Fluctuation-response theorem for Kullback-Leibler divergences to quantify causation
Abstract
We define a new measure of causation from a fluctuation-response theorem for Kullback-Leibler divergences, based on the information-theoretic cost of perturbations. This information response has both the invariance properties required for an information-theoretic measure and the physical interpretation of a propagation of perturbations. In linear systems, the information response reduces to the transfer entropy, providing a connection between Fisher and mutual information.
In the general framework of stochastic dynamical systems, the term causation refers to the influence that a variable exerts over the dynamics of another variable . Measures of causation find application in neuroscience [1], climate studies [2], cancer research [3], and finance [4]. However, a widely accepted quantitative definition of causation is still missing.
Causation manifests itself in two inseparable forms: information flow [5, 6, 7, 8], and propagation of perturbations [9, 10, 11, 12]. Ideally, a quantitative measure of causation should connect both perspectives.
Information flow is commonly quantified by the transfer entropy [13, 14, 15, 16, 17], that is the average conditional mutual information corresponding to the uncertainty reduction in forecasting the time evolution of that is achieved upon knowledge of . The mutual information is a special case of Kullback-Leibler (KL) divergence, a dimensionless measure of distinguishability between probability distributions [18]. As such, the transfer entropy abstracts from the underlying physics to give an invariant description in terms of the strength of probabilistic dependencies.
From the interventional point of view [9, 10, 11, 12], causation is identified with how a perturbation applied to propagates in the system to effect . Although a direct perturbation of observables is unfeasible in most real-world situations, the fluctuation-response theorem establishes a connection between the response to a small perturbation and the correlation of fluctuations in the natural (unperturbed) dynamics [19, 20, 21, 22].
The fluctuation-response theorem considers the first-order expansion of the response with respect to the perturbation. The corresponding linear response coefficient has been suggested as a measure of causation [12, 11]. However, it has the same physical units as , and it can assume negative values; thus, is not directly related to any information-theoretic measure.
In stochastic dynamical systems with nonlinear interactions, perturbing may not only affect the evolution of the expectation value of , but it may also affect the evolution of the variance of , and in fact its entire probability distribution. The KL divergence from the natural to the perturbed probability densities has recently been identified as the universal upper bound to the physical response of any observable relative to its natural fluctuations [23].
In this Letter, we define a new measure of causation in the form of a linear response coefficient between KL divergences, which we would like to call information response. In particular, we consider the ratio of two KL divergences, one for the response and one for the perturbation, where the latter represents an information-theoretic cost of the perturbation. For small perturbations, we formulate a fluctuation-response theorem that expresses this ratio as a ratio of Fisher information.
In linear systems, this new information response reduces to the transfer entropy, which provides a connection between Fisher and mutual information, and thus a connection between fluctuation-response theory and information flows.
Kullback-Leibler (KL) divergence.
Consider two probability distributions and of a random variable . The KL divergence from to is defined as
(1) |
it is not symmetric in its arguments, and non-negative. Importantly, it is invariant under invertible transformations [18], namely .
The problem of causation.
Consider a stochastic system of variables evolving with ergodic Markovian dynamics. Our goal is to define a quantitative measure of causation, i.e., the influence that a variable exerts over the dynamics of another variable . We want this definition to have both the invariance property of KL divergences, and the physical interpretation of a propagation of perturbations.
Since the dynamics is ergodic, and therefore stationary, it suffices to consider the stochastic variables , at , and a time interval later . To avoid cluttered notation, we will implicitly assume that the current values of the remaining variables are absorbed into , e.g., . Conditioning on avoids confounding variables in to introduce spurious causal links between and [24].
Local response divergence.
Let us consider the system at with steady-state distribution . We make an ideal measurement of its actual state . Immediately after the measurement, we perturb the state by introducing a small displacement of the variable , namely . If the effect of this perturbation propagates to , then it is reflected in the KL divergence from the natural to the perturbed prediction
(2) |
which is a function of the local condition and the perturbation strength . We name it local response divergence, and denote its ensemble average by .
The concept of causation, interpreted in the framework of fluctuation-response theory, is only meaningful with respect to an arrow of time. That means to postulate that the perturbation cannot have effects at past times
(3) |
In writing the conditional probability , we implicitly assumed , meaning that the condition provoked by the perturbation is possible under the natural statistics. This implies that the response statistics can be predicted without actually perturbing the system, which is the main idea of fluctuation-response theory [19, 20, 21, 22].
Information-theoretic cost.
The mean local response divergence , like any response function in fluctuation-response theory, is defined in relation to a perturbation, irrespective of how difficult it may be to perform this perturbation. Intuitively, we expect that it takes more effort to perturb those variables that fluctuate less. Therefore, we consider the KL divergence from the natural to the perturbed ensemble of conditions
(4) |
to quantify the information-theoretic cost of perturbations, and call it perturbation divergence.
For example, for an underdamped Brownian particle, the perturbation divergence is equivalent to the average thermodynamic work required to perform an perturbation of its velocity, up to a factor being the temperature, see Supplementary Information (SI). For an equilibrium ensemble in a potential , with Boltzmann distribution , the perturbation divergence is the average reversible work . Note that the definition of Eq. (4) is general, and can be applied to more abstract models where thermodynamic quantities are not clearly identified.
Information response.
We introduce the information response as the ratio between mean local response divergence and perturbation divergence, in the limit of a small perturbation
(5) |
We can interpret as an information-theoretic linear response coefficient. This information response is our measure of causation with respect to the timescale , see Fig. 1. The time arrow requirement (Eq. (3)) implies for .
Introducing the local information response , we can equivalently write .
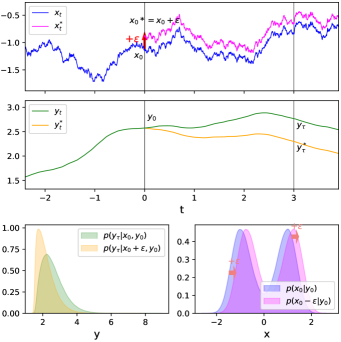
Fisher information.
The one-parameter family of probability densities parametrized by (for fixed ) can be equipped with a Riemannian metric having as squared line element. In fact, the leading order term in the Taylor expansion of a KL divergence between probabilities that differ only by a small perturbation of a parameter is of second order, with coefficients known as Fisher information [18, 25]. Explicitly, expanding the mean response divergence for , we obtain
(6) |
where we used the interventional causality requirement (Eq. (3)), and probability normalization. Similarly, for the perturbation divergence we have
(7) |
Applying the Fisher information representation to the information response, we get for
(8) |
that is the fluctuation-response theorem for KL divergences. For generalizations and a discussion of the connection with the classical fluctuation-response theorem see [26] and SI text. Eq. (8) is the ratio of two second derivatives over the same physical variable , and it can be regarded as an application of L’Hôpital’s rule to Eq. (5).
In general, Fisher information is not easily connected to Shannon entropy and mutual information [27]. Below, we show that for linear stochastic systems, the information response, which is a ratio of Fisher information (Eq. (8)), is equivalent to the transfer entropy, a conditional form of mutual information.
Transfer entropy.
The most widely used measure of information flow is the conditional mutual information
(9) |
which is generally called transfer entropy [13, 14, 15, 16, 17]. It is the average KL divergence from conditional independence of and given .
The transfer entropy is used in nonequilibrium thermodynamics of measurement-feedback systems, where it is related to work extraction and dissipation through fluctuation theorems [28, 16, 29]; in data science, causal network reconstruction from time series is based on statistical significance tests for the presence of transfer entropy [24].
If uncertainty is measured by the Shannon entropy , then the transfer entropy quantifies how much, on average, the uncertainty in predicting from decreases if we additionally get to know , .
While the joint probability contains all the physics of the interacting dynamics of and , the description in terms of the scalar transfer entropy represents a form of coarse-graining.
We introduce the local transfer entropy ; thus for the (macroscopic) transfer entropy .
We next show that and are intimately related for linear systems.
Linear stochastic dynamics.
As example of application, we study the information response in Ornstein-Uhlenbeck (OU) processes [30], i.e., linear stochastic systems of the type
(10) |
where is Gaussian white noise with symmetric and constant covariance matrix. For the system to be stationary, we require the eigenvalues of the interaction matrix to have positive real part. For our setting, we identify and for some particular , and as the remaining variables. Here, probability densities are normal distributions, , with mean and variance , and similarly for and . Expectations depend linearly on the conditions, , and variances are independent of them, . Recall the implicit conditioning on the confounding variables through .
Applying these Gaussian properties to Eq. (8), the information response becomes:
(11) |
where can be interpreted as the coefficient of in the linear regression for based on the predictors , and as its error variance. The variance quantifies the strength of the natural fluctuations of (variable to be perturbed) conditional on (other variables). In fact, the information-theoretic cost of the perturbation, , is higher if and are more correlated.
In linear systems, the transfer entropy is equivalent to Granger causality [31]
(12) |
as can be seen by substituting the Gaussian expressions for and into Eq. (9).
The decrease in uncertainty in adding the predictor to the linear regression of based on reads
(13) |
see SI text. Comparing Eq. (11) with Eq. (12) and using Eq. (13), we obtain a non-trivial equivalence between information response and transfer entropy for OU processes,
(14) |
Remarkably, despite the equivalence of the macroscopic quantities and , the corresponding local quantities are markedly different, see Fig. 2.
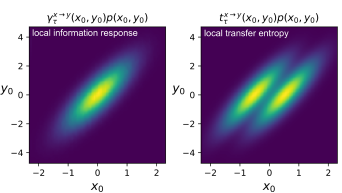
In Fig. 2, we show the local response divergence and local transfer entropy for the hierarchical OU process of two variables
(15) |
with , and parameters , , , . This is possibly the simplest model of nonequilibrium stationary interacting dynamics with continuous variables [32]. However, the pattern of Fig. 2 is qualitatively the same for any linear OU process. In fact, the perturbation shifts the prediction by the same amount on the axis, , independently of the condition , without affecting the variance . Hence, is constant in space, and the local contribution only reflects the density , here a bivariate Gaussian. On the contrary, the KL divergence corresponding to the change of the prediction into given by the knowledge of , is strongly dependent on . In fact, the local transfer entropy reads
(16) |
see SI text. In particular, for likely values , the divergence is smaller compared to the unlikely situations and . Thus, when multiplied by the steady-state density , attains a bimodal shape.
Nonlinear example.
As a counter-example for the general validity of Eq. (14) for nonlinear systems, consider the following nonlinear Langevin equation for two variables
(17) |
Numerical simulations (same parameters as for Eq. (15)) show that Eq. (14) is violated, see SI for details. Hence, in general, the transfer entropy is not easily connected to the information response.
Ensemble information response.
Similar to the above, we can define an analogous information response at the ensemble level. From the same perturbation , we consider the unconditional response divergence
(18) |
i.e., we evaluate the response at the ensemble level, without knowledge of the measurement ,
(19) |
In general .
We define the ensemble information response as
(20) |
where the second line, valid only for , is the corresponding fluctuation-response theorem. A straightforward generalization to arbitrary perturbation profiles is discussed in SI text. Note that we could write through the Fisher information , but the partial derivative would be over the perturbation parameter , and we found it more natural to consider the self-prediction quantity . See SI text for technical details on expectation brakets.
In linear systems, the ensemble information response takes the form
(21) |
where is the mutual information between and , and is the mutual information that the two predictors together have on the output , see SI text.
From the nonnegativity of informations, we obtain the bound . We see that increases with the transfer entropy , and decreases with the autocorrelation . Since diverges for in continuous processes, the perturbation on the ensemble takes a finite time to fully propagate its effect to the ensemble. Since time-lagged informations vanish for in ergodic processes, ensembles relax asymptotically towards steady-state after a perturbation, and correspondingly the ensemble information response vanishes. This provides a trade-off shape for as a function of the timescale . Note the asymptotics for , also resulting from ergodicity.
Discussion.
In this Letter, we introduced a new measure of causation that has both the invariance properties required for an information-theoretic measure and the physical interpretation of a propagation of perturbations. It has the form of a linear response coefficient between Kullback-Leibler divergences, and it is based on the information-theoretic cost of perturbations. We would like to call it information response.
We study the behavior of the information response analytically in linear stochastic systems, and show that it reduces to the known transfer entropy in this case. This establishes a first connection between fluctuation-response theory and information flow, i.e., the two main perspectives to the problem of causation at present. Additionally, it provides a new relation between Fisher and mutual information.
We suggest our information response for the design of new quantitative causal inference methods [24]. Its practical estimation on time series, as it is normally the case for information-theoretic measures, depends on the learnability of probability distributions from a finite amount of data [33, 34].
Acknowledgments
Acknowledgements.
We thank M Scazzocchio for helpful discussions. AA is supported by the DFG through FR3429/3 to BMF; AA, and BMF are supported through the Excellence Initiative by the German Federal and State Governments (Cluster of Excellence PoL EXC-2068).References
- Seth et al. [2015] A. K. Seth, A. B. Barrett, and L. Barnett, Granger causality analysis in neuroscience and neuroimaging, Journal of Neuroscience 35, 3293 (2015).
- Runge et al. [2019] J. Runge, S. Bathiany, E. Bollt, G. Camps-Valls, D. Coumou, E. Deyle, C. Glymour, M. Kretschmer, M. D. Mahecha, J. Muñoz-Marí, et al., Inferring causation from time series in earth system sciences, Nature communications 10, 1 (2019).
- Luzzatto and Pandolfi [2015] L. Luzzatto and P. P. Pandolfi, Causality and chance in the development of cancer, N Engl J Med 373, 84 (2015).
- Kwon and Yang [2008] O. Kwon and J.-S. Yang, Information flow between stock indices, EPL (Europhysics Letters) 82, 68003 (2008).
- Ito and Sagawa [2013] S. Ito and T. Sagawa, Information thermodynamics on causal networks, Physical Review Letters 111, 180603 (2013).
- Horowitz and Esposito [2014] J. M. Horowitz and M. Esposito, Thermodynamics with continuous information flow, Physical Review X 4, 031015 (2014).
- James et al. [2016] R. G. James, N. Barnett, and J. P. Crutchfield, Information flows? a critique of transfer entropies, Physical Review Letters 116, 238701 (2016).
- Auconi et al. [2017] A. Auconi, A. Giansanti, and E. Klipp, Causal influence in linear langevin networks without feedback, Physical Review E 95, 042315 (2017).
- Pearl [2009] J. Pearl, Causality (Cambridge university press, 2009).
- Janzing et al. [2013] D. Janzing, D. Balduzzi, M. Grosse-Wentrup, B. Schölkopf, et al., Quantifying causal influences, The Annals of Statistics 41, 2324 (2013).
- Aurell and Del Ferraro [2016] E. Aurell and G. Del Ferraro, Causal analysis, correlation-response, and dynamic cavity, in Journal of Physics: Conference Series, Vol. 699 (2016) p. 012002.
- Baldovin et al. [2020] M. Baldovin, F. Cecconi, and A. Vulpiani, Understanding causation via correlations and linear response theory, Physical Review Research 2, 043436 (2020).
- Massey [1990] J. Massey, Causality, feedback and directed information, in Proc. Int. Symp. Inf. Theory Applic.(ISITA-90) (Citeseer, 1990) pp. 303–305.
- Schreiber [2000] T. Schreiber, Measuring information transfer, Physical Review Letters 85, 461 (2000).
- Ay and Polani [2008] N. Ay and D. Polani, Information flows in causal networks, Advances in complex systems 11, 17 (2008).
- Parrondo et al. [2015] J. M. Parrondo, J. M. Horowitz, and T. Sagawa, Thermodynamics of information, Nature physics 11, 131 (2015).
- Cover [1999] T. M. Cover, Elements of information theory (John Wiley & Sons, 1999).
- Amari [2016] S. I. Amari, Information geometry and its applications, Vol. 194 (Springer, 2016).
- Kubo [1966] R. Kubo, The fluctuation-dissipation theorem, Reports on progress in physics 29, 255 (1966).
- Kubo [1986] R. Kubo, Brownian motion and nonequilibrium statistical mechanics, Science 233, 330 (1986).
- Marconi et al. [2008] U. M. B. Marconi, A. Puglisi, L. Rondoni, and A. Vulpiani, Fluctuation–dissipation: response theory in statistical physics, Physics reports 461, 111 (2008).
- Maes [2020] C. Maes, Response theory: A trajectory-based approach, Frontiers in Physics 8, 229 (2020).
- Dechant and Sasa [2020] A. Dechant and S.-i. Sasa, Fluctuation–response inequality out of equilibrium, Proceedings of the National Academy of Sciences 117, 6430 (2020).
- Runge [2018] J. Runge, Causal network reconstruction from time series: From theoretical assumptions to practical estimation, Chaos: An Interdisciplinary Journal of Nonlinear Science 28, 075310 (2018).
- Ito and Dechant [2020] S. Ito and A. Dechant, Stochastic time evolution, information geometry, and the cramér-rao bound, Physical Review X 10, 021056 (2020).
- [26] Eq. (8) holds for a larger class of divergences beyond the KL divergence, because the Fisher information is the unique invariant metric [18].
- Wei and Stocker [2016] X.-X. Wei and A. A. Stocker, Mutual information, fisher information, and efficient coding, Neural computation 28, 305 (2016).
- Sagawa and Ueda [2012] T. Sagawa and M. Ueda, Nonequilibrium thermodynamics of feedback control, Physical Review E 85, 021104 (2012).
- Rosinberg and Horowitz [2016] M. L. Rosinberg and J. M. Horowitz, Continuous information flow fluctuations, EPL (Europhysics Letters) 116, 10007 (2016).
- Risken [1996] H. Risken, Fokker-planck equation, in The Fokker-Planck Equation (Springer, 1996) pp. 63–95.
- Barnett et al. [2009] L. Barnett, A. B. Barrett, and A. K. Seth, Granger causality and transfer entropy are equivalent for gaussian variables, Physical Review Letters 103, 238701 (2009).
- Auconi et al. [2019] A. Auconi, A. Giansanti, and E. Klipp, Information thermodynamics for time series of signal-response models, Entropy 21, 177 (2019).
- Bialek et al. [1996] W. Bialek, C. G. Callan, and S. P. Strong, Field theories for learning probability distributions, Physical Review Letters 77, 4693 (1996).
- Bialek et al. [2020] W. Bialek, S. E. Palmer, and D. J. Schwab, What makes it possible to learn probability distributions in the natural world?, arXiv preprint arXiv:2008.12279 (2020).