Graph Based Network with Contextualized Representations of Turns in Dialogue
Abstract
Dialogue-based relation extraction (RE) aims to extract relation(s) between two arguments that appear in a dialogue. Because dialogues have the characteristics of high personal pronoun occurrences and low information density, and since most relational facts in dialogues are not supported by any single sentence, dialogue-based relation extraction requires a comprehensive understanding of dialogue. In this paper, we propose the TUrn COntext awaRE Graph Convolutional Network (TUCORE-GCN) modeled by paying attention to the way people understand dialogues. In addition, we propose a novel approach which treats the task of emotion recognition in conversations (ERC) as a dialogue-based RE. Experiments on a dialogue-based RE dataset and three ERC datasets demonstrate that our model is very effective in various dialogue-based natural language understanding tasks. In these experiments, TUCORE-GCN outperforms the state-of-the-art models on most of the benchmark datasets. Our code is available at https://github.com/BlackNoodle/TUCORE-GCN.
1 Introduction
The task of relation extraction (RE) aims to identify semantic relations between arguments from a text, such as a sentence, a document, or even a dialogue. However, since a large number of relational facts are expressed in multiple sentences, sentence-level RE suffers from inevitable restrictions in practice (Yao et al., 2019). Therefore, cross-sentence RE, which aims to identify relations between two arguments that are not mentioned in the same sentence or relations that cannot be supported by any single sentence, is an essential step in building knowledge bases from large-scale corpora automatically (Ji et al., 2010; Swampillai and Stevenson, 2010; Surdeanu, 2013). In this respect, because dialogues readily exhibit cross-sentence relations (Yu et al., 2020), extracting relations from the dialogue is necessary.
To support the prediction of relation(s) between two arguments that appear within a dialogue, Yu et al. (2020) recently proposed DialogRE, which is a human-annotated dialogue-based RE dataset. Table 1 shows an example of DialogRE. In conversational texts such as DialogRE, because of its higher person pronoun frequency (Biber, 1988) and lower information density (Wang and Liu, 2011) compared to formal written texts, most relational triples require reasoning over multiple sentences in a dialogue. 65.9% of relational triples in DialogRE involve arguments that never appear in the same turn. Therefore, multi-turn information plays an important role in dialogue-based RE.
S1: | Hey Pheebs. |
S2: | Hey! |
S1: | Any sign of your brother? |
S2: | No, but he’s always late. |
S1: | I thought you only met him once? |
S2: | Yeah, I did. I think it sounds y’know big sistery, |
y’know, ‘Frank’s always late.’ | |
S1: | Well relax, he’ll be here. |
Subject: Frank | |
Object: S2 | |
relation: per:siblings | |
Subject: S2 | |
Object: Frank | |
relation: per:siblings | |
Subject: S2 | |
Object: Pheeb | |
relation:per:alternate_names | |
There are several major challenges in effective relation extraction from dialogue, inspired by the way how people understand dialogue in practice. First, the dialogue has speakers, and who speaks each utterance matters. The reason for it is because the subject and object of relational triples depend on who is speaking which utterance. For example, if S3 answered “Hey!” after “Hey Pheebs.”, the relational triple S2, per:alternate_names, Pheebs will be revised to S3, per:alternate_names, Pheebs, in the case of Table 1. Second, when understanding the meaning of each turn in a dialogue, it is important to know the meaning of the surrounding turns. For example, if we look at “No, but he is always late.” in Table 1, we don’t know who’s always late. However, if we look at the previous turn, we can see that S2’s brother is always late. Third, the dialogue consists of several turns. Those turns are sequential, and the arguments may appear in different turns. Consequently, it is important to grasp the multi-turn information in order to capture the relations between the two arguments. This could be done using the sequential characteristics of dialogues. Therefore, we aim to tackle these challenges to better extract relations from dialogues.
In this paper, we propose the TUrn COntext awaRE Graph Convolutional Network (TUCORE-GCN) for dialogue-based RE. It is designed to tackle the aforementioned challenges. TUCORE-GCN encodes the input sequence to reflect speaker information in dialogue by applying BERTs (Yu et al., 2020) and speaker embedding of SA-BERT (Gu et al., 2020). Then, to better extract the representations of each turn from the encoded input sequence, Masked Multi-Head Self-Attention (Vaswani et al., 2017) is applied using a surrounding turn mask. Next, TUCORE-GCN constructs a heterogeneous dialogue graph to capture the relational information between arguments in the dialogue. It consists of four types of nodes, namely dialogue node, turn node, subject node, object node, and three different types of edges, i.e., speaker edge, dialogue edge, and argument edge. Then, the sequential characteristics of the turn nodes should be considered. To obtain a surrounding turn-aware representation for each node, we apply bidirectional LSTM (BiLSTM) (Schuster and Paliwal, 1997) to the turn nodes and a Graph Convolutional Network (Kipf and Welling, 2017) to the heterogeneous dialogue graph. Finally, we classify the relations between arguments with the obtained features.
The task of emotion recognition in conversations (ERC) aims to identify the emotion of utterances in dialogue. ERC is a challenging task that has recently gained popularity due to its potential applications (Poria et al., 2019). It can be used to analyze user behaviors (Lee and Hong, 2016) and detect fake news (Guo et al., 2019). Table 2 shows an example from EmoryNLP (Zahiri and Choi, 2018), a dataset widely used in the ERC task. We propose a novel approach to treat the ERC task as a dialogue-based RE. If we define the emotion relation of each utterance when the subject says the object with a particular emotion (e.g., joyful, neutral, scared), the emotion of each utterance in the dialogue can be seen as a triple (speaker of utterance, emotion, utterance) as shown in Table 3. To the best of our knowledge, this approach was not introduced in previous studies.
Speaker | Utterance | Emotion |
Monica | He is so cute. So, where did | Joyful |
you guys grow up? | ||
Angela | Brooklyn Heights. | Neutral |
Bob | Cleveland. | Neutral |
Monica | How, how did that happen? | Neutral |
Joey | Oh my god. | Scared |
Monica | What? | Neutral |
Joey | I suddenly had the feeling that | Scared |
I was falling. But I’m not. |
S1: | He is so cute. So, where did you guys grow up? |
S2: | Brooklyn Heights. |
S3: | Cleveland. |
S1: | How, how did that happen? |
S4: | Oh my god. |
S1: | What? |
S4: | I suddenly had the feeling that I was falling. But |
I’m not. | |
Subject: S1 | |
Object: He is so cute. So, where did you guys grow up? | |
relation: Joyful | |
Subject: S2 | |
Object: Brooklyn Heights. | |
relation: Neutral | |
Subject: S3 | |
Object: Cleveland. | |
relation: Neutral | |
In summary, our main contributions are as follows:
-
•
We propose a novel method, TUrn COntext awaRE Graph Convolutional Network (TUCORE-GCN), to better cope with a dialogue-based RE task.
-
•
We introduce a surrounding turn mask to better capture the representation of the turns.
-
•
We introduce a heterogeneous dialogue graph to model the interaction among elements (e.g., speakers, turns, arguments) across the dialogue and propose a GCN mechanism combined with BiLSTM.
-
•
We propose a novel approach to treat the ERC task as a dialogue-based RE.
2 Related Work
2.1 Dialogue-Based Relation Extraction
Relation extraction has been studied extensively over the past few years and many approaches have achieved remarkable success. Most previous approaches focused on sentence-level RE (Zeng et al., 2014; Wang et al., 2016; Zhang et al., 2017; Zhu et al., 2019), but recently cross-sentence RE has been studied more because a large number of relational facts are expressed in multiple sentences in practice.
Recent work begins to explore cross-sentence relation extraction on documents that are formal genres, such as professionally written and edited news reports or well-edited websites. In document-level RE, various approaches including transformer-based methods (Tang et al., 2020; Ye et al., 2020; Wang et al., 2019) and graph-based methods (Christopoulou et al., 2019; Nan et al., 2020; Zeng et al., 2020) have been proposed. Among these, graph-based methods are widely adopted in document-level RE due to their effectiveness and strength in representing complicated syntactic and semantic relations among structured language data. Unlike previous work, we focused on extracting relations from dialogues, which are texts with high pronoun frequencies and low information density.
(Yu et al., 2020; Xue et al., 2021) were among the early works on dialogue-based RE. Yu et al. (2020) introduced several dialogue-based RE approaches with the DialogRE dataset. Among the various approaches, BERTs, a model that uses BERT (Devlin et al., 2019), shows good performance. BERTs is a model that slightly modified the original input sequence of BERT in consideration of speaker information. However, it has a limitation in that it cannot predict asymmetric inverse relations well. Our model basically follows the input sequence of BERTs, but we designed it to overcome this limitation to some extent. More detailed explanation is in Sec 4.1.5. Xue et al. (2021) proposed a graph-based approach, GDPNet, that constructs a latent multi-view graph to capture various possible relationships among tokens and refines this graph to select important words for relation prediction. In this approach, the refined graph and the BERT-based sequence representations are concatenated for relation extraction. The graph of GDPNet is a multi-view directed graph aiming to model all possible relationships between tokens. Unlike GDPNet, we combine tokens into meaningful units to form nodes and connect the nodes with speaker edges, dialogue edges, and argument edges to model what each edge means. In addition, GPDNet focuses on refining this multi-view graph to capture important words from long texts for RE, but we extract the relations using the features of the nodes in the graph.
2.2 Emotion Recognition in Conversation
Emotion recognition in conversation has emerged as an important problem in recent years and many successful approaches have been proposed. In ERC, numerous approaches including recurrence-based methods (Majumder et al., 2019; Ghosal et al., 2020) and graph-based methods (Ghosal et al., 2019; Ishiwatari et al., 2020) have been proposed. For instance, DialogueRNN (Majumder et al., 2019) uses an attention mechanism to grasp the relevant utterance from the whole conversation and models the party state, global state, and emotional dynamics with several RNNs. COSMIC (Ghosal et al., 2020) adopts a network structure, which is similar to DialogueRNN but adds external common sense knowledge to improve performance. DialogueGCN (Ghosal et al., 2019) treats each dialogue as a graph where each node represents utterance and is connected to the surrounding utterances. RGAT (Ishiwatari et al., 2020) is based on DialogueGCN. It adds relational positional encodings that can capture speaker dependency, along with sequential information. Many studies with remarkable success have been proposed, but none can be used in ERC as well as other dialogue-based tasks like our approaches.
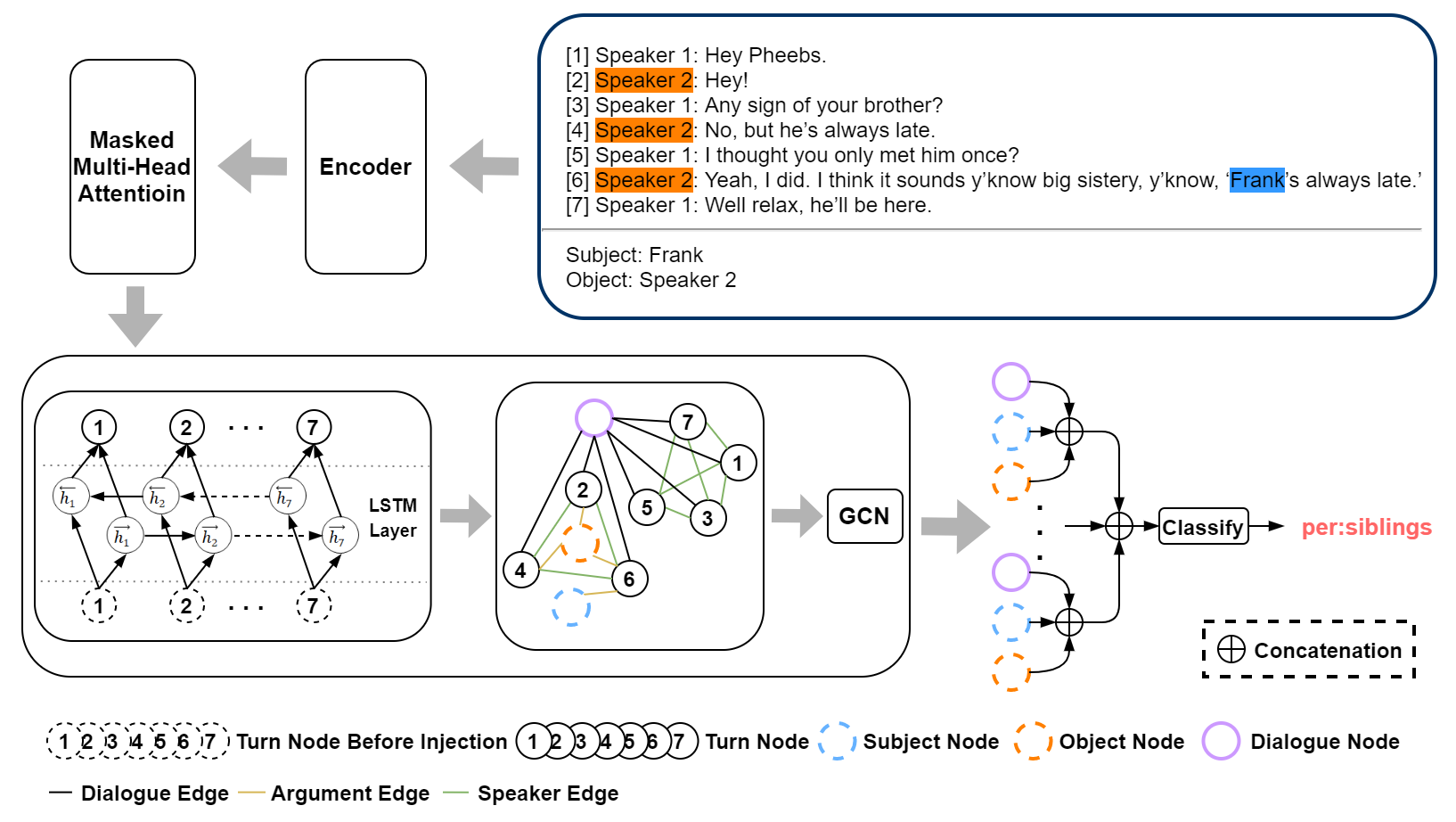
3 Model
TUCORE-GCN mainly consist of four modules: encoding module (Sec 3.1), turn attention module (Sec 3.2), dialogue graph with sequential nodes module (Sec 3.3), and classification module (Sec 3.4), as shown in Figure 1.
3.1 Encoding Module
We follow BERTs (Yu et al., 2020) as the input sequence of the encoding module. Given a dialogue and its associated argument pair , where and denote the speaker ID and text of the turn, respectively, and is the total number of turns, BERTs constructs , where is:
(1) |
where and are special tokens. In addition, it defines to be if , and otherwise. Then, we concatenate and with a classification token [CLS] and a separator token [SEP] in BERT(Devlin et al., 2019) as the input sequence [CLS][SEP][SEP][SEP].
To model the speaker change information, following SA-BERT(Gu et al., 2020), we add additional speaker embeddings to the token representations. is added to each token representation of , is added to each token representation of if , and is added to all token representations without speaker embedding added, where denotes the speaker embedding layer. is an embedding output for token representations without speaker information. A visual architecture of our input representation is illustrated in Appendix.
3.2 Turn Attention Module
To obtain the turn context-sensitive representation for each turn, we apply Masked Multi-Head Self-Attention (Vaswani et al., 2017) to the output of the encoder using the surrounding turn mask. The range of this surrounding turn is called the window, and the number of turns from the front and rear are viewed as the surrounding turn which is called the surround turn window size. The surround turn window size is a hyper-parameter.
Let be an output of the encoding module, where is the token representation in the output and N is the number of tokens. For token representations corresponding to range from to , denotes representations of the turn, denotes representations of a dialogue , and denotes the turn number in which is included (e.g., if ). We implement the surrounding turn mask as follows:
(2) |
where denotes . A visual architecture of an example regarding the surrounding turn mask is illustrated in Appendix.
Then, we reinforce the representation of each turn from representations of surrounding turns.
3.3 Dialogue Graph with Sequential Nodes Module
To model the dialogue-level information, interactions between turns and arguments, and interactions between turns, a heterogeneous dialogue graph is constructed.
We form four distinct types of nodes in the graph: dialogue node, turn node, subject node, and object node. The dialogue node is a node with the purpose of containing overall dialogue information. Turn nodes represent information about each turn in the dialogue and are created as many as the total number of turns in the dialogue. The subject node and object node represent the information of each argument. In our work, the initial representation of the dialogue node uses a feature corresponding to [CLS] in the output of the turn attention module. The initial representation of the turn node, subject node, and object node use the average of the token representations corresponding to , , and in the output of the turn attention module, respectively.
There are three different types of edge:
-
•
dialogue edge: All turn nodes are connected to the dialogue node with the dialogue edge so that the dialogue node learns while being aware of turn-level information.
-
•
argument edge: To model the interaction between turns and arguments, the turn node and argument nodes (i.e., subject node and object node) are connected with the argument edge if the argument is mentioned in .
-
•
speaker edge: To model the interaction among different turns of the same speaker, turn nodes uttered by the same speaker are fully connected with speaker edges.
Next, we apply a Graph Convolutional Network (GCN) (Kipf and Welling, 2017) to aggregate each node feature from the features of the neighbors. At this time, in order to inject sequential information to the turn nodes, GCN is applied after the turn nodes pass through the bidirectional LSTM (Schuster and Paliwal, 1997) layers. Given node at the GCN layer, and denote the representation of the node before injecting sequential information and the representation of the node after injecting sequential information, respectively. can be defined as:
(3) |
(4) |
where represents an turn node and represents turn node feature injected sequential information in the dialogue by concatenating the hidden states of two directions. , , and is the dimension. Then, the graph convolution operation can be defined as:
(5) |
where are different types of edges, denotes neighbors for node connected in the type edge, , and .
3.4 Classification Module
We concatenate the dialogue node, subject node, and object node to classify the relation between arguments. Furthermore, to cover features of all different abstract levels from each layer of the GCN, we concatenate the hidden states of each GCN layer as follows:
(6) |
where is the number of GCN layers and , , and denote the dialogue node, subject node, and object node, respectively. For each relation type , we introduce a vector and obtain the probability of the existence of between arguments by . We use cross-entropy loss as the classification loss to train our model in an end-to-end way.
4 Experiments
In this section, we report our experimental results on two tasks, dialogue-based RE and ERC. We experiment with two versions of TUCORE-GCN, TUCORE-GCNBERT and TUCORE-GCNRoBERTa, respectively based on the uncased base model of BERT (Devlin et al., 2019) and the large model of RoBERTa (Liu et al., 2019). TUCORE-GCN is trained using Adam (Kingma and Ba, 2015) as an optimizer with weight decay 0.01. We run each experiment five times and report the average score along with the standard deviation () for each metric. The full details of our training settings are provided in the Appendix.
4.1 Dialogue Based Relation Extraction
4.1.1 Dataset
We evaluate our model on DialogRE (Yu et al., 2020), an updated English version with a few annotation errors fixed 111https://dataset.org/dialogre. DialogRE has 36 relation types, 1,788 dialogues, and 8,119 triples, not including no-relation argument pairs, in total. We follow the standard split of the dataset.
4.1.2 Metrics
For DialogRE, We calculate both the and (Yu et al., 2020) scores as the evaluation metrics. is an evaluation metric to supplement the standard . is computed by taking in the part of dialogue as input, instead of only considering the entire dialogue.
4.1.3 Baselines and State-of-the-Art
For a comprehensive performance evaluation, we compared our model with the models using the following baseline and state-of-the-art methods:
BERT (Devlin et al., 2019): The BERT baseline for dialog-based RE, initialized with pre-trained parameters of BERT-base. It is classified using a final hidden vector corresponding to the [CLS] token.
BERTs (Yu et al., 2020): A modification to the input sequence of the above BERT baseline. This modification prevents a model from overfitting to the training data and helps a model locate the start positions of relevant turns.
GDPNet (Xue et al., 2021): A state-of-the-art model for the DialogRE. GDPNet finds indicative words from long sequences by constructing a latent multi-view graph and refining the graph. It uses the same input format of BERTs and pre-trained parameters of BERT-base.
RoBERTas: A model that uses the pre-trained parameters of RoBERTa-large (Liu et al., 2019) instead of pre-trained parameters of the BERTs above.
Method | Dev | Test | ||
F1 () | F1c () | F1 () | F1c () | |
BERT | 59.4 (0.7) | 54.7 (0.8) | 57.9 (1.0) | 53.1 (0.7) |
BERTs | 62.2 (1.3) | 57.0 (1.0) | 59.5 (2.1) | 54.2 (1.4) |
GDPNet | 61.8 (1.4)* | 58.5 (1.4)* | 60.2 (1.0)* | 57.3 (1.2)* |
RoBERTas | 72.6 (1.7) | 65.1 (1.7) | 71.3 (1.6) | 63.7 (1.2) |
TUCORE-GCNBERT | 66.8 (0.7) | 61.0 (0.5) | 65.5 (0.4) | 60.2 (0.6) |
TUCORE-GCNRoBERTa | 74.3 (0.6) | 67.0 (0.6) | 73.1 (0.4) | 65.9 (0.6) |
4.1.4 Results
We show the performance of TUCORE-GCN on the DialogRE dataset in Table 4 compared with other baselines.
Among the models using BERT, TUCORE-GCNBERT outperforms all baselines by 5.3 7.6 scores and 2.9 7.1 scores on the test set. GDPNet, the state-of-the-art model, achieved high-performance improvement at , but TUCORE-GCN showed high-performance improvement at both and . Among the models using RoBERTa, TUCORE-GCNRoBERTa yields a great improvement of / on the test set by 1.8/2.2, in comparison with the strong baseline RoBERTas. Our model can use BERT (or its variants) as an encoder, and in the experiment, we used both the BERT-base model and also the RoBERTa-large model. TUCORE-GCN show outstanding performance even when the BERT-base was used as the encoder. RoBERTa-large was also used, and it achieves state-of-the-art performance on DialogRE dataset with score 73.1 and score 65.9. It suggests that TUCORE-GCN is very effective in this dialog-based RE task.
4.1.5 Analysis on Inverse Relations
We analyze asymmetric inverse relations and symmetric inverse relations performance on the dialogue-based RE task. We divide the DialogRE dev set into three groups depending on whether it was asymmetric inverse relation, symmetric inverse relation, or other. Then, we report the score for each group in Appendix. In the dialogue-based RE task, when asymmetric inverse relations are predicted to exists, BERT makes more mistakes compared to symmetric inverse relations (Yu et al., 2020). Since BERT learns the tokenized representation of the input sequence through a Self-Attention mechanism, whether the arguments in the input sequence are a subject or an object is not learned in detail. As a result, the performance of asymmetric inverse relations that indicate different relations when subject and object are changed is significantly lower than in symmetric inverse relations that indicate the same relations even when subject and object are changed. However, TUCORE-GCN creates nodes for arguments separately, learns features of these nodes, and classifies relations. Thus, these issues with BERT can be improved. TUCORE-GCNBERT has improved performance in all groups compared to BERT and BERTs, especially for asymmetric inverse relations.
4.2 Emotion Recognition in Conversations
4.2.1 Dataset
We evaluate our model on three ERC benchmark datasets. We follow the standard split of the datasets and classify the emotion label of each utterance in the ERC benchmark datasets as the relation between the speaker and the utterance in the dialogue as in Table 3.
MELD (Poria et al., 2019)222https://affective-meld.github.io is a multimodal dataset collected from the TV show, Friends. We only used textual modality in this dataset. It has seven emotion labels, 2,458 dialogues, and 12,708 utterances. Each utterance is annotated with one of the seven emotion labels.
EmoryNLP (Zahiri and Choi, 2018)333https://github.com/emorynlp/emotion-detection is also collected from the TV show, Friends. It has seven emotion labels, 897 dialogues, and 12,606 utterances. Each utterance is annotated with one of the seven emotion labels.
DailyDialog (Li et al., 2017)444http://yanran.li/dailydialog.html reflects our daily communication way and covers various topics about our daily life. It has seven emotion labels, 13,118 dialogues, and 102,979 utterances. Each utterance is annotated with one of the seven emotion labels. Since it does not have speaker information, we consider the utterance turns as two anonymized speaker turns by default.
Method | MELD | EmoryNLP | DailyDialog |
CNN | 55.86 | 32.59 | 49.34 |
CNN+cLSTM | 56.87 | 32.89 | 50.24 |
DialogueRNN | 57.03 | 31.70 | 50.65 |
DialogueGCN | 58.10 | - | - |
RGAT | 60.91 | 34.42 | 54.31 |
RoBERTa | 62.02 | 37.29 | 55.16 |
RoBERTar | 64.19 | 38.03 | 61.65 |
COSMIC | 65.21 | 38.11 | 58.48 |
CESTa | 58.36 | - | 63.12 |
TUCORE-GCNBERT | 62.47 | 36.01 | 58.34 |
TUCORE-GCNRoBERTa | 65.36 | 39.24 | 61.91 |
4.2.2 Metrics
For DailyDialog, we calculate micro- except for the neutral class, because of its extremely high majority. For MELD and EmoryNLP, we calculate weighted-.
4.2.3 Baselines and State-of-the-Art
For a comprehensive performance evaluation, we compared our model with the models using the following baseline and state-of-the-art methods:
Previous methods: CNN (Kim, 2014), CNN+cLSTM (Poria et al., 2017), DialogueRNN (Majumder et al., 2019), DialogueGCN (Ghosal et al., 2019), and RoBERTa (Liu et al., 2019).
RGAT (Ishiwatari et al., 2020): A model that is provided with some information reflecting relation types through relational position encodings that can capture speaker dependency and sequential information.
RoBERTar: The RoBERTa baseline for ERC as our proposed approach, initialized with pre-trained parameters of RoBERTa-large (Liu et al., 2019). We set the input sequence of RoBERTa to [CLS]d[SEP]a1[SEP]a2[SEP] and feed them into RoBERTa for classification.
COSMIC (Ghosal et al., 2020): A state-of-the-art model for MELD and EmoryNLP. It uses RoBERTa-large as an encoder. It is a framework that models various aspects of commonsense knowledge by considering mental states, events, actions, and cause-effect relations for emotional recognition in conversation.
CESTa (Wang et al., 2020): A state-of-the-art model for DailyDialog that uses Conditional Random Field layer. The layer is used for sequential tagging, and it has an advantage in learning when there is an emotional consistency in conversation.
4.2.4 Results
We show the performance of TUCORE-GCN on the ERC datasets in Table 5, in comparison with other baselines. In addition, the performance of TUCORE-GCNBERT (()) on the development sets of MELD, EmoryNLP, and DailyDialog is 59.75 (0.5), 37.95 (0.8), 60.25 (0.4), respectively, and the performance of TUCORE-GCNRoBERTa is 65.94 (0.5), 40.17 (0.6), 62.83 (0.5), respectively. We have quoted the results for the baselines and state-of-the-art results reported in (Ishiwatari et al., 2020; Ghosal et al., 2020; Wang et al., 2020), except for the results of RoBERTar.
The only difference between RoBERTa and RoBERTar is the form of the input sequence, but RoBERTar is better at solving ERC task. Accordingly, we claim that treating the ERC as a dialogue-based RE is useful in practice. TUCORE-GCNRoBERTa outperforms COSMIC, the previous state-of-the-art model for MELD and EmoryNLP, by 0.15, 1.13, and 3.43 on test sets of MELD, EmoryNLP, and DailyDialog respectively. It shows state-of-art performance on both MELD and EmoryNLP. On the other hand, TUCORE-GCNRoBERTa shows slightly lower performance than CESTa on DailyDialog dataset. When utterances in a conversation are adjacent to one another, they tend to show similar emotions. This is called emotional consistency, and CRF layer of CESTa fits well with this tendency. Therefore, it has better performance on DailyDialog, which shows emotional consistency well. However, it shows very poor performance on MELD, where emotional consistency does not appear much (Wang et al., 2020). Considering these observations, our model generally shows outstanding performance on MELD, EmoryNLP, and also DailyDialog. It suggests that TUCORE-GCN is effective in ERC as well as dialogue-based RE.
Method | DialogRE | MELD | EmoryNLP | |
F1 () | F1c () | F1 () | F1 () | |
TUCORE-GCNRoBERTa | 73.1 (0.4) | 65.9 (0.6) | 65.36 (0.4) | 39.24 (0.6) |
w/o speaker embedding | 72.7 (1.0) | 65.8 (0.6) | 64.64 (0.9) | 37.59 (0.5) |
w/o turn attention | 72.0 (0.6) | 65.3 (0.3) | 64.59 (0.4) | 37.07 (1.1) |
w/o turn-level BiLSTM | 72.5 (0.4) | 65.7 (0.3) | 65.02 (0.4) | 38.35 (0.5) |
4.3 Ablation Study
We conduct ablation studies to evaluate the effectiveness of different modules and mechanisms in TUCORE-GCN. The results are shown in Table 6.
First, we removed the speaker embedding in the encoder module. To be specific, the encoder and input format of TUCORE-GCNRoBERTa are the same as RoBERTas. Without speaker embedding, the performance of TUCORE-GCNRoBERTa drops by 0.4 score and 0.1 score on the DialogRE test set and 0.72 and 1.65 scores on the MELD and EmoryNLP test set, respectively. This drop shows that when encoding a dialogue, a better representation can be obtained through speaker change information.
Next, we removed the turn attention module. To be specific, the output of the encoding module is delivered to the dialogue graph with sequential nodes module. Without turn attention, the performance of TUCORE-GCNRoBERTa sharply drops by 1.1 score and 0.6 score on DialogRE test set and 0.77 and 2.17 scores on the MELD and EmoryNLP test set, respectively. This drop shows that the turn attention module helps capture the representation of the turns and, therefore, improves dialogue-based RE and ERC performance.
Finally, we removed the turn-level BiLSTM for turn nodes in the dialogue graph with sequential nodes module. To be specific, in the module, we apply GCN without injecting sequential information of the turn nodes. Without turn-level BiLSTM, the performance of TUCORE-GCNRoBERTa drops by 0.6 score and 0.2 score on DialogRE test set and 0.34 and 0.89 scores on MELD and EmoryNLP test set, respectively. This means that reflecting the characteristics of the sequential nodes when learning the graph helps to learn the features of each node and, therefore, improves dialogue-based RE and ERC performance.
5 Conclusion and Future Work
In this paper, we propose TUCORE-GCN for dialogue-based RE. TUCORE-GCN is designed according to the way people understand dialogues in practice to better cope with dialogue-based RE. In addition, we propose a way to treat the ERC task as dialogue-based RE and showed its effectiveness through experiments. Experimental results on a dialogue-based RE dataset and three ERC task datasets demonstrate that TUCORE-GCN model significantly outperforms existing models and yields the new state-of-the-art results on both tasks.
Since TUCORE-GCN is modeled for the dialogue text type, we expect it to perform well in dialogue-based natural language understanding tasks. In future work, we are going to explore the effectiveness of it on other dialogue-based tasks.
Acknowledgements
We would like to thank Jinwoo Jeong, Tae Hee Jo, Sangwoo Seo, and the anonymous reviewers for their thoughtful and constructive comments. This work was supported by Institute of Information & communications Technology Planning & Evaluation(IITP) grant funded by the Korea government(MSIT) (No. 2020-0-01373, Artificial Intelligence Graduate School Program (Hanyang University)) and the National Research Foundation of Korea(NRF) grant funded by the Korea government(*MSIT) (No.2018R1A5A7059549). *Ministry of Science and ICT.
References
- Biber (1988) Douglas Biber. 1988. Variation across Speech and Writing. Cambridge University Press, Cambridge.
- Christopoulou et al. (2019) Fenia Christopoulou, Makoto Miwa, and Sophia Ananiadou. 2019. Connecting the dots: Document-level neural relation extraction with edge-oriented graphs. In Proceedings of the 2019 Conference on Empirical Methods in Natural Language Processing and the 9th International Joint Conference on Natural Language Processing (EMNLP-IJCNLP), pages 4925–4936, Hong Kong, China. Association for Computational Linguistics.
- Conneau and Lample (2019) Alexis Conneau and Guillaume Lample. 2019. Cross-lingual language model pretraining. In Advances in Neural Information Processing Systems 32: Annual Conference on Neural Information Processing Systems 2019, NeurIPS 2019, December 8-14, 2019, Vancouver, BC, Canada, pages 7057–7067.
- Devlin et al. (2019) Jacob Devlin, Ming-Wei Chang, Kenton Lee, and Kristina Toutanova. 2019. BERT: Pre-training of deep bidirectional transformers for language understanding. In Proceedings of the 2019 Conference of the North American Chapter of the Association for Computational Linguistics: Human Language Technologies, Volume 1 (Long and Short Papers), pages 4171–4186, Minneapolis, Minnesota. Association for Computational Linguistics.
- Ghosal et al. (2020) Deepanway Ghosal, Navonil Majumder, Alexander Gelbukh, Rada Mihalcea, and Soujanya Poria. 2020. COSMIC: COmmonSense knowledge for eMotion identification in conversations. In Findings of the Association for Computational Linguistics: EMNLP 2020, pages 2470–2481, Online. Association for Computational Linguistics.
- Ghosal et al. (2019) Deepanway Ghosal, Navonil Majumder, Soujanya Poria, Niyati Chhaya, and Alexander Gelbukh. 2019. DialogueGCN: A graph convolutional neural network for emotion recognition in conversation. In Proceedings of the 2019 Conference on Empirical Methods in Natural Language Processing and the 9th International Joint Conference on Natural Language Processing (EMNLP-IJCNLP), pages 154–164, Hong Kong, China. Association for Computational Linguistics.
- Gu et al. (2020) Jia-Chen Gu, Tianda Li, Quan Liu, Zhen-Hua Ling, Zhiming Su, Si Wei, and Xiaodan Zhu. 2020. Speaker-aware BERT for multi-turn response selection in retrieval-based chatbots. In CIKM ’20: The 29th ACM International Conference on Information and Knowledge Management, Virtual Event, Ireland, October 19-23, 2020, pages 2041–2044. ACM.
- Guo et al. (2019) Chuan Guo, Juan Cao, Xueyao Zhang, Kai Shu, and Huan Liu. 2019. Dean: Learning dual emotion for fake news detection on social media. ArXiv preprint, abs/1903.01728.
- Ishiwatari et al. (2020) Taichi Ishiwatari, Yuki Yasuda, Taro Miyazaki, and Jun Goto. 2020. Relation-aware graph attention networks with relational position encodings for emotion recognition in conversations. In Proceedings of the 2020 Conference on Empirical Methods in Natural Language Processing (EMNLP), pages 7360–7370, Online. Association for Computational Linguistics.
- Ji et al. (2010) Heng Ji, Ralph Grishman, Hoa Trang Dang, Kira Griffitt, and Joe Ellis. 2010. Overview of the tac 2010 knowledge base population track. In In Third Text Analysis Conference.
- Kim (2014) Yoon Kim. 2014. Convolutional neural networks for sentence classification. In Proceedings of the 2014 Conference on Empirical Methods in Natural Language Processing (EMNLP), pages 1746–1751, Doha, Qatar. Association for Computational Linguistics.
- Kingma and Ba (2015) Diederik P. Kingma and Jimmy Ba. 2015. Adam: A method for stochastic optimization. In 3rd International Conference on Learning Representations, ICLR 2015, San Diego, CA, USA, May 7-9, 2015, Conference Track Proceedings.
- Kipf and Welling (2017) Thomas N. Kipf and Max Welling. 2017. Semi-supervised classification with graph convolutional networks. In 5th International Conference on Learning Representations, ICLR 2017, Toulon, France, April 24-26, 2017, Conference Track Proceedings. OpenReview.net.
- Lan et al. (2020) Zhenzhong Lan, Mingda Chen, Sebastian Goodman, Kevin Gimpel, Piyush Sharma, and Radu Soricut. 2020. ALBERT: A lite BERT for self-supervised learning of language representations. In 8th International Conference on Learning Representations, ICLR 2020, Addis Ababa, Ethiopia, April 26-30, 2020. OpenReview.net.
- Lee and Hong (2016) Jieun Lee and Ilyoo B. Hong. 2016. Predicting positive user responses to social media advertising: The roles of emotional appeal, informativeness, and creativity. International Journal of Information Management, 36(3):360–373.
- Li et al. (2017) Yanran Li, Hui Su, Xiaoyu Shen, Wenjie Li, Ziqiang Cao, and Shuzi Niu. 2017. DailyDialog: A manually labelled multi-turn dialogue dataset. In Proceedings of the Eighth International Joint Conference on Natural Language Processing (Volume 1: Long Papers), pages 986–995, Taipei, Taiwan. Asian Federation of Natural Language Processing.
- Liu et al. (2019) Yinhan Liu, Myle Ott, Naman Goyal, Jingfei Du, Mandar Joshi, Danqi Chen, Omer Levy, Mike Lewis, Luke Zettlemoyer, and Veselin Stoyanov. 2019. Roberta: A robustly optimized bert pretraining approach. ArXiv preprint, abs/1907.11692.
- Majumder et al. (2019) Navonil Majumder, Soujanya Poria, Devamanyu Hazarika, Rada Mihalcea, Alexander F. Gelbukh, and Erik Cambria. 2019. Dialoguernn: An attentive RNN for emotion detection in conversations. In The Thirty-Third AAAI Conference on Artificial Intelligence, AAAI 2019, The Thirty-First Innovative Applications of Artificial Intelligence Conference, IAAI 2019, The Ninth AAAI Symposium on Educational Advances in Artificial Intelligence, EAAI 2019, Honolulu, Hawaii, USA, January 27 - February 1, 2019, pages 6818–6825. AAAI Press.
- Nan et al. (2020) Guoshun Nan, Zhijiang Guo, Ivan Sekulic, and Wei Lu. 2020. Reasoning with latent structure refinement for document-level relation extraction. In Proceedings of the 58th Annual Meeting of the Association for Computational Linguistics, pages 1546–1557, Online. Association for Computational Linguistics.
- Poria et al. (2017) Soujanya Poria, Erik Cambria, Devamanyu Hazarika, Navonil Majumder, Amir Zadeh, and Louis-Philippe Morency. 2017. Context-dependent sentiment analysis in user-generated videos. In Proceedings of the 55th Annual Meeting of the Association for Computational Linguistics (Volume 1: Long Papers), pages 873–883, Vancouver, Canada. Association for Computational Linguistics.
- Poria et al. (2019) Soujanya Poria, Devamanyu Hazarika, Navonil Majumder, Gautam Naik, Erik Cambria, and Rada Mihalcea. 2019. MELD: A multimodal multi-party dataset for emotion recognition in conversations. In Proceedings of the 57th Annual Meeting of the Association for Computational Linguistics, pages 527–536, Florence, Italy. Association for Computational Linguistics.
- Schuster and Paliwal (1997) M. Schuster and K. K. Paliwal. 1997. Bidirectional recurrent neural networks. IEEE Transactions on Signal Processing, 45(11):2673–2681.
- Surdeanu (2013) M. Surdeanu. 2013. Overview of the tac2013 knowledge base population evaluation: English slot filling and temporal slot filling. Theory and Applications of Categories.
- Swampillai and Stevenson (2010) Kumutha Swampillai and Mark Stevenson. 2010. Inter-sentential relations in information extraction corpora. In Proceedings of the Seventh International Conference on Language Resources and Evaluation (LREC’10), Valletta, Malta. European Language Resources Association (ELRA).
- Tang et al. (2020) Hengzhu Tang, Yanan Cao, Zhenyu Zhang, Jiangxia Cao, Fang Fang, Shi Wang, and Pengfei Yin. 2020. HIN: hierarchical inference network for document-level relation extraction. In Advances in Knowledge Discovery and Data Mining - 24th Pacific-Asia Conference, PAKDD 2020, Singapore, May 11-14, 2020, Proceedings, Part I, volume 12084 of Lecture Notes in Computer Science, pages 197–209. Springer.
- Vaswani et al. (2017) Ashish Vaswani, Noam Shazeer, Niki Parmar, Jakob Uszkoreit, Llion Jones, Aidan N. Gomez, Lukasz Kaiser, and Illia Polosukhin. 2017. Attention is all you need. In Advances in Neural Information Processing Systems 30: Annual Conference on Neural Information Processing Systems 2017, December 4-9, 2017, Long Beach, CA, USA, pages 5998–6008.
- Wang and Liu (2011) Dong Wang and Yang Liu. 2011. A pilot study of opinion summarization in conversations. In Proceedings of the 49th Annual Meeting of the Association for Computational Linguistics: Human Language Technologies, pages 331–339, Portland, Oregon, USA. Association for Computational Linguistics.
- Wang et al. (2019) Hong Wang, Christfried Focke, Rob Sylvester, Nilesh Mishra, and William Yang Wang. 2019. Fine-tune bert for docred with two-step process. CoRR, abs/1909.11898.
- Wang et al. (2016) Linlin Wang, Zhu Cao, Gerard de Melo, and Zhiyuan Liu. 2016. Relation classification via multi-level attention CNNs. In Proceedings of the 54th Annual Meeting of the Association for Computational Linguistics (Volume 1: Long Papers), pages 1298–1307, Berlin, Germany. Association for Computational Linguistics.
- Wang et al. (2020) Yan Wang, Jiayu Zhang, Jun Ma, Shaojun Wang, and Jing Xiao. 2020. Contextualized emotion recognition in conversation as sequence tagging. In Proceedings of the 21th Annual Meeting of the Special Interest Group on Discourse and Dialogue, pages 186–195, 1st virtual meeting. Association for Computational Linguistics.
- Xue et al. (2021) Fuzhao Xue, Aixin Sun, Hao Zhang, and Eng Siong Chng. 2021. Gdpnet: Refining latent multi-view graph for relation extraction. In Thirty-Fifth AAAI Conference on Artificial Intelligence, AAAI 2021, Thirty-Third Conference on Innovative Applications of Artificial Intelligence, IAAI 2021, The Eleventh Symposium on Educational Advances in Artificial Intelligence, EAAI 2021, Virtual Event, February 2-9, 2021, pages 14194–14202. AAAI Press.
- Yao et al. (2019) Yuan Yao, Deming Ye, Peng Li, Xu Han, Yankai Lin, Zhenghao Liu, Zhiyuan Liu, Lixin Huang, Jie Zhou, and Maosong Sun. 2019. DocRED: A large-scale document-level relation extraction dataset. In Proceedings of the 57th Annual Meeting of the Association for Computational Linguistics, pages 764–777, Florence, Italy. Association for Computational Linguistics.
- Ye et al. (2020) Deming Ye, Yankai Lin, Jiaju Du, Zhenghao Liu, Peng Li, Maosong Sun, and Zhiyuan Liu. 2020. Coreferential Reasoning Learning for Language Representation. In Proceedings of the 2020 Conference on Empirical Methods in Natural Language Processing (EMNLP), pages 7170–7186, Online. Association for Computational Linguistics.
- Yu et al. (2020) Dian Yu, Kai Sun, Claire Cardie, and Dong Yu. 2020. Dialogue-based relation extraction. In Proceedings of the 58th Annual Meeting of the Association for Computational Linguistics, pages 4927–4940, Online. Association for Computational Linguistics.
- Zahiri and Choi (2018) Sayyed M. Zahiri and Jinho D. Choi. 2018. Emotion detection on TV show transcripts with sequence-based convolutional neural networks. In The Workshops of the The Thirty-Second AAAI Conference on Artificial Intelligence, New Orleans, Louisiana, USA, February 2-7, 2018, volume WS-18 of AAAI Workshops, pages 44–52. AAAI Press.
- Zeng et al. (2014) Daojian Zeng, Kang Liu, Siwei Lai, Guangyou Zhou, and Jun Zhao. 2014. Relation classification via convolutional deep neural network. In Proceedings of COLING 2014, the 25th International Conference on Computational Linguistics: Technical Papers, pages 2335–2344, Dublin, Ireland. Dublin City University and Association for Computational Linguistics.
- Zeng et al. (2020) Shuang Zeng, Runxin Xu, Baobao Chang, and Lei Li. 2020. Double graph based reasoning for document-level relation extraction. In Proceedings of the 2020 Conference on Empirical Methods in Natural Language Processing (EMNLP), pages 1630–1640, Online. Association for Computational Linguistics.
- Zhang et al. (2017) Yuhao Zhang, Victor Zhong, Danqi Chen, Gabor Angeli, and Christopher D. Manning. 2017. Position-aware attention and supervised data improve slot filling. In Proceedings of the 2017 Conference on Empirical Methods in Natural Language Processing, pages 35–45, Copenhagen, Denmark. Association for Computational Linguistics.
- Zhu et al. (2019) Hao Zhu, Yankai Lin, Zhiyuan Liu, Jie Fu, Tat-Seng Chua, and Maosong Sun. 2019. Graph neural networks with generated parameters for relation extraction. In Proceedings of the 57th Annual Meeting of the Association for Computational Linguistics, pages 1331–1339, Florence, Italy. Association for Computational Linguistics.
Appendix A Appendix
A.1 Input Representation
A visual architecture of our input representation is illustrated in Figure 2.
A.2 Surrounding Turn Mask
A visual architecture of our surrounding turn mask of Turn Attention Module is illustrated in Figure 3. 1 was given as an example for surround turn window size c .
A.3 Experimental Settings
A.3.1 Hyperparameter Settings
We truncate a dialogue when the input sequence length exceeds 512 and use the development set to manually tune the optimal hyperparameters for TUCORE-GCN, based on the score. Hyperparameter settings for TUCORE-GCN on a dialogue-based RE dataset are listed in Table 7 and the ones on ERC datasets are listed in Table 8. The final values of hyperparameters we adopted are in bold. We do not tune all the hyperparameters.
A.3.2 Other Settings
TUCORE-GCNBERT is implemented by using PyTorch 1.6.0 with CUDA 10.1 and TUCORE-GCNRoBERTa is implemented by using PyTorch 1.7.0 with CUDA 11.0. Our implementation of TUCORE-GCNBERT uses the DGL555https://www.dgl.ai 0.4.3 and our implementation of TUCORE-GCNRoBERTa uses the DGL5 0.5.3. We used the official code666https://github.com/nlpdata/dialogre of (Yu et al., 2020) to calculate and scores on DialogRE, and scikit-learn777https://scikit-learn.org/stable to calculate score on ERC datasets. It takes about 2 hours, 1.25 hours, 1.5 hours, 12 hours to run TUCORE-GCNBERT on DialogRE, MELD, EmoryNLP, and DailyDialog once, respectively. Additionally, it takes about 4 hours, 2.2 hours, 2.3 hours, 20 hours to run TUCORE-GCNRoBERTa on DialogRE, MELD, EmoryNLP, and DailyDialog once, respectively. We conducted all experiments that uses TUCORE-GCNBERT on a Ubuntu server using Intel(R) Core(TM) i9-10900X CPU with 128GB of memory, and used GeForce RTX 2080 Ti GPU with 11GB of memory. We conducted all experiments that uses TUCORE-GCNRoBERTa on a Ubuntu server using Intel(R) Core(TM) i9-10980XE CPU with 128GB of memory, and used GeForce RTX 3090 GPU with 24GB of memory.
A.4 Experimental results on Inverse Relations
We show the performance of TUCORE-GCN on asymmetric inverse relation, symmetric inverse relation, and other of DialogRE (Yu et al., 2020) in Table 9 compared with other baselines.
Among the models using BERT (Devlin et al., 2019), TUCORE-GCNBERT has significantly reduced difference between the scores of asymmetric relation group and the symmetric relation group. The score difference between two groups were 5.8, which was the smallest score difference compared with the other models that use BERT. In addition, compared to RoBERTas’s score difference between asymmetric relation group and the symmetric relation group, TUCORE-GCNRoBERTa’s score difference was reduced by 2.9. This suggests that TUCORE-GCN solves the limitations of BERT and its variants’ inability to predict the inverse relation well.

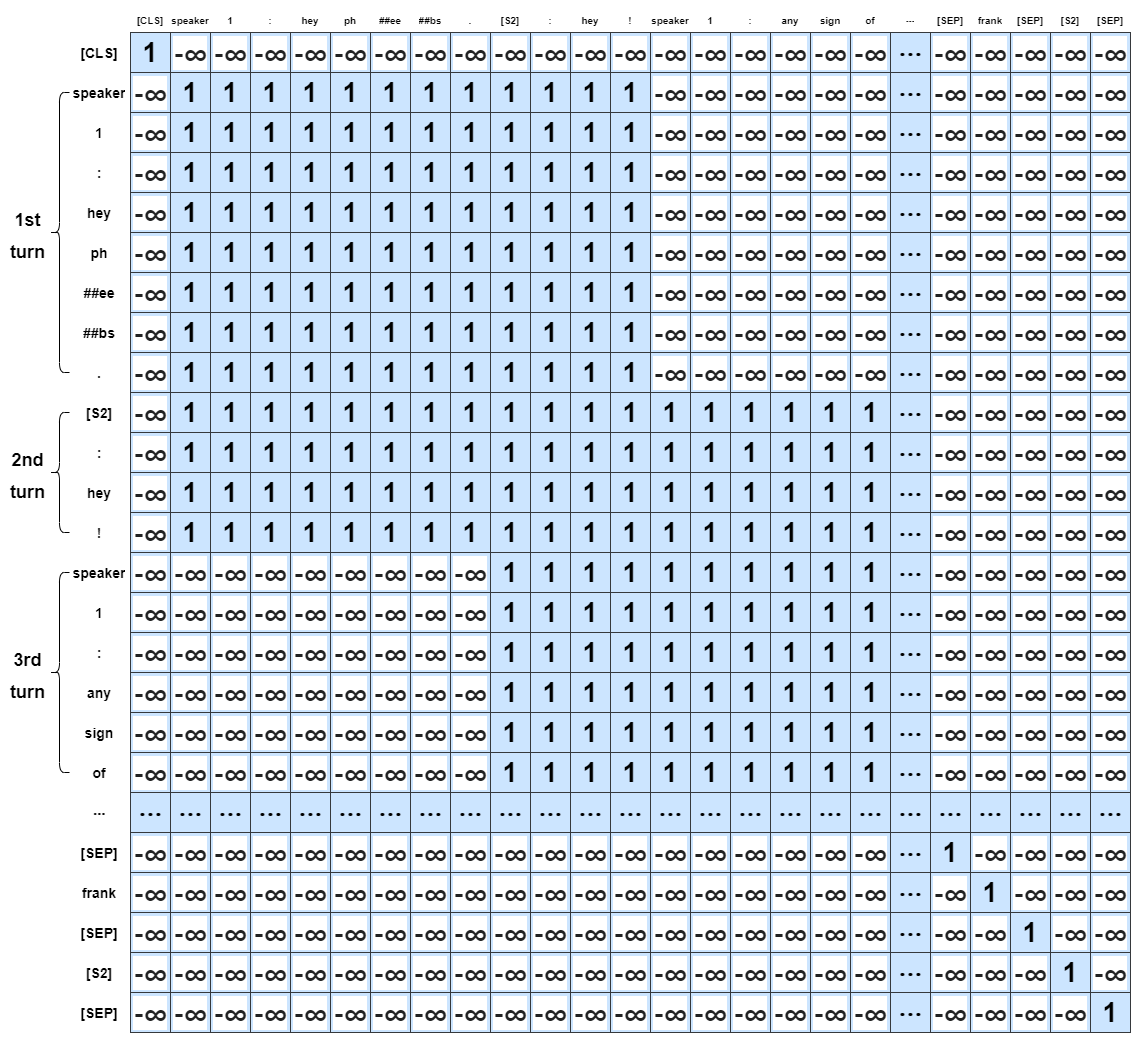
Hyperparameter | Value | |
BERT | RoBERTa | |
Epoch | 20 | 20, 30 |
Batch Size | 12 | 12 |
Learning Rate | 3e-5 | 3e-5, 1e-5, 5e-6, 1e-6 |
Speaker Embedding Size | 768 | 768 |
Layers of Turn Attention | 1 | 1 |
Heads of Turn Attention | 12 | 12 |
Surround Turn Window Size | 1, 2, 3 | 1, 2 |
Dropout of Turn Attention | 0.1 | 0.1 |
Layers of LSTM | 1, 2, 3 | 1, 2, 3 |
LSTM Hidden Size | 768 | 768 |
Dropout of LSTM | 0.2, 0.4, 0.6 | 0.2, 0.4 |
Layers of GCN | 2 | 2 |
GCN Hidden Size | 768 | 768 |
Dropout of GCN | 0.6 | 0.6 |
Numbers of Parameters | 156M | 401M |
Hyperparameter Search Trials | 12 | 12 |
Hyperparameter | Value | |
BERT | RoBERTa | |
Epoch | 10 | 10 |
Batch Size | 12 | 12 |
Learning Rate | 3e-5 | 3e-5, 1e-5, 5e-6, 1e-6 |
Speaker Embedding Size | 768 | 768 |
Layers of Turn Attention | 1 | 1 |
Heads of Turn Attention | 12 | 12 |
Surround Turn Window Size | 1 | 1, 2 |
Dropout of Turn Attention | 0.1 | 0.1 |
Layers of LSTM | 1, 2, 3 | 2 |
LSTM Hidden Size | 768 | 768 |
Dropout of LSTM | 0.2, 0.4 | 0.2 |
Layers of GCN | 2 | 2 |
GCN Hidden Size | 768 | 768 |
Dropout of GCN | 0.6 | 0.6 |
Numbers of Parameters | 156M | 401M |
Hyperparameter Search Trials | 6 | 6 |
Method | Asymmetric | Symmetric | Other |
BERT (Devlin et al., 2019) | 42.5 (3.2) | 60.7 (1.2) | 65.6 (0.8) |
BERTs (Yu et al., 2020) | 46.5 (3.3) | 61.5 (0.7) | 69.4 (0.3) |
GDPNet (Xue et al., 2021) | 47.4 (1.9) | 59.8 (2.5) | 68.1 (0.8) |
RoBERTas | 57.4 (3.2) | 69.3 (2.1) | 79.6 (1.3) |
TUCORE-GCNBERT | 57.9 (1.9) | 63.7 (1.9) | 72.2 (0.6) |
TUCORE-GCNRoBERTa | 62.3 (3.1) | 71.3 (0.8) | 79.9 (0.4) |