173–182. PMLR, 2016.
- [2] ShahRukh Athar, Zexiang Xu, Kalyan Sunkavalli, Eli Shechtman, and Zhixin Shu. Rignerf: Fully controllable neural 3d portraits. In Proceedings of the IEEE/CVF Conference on Computer Vision and Pattern Recognition, pages 20364–20373, 2022.
- [3] Volker Blanz and Thomas Vetter. A morphable model for the synthesis of 3d faces. In Proceedings of the 26th annual conference on Computer graphics and interactive techniques, pages 187–194, 1999.
- [4] Chen Cao, Yanlin Weng, Shun Zhou, Yiying Tong, and Kun Zhou. Facewarehouse: A 3d facial expression database for visual computing. IEEE Transactions on Visualization and Computer Graphics, 20(3):413–425, 2013.
- [5] Eric R Chan, Connor Z Lin, Matthew A Chan, Koki Nagano, Boxiao Pan, Shalini De Mello, Orazio Gallo, Leonidas J Guibas, Jonathan Tremblay, Sameh Khamis, et al. Efficient geometry-aware 3d generative adversarial networks. In Proceedings of the IEEE/CVF Conference on Computer Vision and Pattern Recognition, pages 16123–16133, 2022.
- [6] Eric R Chan, Marco Monteiro, Petr Kellnhofer, Jiajun Wu, and Gordon Wetzstein. pi-gan: Periodic implicit generative adversarial networks for 3d-aware image synthesis. In Proceedings of the IEEE/CVF conference on computer vision and pattern recognition, pages 5799–5809, 2021.
- [7] Xingyu Chen, Qi Zhang, Xiaoyu Li, Yue Chen, Ying Feng, Xuan Wang, and Jue Wang. Hallucinated neural radiance fields in the wild. In Proceedings of the IEEE/CVF Conference on Computer Vision and Pattern Recognition, pages 12943–12952, 2022.
- [8] Julian Chibane, Aayush Bansal, Verica Lazova, and Gerard Pons-Moll. Stereo radiance fields (srf): Learning view synthesis for sparse views of novel scenes. In Proceedings of the IEEE/CVF Conference on Computer Vision and Pattern Recognition, pages 7911–7920, 2021.
- [9] Joon Son Chung and Andrew Zisserman. Out of time: automated lip sync in the wild. In Asian conference on computer vision, pages 251–263. Springer, 2016.
- [10] Yu Deng, Jiaolong Yang, Sicheng Xu, Dong Chen, Yunde Jia, and Xin Tong. Accurate 3d face reconstruction with weakly-supervised learning: From single image to image set. In Proceedings of the IEEE/CVF Conference on Computer Vision and Pattern Recognition Workshops, pages 0–0, 2019.
- [11] Guy Gafni, Justus Thies, Michael Zollhofer, and Matthias Nießner. Dynamic neural radiance fields for monocular 4d facial avatar reconstruction. In Proceedings of the IEEE/CVF Conference on Computer Vision and Pattern Recognition, pages 8649–8658, 2021.
- [12] Chen Gao, Yichang Shih, Wei-Sheng Lai, Chia-Kai Liang, and Jia-Bin Huang. Portrait neural radiance fields from a single image. arXiv preprint arXiv:2012.05903, 2020.
- [13] Xuan Gao, Chenglai Zhong, Jun Xiang, Yang Hong, Yudong Guo, and Juyong Zhang. Reconstructing personalized semantic facial nerf models from monocular video. arXiv preprint arXiv:2210.06108, 2022.
- [14] Ian Goodfellow, Jean Pouget-Abadie, Mehdi Mirza, Bing Xu, David Warde-Farley, Sherjil Ozair, Aaron Courville, and Yoshua Bengio. Generative adversarial networks. Communications of the ACM, 63(11):139–144, 2020.
- [15] Philip-William Grassal, Malte Prinzler, Titus Leistner, Carsten Rother, Matthias Nießner, and Justus Thies. Neural head avatars from monocular rgb videos. In Proceedings of the IEEE/CVF Conference on Computer Vision and Pattern Recognition, pages 18653–18664, 2022.
- [16] Jiatao Gu, Lingjie Liu, Peng Wang, and Christian Theobalt. Stylenerf: A style-based 3d-aware generator for high-resolution image synthesis. arXiv preprint arXiv:2110.08985, 2021.
- [17] Yudong Guo, Keyu Chen, Sen Liang, Yong-Jin Liu, Hujun Bao, and Juyong Zhang. Ad-nerf: Audio driven neural radiance fields for talking head synthesis. In Proceedings of the IEEE/CVF International Conference on Computer Vision, pages 5784–5794, 2021.
- [18] Xinya Ji, Hang Zhou, Kaisiyuan Wang, Wayne Wu, Chen Change Loy, Xun Cao, and Feng Xu. Audio-driven emotional video portraits. In Proceedings of the IEEE/CVF conference on computer vision and pattern recognition, pages 14080–14089, 2021.
- [19] Tero Karras, Timo Aila, Samuli Laine, and Jaakko Lehtinen. Progressive growing of gans for improved quality, stability, and variation. arXiv preprint arXiv:1710.10196, 2017.
- [20] Tero Karras, Samuli Laine, and Timo Aila. A style-based generator architecture for generative adversarial networks. In Proceedings of the IEEE/CVF conference on computer vision and pattern recognition, pages 4401–4410, 2019.
- [21] Tero Karras, Samuli Laine, Miika Aittala, Janne Hellsten, Jaakko Lehtinen, and Timo Aila. Analyzing and improving the image quality of stylegan. In Proceedings of the IEEE/CVF conference on computer vision and pattern recognition, pages 8110–8119, 2020.
- [22] Diederik P Kingma and Jimmy Ba. Adam: A method for stochastic optimization. arXiv preprint arXiv:1412.6980, 2014.
- [23] Marek Kowalski, Stephan J Garbin, Virginia Estellers, Tadas Baltrušaitis, Matthew Johnson, and Jamie Shotton. Config: Controllable neural face image generation. In European Conference on Computer Vision, pages 299–315. Springer, 2020.
- [24] Tianye Li, Timo Bolkart, Michael J Black, Hao Li, and Javier Romero. Learning a model of facial shape and expression from 4d scans. ACM Trans. Graph., 36(6):194–1, 2017.
- [25] Zhengqi Li, Simon Niklaus, Noah Snavely, and Oliver Wang. Neural scene flow fields for space-time view synthesis of dynamic scenes. In Proceedings of the IEEE/CVF Conference on Computer Vision and Pattern Recognition, pages 6498–6508, 2021.
- [26] Stephen Lombardi, Tomas Simon, Gabriel Schwartz, Michael Zollhoefer, Yaser Sheikh, and Jason Saragih. Mixture of volumetric primitives for efficient neural rendering. ACM Transactions on Graphics (TOG), 40(4):1–13, 2021.
- [27] Li Ma, Xiaoyu Li, Jing Liao, Xuan Wang, Qi Zhang, Jue Wang, and Pedro Sander. Neural parameterization for dynamic human head editing. arXiv preprint arXiv:2207.00210, 2022.
- [28] Ricardo Martin-Brualla, Noha Radwan, Mehdi SM Sajjadi, Jonathan T Barron, Alexey Dosovitskiy, and Daniel Duckworth. Nerf in the wild: Neural radiance fields for unconstrained photo collections. In Proceedings of the IEEE/CVF Conference on Computer Vision and Pattern Recognition, pages 7210–7219, 2021.
- [29] Ben Mildenhall, Pratul P Srinivasan, Matthew Tancik, Jonathan T Barron, Ravi Ramamoorthi, and Ren Ng. Nerf: Representing scenes as neural radiance fields for view synthesis. Communications of the ACM, 65(1):99–106, 2021.
- [30] Yuval Nirkin, Yosi Keller, and Tal Hassner. Fsgan: Subject agnostic face swapping and reenactment. In Proceedings of the IEEE/CVF international conference on computer vision, pages 7184–7193, 2019.
- [31] Yotam Nitzan, Kfir Aberman, Qiurui He, Orly Liba, Michal Yarom, Yossi Gandelsman, Inbar Mosseri, Yael Pritch, and Daniel Cohen-Or. Mystyle: A personalized generative prior. arXiv preprint arXiv:2203.17272, 2022.
- [32] Roy Or-El, Xuan Luo, Mengyi Shan, Eli Shechtman, Jeong Joon Park, and Ira Kemelmacher-Shlizerman. Stylesdf: High-resolution 3d-consistent image and geometry generation. In Proceedings of the IEEE/CVF Conference on Computer Vision and Pattern Recognition, pages 13503–13513, 2022.
- [33] Keunhong Park, Utkarsh Sinha, Jonathan T Barron, Sofien Bouaziz, Dan B Goldman, Steven M Seitz, and Ricardo Martin-Brualla. Nerfies: Deformable neural radiance fields. In Proceedings of the IEEE/CVF International Conference on Computer Vision, pages 5865–5874, 2021.
- [34] Keunhong Park, Utkarsh Sinha, Peter Hedman, Jonathan T Barron, Sofien Bouaziz, Dan B Goldman, Ricardo Martin-Brualla, and Steven M Seitz. Hypernerf: A higher-dimensional representation for topologically varying neural radiance fields. arXiv preprint arXiv:2106.13228, 2021.
- [35] Sida Peng, Yuanqing Zhang, Yinghao Xu, Qianqian Wang, Qing Shuai, Hujun Bao, and Xiaowei Zhou. Neural body: Implicit neural representations with structured latent codes for novel view synthesis of dynamic humans. In Proceedings of the IEEE/CVF Conference on Computer Vision and Pattern Recognition, pages 9054–9063, 2021.
- [36] KR Prajwal, Rudrabha Mukhopadhyay, Vinay P Namboodiri, and CV Jawahar. A lip sync expert is all you need for speech to lip generation in the wild. In Proceedings of the 28th ACM International Conference on Multimedia, pages 484–492, 2020.
- [37] Albert Pumarola, Enric Corona, Gerard Pons-Moll, and Francesc Moreno-Noguer. D-nerf: Neural radiance fields for dynamic scenes. In Proceedings of the IEEE/CVF Conference on Computer Vision and Pattern Recognition, pages 10318–10327, 2021.
- [38] Yurui Ren, Ge Li, Yuanqi Chen, Thomas H Li, and Shan Liu. Pirenderer: Controllable portrait image generation via semantic neural rendering. In Proceedings of the IEEE/CVF International Conference on Computer Vision, pages 13759–13768, 2021.
- [39] Daniel Roich, Ron Mokady, Amit H Bermano, and Daniel Cohen-Or. Pivotal tuning for latent-based editing of real images. ACM Transactions on Graphics (TOG), 42(1):1–13, 2022.
- [40] Shuai Shen, Wanhua Li, Zheng Zhu, Yueqi Duan, Jie Zhou, and Jiwen Lu. Learning dynamic facial radiance fields for few-shot talking head synthesis. In European Conference on Computer Vision, pages 666–682. Springer, 2022.
- [41] Aliaksandr Siarohin, Stéphane Lathuilière, Sergey Tulyakov, Elisa Ricci, and Nicu Sebe. First order motion model for image animation. Advances in Neural Information Processing Systems, 32, 2019.
- [42] Jingxiang Sun, Xuan Wang, Yichun Shi, Lizhen Wang, Jue Wang, and Yebin Liu. Ide-3d: Interactive disentangled editing for high-resolution 3d-aware portrait synthesis. arXiv preprint arXiv:2205.15517, 2022.
- [43] Jingxiang Sun, Xuan Wang, Yong Zhang, Xiaoyu Li, Qi Zhang, Yebin Liu, and Jue Wang. Fenerf: Face editing in neural radiance fields. In Proceedings of the IEEE/CVF Conference on Computer Vision and Pattern Recognition, pages 7672–7682, 2022.
- [44] Supasorn Suwajanakorn, Steven M Seitz, and Ira Kemelmacher-Shlizerman. Synthesizing obama: learning lip sync from audio. ACM Transactions on Graphics (ToG), 36(4):1–13, 2017.
- [45] Sarah Taylor, Taehwan Kim, Yisong Yue, Moshe Mahler, James Krahe, Anastasio Garcia Rodriguez, Jessica Hodgins, and Iain Matthews. A deep learning approach for generalized speech animation. ACM Transactions on Graphics (TOG), 36(4):1–11, 2017.
- [46] Ting-Chun Wang, Arun Mallya, and Ming-Yu Liu. One-shot free-view neural talking-head synthesis for video conferencing. In Proceedings of the IEEE/CVF conference on computer vision and pattern recognition, pages 10039–10049, 2021.
- [47] Yaohui Wang, Di Yang, Francois Bremond, and Antitza Dantcheva. Latent image animator: Learning to animate images via latent space navigation. arXiv preprint arXiv:2203.09043, 2022.
- [48] Wenqi Xian, Jia-Bin Huang, Johannes Kopf, and Changil Kim. Space-time neural irradiance fields for free-viewpoint video. In Proceedings of the IEEE/CVF Conference on Computer Vision and Pattern Recognition, pages 9421–9431, 2021.
- [49] Fei Yin, Yong Zhang, Xiaodong Cun, Mingdeng Cao, Yanbo Fan, Xuan Wang, Qingyan Bai, Baoyuan Wu, Jue Wang, and Yujiu Yang. Styleheat: One-shot high-resolution editable talking face generation via pretrained stylegan. arXiv preprint arXiv:2203.04036, 2022.
- [50] Kai Zhang, Gernot Riegler, Noah Snavely, and Vladlen Koltun. Nerf++: Analyzing and improving neural radiance fields. arXiv preprint arXiv:2010.07492, 2020.
- [51] Richard Zhang, Phillip Isola, Alexei A Efros, Eli Shechtman, and Oliver Wang. The unreasonable effectiveness of deep features as a perceptual metric. In Proceedings of the IEEE conference on computer vision and pattern recognition, pages 586–595, 2018.
- [52] Zhimeng Zhang, Lincheng Li, Yu Ding, and Changjie Fan. Flow-guided one-shot talking face generation with a high-resolution audio-visual dataset. In Proceedings of the IEEE/CVF Conference on Computer Vision and Pattern Recognition, pages 3661–3670, 2021.
- [53] Peng Zhou, Lingxi Xie, Bingbing Ni, and Qi Tian. Cips-3d: A 3d-aware generator of gans based on conditionally-independent pixel synthesis. arXiv preprint arXiv:2110.09788, 2021.