ERIT Lightweight Multimodal Dataset for Elderly Emotion Recognition and Multimodal Fusion Evaluation
Abstract
ERIT is a novel multimodal dataset designed to facilitate research in a lightweight multimodal fusion. It contains text and image data collected from videos of elderly individuals reacting to various situations, as well as seven emotion labels for each data sample. Because of the use of labeled images of elderly users reacting emotionally, it is also facilitating research on emotion recognition in an underrepresented age group in machine learning visual emotion recognition. The dataset is validated through comprehensive experiments indicating its importance in neural multimodal fusion research.
1 Introduction
Emotion recognition plays a crucial role in understanding human behavior and improving human-computer interaction. With the growing elderly population, there is an increasing need for effective emotion recognition systems tailored to the specific characteristics of this demographic. ERIT is a multimodal dataset that aims to address this need by providing a rich source of data for training and evaluating emotion recognition models for elderly individuals. This paper describes the motivation behind creating the ERIT dataset, the methodology used to obtain and validate the emotion labels, and the potential applications of the dataset in the field of elderly emotion recognition.
The primary motivation for creating ERIT dataset is the lightweight evaluation of multimodal fusion. The task chosen for research on multimodal fusion needs to be able to perform across different modalities, possibly with a limited amount of classes for an easy evaluation. Emotion recognition meets the demands of such a task, with only seven classes and the possibility of performing emotion recognition among different modalities, such as facial expresion Leong et al. (2023); Huang et al. (2023), pose Yang et al. (2020), text Acheampong et al. (2020); Nandwani and Verma (2021) or audio Zhao and Shu (2023); George and Muhamed Ilyas (2024) or non-contact physiological signals Li and Peng (2024).
The secondary motivation behind the creation of the ERIT dataset is to facilitate research in elderly emotion recognition and contribute to the development of more accurate and robust emotion recognition systems for this demographic. The dataset can be used for various applications, including healthcare, elderly care, and assistive technologies Sharma et al. (2021).
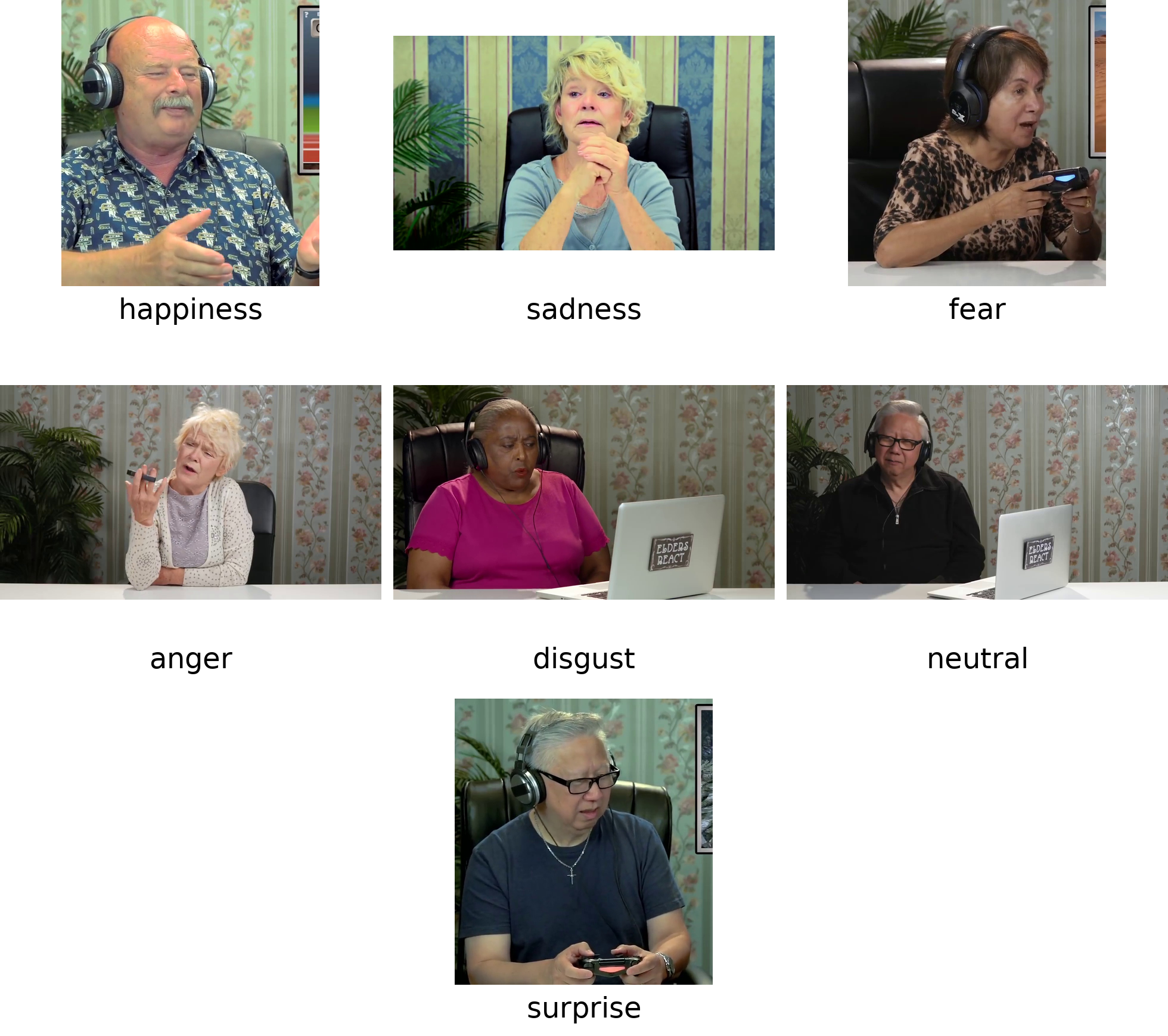
2 Methodology
The ERIT dataset contains lightweight text and image data collected from videos of elderly people reacting to various stimuli. The dataset includes transcriptions of the speech and emotion labels extracted from the video frames. The emotion labels are provided for seven basic emotions: anger, disgust, fear, happiness, sadness, surprise, and neutral. The dataset was built from frames extracted from the ElderReact video dataset by Ma et al. (2019). The reasons behind creating an image and text-based dataset were: lightweight processing of text and image, accuracy of evaluating fusion compared to frames randomly extracted from the video and filling the gap in emotion recognition among different age groups.
Text and image are better for lightweight fusion evaluation than computationally expensive audio or video processing and can be processed by most of the large multimodal models (LMMs) such as GPT4v OpenAI (2023b) and GPT4o OpenAI (2023a), multimodal versions of LLaMA Zhang et al. (2023), or Flamingo Alayrac et al. (2022) etc.
Due to the labeling of specific frames in the video, the prediction is more accurate than in cases of videos labeled and evaluated by sampling a few frames, the method used on evaluated MER-MULTI dataset by Lian et al. (2024). The authors admit that this method could potentially ignore key samples and decrease the performance.
Finally, the emotion recognition images try to fill the age gap in the emotion recognition community by creating of an openly public dataset for facial emotion recognition. While some of the elderly facial emotion recognition image datasets Ebner et al. (2010) (1068 images of older faces) and Minear and Park (2004) provide acted emotions, emotions of the elderly users in ERIT were their natural reaction to the presented material.
2.1 Data Collection and Verification
The data for the ERIT dataset was collected from videos of older people reacting to things associated with younger generations such as various video games, popular music and entertainment, slang, modern technology, etc. The videos were obtained from the YouTube video series called Elders React which has been on YouTube for over 10 years with 20M subscribers. We built upon the ElderReact dataset that was labeled for emotions by AmazonTurk respondents Ma et al. (2019). The dataset is split into separate training, validation, and testing sets to facilitate model development and evaluation. Each set contains a diverse range of emotional expressions and individual differences, ensuring a robust dataset for training and evaluating emotion recognition models (see: Fig 2).
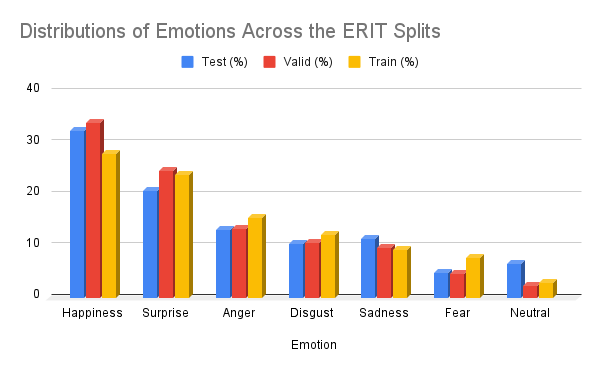
The audio from the videos was transcribed using automatic speech recognition (ASR), specifically Whisper Radford et al. (2023), which performs with WER similar to supervised models on LibriSpeech and outperforms the wav2vec2 model. We also tested Google Speech Recognition but did not annotate multiple samples, whereas Whisper returned all the videos annotated.
For the frame selection, we used labels from the ElderReact dataset as a ground truth. Since each video was labeled with one or more labels, we extracted as many frames as there were labels assigned to the video. Subsequently, we performed a search for each label in the video and selected the one with the highest emotion score by using the DeepFace framework, which is a state-of-the-art facial emotion recognition system. As a result of each video, we obtained appx. 2 different frames, with different labels but with the same transcription. That roughly doubled the amount of labels in the video dataset 1.
Test | Valid | Train | |
---|---|---|---|
ElderReact | 353 | 355 | 615 |
ERIT: | 576 | 643 | 1169 |
Emotion | Test | Valid | Train |
---|---|---|---|
Happiness | 187 | 219 | 327 |
Surprise | 120 | 159 | 280 |
Anger | 76 | 87 | 182 |
Disgust | 61 | 69 | 144 |
Sadness | 66 | 63 | 110 |
Fear | 28 | 30 | 91 |
Neutral | 38 | 16 | 35 |
To validate the emotion labels, original emotion labels were extracted from accompanying text files and compared with the labels obtained using the DeepFace framework. The original emotion labels were prioritized when inconsistencies were found between the original labels and the DeepFace-derived labels. This validation process ensures that the emotion labels in the dataset are accurate and reliable.
3 Experiments and Results
This analysis focuses on how different models perform across various data types, particularly using the ERIT dataset as a benchmark for evaluating multimodal fusion compared to single-mode text and image analyses. By assessing models’ abilities to integrate both textual and visual inputs effectively, the study underscores the importance of leveraging combined data sources for enhanced sentiment analysis and recognition tasks. Models that excel in multimodal fusion demonstrate a clear advantage in leveraging the complementary strengths of text and image inputs, showcasing their capability to deliver nuanced and accurate analyses across diverse datasets like ERIT.
Prompts used for evaluation of LMMs were similar for each case, and varied only in the type of modal information passed. The correct prompts were generated with the help of the GPT 3.5 prompt generator which largely improved the number of predictions compared to hand-engineered prompts.
GPT4v | GPT4o | LLaMA w Adapter | ||||
---|---|---|---|---|---|---|
Dev (↑) | Test (↑) | Dev (↑) | Test (↑) | Dev (↑) | Test (↑) | |
Image | 38.97 | 40.14 | 37.38 | 38.26 | 35.3 | 33.16 |
Text | 29.39 | 34.48 | 24.11 | 30.16 | 35.46 | 33.68 |
Fusion | 39.47 | 42.3 | 39.5 | 41.18 | 35.61 | 33.51 |
GPT_4_V | ||||||||||||||||||||||||||||
---|---|---|---|---|---|---|---|---|---|---|---|---|---|---|---|---|---|---|---|---|---|---|---|---|---|---|---|---|
|
|
|
|
|
|
|
||||||||||||||||||||||
Image | 38.97 | 40.14 | 58.68 | 63.35 | 76.13 | 51.17 | 46.23 | |||||||||||||||||||||
Text | 29.39 | 34.48 | 57.65 | 62.71 | 70.07 | 82.32 | 34.57 | |||||||||||||||||||||
Fusion | 39.47 | 42.3 | 61.25 | 66.82 | 81.24 | 80.43 | 65.39 |
,
Finally, we analyze the performance of LLMs on emotion recognition tasks using only textual data across various emotion datasets, including ERIT. Models such as GPT-4 and LLaMA-7B demonstrate competitive performance on datasets like EmoWOZ and DAIC-WOZ, indicating strong proficiency in text-based emotion recognition tasks. However, their effectiveness varies when applied to the ERIT dataset, which poses challenges due to its complex emotional expressions and different age groups that might necessitate the integration of multimodal inputs for more accurate analysis.
Model |
|
|
|
|
|
|
|
||||||||||||||||||||||
---|---|---|---|---|---|---|---|---|---|---|---|---|---|---|---|---|---|---|---|---|---|---|---|---|---|---|---|---|---|
GPT-2 | 25.8 | 19 | 24 | - | - | - | - | ||||||||||||||||||||||
LLaMA-7B | 41.1 | 35.6 | 0.3 | - | - | 35.46 | 33.68 | ||||||||||||||||||||||
Alpaca-7B | 48.8 | 40.5 | 44.6 | - | - | - | - | ||||||||||||||||||||||
GPT-3.5 | 42.2 | 37.9 | 40 | 54.5 | 64.3 | - | - | ||||||||||||||||||||||
GPT-4 | 42.4 | 37.5 | 62.3 | 63.6 | 59.3 | 29.39 | 34.48 | ||||||||||||||||||||||
|
77.6 | 73.3 | 83.9 | 88.6 | 70 | - | - |
4 Conclusion
The ERIT dataset is a valuable resource for researchers and practitioners working in the field of both multimodal fusion as well as elderly emotion recognition. The dataset provides a rich source of multimodal data, that is images paired with text and labeled with emotions, which can be used to research multimodal fusion performance. It can also be used for emotion recognition models specifically tailored to the elderly population. The comprehensive experiments on LMMs ensure the accuracy and reliability of the emotion labels and make the dataset applicable to a wide range of lightweight multimodal experiments.
5 Data Availability Statement
The datasets generated and analyzed during the current study are available in the Zenodo repository, DOI: 10.5281/zenodo.12803448. The data includes text transcriptions, labels, and images used for training and evaluation of the models. The code for data preprocessing is available at https://github.com/khleeloo/ERIT.
References
- Acheampong et al. (2020) Francisca Adoma Acheampong, Chen Wenyu, and Henry Nunoo-Mensah. Text-based emotion detection: Advances, challenges, and opportunities. Engineering Reports, 2(7), May 2020. ISSN 2577-8196. doi: 10.1002/eng2.12189. URL http://dx.doi.org/10.1002/eng2.12189.
- Alayrac et al. (2022) Jean-Baptiste Alayrac, Jeff Donahue, Pauline Luc, Antoine Miech, Iain Barr, Yana Hasson, Karel Lenc, Arthur Mensch, Katherine Millican, Malcolm Reynolds, Roman Ring, Eliza Rutherford, Serkan Cabi, Tengda Han, Zhitao Gong, Sina Samangooei, Marianne Monteiro, Jacob Menick, Sebastian Borgeaud, Andrew Brock, Aida Nematzadeh, Sahand Sharifzadeh, Mikolaj Binkowski, Ricardo Barreira, Oriol Vinyals, Andrew Zisserman, and Karen Simonyan. Flamingo: a visual language model for few-shot learning. In Alice H. Oh, Alekh Agarwal, Danielle Belgrave, and Kyunghyun Cho, editors, Advances in Neural Information Processing Systems, 2022. URL https://openreview.net/forum?id=EbMuimAbPbs.
- Ebner et al. (2010) Natalie C. Ebner, Michaela Riediger, and Ulman Lindenberger. Faces—a database of facial expressions in young, middle-aged, and older women and men: Development and validation. Behavior Research Methods, 42(1):351–362, feb 2010. ISSN 1554-3528. doi: 10.3758/brm.42.1.351. URL http://dx.doi.org/10.3758/BRM.42.1.351.
- George and Muhamed Ilyas (2024) Swapna Mol George and P. Muhamed Ilyas. A review on speech emotion recognition: A survey, recent advances, challenges, and the influence of noise. Neurocomputing, 568:127015, February 2024. ISSN 0925-2312. doi: 10.1016/j.neucom.2023.127015. URL http://dx.doi.org/10.1016/j.neucom.2023.127015.
- Huang et al. (2023) Zi-Yu Huang, Chia-Chin Chiang, Jian-Hao Chen, Yi-Chian Chen, Hsin-Lung Chung, Yu-Ping Cai, and Hsiu-Chuan Hsu. A study on computer vision for facial emotion recognition. Scientific Reports, 13(1), May 2023. ISSN 2045-2322. doi: 10.1038/s41598-023-35446-4. URL http://dx.doi.org/10.1038/s41598-023-35446-4.
- Leong et al. (2023) Sze Chit Leong, Yuk Ming Tang, Chung Hin Lai, and C.K.M. Lee. Facial expression and body gesture emotion recognition: A systematic review on the use of visual data in affective computing. Computer Science Review, 48:100545, May 2023. ISSN 1574-0137. doi: 10.1016/j.cosrev.2023.100545. URL http://dx.doi.org/10.1016/j.cosrev.2023.100545.
- Li and Peng (2024) Jixiang Li and Jianxin Peng. End-to-end multimodal emotion recognition based on facial expressions and remote photoplethysmography signals. IEEE Journal of Biomedical and Health Informatics, pages 1–10, 2024. doi: 10.1109/JBHI.2024.3430310.
- Lian et al. (2024) Zheng Lian, Licai Sun, Haiyang Sun, Kang Chen, Zhuofan Wen, Hao Gu, Bin Liu, and Jianhua Tao. Gpt-4v with emotion: A zero-shot benchmark for generalized emotion recognition. Information Fusion, 108:102367, aug 2024. ISSN 1566-2535. doi: 10.1016/j.inffus.2024.102367. URL http://dx.doi.org/10.1016/j.inffus.2024.102367.
- Ma et al. (2019) Kaixin Ma, Xinyu Wang, Xinru Yang, Mingtong Zhang, Jeffrey M Girard, and Louis-Philippe Morency. Elderreact: A multimodal dataset for recognizing emotional response in aging adults. In 2019 International Conference on Multimodal Interaction, ICMI ’19, page 349–357, New York, NY, USA, 2019. Association for Computing Machinery. ISBN 9781450368605. doi: 10.1145/3340555.3353747. URL https://doi.org/10.1145/3340555.3353747.
- Minear and Park (2004) Meredith Minear and Denise C. Park. A lifespan database of adult facial stimuli. Behavior Research Methods, Instruments, & Computers, 36(4):630–633, nov 2004. ISSN 1532-5970. doi: 10.3758/bf03206543. URL http://dx.doi.org/10.3758/BF03206543.
- Nandwani and Verma (2021) Pansy Nandwani and Rupali Verma. A review on sentiment analysis and emotion detection from text. Social Network Analysis and Mining, 11(1), August 2021. ISSN 1869-5469. doi: 10.1007/s13278-021-00776-6. URL http://dx.doi.org/10.1007/s13278-021-00776-6.
- OpenAI (2023a) OpenAI. Gpt-4o technical report. arXiv preprint arXiv:2303.08774, 2023a. URL https://huggingface.co/papers/2303.08774.
- OpenAI (2023b) OpenAI. Gpt-4v technical report. arXiv preprint arXiv:2303.08774, 2023b. URL https://arxiv.org/abs/2303.08774.
- Radford et al. (2023) Alec Radford, Jong Wook Kim, Tao Xu, Greg Brockman, Christine McLeavey, and Ilya Sutskever. Robust speech recognition via large-scale weak supervision. In Proceedings of the 40th International Conference on Machine Learning, ICML’23, page 27. JMLR.org, 2023.
- Sharma et al. (2021) Aparna Sharma, Yash Rathi, Vibhor Patni, and Deepak Kumar Sinha. A systematic review of assistance robots for elderly care. In 2021 International Conference on Communication information and Computing Technology (ICCICT), pages 1–6, 2021. doi: 10.1109/ICCICT50803.2021.9510142.
- Yang et al. (2020) Zhengyuan Yang, Amanda Kay, Yuncheng Li, Wendi F. Cross, and Jiebo Luo. Pose-based body language recognition for emotion and psychiatric symptom interpretation. 2020 25th International Conference on Pattern Recognition (ICPR), pages 294–301, 2020. URL https://api.semanticscholar.org/CorpusID:226226561.
- Zhang et al. (2023) Renrui Zhang, Jiaming Han, Chris Liu, Peng Gao, Aojun Zhou, Xiangfei Hu, Shilin Yan, Pan Lu, Hongsheng Li, and Yu Qiao. Llama-adapter: Efficient fine-tuning of language models with zero-init attention. arXiv preprint arXiv:2303.16199, 2023.
- Zhao and Shu (2023) Yunhao Zhao and Xiaoqing Shu. Speech emotion analysis using convolutional neural network (cnn) and gamma classifier-based error correcting output codes (ecoc). Scientific Reports, 13(1), November 2023. ISSN 2045-2322. doi: 10.1038/s41598-023-47118-4. URL http://dx.doi.org/10.1038/s41598-023-47118-4.