HawkI: Homography & Mutual Information Guidance for 3D-free Single Image to Aerial View
Abstract
We present HawkI, for synthesizing aerial-view images from text and an exemplar image, without any additional multi-view or 3D information for finetuning or at inference. HawkI uses techniques from classical computer vision and information theory. It seamlessly blends the visual features from the input image within a pretrained text-to-2D-image stable diffusion model with a test-time optimization process for a careful bias-variance trade-off, which uses an Inverse Perspective Mapping (IPM) homography transformation to provide subtle cues for aerial-view synthesis. At inference, HawkI employs a unique mutual information guidance formulation to steer the generated image towards faithfully replicating the semantic details of the input-image, while maintaining a realistic aerial perspective. Mutual information guidance maximizes the semantic consistency between the generated image and the input image, without enforcing pixel-level correspondence between vastly different viewpoints. Through extensive qualitative and quantitative comparisons against text + exemplar-image based methods and 3D/ multi-view based novel-view synthesis methods on proposed synthetic and real datasets, we demonstrate that our method achieves a significantly better bias-variance trade-off towards generating high fidelity aerial-view images. Code and data is available at https://github.com/divyakraman/HawkI2024.
![[Uncaptioned image]](https://cdn.awesomepapers.org/papers/525c2169-6176-4606-8628-703d70660ffc/coverPic.jpg)
1 Introduction
Widely available text-to-image models such as Stable Diffusion [64], trained on large-scale text and 2D image data, contain rich knowledge of the 3D world. They are capable of generating scenes from various viewpoints, including aerial views. However, due to limitations of the expressiveness of the text, we may not be able to completely describe the precise scene that we wish to generate. Moreover, the generative capabilities of the pretrained model is constrained by the aerial-view images in the dataset that it was trained on, which is typically limited. Consequentially, along with text, it is beneficial to use an easily available representative front-view image describing the aerial view of the scene we wish to generate. The task of generating aerial-view images from a given input image and its text description finds applications in the generation of realistic diverse aerial view synthetic data for improved aerial view perception tasks [36, 41, 37, 12, 4], and weak supervision for cross-view synthesis applications [49] such as localization and mapping [31], autonomous driving [10], augmented and virtual reality [21], 3D reconstruction [82], medical imaging [80], drone-enabled surveillance [2].
Aerial-view images corresponding to text and an input image can be sampled using text-to-3D and novel view synthesis (NVS) [48, 56]. These methods sample different camera viewpoints by explicitly specifying the camera angle. However, they often need to trained on enormous, large-scale datasets with 3D details and scenes from multiple views. Is it possible for text-to-image(2D) diffusion models to generate aerial-view images without any multi-view or 3D information?
Another closely related task is image editing [34] and personalization [65], where the goal is to use an input image and a target text to generate an image consistent with both inputs. These methods are generally successful in performing a wide range of non-rigid transformations including text-controlled view synthesis. However, the large translation required for aerial view synthesis makes them sub-optimal [56]. This is due to bias-variance trade-off issues, even more amplified when only a single input image is provided. Aerial Diffusion [38] attempted to alleviate this bias-variance trade-off, but at the cost of per-sample hyperparameter tuning, residual diagonal artifacts in many of the generated images arising from direct finetuning on sub-optimal homography projections and severe performance drops on complex scenes with multiple objects.
Main contributions.
We propose HawkI for aerial view synthesis, guided by text and a single input image. Our method leverages text-to-image diffusion models for prior knowledge and does not require any 3D or multi-view data. Since explicitly specifying camera details in text descriptions isn’t always possible, similar to prior work on text-based viewpoint generation [65, 38], we consider any generated image with a significantly higher viewpoint and altitude compared to the original image to be an aerial view. HawkI fuses techniques from classical computer vision and information theory within a stable diffusion backbone model to guide the synthesis of the aerial-view image. The key novel components of our algorithm include:
-
1.
Test-time optimization: This step enables the model to acquire the characteristics of the input image, while maintaining sufficient variability in the embedding space for aerial-view synthesis. We condition the embedding space by sequentially optimizing the CLIP text-image embedding and the LoRA layers corresponding to the diffusion UNet on the input image and its Inverse Perspective Mapping (IPM) homography transformation in close vicinity. In addition to creating variance, IPM provides implicit guidance towards the direction of transformation for aerial-view synthesis.
-
2.
Mutual Information Guided Inference: This step generates a semantically consistent aerial-view image while accounting for viewpoint differences. Unlike conventional approaches [3, 22] that rely on restrictive pixel-level constraints (often ineffective for different viewpoints), we propose a mutual information vastly guidance formulation. Mutual information guidance, rooted in information theory, ensures consistency between the contents of the generated image and the input image by maximizing the information contained between the probability distributions of the input image and the generated aerial image.
Our method performs inference-time optimization on the given text-image inputs and does not require a dataset to train on, hence, it is easily applicable to any in-the-wild image. To test our method, we collect a diverse set of synthetic images (from Stable Diffusion XL) and real images (from Unsplash), spanning across natural scenes, indoor scenes, human actions and animations. Qualitative and quantitative comparisons with prior work, on metrics such as CLIP [58] (measuring viewpoint and text consistency) and SSCD [54], DINOv2 [52] (measuring consistency w.r.t. input image), demonstrate that HawkI generates aerial-view images with a significantly better viewpoint-fidelity (or bias-variance) trade-off. We also present extensive ablation experiments and comparisons with 3D-based novel-view synthesis methods highlighting the benefits of our 3D-free classical guidance approaches. Our method can also be extended to generate more views that can be text-controlled (such as ‘side view’, bottom view’, ‘back view’), as evidenced by our results.
2 Related work
3D and novel view synthesis. Novel view synthesis [83, 79, 53] from a single image is an active area of research in generative AI. Many methods [75, 33, 25, 92] use NeRF based techniques. Nerdi [16] use language guidance with NeRFs for view synthesis. Many recent methods use diffusion [47, 72, 48, 57, 73, 70, 6, 67] to sample different views. 3D generation methods [56, 42, 86, 59, 11] use text to guide the reconstruction. All of these methods use large amounts of multi-view and 3D data for supervised training. Methods like Zero-1-to-3 [48] and Zero-123++ [70] use a pretrained stable diffusion [64] model, along with large data for supervised training, to learn different camera viewpoints. 3D-free methods such as Free3D [91] still require multi-view and 3D information while training.
Warping, scene extrapolation and homography. Scenescape [23], DiffDreamer [7] and similar methods [83, 63, 9] estimate a depth map, reproject the pixels into the desired camera perspective and outpaint the scene. Again, these methods require 3D and multi-view information at training stage. Using a homography to estimate the scene from an aerial perspective is highly inaccurate, hence, attempting to create realistic aerial view images by simply filling in missing information based on the homography (outpainting) leads to poor outcomes. Homography maps have also been used in various deep learning based computer vision solutions [88, 18, 46, 26, 14].
Image editing/ personalization. Diffusion models have emerged as successful tools for single image editing and personalization. Methods such as DreamBooth [65], DreamBooth LoRA [30], HyperDreamBooth [66], Textual Inversion [24], Custom Diffusion [39] are able to generated personalized images of subjects. Image editing methods such as Imagic [34], Paint-by-Example [87], ControlNet [89], DiffEdit [13] are able to edit images to perform non-rigid transformations and also use exemplar signals for guidance. However, these methods can either generate aerial images with low fidelity w.r.t. the input image or generate high-fidelity images with viewpoints very close to the input image.
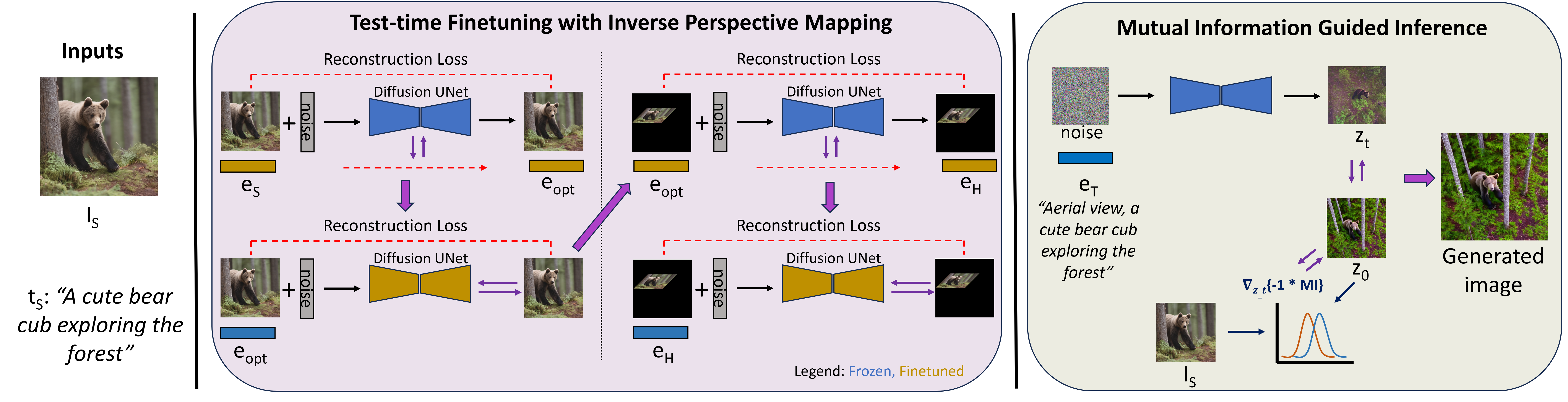
Cross-view synthesis. Prior work on cross-view synthesis [60, 76, 62, 78, 19, 49, 45, 71, 44, 61, 43, 84, 69, 1, 90] are data intensive - they use paired data and modalities such as semantic maps, depth, multi-views, etc within their architectures. Aerial Diffusion [38] uses text and an exemplar image for the task by alternating sampling between viewpoint and homography projects. However, the generated images have diagonal artifacts with poor quality results for complex scenes that typically contain more than one object and requires manual per-sample hyperparameter tuning.
Guidance techniques in diffusion. Guidance methods [29, 3, 17, 51] have been used to control and guide diffusion denoising towards semantic maps, image classes, etc. These guidance techniques cannot enforce view-invariant image-image similarity, critical for aligning the contents in two images with vastly different viewpoints.
3 Method
We present HawkI to generate aerial view images using a single input image and its text description (e.g. ‘a cosy living room’, can be obtained using the BLIP-2 model [40]). We do not use any training data or 3D/multi-view data. We leverage the pretrained text-to-2D image stable diffusion [64] model to serve as a strong prior, and utilize classical computer vision and information theory principles to achieve the desired goal in a holistic manner. We present an overview of our method in Figure 2.
-
•
Test-time optimization: We perform multi-step test-time optimization to incorporate the input image within the pretrained model, at an appropriate bias-variance trade-off. Specifically, we optimize the CLIP text-image embedding and the LoRA layers in the diffusion UNet sequentially on the input image and its inverse perspective mapping, in close vicinity. This additionally conditions the embedding space viewpoint transformations, along with acquiring the characteristics of the input image.
-
•
Inference: To generate the aerial-view image, we use the target text description , that takes the form ‘aerial view, ’ + (e.g. ‘aerial view, a cosy living room’). To ensure that the generated aerial image is semantically close to the input image, we use mutual information guidance.
Next, we describe our method in detail.
3.1 Test-time optimization
The text-to-2D image stable diffusion model has knowledge of the 3D world as a consequence of the large amount of diverse data it has been trained on. It understands [68] different viewpoints, different styles, backgrounds, etc. Image editing and personalization methods such as DreamBooth [65], DreamBooth LoRA [30], Imagic [34], SVDiff [27] exploit this property to perform transformations such as making a standing dog sit and generating its image in front of the Eiffel tower. At a high level, the standard procedure adopted by these methods to generate edited or personalized images is to finetune the model on the input image, followed by inferencing. These methods are however not very successful in text-guided aerial view synthesis, which demands a large transformation. Specifically, directly finetuning the diffusion model on to reconstruct results in severe overfitting, where is the CLIP text embedding for . This makes it difficult for the model to generate large variations to the scene required for aerial view synthesis.
We propose a four-step finetuning approach to enable the model to learn the characteristics of , while ensuring sufficient variance for aerial view generation.
3.1.1 Optimization using :
In the first step, we start from and compute the optimized CLIP text-image embedding to reconstruct using a frozen diffusion model UNet using the denoising diffusion loss function [28].
(1) |
where is the diffusion timestep and is the latents at time . This formulation allows us to find the text embedding that characterizes better than the generic text embedding .
Next, to enable accurately reconstruct , we optimize the diffusion UNet using the denoising diffusion objective function. Note that we insert LoRA layers within the attention modules in the diffusion UNet and finetune only the LoRA layers with parameters , the rest of the UNet parameters are frozen,
(2) |
While optimizing instead of to reconstruct ensures lesser bias (or more variance), the embedding space is still not sufficiently conditioned to generate an aerial view of the image.
3.1.2 Optimization using inverse perspective mapping.
Inverse perspective mapping (IPM) [74] is a homography transformation from classical computer vision to generate the aerial-view of an image from its ground-view. Despite not being accurate, it can provide pseudo weak supervision for the generation of the aerial image and also add more variance to the embedding space. We denote the inverse perspective mapping of the input image by , computed following [38]. We perform the following optimization steps to condition the embedding space towards the desired viewpoint transformation. To find the text embedding that best characterises , we start from and optimize the text embedding with a frozen diffusion model, similar to Equation 1. Finding in the vicinity of instead of ensures that the text-image space corresponding to doesn’t get distorted to generate the poor quality IPM image. Next, we finetune the diffusion model using the denoising diffusion objective function to reconstruct at , similar to Equation 2. Again, only the LoRA layers are finetuned, the rest of the UNet is frozen.
Note that we find and by optimizing and , respectively for a small number of iterations. We need to ensure that , and are all in close vicinity. Our finetuning approach conditions the embedding space to encapsulate the details of and viewpoint, while having sufficient variance to generate large transformations required for the generation of the aerial image.
3.2 Mutual Information Guided Inference
Our next step is to use the finetuned diffusion model to generate the aerial view image for the text prompt . The text embedding for is . Diffusion denoising, conditioned on is capable of generating aerial images corresponding to . However, oftentimes, the contents of the generated aerial view image does not align well with the contents of . Consequently, to ensure high fidelity generations, our goal is to guide the contents of the aerial view image towards the contents of .
Similarity measures such as L1 distance, cosine similarity are capable of providing this guidance. However, they are not invariant to viewpoint/ structure. Since we want the two images to be similar (while observed from different viewpoints), using metrics that impose matching at the pixel (or feature) level is not the best approach. Rather, it is judicious to use the probability distribution of the features.
In information theory, mutual information quantifies the ‘amount of information’ obtained about one random variable by observing the other random variable. Mutual information has been used [81, 50, 35, 85] to measure the similarity between images in various computer vision tasks such as medical image registration, frame sampling, etc. It yields smooth cost functions for optimization [77]. The mutual information between two probability distribution functions (pdf) for two random variables is defined as where are the entropies of and is the joint entropy. Entropy of a random variable is a measure of its uncertainity, ;
Thus,
Hence, mutual information, in some sense, measures the distance between the actual joint distribution between two probability distributions and the distribution under an assumption that the two variables are completely independent. Thus, it is a measure of dependence [32] and can be used to measure the information between two images. In order to maximize the similarity in content between and the generated aerial image, we maximize the mutual information between them. We define our mutual information guidance function as follows.
Let denote the predicted latents at timestep . We denote as the latents of the final predicted image extrapolated from i.e. if the denoising were to proceed in a vanilla fashion in the same direction that computed , the latents of the final predicted image would be . At every step of sampling (except the final step), we wish to maximize the mutual information between and where are the latents corresponding to . Hence, the guidance function we wish to maximize is,
The computation of mutual information requires us to compute the marginal and joint probability density functions (pdf) of and . We construct 2D histograms of the latents (by reshaping the latents of size into ) and compute their marginal pdfs. The joint pdfs can then be computed from the marginal pdfs, which can be plugged into the formula for mutual information. Our next task is to use to guide the generation of the aerial image.
Guidance techniques such as classifier-free guidance [29], universal guidance [3] and steered diffusion [51] modify the sampling method to guide the image generation with feedback from the guidance function. The gradient of the guidance function w.r.t. the predicted noise at timestep is an indicator of the additional noise that needs to be removed from the latents to steer the generated image towards the guidance signal. Synonymously, the gradient of the guidance function w.r.t. the predicted latents is an indicator of the direction in which the latents need to move in order to maximize their alignment with the guidance function. Specifically, at every step of sampling (except the final step), we modify the predicted latents as . Note that we use the negative of the mutual information to compute the gradients since we want to maximize the mutual information between the generated latents and the input image.
4 Experiments and Results
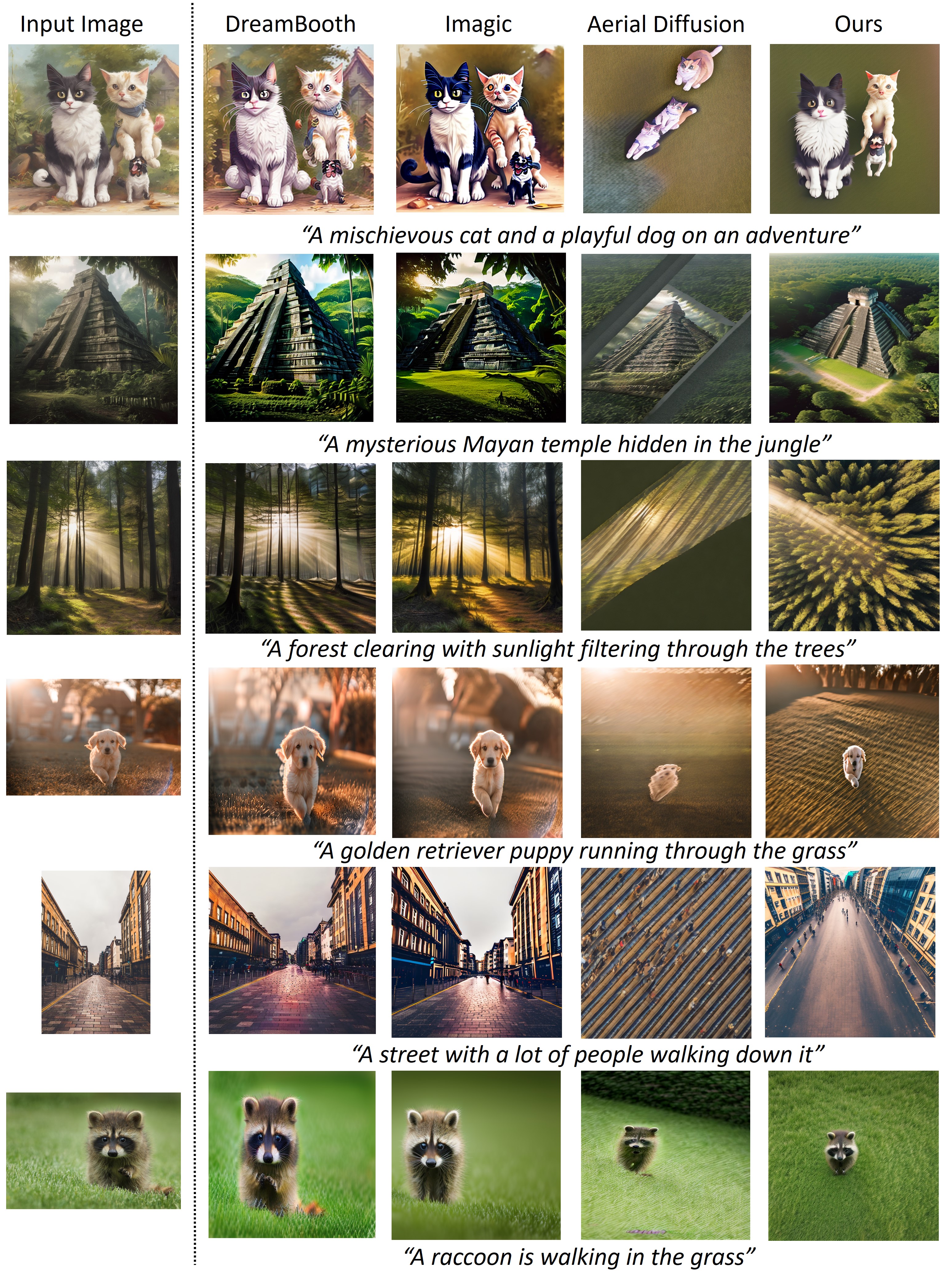
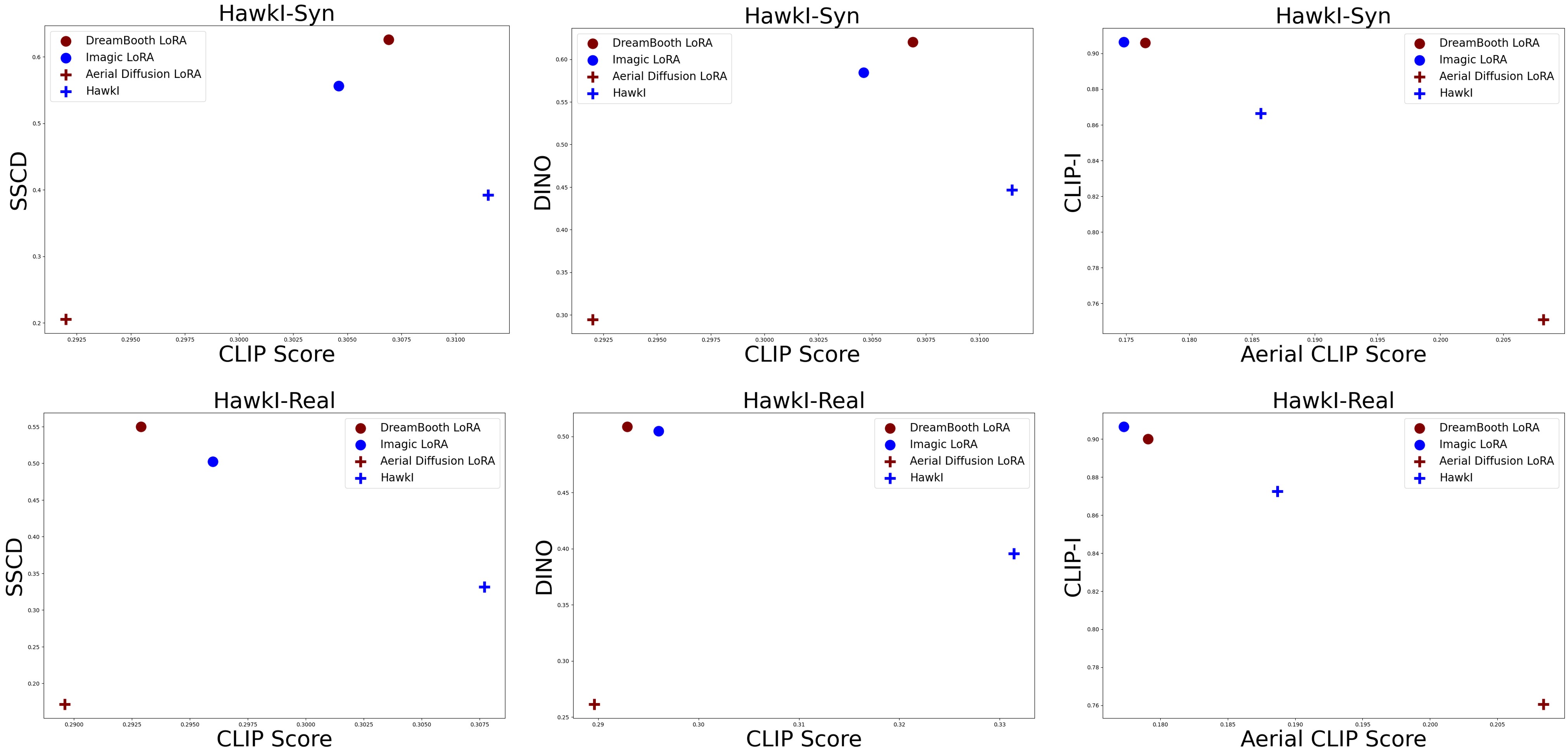
Data.
We collect a synthetic dataset, HawkI-Syn and a real dataset, HawkI-Real. Both datasets contain images across a wide variety of categories including indoor scenes, natural scenes, human actions, birds/ animals, animations, traffic scenes and architectures. HawkI-Syn contains images that were generated using Stable Diffusion XL [55]. To generate the text prompts for the generated images in HawkI-Syn, we used Large Languuage Models (LLMs) such as ChatGPT and Bard. HawkI-Real contains images downloaded from the Unsplash website, the text descriptions for these images were obtained using the BLIP-2 [40] model.
Training details.
We use the stable diffusion 2.1 model in all our experiments, ablations and comparisons. All our images (except for images in HawkI-Real) are at a resolution of . With respect to , we train the text embedding and the diffusion model for and iterations respectively, at a learning rate of and respectively. Using iterations to optimize the text embedding ensures that the text embedding at which is reconstructed is not too close to , which would make it biased towards otherwise. Similarly, it is not too far from either, hence the text embedding space learns the characteristics of . With respect to , we train the text embedding and the diffusion UNet for and iterations respectively. We want to be in close vicinity of ; we train the diffusion model for just iterations so that the model does not completely overfit to . The role of is to create variance and provide pseudo supervision, it is not an accurate approximation of the aerial view. We set the hyperparameter for mutual information guidance at or , the inference is run for steps.
Computational cost.
For each input image, HawkI takes minutes to perform test-time optimization on one NVIDIA A5000 GPU with 24 GB memory. The inference time is consistent with that of Stable Diffusion, about seconds to generate each sample with denoising steps. The computational cost is on par with text-based image personalization [65, 34] and text-based aerial-view synthesis [38] methods. The number of network parameters is also consistent across all these models, we use the Stable Diffusion v2.1 + LoRA backbone across methods.
Quantitative evaluation metrics.
We follow prior work on text-based image editing/ personalization and text-based view synthesis to evaluate our method:
-
•
Viewpoint and text alignment: We use the text description ‘aerial view, ’ + and ‘aerial view’, along with the generated image, to compute the CLIP-Score [58] and the A-CLIP Score respectively. The former indicates alignment of the generated image with the detailed textual description of the image describing the contents along with the viewpoint; the latter focuses more on the viewpoint.
-
•
Image fidelity and 3D coherence: To evaluate the overall alignment of the contents of the generated aerial-view image with the input image, we compute the CLIP-I score [65] which measures the cosine similarity between the embeddings of the aerial-view image and the input image in the CLIP space. For a better indicator of the fidelity and 3D coherence between the two images, we also use the self-supervised similarity detection metrics, DINOv2 [8, 52] and SSCD [54].
-
•
Top-1 accuracy on a downstream UAV task.
-
•
Ground-truth comparison with images sampled from 3D models using CLIP, LPIPS, and DINO metrics.
Higher values are desired for each of these metrics. Viewpoint faithfulness and fidelity w.r.t input image are a direct result of the bias-variance trade-off of the model, and high values for both are desired. However, as noted by Blau et. al. [5], maximizing both is not straightforward; inevitably one of the factors will degrade in response to the improvement in the other. For each input image, we generate aerial images, with random noise initializations, and choose the image with the highest CLIP + SSCD score (since CLIP is an indicator of viewpoint + content alignment and SSCD score measures the fidelity w.r.t. the input image).
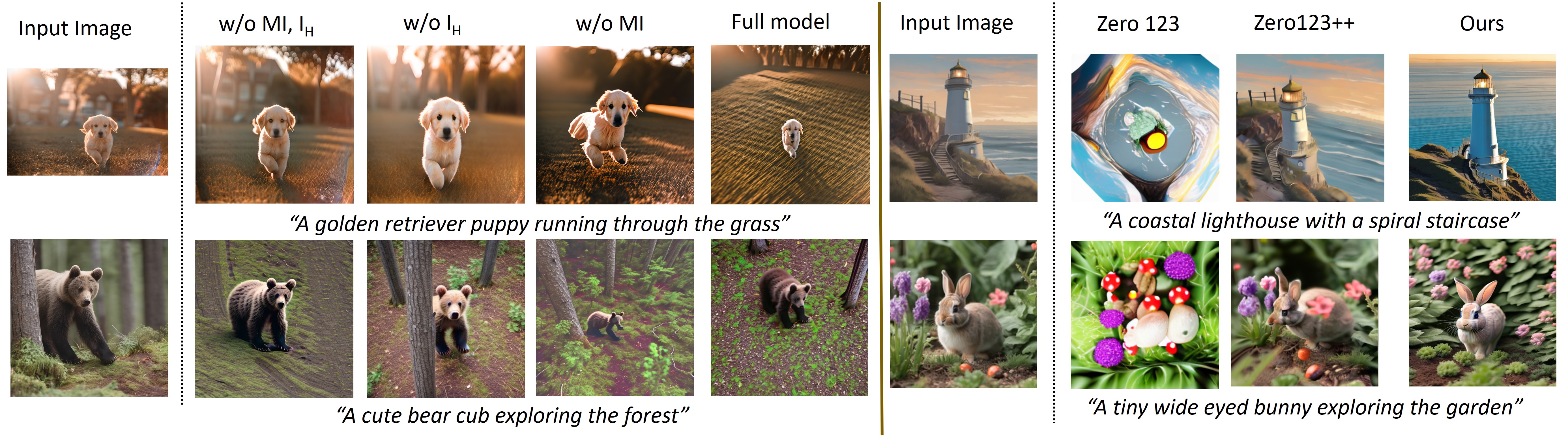
4.1 Comparisons against text + exemplar image based methods
We compare our method with DreamBooth LoRA [65], a text-based image personalization method; Imagic LoRA [34], a text-based image editing method; and Aerial Diffusion LoRA [38], a method for text-based aerial image generation from a single image. We keep the backbone stable diffusion model, image prompts, training details, and evaluation method consistent across all comparisons.
We show qualitative results in Figure 3. Our method is able to generate aerial views as per input image guidance across a diverse set of scenes. Our method generates results that are more aerial in viewpoint than DreamBooth and Imagic, while being largely consistent with the contents of the input image. Aerial Diffusion is unable to generate good quality images of scenes that have many objects. Our method is able to deal with complex scenes as well as modify the viewpoint.
We show the quantitative results in Figure 7. Our method achieves a higher CLIP score than all prior work, indicating that it is able to generate an aerial view of the scene with contents dictated by the text better than prior work. The A-CLIP score achieved by our method is higher than that of DreamBooth and Imagic, indicating better conformance to the aerial viewpoint. Even though the A-CLIP score of HawkI is lower than than of Aerial Diffusion, Aerial Diffusion generates poor quality images for scenes with more than one object and also has diagonal artifacts in its generated images, as we observe from the other metrics and qualitative results, thus, offsetting its high A-CLIP Score.
CLIP-I and self-supervised metrics such as SSCD and DINO are not viewpoint invariant. In many cases, since Imagic and DreamBooth generate views close to the input view, rather than aerial views, it is natural for them to have higher CLIP-I, SSCD and DINO Scores. Our method has a much higher CLIP-I, SSCD and DINO score than Aerial Diffusion, showing considerable improvement over prior work in retaining the fidelity and 3D consistency w.r.t. the input image, while modifying the viewpoint. In summary, our specialized aerial-view synthesis method achieves the best viewpoint-fidelity trade-off amongst all related prior work.
4.2 Comparisons against 3D based novel view synthesis (NVS) methods
We compare with state-of-the-art benchmark methods on stable diffusion based novel view synthesis from a single image in Figure 5. Zero-1-to-3[48] and Zero123++ [70], both, train on large amounts of multi-view and 3D data from Objaverse [15] contain 3D models; in addition to leveraging a pretrained text-to-2Dimage stable diffusion model. In contrast, our method does not use any multi-view or 3D information and is capable of generating better results in multiple cases. Another task-level difference between our method and prior work on NVS is that the latter aim to explicitly control the camera angle and generate 3D objects in Zero-1-to-3[48], the camera-angle generated by our method is arbitrary within the realms of the text control. The CLIP scores on HawkI-Syn for Zero123++ and HawkI are and respectively, the DINO scores are and respectively. On HawkI-Real, the CLIP score for Zero123++ and HawkI are and respectively, the DINO scores are and respectively. Our aerial-view synthesis method, even without any 3D/ multi-view information and large dataset training, is better than or comparable to 3D-based NVS methods.
4.3 Downstream application.
We performed a proof-of-concept experiment using HawkI to generate synthetic images for key-frames of scene-based human actions in the UAV Human [41] dataset. By computing the L2 distance between self-supervised features of UAV Human video frames and synthetic images on actions: ‘smoking’, ‘pushing someone’, ‘high five’ and ‘walking’, we achieved a accuracy in zero-shot action recognition, representing a (absolute) improvement.
4.4 Ground-truth comparison
We obtained 3D models and text descriptions from Dreamfusion [56] to extract the front-view and top-view (ground-truth or GT). We evaluated Zero123++ and HawkI using CLIP Scores (higher the better), and the numbers were , , respectively. The DINO score (higher the better), which measures self-supervised similarity between the generated images and the GT, are , for Zero123++ and HawkI respectively. The LPIPS scores (lower the better) are , for Zero123++ and HawkI respectively - our method generates images that are higher in elevation due to ‘aerial view’ being the text-control. Overall, our 3D-free method, built on stable diffusion, is comparable to 3D methods such as Zero123++ which uses stable diffusion + 800k+ 3D objects.
4.5 Ablation studies
We show ablation experiments in Figure 5. In the second column, we show results of our model where it is neither finetuned on the homography image nor uses mutual information guidance for sampling. Thus, the text embedding for the input image, followed by the diffusion UNet are finetuned and the diffusion model generates the aerial image by diffusion denoising, without any mutual information guidance. Many of the generated images either have low fidelity or have low correspondence to the aerial viewpoint. In the experiment in the third column, we add mutual information guidance to the model in column 2. We see higher fidelity (than column 2) of the generated images w.r.t. the input image. In the fourth column, we add the homography image finetuning step to the model in column 2, but do not use mutual information guidance at inference. The generated images, in many cases, are aerial, but have lower fidelity w.r.t. the input image. In the final column, we show results with our full model. The generated images achieve the best trade-off between the viewpoint being aerial and fidelity w.r.t. the input image, in comparison to all ablation experiments. Quantitative analysis:
-
•
Effect of : To study the effect of , we compare the CLIP and A-CLIP scores for the full model vs full model w/o . The scores in all cases where is not present are lower, indicating lower consistency with the viewpoint being ‘aerial’. For instance, on HawkI-Real, the CLIP scores for the full model and full model w/o are and respectively. The A-CLIP scores for the full model and full model w/o are and respectively.
-
•
Effect of : To study the effect of , we compare the SSCD and DINO scores for the full model vs model w/o . The scores in all cases where is not present are lower. For instance, on HawkI-Real, the SSCD scores for the full model and model w/o are and respectively. The DINO scores for the full model and model w/o are and respectively.
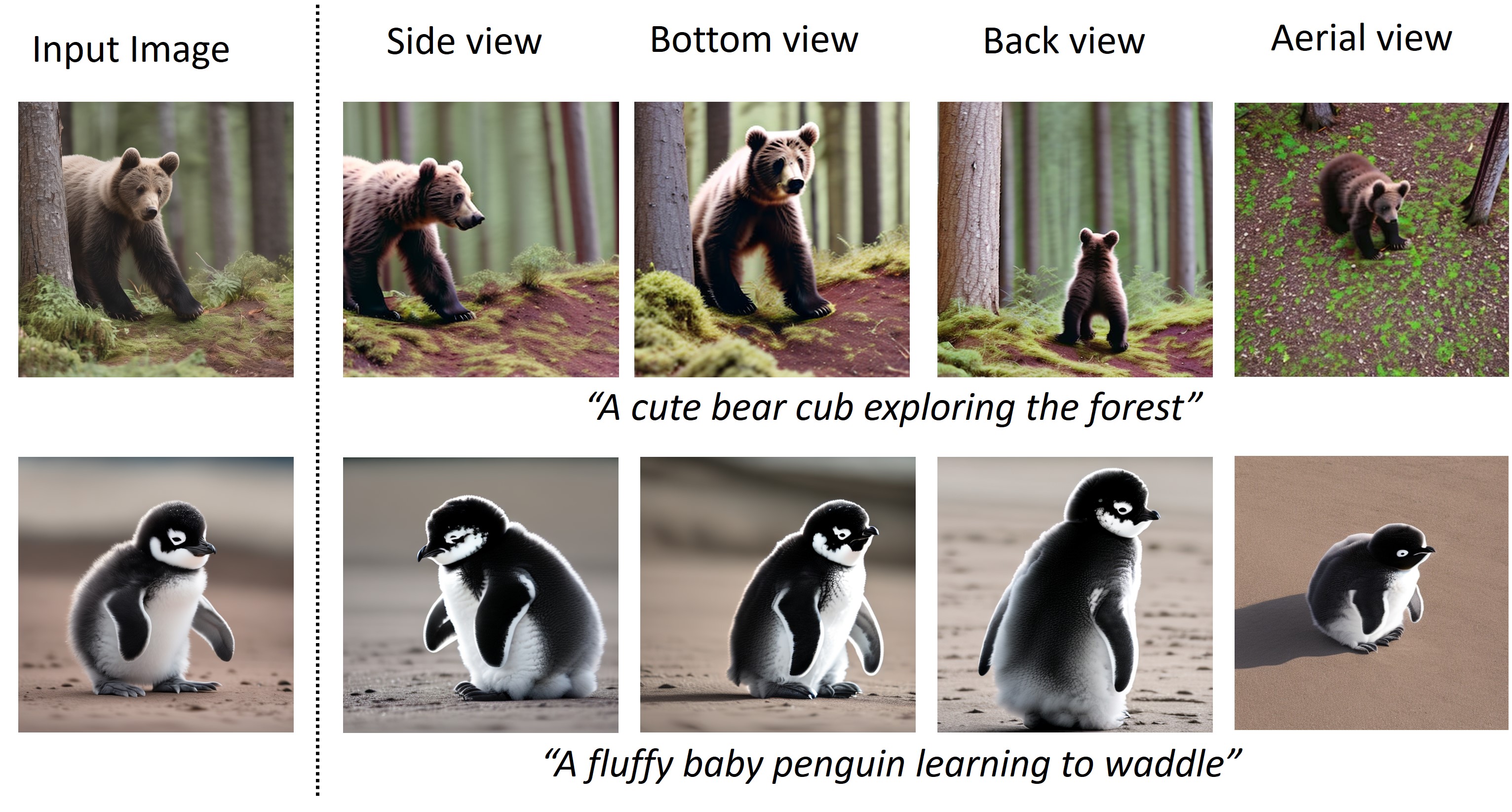
4.6 Comparison with other metrics for guidance.
We compare with two other metrics for diffusion guidance at inference: (i) L2 distance between the features of the generated image and the input image, (ii) a metric inspired by Wasserstein distance or Earth Mover’s distance, for which we compute the distance between the histograms of the probability distributions of the two images. Our mutual information guidance method is better at preserving the fidelity w.r.t the input image, as evidenced by higher SSCD scores. The SSCD score on HawkI-Real for Wasserstein guidance, guidance, and mutual information guidance are , and respectively. The DINO score on HawkI-Real for Wasserstein guidance, guidance, and mutual information guidance are , and respectively.
4.7 Text controlled view synthesis: Other views
HawkI can be extended to generate other text-controlled views such as side view, bottom view and back view. For the results shown in Figure 19, we modify the target text to indicate different viewpoints, and retain the other finetuning/ inference details.
4.8 3D-free HawkI + 3D priors?
Our 3D-free approach complements 3D-based methods [70, 42]. While 3D data collection and large-scale training are expensive and unsustainable, front-view 2D images are more readily available[35]. Hence, it is beneficial to solve the fundamental problems associated with the task in a data-efficient (or 3D-free) manner. Our goal is to push the frontiers of 3D-free aerial-view generation from a single image, achieving results comparable to methods [70] using stable diffusion + 800k 3D objects. That being said, HawkI be combined with 3D approaches, to multiply the benefits of 3D and 3D-free approaches. To validate this, we replaced the homography prior with the image from the 3D-based Zero123++ [70] approach and evaluated our HawkI model on images from HawkI-Syn. The CLIP scores for Zero123++, HawkI, and “HawkI with Zero123++ prior” are , , and , respectively. The DINO scores for Zero123++, HawkI, and “HawkI with Zero123++ prior” are , , and , respectively, thus demonstrating our claim!. Also, using 3D priors with HawkI allows finer camera control.
More results. Please refer to the supplementary material for (i) more qualitative comparisons with text + exemplar image and 3D based NVS methods, (ii) qualitative comparisons with other guidance metrics, (iii) detailed quantitative results for ablations, (iv) comparison of IPM with data augmentation, (v) more results on other text-controlled views, (vi) qualitative comparisons with warping + outpainting (scene extrapolation) and ControlNet variations.
5 Conclusions, Limitations and Future Work
We present a novel method for aerial view synthesis. Our goal is to leverage pretrained text-to-image models to advance the frontiers of text + exemplar image based view synthesis without any additional 3D or multi-view data at train/ test time. Our method has a few limitations, which form an avenue for future work - (i) Further exploration of combining our 3D-free approach with 3D priors can enable the generation of camera-controlled views. (ii) Alleviating hallucination, a widespread issue in all diffusion models, will enable the generation of images with higher fidelity w.r.t. input images. Other directions for future work are - (i) IPM is as an important tool for aerial view generation – similar homography projections to synthesize other views can be explored, (ii) our mutual information guidance formulation can be used in other image editing and prsonalization problems, (iii) using generated data for various downstream UAV and aerial-view applications such as cross-view mapping, 3D reconstruction and synthetic data augmentation.
Acknowledgements:
This research has been supported by ARO Grants W911NF2110026 and Army Cooperative Agreement W911NF2120076
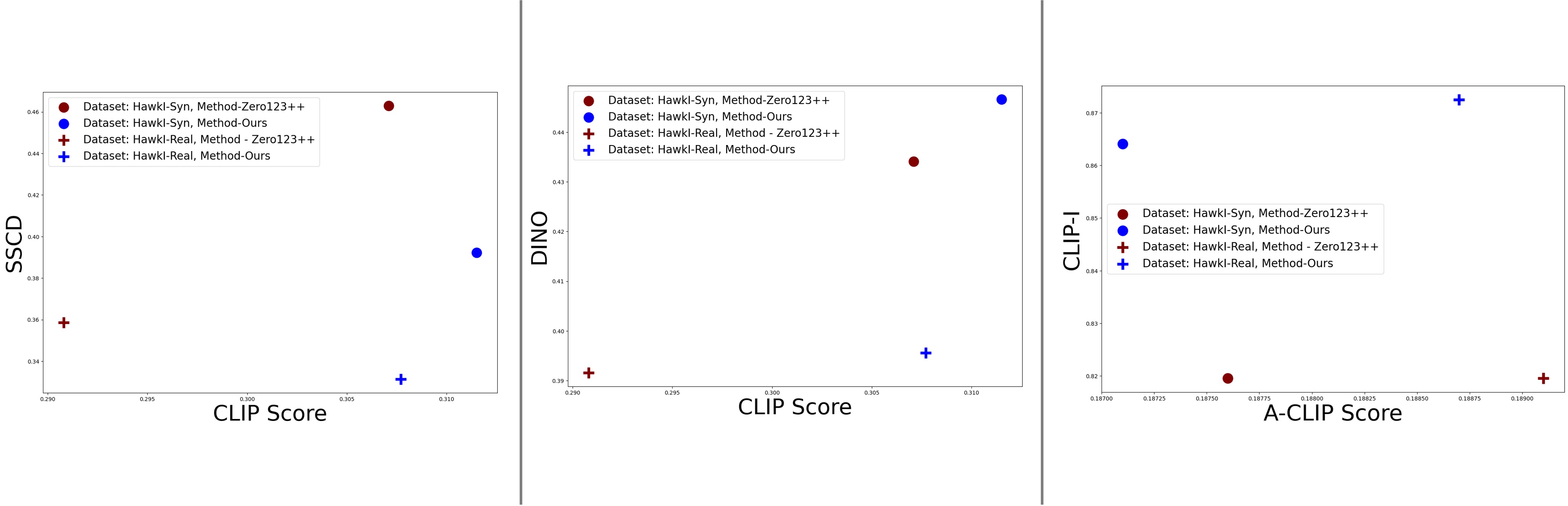
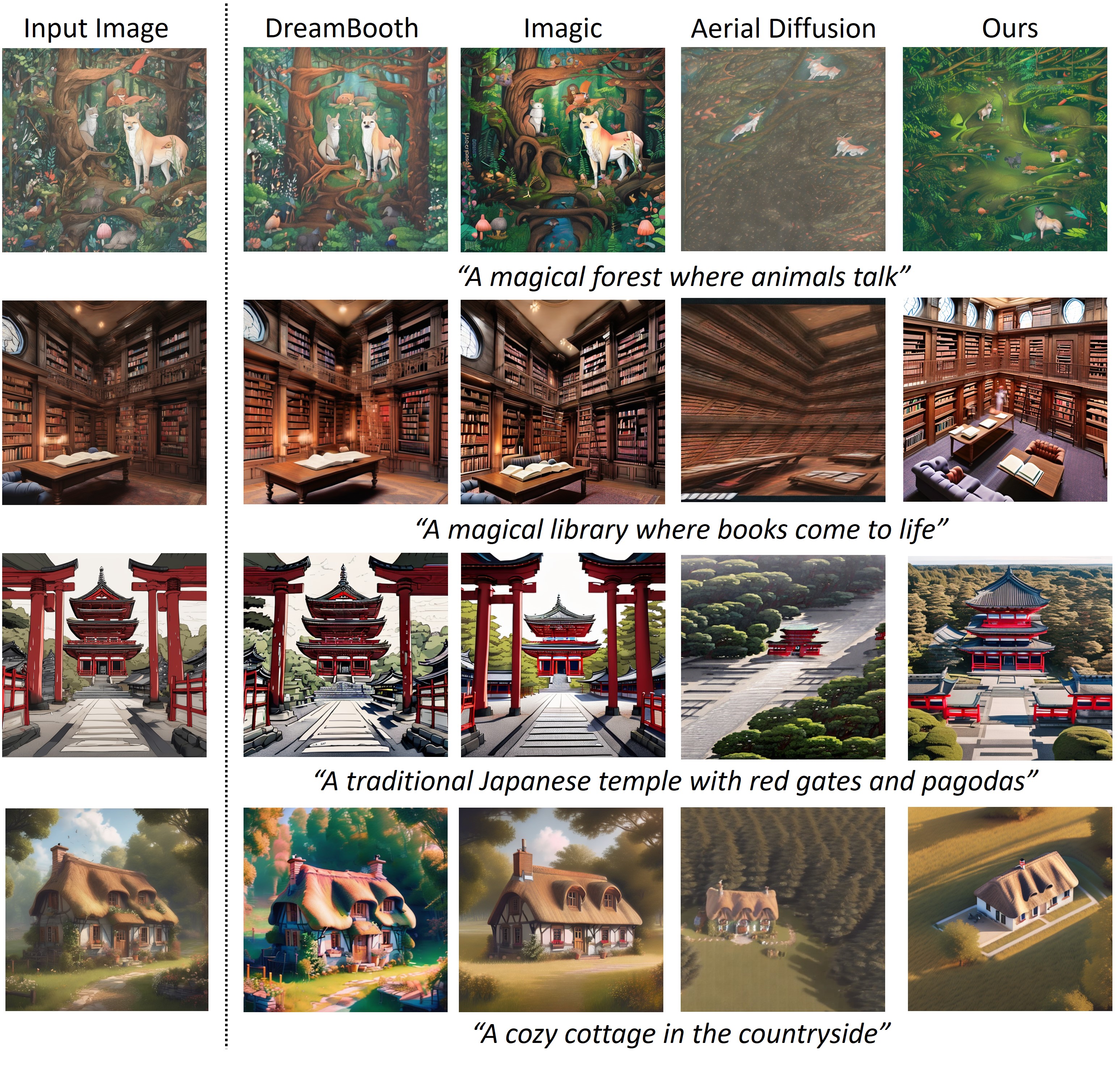
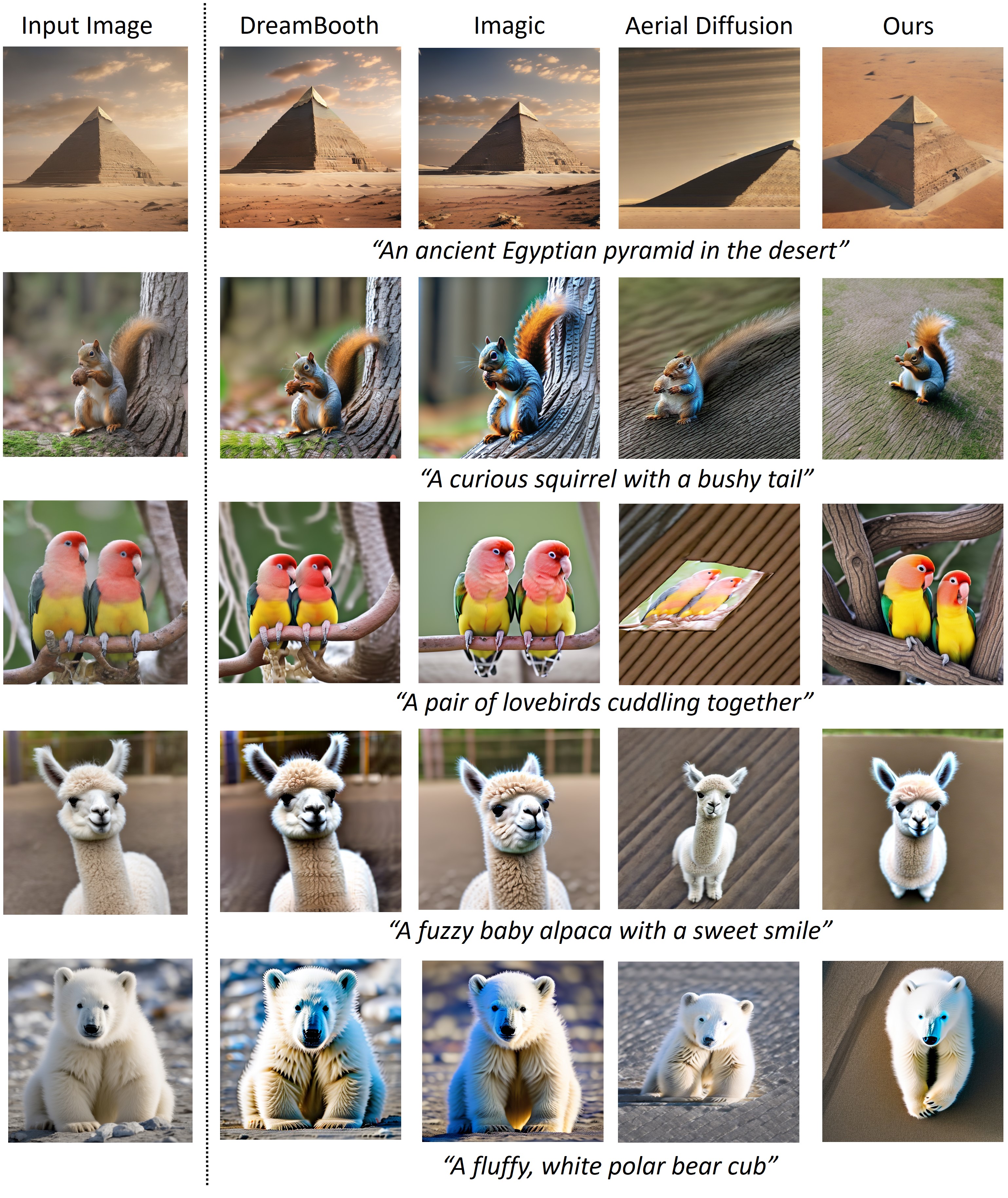
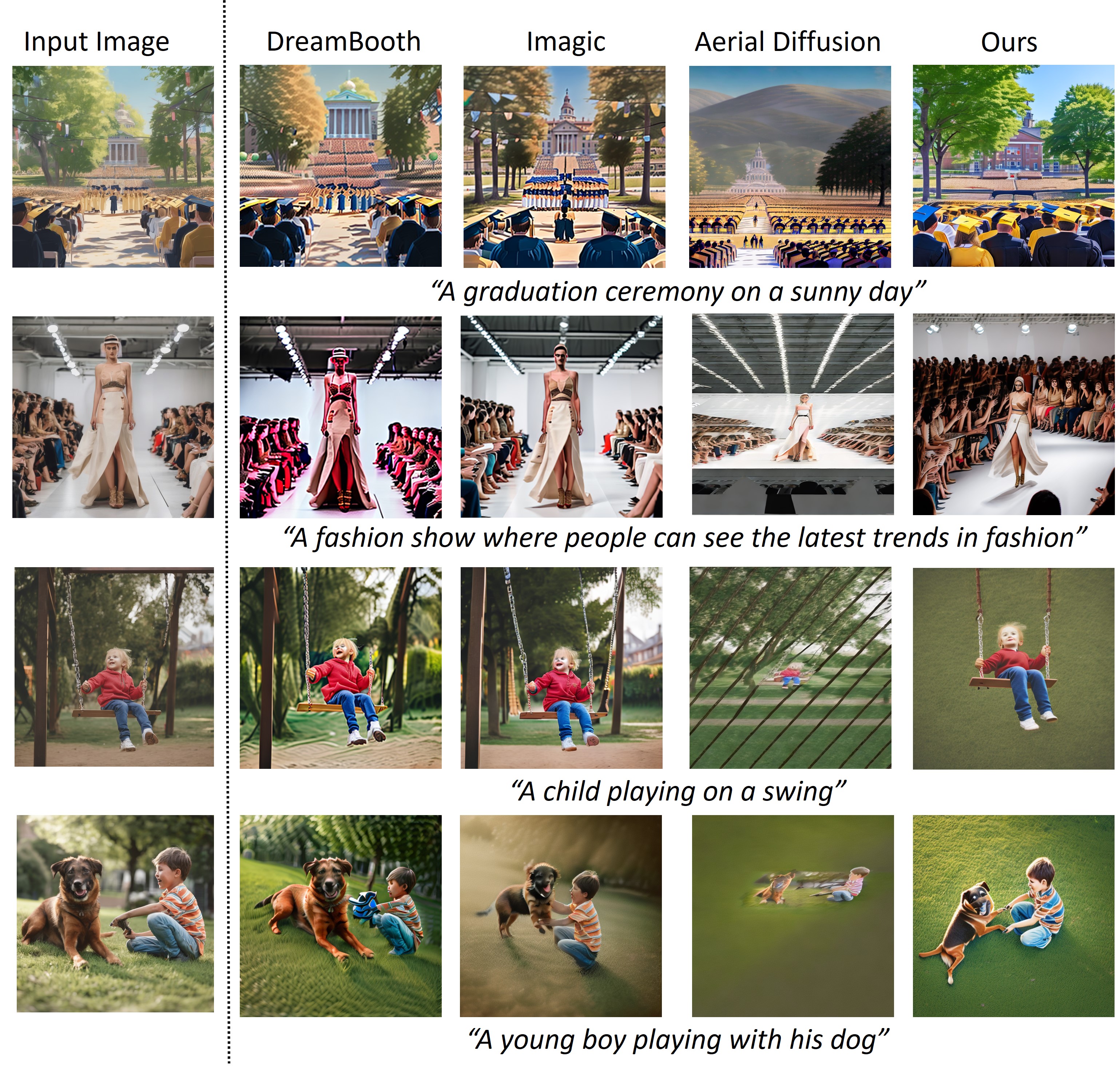
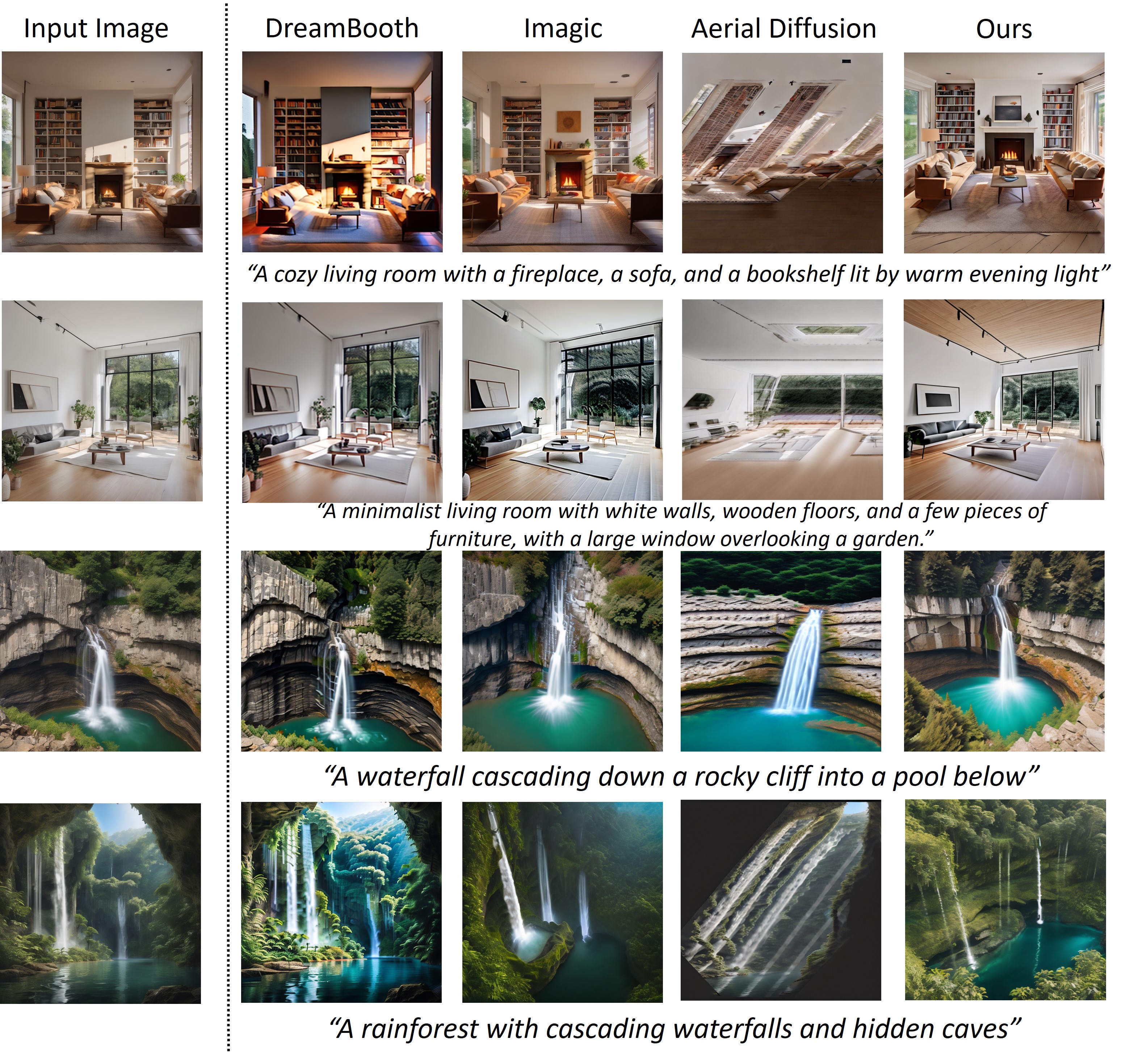
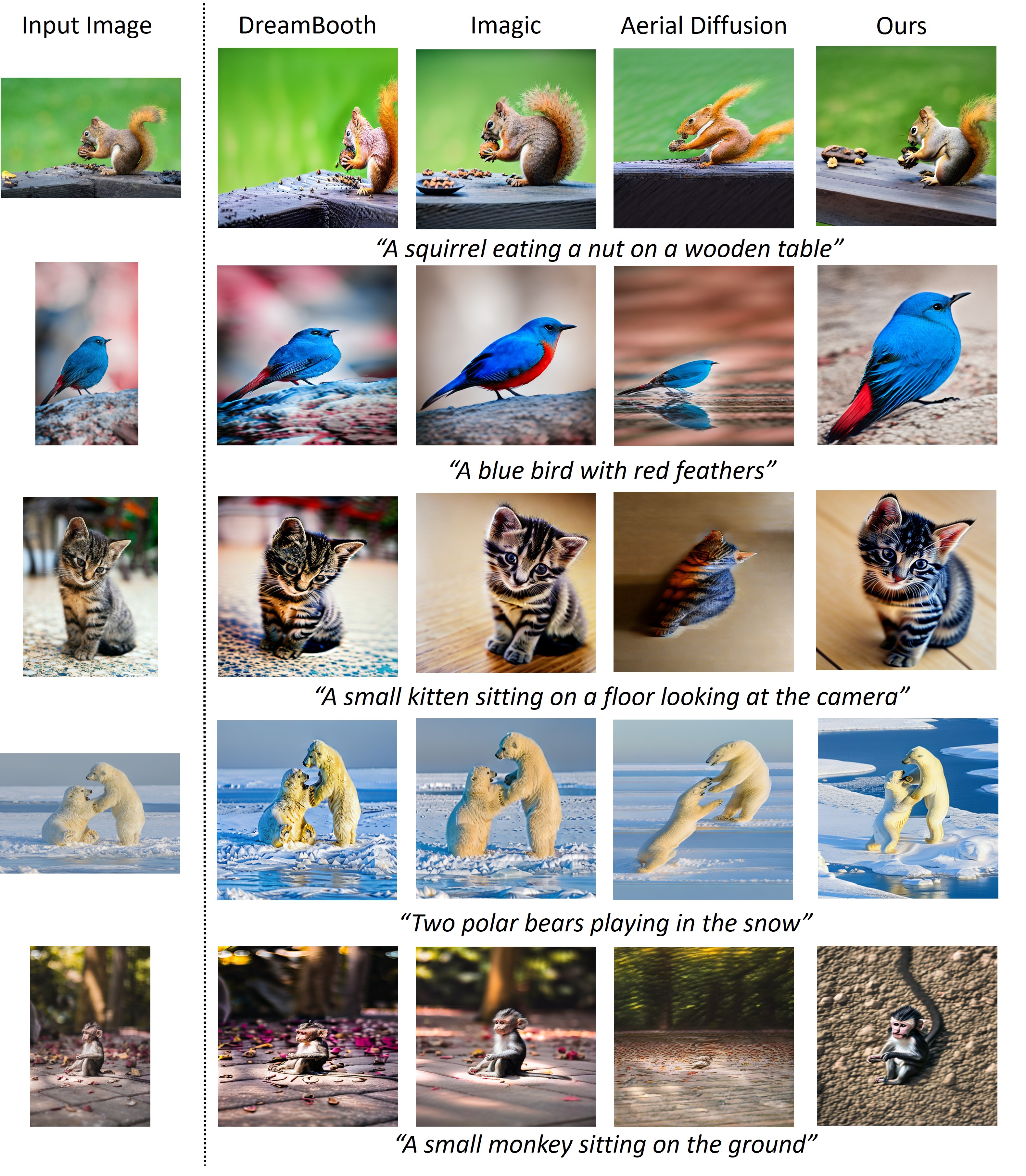
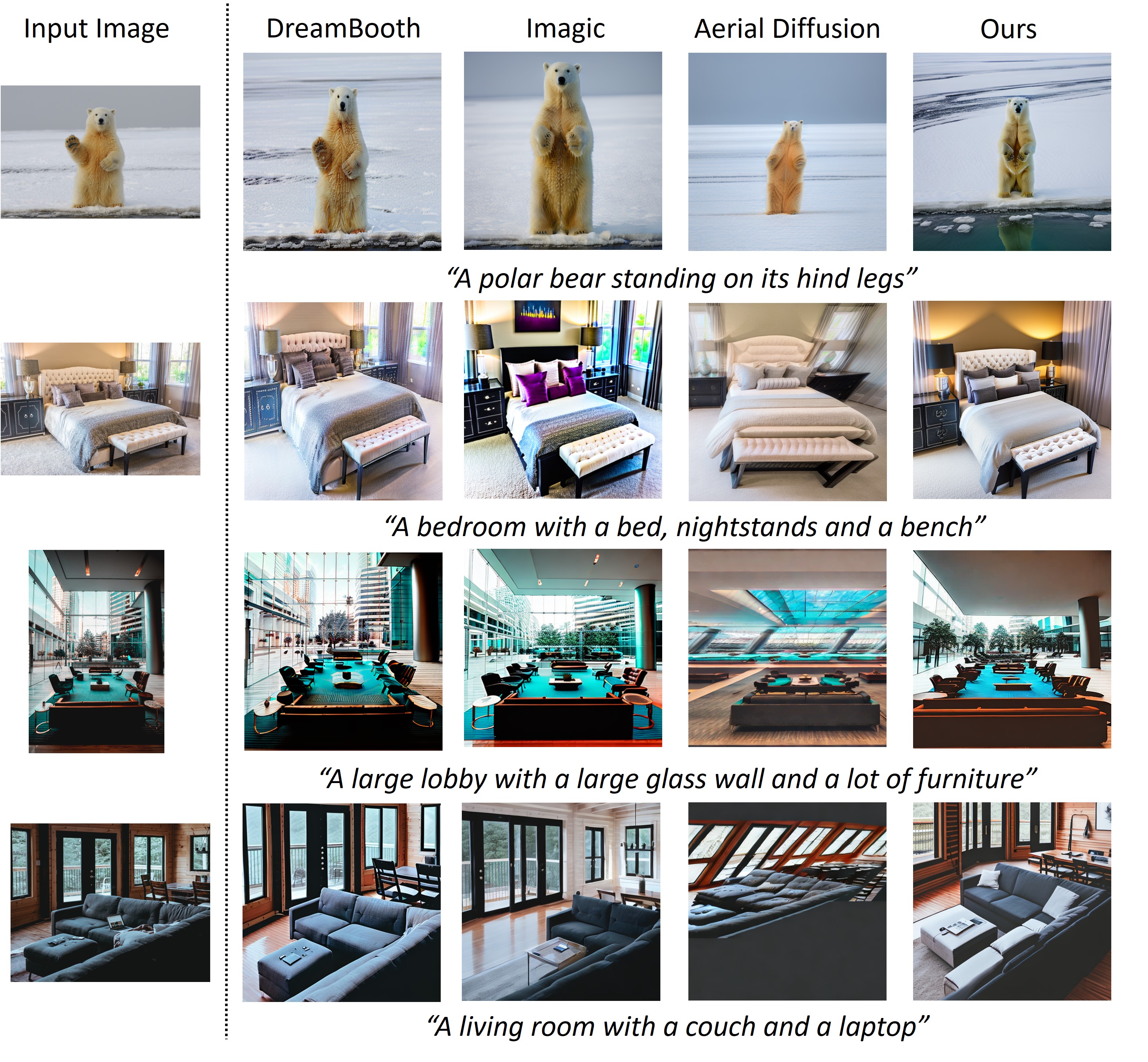
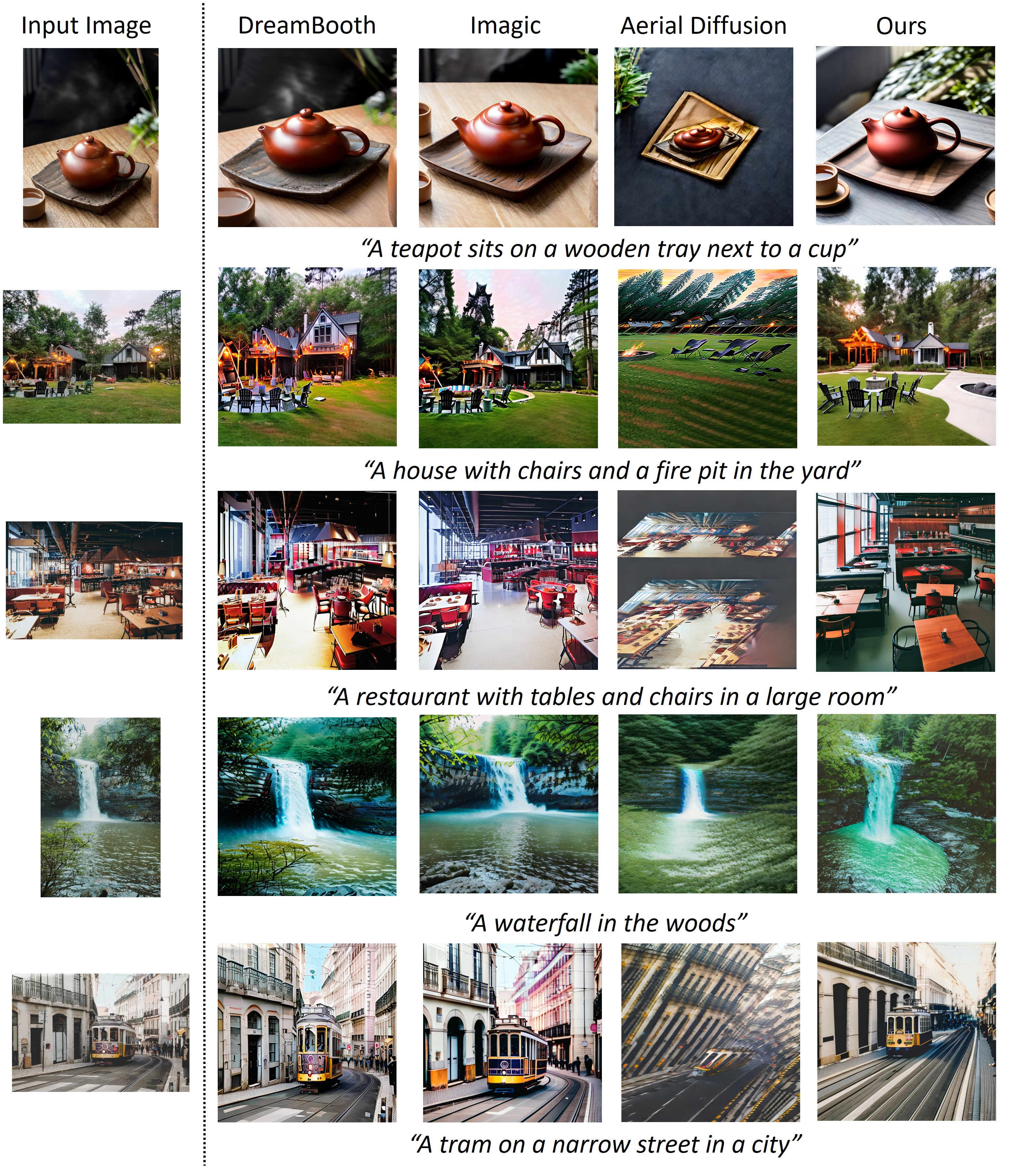
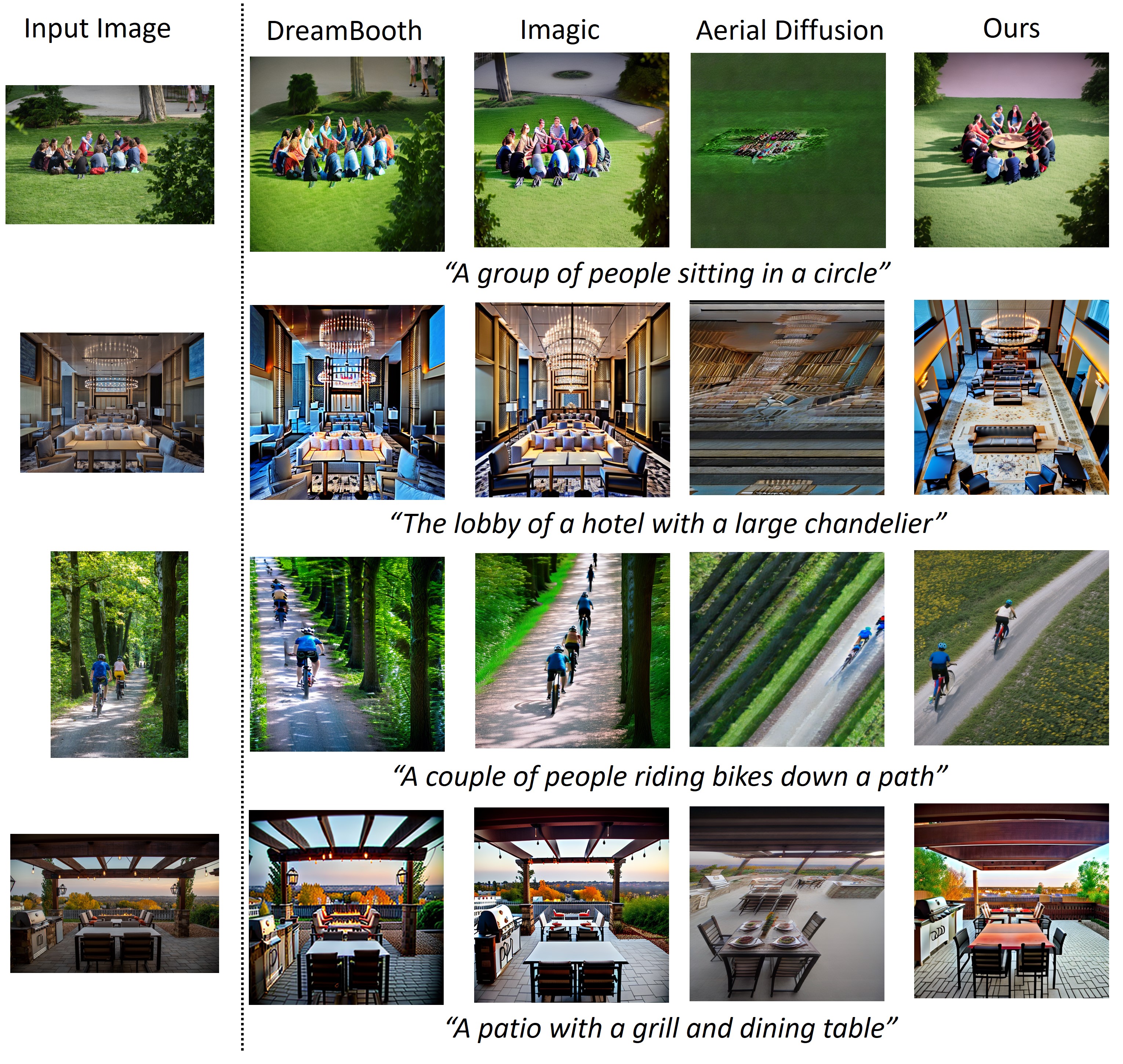
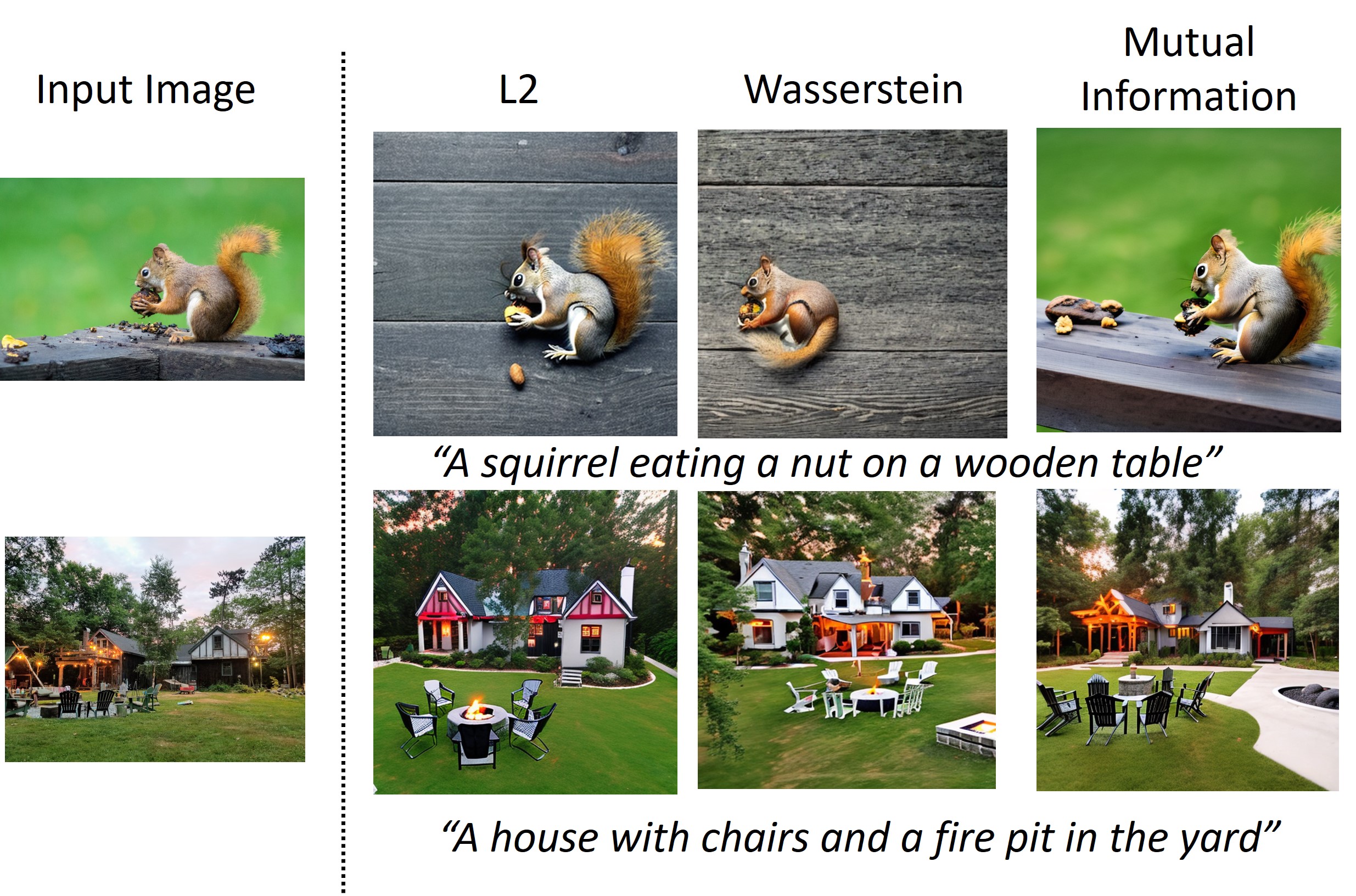
Method | CLIP | A-CLIP | SSCD | DINO | CLIP-I |
Dataset: HawkI - Syn | |||||
w/o MI, w/o | |||||
w/o MI | |||||
w/o | |||||
Ours () | |||||
Ours () | |||||
Dataset: HawkI - Real | |||||
w/o MI, w/o | |||||
w/o MI | |||||
w/o | |||||
Ours () | |||||
Ours () |
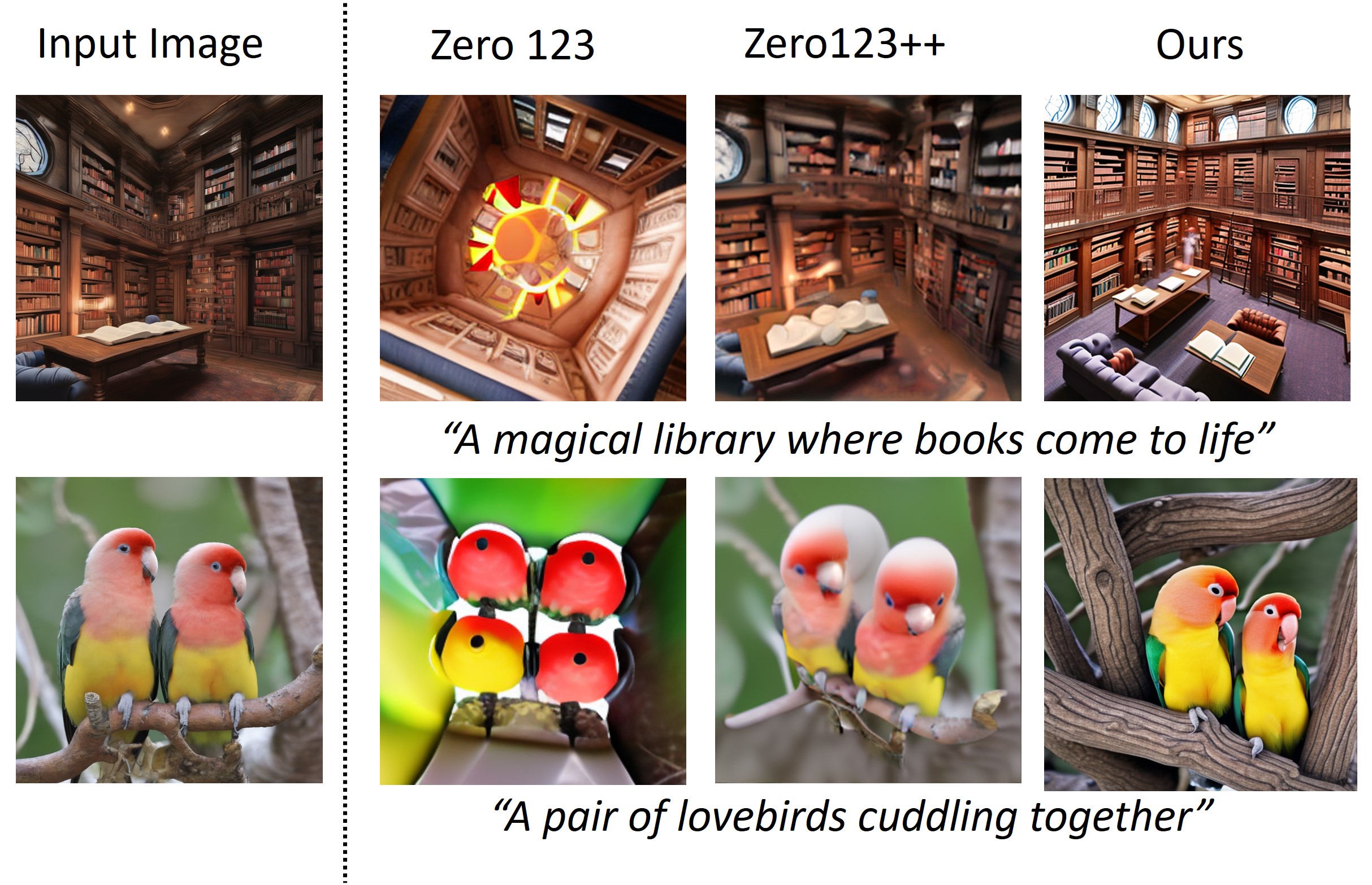
3D generation methods like Zero123++ are capable of generating different views with high fidelity by using pretrained stable diffusion models to finetune on large-scale 3D objects datasets. However, their generalization capabilities are limited. Our method is able to generate high quality aerial images for the given input images without any 3D data and using just the pretrained text-to-2D image stable diffusion model, however, there is scope for improving the fidelity of the generated aerial image w.r.t the input image. Moreover, our method controls the viewpoint via text and does not provide the provision to quantitatively control the camera angle. Both of these limitations of our method can be alleviated by exploring the combination of pretrained Zero123++ models (or other 3D models) and our method, as a part of future work.
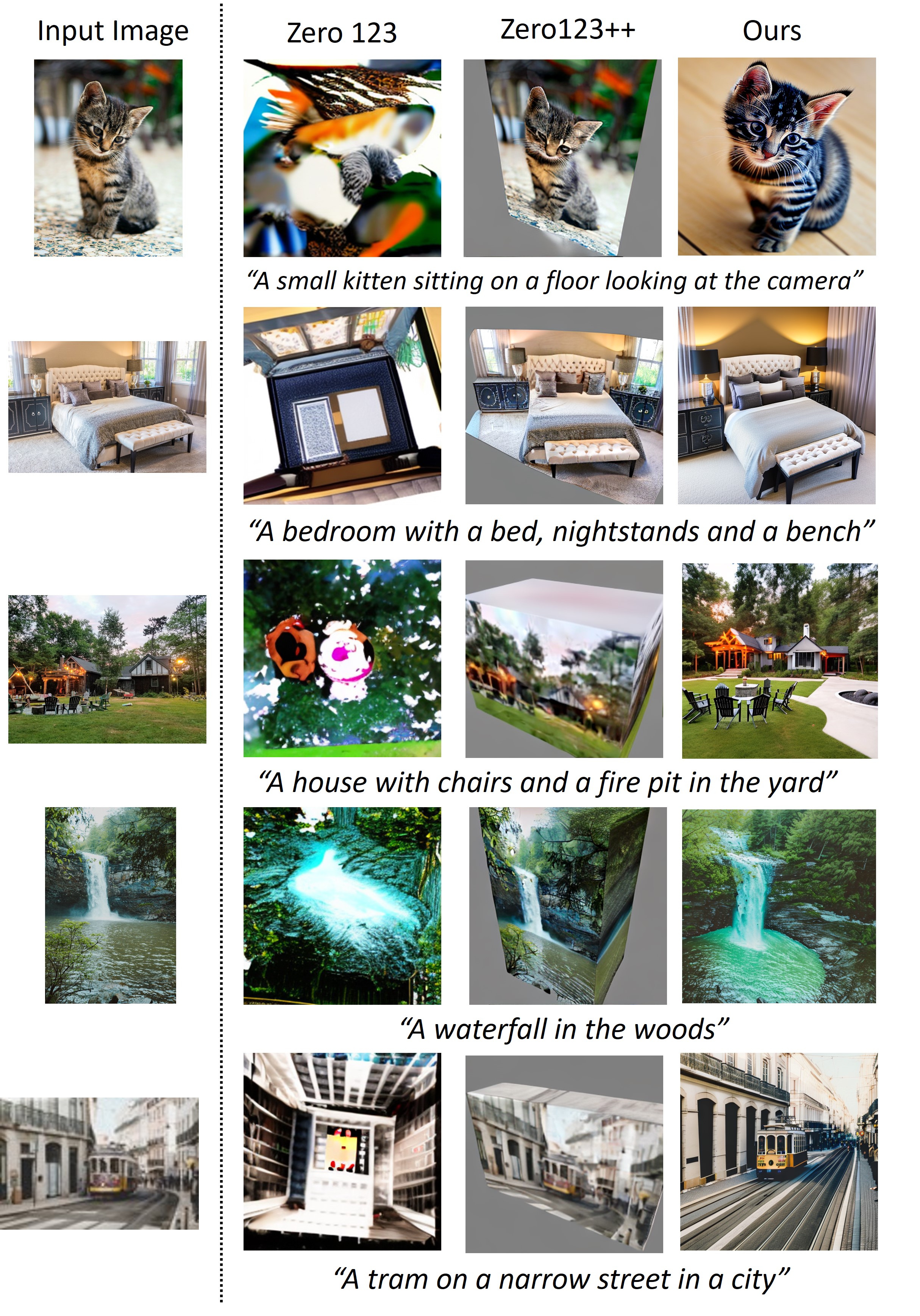
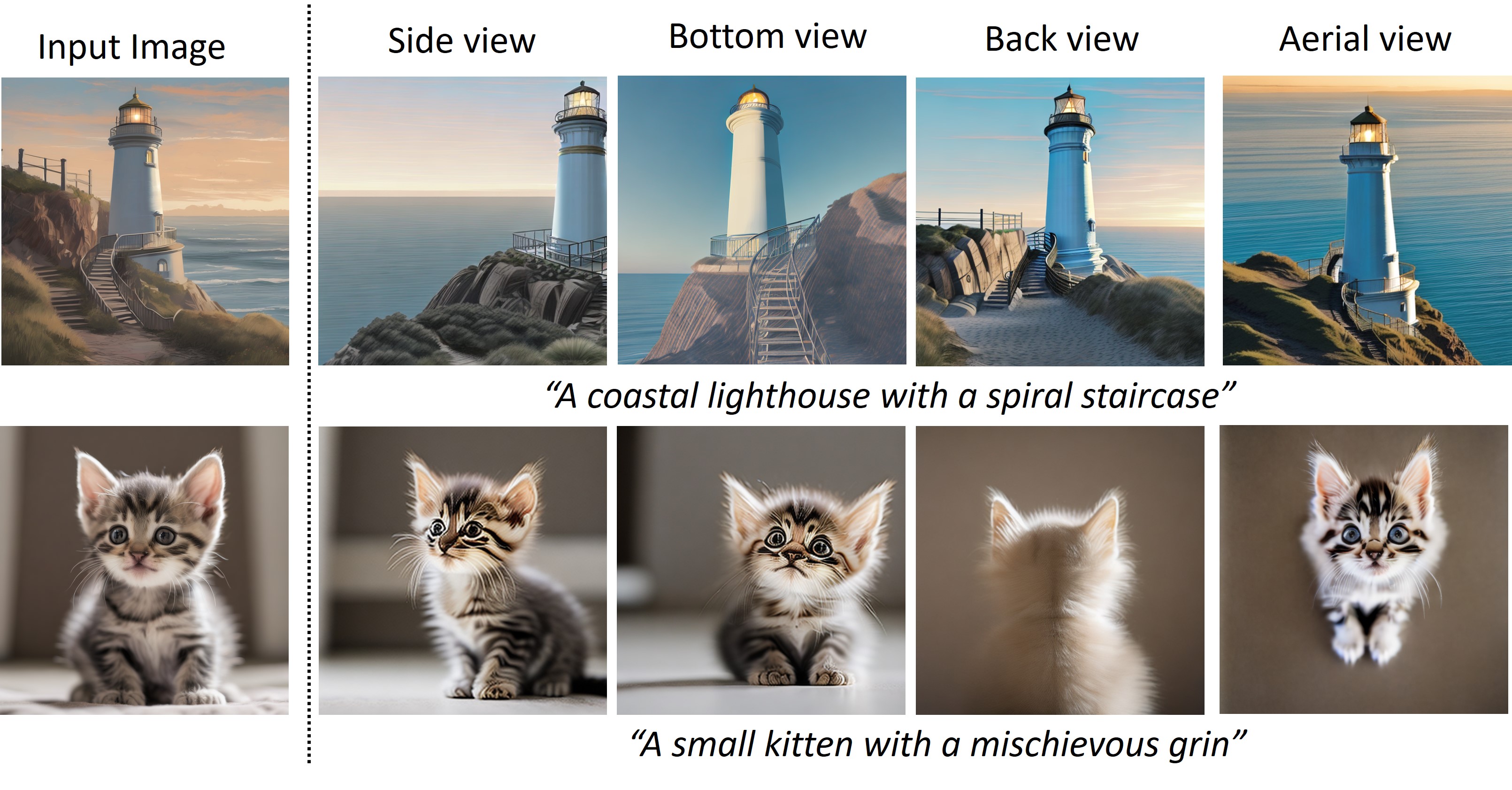
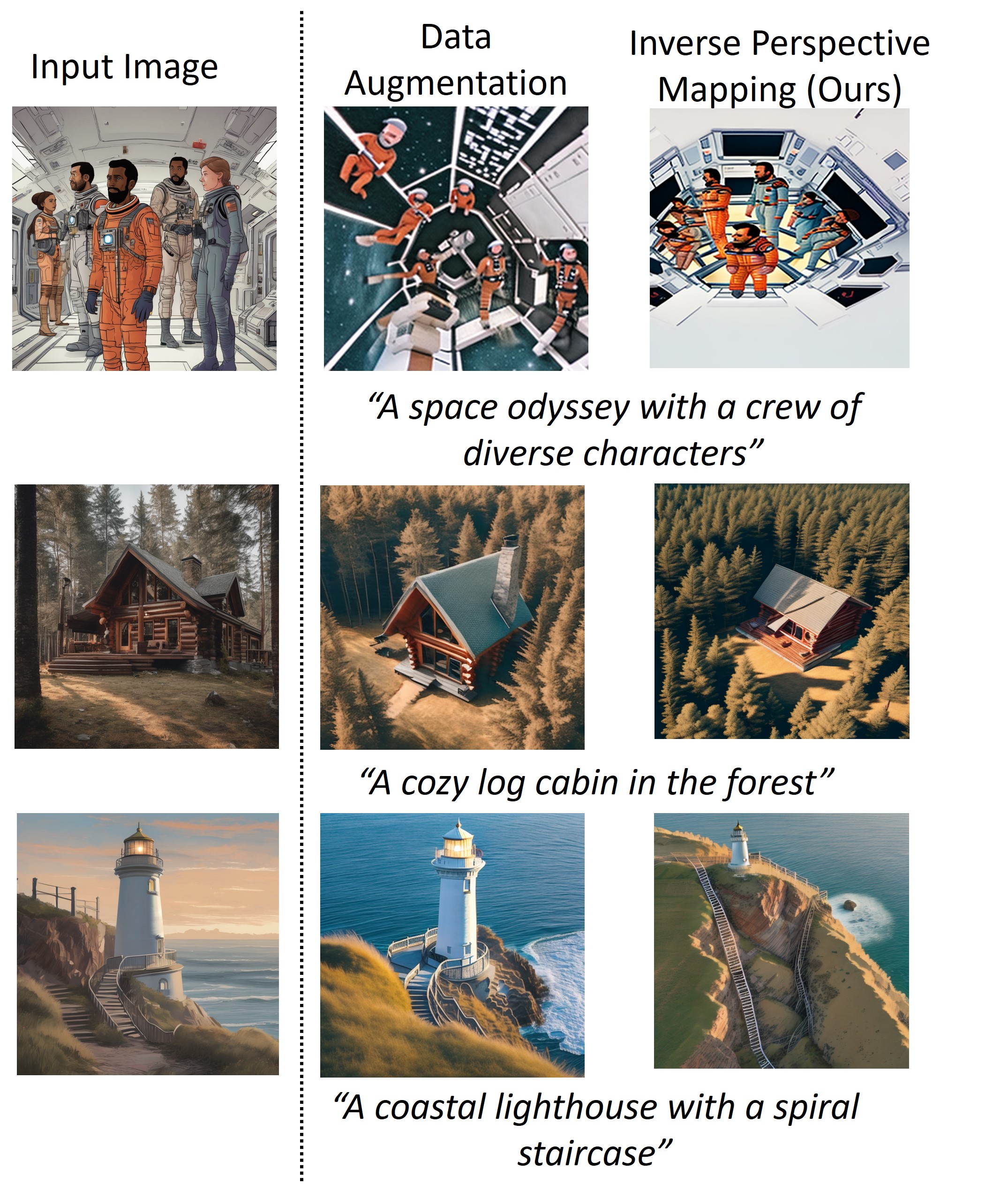
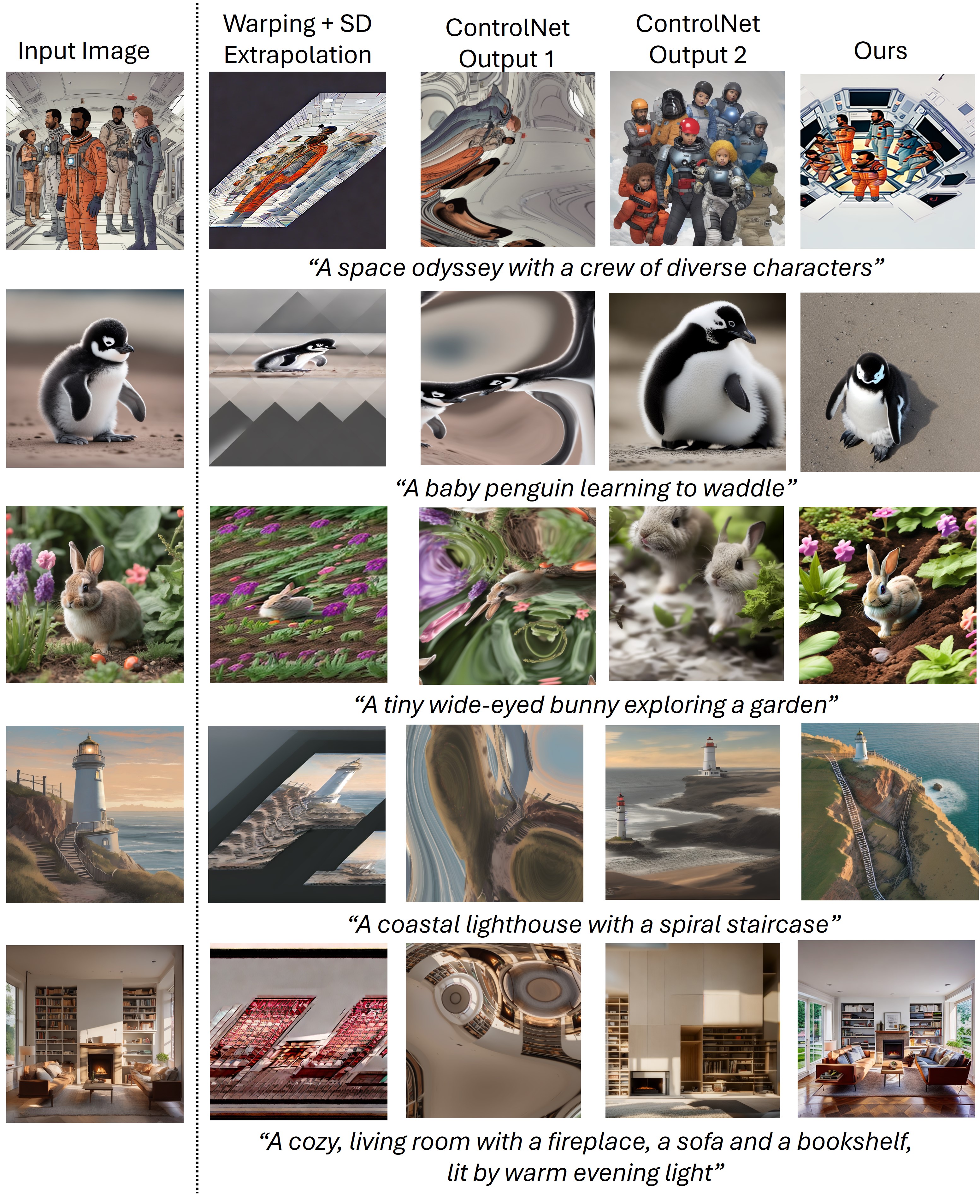
References
- [1] Syed Ammar Abbas and Andrew Zisserman. A geometric approach to obtain a bird’s eye view from an image. In Proceedings of the IEEE/CVF International Conference on Computer Vision Workshops, pages 0–0, 2019.
- [2] Shervin Ardeshir and Ali Borji. Integrating egocentric videos in top-view surveillance videos: Joint identification and temporal alignment. In Proceedings of the European Conference on Computer Vision (ECCV), pages 285–300, 2018.
- [3] Arpit Bansal, Hong-Min Chu, Avi Schwarzschild, Soumyadip Sengupta, Micah Goldblum, Jonas Geiping, and Tom Goldstein. Universal guidance for diffusion models. In Proceedings of the IEEE/CVF Conference on Computer Vision and Pattern Recognition, pages 843–852, 2023.
- [4] Mohammadamin Barekatain, Miquel Martí, Hsueh-Fu Shih, Samuel Murray, Kotaro Nakayama, Yutaka Matsuo, and Helmut Prendinger. Okutama-action: An aerial view video dataset for concurrent human action detection. In Proceedings of the IEEE conference on computer vision and pattern recognition workshops, pages 28–35, 2017.
- [5] Yochai Blau and Tomer Michaeli. The perception-distortion tradeoff. In Proceedings of the IEEE conference on computer vision and pattern recognition, pages 6228–6237, 2018.
- [6] James Burgess, Kuan-Chieh Wang, and Serena Yeung. Viewpoint textual inversion: Unleashing novel view synthesis with pretrained 2d diffusion models. arXiv preprint arXiv:2309.07986, 2023.
- [7] Shengqu Cai, Eric Ryan Chan, Songyou Peng, Mohamad Shahbazi, Anton Obukhov, Luc Van Gool, and Gordon Wetzstein. Diffdreamer: Towards consistent unsupervised single-view scene extrapolation with conditional diffusion models. In Proceedings of the IEEE/CVF International Conference on Computer Vision, pages 2139–2150, 2023.
- [8] Mathilde Caron, Hugo Touvron, Ishan Misra, Hervé Jégou, Julien Mairal, Piotr Bojanowski, and Armand Joulin. Emerging properties in self-supervised vision transformers. In Proceedings of the IEEE/CVF international conference on computer vision, pages 9650–9660, 2021.
- [9] Qifeng Chen and Vladlen Koltun. Photographic image synthesis with cascaded refinement networks. In Proceedings of the IEEE international conference on computer vision, pages 1511–1520, 2017.
- [10] Xiaozhi Chen, Huimin Ma, Ji Wan, Bo Li, and Tian Xia. Multi-view 3d object detection network for autonomous driving. In Proceedings of the IEEE conference on Computer Vision and Pattern Recognition, pages 1907–1915, 2017.
- [11] Yiwen Chen, Chi Zhang, Xiaofeng Yang, Zhongang Cai, Gang Yu, Lei Yang, and Guosheng Lin. It3d: Improved text-to-3d generation with explicit view synthesis. arXiv preprint arXiv:2308.11473, 2023.
- [12] Jinwoo Choi, Gaurav Sharma, Manmohan Chandraker, and Jia-Bin Huang. Unsupervised and semi-supervised domain adaptation for action recognition from drones. In Proceedings of the IEEE/CVF Winter Conference on Applications of Computer Vision, pages 1717–1726, 2020.
- [13] Guillaume Couairon, Jakob Verbeek, Holger Schwenk, and Matthieu Cord. Diffedit: Diffusion-based semantic image editing with mask guidance. arXiv preprint arXiv:2210.11427, 2022.
- [14] Giacomo D’Amicantonio, Egor Bondarev, et al. Automated camera calibration via homography estimation with gnns. In Proceedings of the IEEE/CVF Winter Conference on Applications of Computer Vision, pages 5876–5883, 2024.
- [15] Matt Deitke, Dustin Schwenk, Jordi Salvador, Luca Weihs, Oscar Michel, Eli VanderBilt, Ludwig Schmidt, Kiana Ehsani, Aniruddha Kembhavi, and Ali Farhadi. Objaverse: A universe of annotated 3d objects. In Proceedings of the IEEE/CVF Conference on Computer Vision and Pattern Recognition, pages 13142–13153, 2023.
- [16] Congyue Deng, Chiyu Jiang, Charles R Qi, Xinchen Yan, Yin Zhou, Leonidas Guibas, Dragomir Anguelov, et al. Nerdi: Single-view nerf synthesis with language-guided diffusion as general image priors. In Proceedings of the IEEE/CVF Conference on Computer Vision and Pattern Recognition, pages 20637–20647, 2023.
- [17] Prafulla Dhariwal and Alexander Nichol. Diffusion models beat gans on image synthesis. Advances in neural information processing systems, 34:8780–8794, 2021.
- [18] Changxing Ding and Dacheng Tao. Pose-invariant face recognition with homography-based normalization. Pattern Recognition, 66:144–152, 2017.
- [19] Hao Ding, Songsong Wu, Hao Tang, Fei Wu, Guangwei Gao, and Xiao-Yuan Jing. Cross-view image synthesis with deformable convolution and attention mechanism. In Pattern Recognition and Computer Vision: Third Chinese Conference, PRCV 2020, Nanjing, China, October 16–18, 2020, Proceedings, Part I 3, pages 386–397. Springer, 2020.
- [20] Huggingface docs. Clip directional similarity. In url: https://huggingface.co/docs/diffusers/conceptual/evaluation.
- [21] René Emmaneel, Martin R Oswald, Sjoerd de Haan, and Dragos Datcu. Cross-view outdoor localization in augmented reality by fusing map and satellite data. Applied Sciences, 13(20):11215, 2023.
- [22] Dave Epstein, Allan Jabri, Ben Poole, Alexei Efros, and Aleksander Holynski. Diffusion self-guidance for controllable image generation. Advances in Neural Information Processing Systems, 36, 2024.
- [23] Rafail Fridman, Amit Abecasis, Yoni Kasten, and Tali Dekel. Scenescape: Text-driven consistent scene generation. Advances in Neural Information Processing Systems, 36, 2024.
- [24] Rinon Gal, Yuval Alaluf, Yuval Atzmon, Or Patashnik, Amit H Bermano, Gal Chechik, and Daniel Cohen-Or. An image is worth one word: Personalizing text-to-image generation using textual inversion. arXiv preprint arXiv:2208.01618, 2022.
- [25] Jiatao Gu, Alex Trevithick, Kai-En Lin, Joshua M Susskind, Christian Theobalt, Lingjie Liu, and Ravi Ramamoorthi. Nerfdiff: Single-image view synthesis with nerf-guided distillation from 3d-aware diffusion. In International Conference on Machine Learning, pages 11808–11826. PMLR, 2023.
- [26] Jiaqi Gu, Bojian Wu, Lubin Fan, Jianqiang Huang, Shen Cao, Zhiyu Xiang, and Xian-Sheng Hua. Homography loss for monocular 3d object detection. In Proceedings of the IEEE/CVF Conference on Computer Vision and Pattern Recognition, pages 1080–1089, 2022.
- [27] Ligong Han, Yinxiao Li, Han Zhang, Peyman Milanfar, Dimitris Metaxas, and Feng Yang. Svdiff: Compact parameter space for diffusion fine-tuning. arXiv preprint arXiv:2303.11305, 2023.
- [28] Jonathan Ho, Ajay Jain, and Pieter Abbeel. Denoising diffusion probabilistic models. Advances in neural information processing systems, 33:6840–6851, 2020.
- [29] Jonathan Ho and Tim Salimans. Classifier-free diffusion guidance. arXiv preprint arXiv:2207.12598, 2022.
- [30] Edward J Hu, Yelong Shen, Phillip Wallis, Zeyuan Allen-Zhu, Yuanzhi Li, Shean Wang, Lu Wang, and Weizhu Chen. Lora: Low-rank adaptation of large language models. arXiv preprint arXiv:2106.09685, 2021.
- [31] Sixing Hu, Mengdan Feng, Rang MH Nguyen, and Gim Hee Lee. Cvm-net: Cross-view matching network for image-based ground-to-aerial geo-localization. In Proceedings of the IEEE Conference on Computer Vision and Pattern Recognition, pages 7258–7267, 2018.
- [32] Aapo Hyvärinen and Erkki Oja. Independent component analysis: algorithms and applications. Neural networks, 13(4-5):411–430, 2000.
- [33] Ajay Jain, Matthew Tancik, and Pieter Abbeel. Putting nerf on a diet: Semantically consistent few-shot view synthesis. In Proceedings of the IEEE/CVF International Conference on Computer Vision, pages 5885–5894, 2021.
- [34] Bahjat Kawar, Shiran Zada, Oran Lang, Omer Tov, Huiwen Chang, Tali Dekel, Inbar Mosseri, and Michal Irani. Imagic: Text-based real image editing with diffusion models. In Proceedings of the IEEE/CVF Conference on Computer Vision and Pattern Recognition, pages 6007–6017, 2023.
- [35] Stefan Klein, Marius Staring, and Josien PW Pluim. Evaluation of optimization methods for nonrigid medical image registration using mutual information and b-splines. IEEE transactions on image processing, 16(12):2879–2890, 2007.
- [36] Divya Kothandaraman, Tianrui Guan, Xijun Wang, Shuowen Hu, Ming Lin, and Dinesh Manocha. Far: Fourier aerial video recognition. In European Conference on Computer Vision, pages 657–676. Springer, 2022.
- [37] Divya Kothandaraman, Ming Lin, and Dinesh Manocha. Diffar: Differentiable frequency-based disentanglement for aerial video action recognition. In 2023 IEEE International Conference on Robotics and Automation (ICRA), pages 8254–8261. IEEE, 2023.
- [38] Divya Kothandaraman, Tianyi Zhou, Ming Lin, and Dinesh Manocha. Aerial diffusion: Text guided ground-to-aerial view translation from a single image using diffusion models. arXiv preprint arXiv:2303.11444, 2023.
- [39] Nupur Kumari, Bingliang Zhang, Richard Zhang, Eli Shechtman, and Jun-Yan Zhu. Multi-concept customization of text-to-image diffusion. In Proceedings of the IEEE/CVF Conference on Computer Vision and Pattern Recognition, pages 1931–1941, 2023.
- [40] Junnan Li, Dongxu Li, Silvio Savarese, and Steven Hoi. Blip-2: Bootstrapping language-image pre-training with frozen image encoders and large language models. arXiv preprint arXiv:2301.12597, 2023.
- [41] Tianjiao Li, Jun Liu, Wei Zhang, Yun Ni, Wenqian Wang, and Zhiheng Li. Uav-human: A large benchmark for human behavior understanding with unmanned aerial vehicles. In Proceedings of the IEEE/CVF conference on computer vision and pattern recognition, pages 16266–16275, 2021.
- [42] Chen-Hsuan Lin, Jun Gao, Luming Tang, Towaki Takikawa, Xiaohui Zeng, Xun Huang, Karsten Kreis, Sanja Fidler, Ming-Yu Liu, and Tsung-Yi Lin. Magic3d: High-resolution text-to-3d content creation. In Proceedings of the IEEE/CVF Conference on Computer Vision and Pattern Recognition, pages 300–309, 2023.
- [43] Gaowen Liu, Hugo Latapie, Ozkan Kilic, and Adam Lawrence. Parallel generative adversarial network for third-person to first-person image generation. In Proceedings of the IEEE/CVF Conference on Computer Vision and Pattern Recognition, pages 1917–1923, 2022.
- [44] Gaowen Liu, Hao Tang, Hugo Latapie, and Yan Yan. Exocentric to egocentric image generation via parallel generative adversarial network. In ICASSP 2020-2020 IEEE International Conference on Acoustics, Speech and Signal Processing (ICASSP), pages 1843–1847. IEEE, 2020.
- [45] Gaowen Liu, Hao Tang, Hugo M Latapie, Jason J Corso, and Yan Yan. Cross-view exocentric to egocentric video synthesis. In Proceedings of the 29th ACM International Conference on Multimedia, pages 974–982, 2021.
- [46] Jiazhen Liu and Xirong Li. Geometrized transformer for self-supervised homography estimation. In Proceedings of the IEEE/CVF International Conference on Computer Vision, pages 9556–9565, 2023.
- [47] Minghua Liu, Chao Xu, Haian Jin, Linghao Chen, Zexiang Xu, Hao Su, et al. One-2-3-45: Any single image to 3d mesh in 45 seconds without per-shape optimization. arXiv preprint arXiv:2306.16928, 2023.
- [48] Ruoshi Liu, Rundi Wu, Basile Van Hoorick, Pavel Tokmakov, Sergey Zakharov, and Carl Vondrick. Zero-1-to-3: Zero-shot one image to 3d object. In Proceedings of the IEEE/CVF International Conference on Computer Vision, pages 9298–9309, 2023.
- [49] Yuexin Ma, Tai Wang, Xuyang Bai, Huitong Yang, Yuenan Hou, Yaming Wang, Yu Qiao, Ruigang Yang, Dinesh Manocha, and Xinge Zhu. Vision-centric bev perception: A survey. arXiv preprint arXiv:2208.02797, 2022.
- [50] Frederik Maes, Andre Collignon, Dirk Vandermeulen, Guy Marchal, and Paul Suetens. Multimodality image registration by maximization of mutual information. IEEE transactions on Medical Imaging, 16(2):187–198, 1997.
- [51] Nithin Gopalakrishnan Nair, Anoop Cherian, Suhas Lohit, Ye Wang, Toshiaki Koike-Akino, Vishal M Patel, and Tim K Marks. Steered diffusion: A generalized framework for plug-and-play conditional image synthesis. In Proceedings of the IEEE/CVF International Conference on Computer Vision, pages 20850–20860, 2023.
- [52] Maxime Oquab, Timothée Darcet, Théo Moutakanni, Huy Vo, Marc Szafraniec, Vasil Khalidov, Pierre Fernandez, Daniel Haziza, Francisco Massa, Alaaeldin El-Nouby, et al. Dinov2: Learning robust visual features without supervision. arXiv preprint arXiv:2304.07193, 2023.
- [53] Eunbyung Park, Jimei Yang, Ersin Yumer, Duygu Ceylan, and Alexander C Berg. Transformation-grounded image generation network for novel 3d view synthesis. In Proceedings of the ieee conference on computer vision and pattern recognition, pages 3500–3509, 2017.
- [54] Ed Pizzi, Sreya Dutta Roy, Sugosh Nagavara Ravindra, Priya Goyal, and Matthijs Douze. A self-supervised descriptor for image copy detection. In Proceedings of the IEEE/CVF Conference on Computer Vision and Pattern Recognition, pages 14532–14542, 2022.
- [55] Dustin Podell, Zion English, Kyle Lacey, Andreas Blattmann, Tim Dockhorn, Jonas Müller, Joe Penna, and Robin Rombach. Sdxl: Improving latent diffusion models for high-resolution image synthesis. arXiv preprint arXiv:2307.01952, 2023.
- [56] Ben Poole, Ajay Jain, Jonathan T Barron, and Ben Mildenhall. Dreamfusion: Text-to-3d using 2d diffusion. arXiv preprint arXiv:2209.14988, 2022.
- [57] Guocheng Qian, Jinjie Mai, Abdullah Hamdi, Jian Ren, Aliaksandr Siarohin, Bing Li, Hsin-Ying Lee, Ivan Skorokhodov, Peter Wonka, Sergey Tulyakov, et al. Magic123: One image to high-quality 3d object generation using both 2d and 3d diffusion priors. arXiv preprint arXiv:2306.17843, 2023.
- [58] Alec Radford, Jong Wook Kim, Chris Hallacy, Aditya Ramesh, Gabriel Goh, Sandhini Agarwal, Girish Sastry, Amanda Askell, Pamela Mishkin, Jack Clark, et al. Learning transferable visual models from natural language supervision. In International conference on machine learning, pages 8748–8763. PMLR, 2021.
- [59] Amit Raj, Srinivas Kaza, Ben Poole, Michael Niemeyer, Nataniel Ruiz, Ben Mildenhall, Shiran Zada, Kfir Aberman, Michael Rubinstein, Jonathan Barron, et al. Dreambooth3d: Subject-driven text-to-3d generation. arXiv preprint arXiv:2303.13508, 2023.
- [60] Krishna Regmi and Ali Borji. Cross-view image synthesis using geometry-guided conditional gans. Computer Vision and Image Understanding, 187:102788, 2019.
- [61] Bin Ren, Hao Tang, and Nicu Sebe. Cascaded cross mlp-mixer gans for cross-view image translation. arXiv preprint arXiv:2110.10183, 2021.
- [62] Bin Ren, Hao Tang, Yiming Wang, Xia Li, Wei Wang, and Nicu Sebe. Pi-trans: Parallel-convmlp and implicit-transformation based gan for cross-view image translation. arXiv preprint arXiv:2207.04242, 2022.
- [63] Chris Rockwell, David F Fouhey, and Justin Johnson. Pixelsynth: Generating a 3d-consistent experience from a single image. In Proceedings of the IEEE/CVF International Conference on Computer Vision, pages 14104–14113, 2021.
- [64] Robin Rombach, Andreas Blattmann, Dominik Lorenz, Patrick Esser, and Björn Ommer. High-resolution image synthesis with latent diffusion models. In Proceedings of the IEEE/CVF conference on computer vision and pattern recognition, pages 10684–10695, 2022.
- [65] Nataniel Ruiz, Yuanzhen Li, Varun Jampani, Yael Pritch, Michael Rubinstein, and Kfir Aberman. Dreambooth: Fine tuning text-to-image diffusion models for subject-driven generation. In Proceedings of the IEEE/CVF Conference on Computer Vision and Pattern Recognition, pages 22500–22510, 2023.
- [66] Nataniel Ruiz, Yuanzhen Li, Varun Jampani, Wei Wei, Tingbo Hou, Yael Pritch, Neal Wadhwa, Michael Rubinstein, and Kfir Aberman. Hyperdreambooth: Hypernetworks for fast personalization of text-to-image models. arXiv preprint arXiv:2307.06949, 2023.
- [67] Kyle Sargent, Zizhang Li, Tanmay Shah, Charles Herrmann, Hong-Xing Yu, Yunzhi Zhang, Eric Ryan Chan, Dmitry Lagun, Li Fei-Fei, Deqing Sun, et al. Zeronvs: Zero-shot 360-degree view synthesis from a single real image. arXiv preprint arXiv:2310.17994, 2023.
- [68] Christoph Schuhmann, Romain Beaumont, Richard Vencu, Cade Gordon, Ross Wightman, Mehdi Cherti, Theo Coombes, Aarush Katta, Clayton Mullis, Mitchell Wortsman, et al. Laion-5b: An open large-scale dataset for training next generation image-text models. Advances in Neural Information Processing Systems, 35:25278–25294, 2022.
- [69] Yan Shen, Meng Luo, Yun Chen, Xiaotao Shao, Zhongli Wang, Xiaoli Hao, and Ya-Li Hou. Cross-view image translation based on local and global information guidance. IEEE Access, 9:12955–12967, 2021.
- [70] Ruoxi Shi, Hansheng Chen, Zhuoyang Zhang, Minghua Liu, Chao Xu, Xinyue Wei, Linghao Chen, Chong Zeng, and Hao Su. Zero123++: a single image to consistent multi-view diffusion base model. arXiv preprint arXiv:2310.15110, 2023.
- [71] Yujiao Shi, Dylan Campbell, Xin Yu, and Hongdong Li. Geometry-guided street-view panorama synthesis from satellite imagery. IEEE Transactions on Pattern Analysis and Machine Intelligence, 44(12):10009–10022, 2022.
- [72] Yukai Shi, Jianan Wang, He Cao, Boshi Tang, Xianbiao Qi, Tianyu Yang, Yukun Huang, Shilong Liu, Lei Zhang, and Heung-Yeung Shum. Toss: High-quality text-guided novel view synthesis from a single image. arXiv preprint arXiv:2310.10644, 2023.
- [73] Yichun Shi, Peng Wang, Jianglong Ye, Mai Long, Kejie Li, and Xiao Yang. Mvdream: Multi-view diffusion for 3d generation. arXiv preprint arXiv:2308.16512, 2023.
- [74] Richard Szeliski. Computer vision: algorithms and applications. Springer Nature, 2022.
- [75] Matthew Tancik, Vincent Casser, Xinchen Yan, Sabeek Pradhan, Ben Mildenhall, Pratul P Srinivasan, Jonathan T Barron, and Henrik Kretzschmar. Block-nerf: Scalable large scene neural view synthesis. In Proceedings of the IEEE/CVF Conference on Computer Vision and Pattern Recognition, pages 8248–8258, 2022.
- [76] Hao Tang, Dan Xu, Nicu Sebe, Yanzhi Wang, Jason J Corso, and Yan Yan. Multi-channel attention selection gan with cascaded semantic guidance for cross-view image translation. In Proceedings of the IEEE/CVF conference on computer vision and pattern recognition, pages 2417–2426, 2019.
- [77] Joy A Thomas. Elements of information theory, 1991.
- [78] Aysim Toker, Qunjie Zhou, Maxim Maximov, and Laura Leal-Taixé. Coming down to earth: Satellite-to-street view synthesis for geo-localization. In Proceedings of the IEEE/CVF Conference on Computer Vision and Pattern Recognition, pages 6488–6497, 2021.
- [79] Richard Tucker and Noah Snavely. Single-view view synthesis with multiplane images. In Proceedings of the IEEE/CVF Conference on Computer Vision and Pattern Recognition, pages 551–560, 2020.
- [80] Gijs van Tulder, Yao Tong, and Elena Marchiori. Multi-view analysis of unregistered medical images using cross-view transformers. In Medical Image Computing and Computer Assisted Intervention–MICCAI 2021: 24th International Conference, Strasbourg, France, September 27–October 1, 2021, Proceedings, Part III 24, pages 104–113. Springer, 2021.
- [81] Paul Viola and William M Wells III. Alignment by maximization of mutual information. International journal of computer vision, 24(2):137–154, 1997.
- [82] Dan Wang, Xinrui Cui, Xun Chen, Zhengxia Zou, Tianyang Shi, Septimiu Salcudean, Z Jane Wang, and Rabab Ward. Multi-view 3d reconstruction with transformers. In Proceedings of the IEEE/CVF International Conference on Computer Vision, pages 5722–5731, 2021.
- [83] Olivia Wiles, Georgia Gkioxari, Richard Szeliski, and Justin Johnson. Synsin: End-to-end view synthesis from a single image. In Proceedings of the IEEE/CVF Conference on Computer Vision and Pattern Recognition, pages 7467–7477, 2020.
- [84] Songsong Wu, Hao Tang, Xiao-Yuan Jing, Haifeng Zhao, Jianjun Qian, Nicu Sebe, and Yan Yan. Cross-view panorama image synthesis. IEEE Transactions on Multimedia, 2022.
- [85] Ruiqi Xian, Xijun Wang, Divya Kothandaraman, and Dinesh Manocha. Pmi sampler: Patch similarity guided frame selection for aerial action recognition. arXiv preprint arXiv:2304.06866, 2023.
- [86] Jiale Xu, Xintao Wang, Weihao Cheng, Yan-Pei Cao, Ying Shan, Xiaohu Qie, and Shenghua Gao. Dream3d: Zero-shot text-to-3d synthesis using 3d shape prior and text-to-image diffusion models. In Proceedings of the IEEE/CVF Conference on Computer Vision and Pattern Recognition, pages 20908–20918, 2023.
- [87] Binxin Yang, Shuyang Gu, Bo Zhang, Ting Zhang, Xuejin Chen, Xiaoyan Sun, Dong Chen, and Fang Wen. Paint by example: Exemplar-based image editing with diffusion models. In Proceedings of the IEEE/CVF Conference on Computer Vision and Pattern Recognition, pages 18381–18391, 2023.
- [88] Jirong Zhang, Chuan Wang, Shuaicheng Liu, Lanpeng Jia, Nianjin Ye, Jue Wang, Ji Zhou, and Jian Sun. Content-aware unsupervised deep homography estimation. In Computer Vision–ECCV 2020: 16th European Conference, Glasgow, UK, August 23–28, 2020, Proceedings, Part I 16, pages 653–669. Springer, 2020.
- [89] Lvmin Zhang, Anyi Rao, and Maneesh Agrawala. Adding conditional control to text-to-image diffusion models. In Proceedings of the IEEE/CVF International Conference on Computer Vision, pages 3836–3847, 2023.
- [90] Yun Zhao, Yu Zhang, Zhan Gong, and Hong Zhu. Scene representation in bird’s-eye view from surrounding cameras with transformers. In Proceedings of the IEEE/CVF Conference on Computer Vision and Pattern Recognition, pages 4511–4519, 2022.
- [91] Chuanxia Zheng and Andrea Vedaldi. Free3d: Consistent novel view synthesis without 3d representation. arXiv preprint arXiv:2312.04551, 2023.
- [92] Zhizhuo Zhou and Shubham Tulsiani. Sparsefusion: Distilling view-conditioned diffusion for 3d reconstruction. In Proceedings of the IEEE/CVF Conference on Computer Vision and Pattern Recognition, pages 12588–12597, 2023.