Elsevier LaTeX template111Fully documented templates are available in the elsarticle package on CTAN.
Abstract
This template helps you to create a properly formatted LaTeX manuscript.
keywords:
elsarticle.cls, LaTeX, Elsevier , templateMSC:
[2010] 00-01, 99-00url]www.elsevier.com

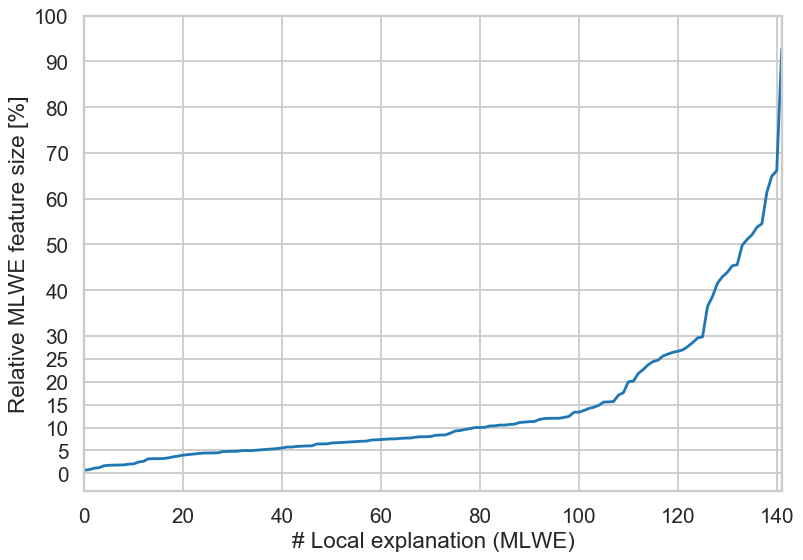
[Original text]
They are nuts that have the Liberal government by the short and curlies, the good news is we see them a d they are being rejected on a global stage! It can’t happen fast enough, the Marxist pigs are a freak show better suited for a circus.
\subfloat[EXP1: Noun - POS feature extraction]
They are \textcoloransi-red-intensenuts that have the Liberal \textcoloransi-red-intensegovernment by the short and \textcoloransi-red-intensecurlies, the good \textcoloransi-red-intensenews is we see them a \textcoloransi-red-intensed they are being rejected on a global \textcoloransi-red-intensestage! It can’t happen fast enough, the \textcoloransi-red-intenseMarxist pigs are a freak \textcoloransi-red-intenseshow better suited for a \textcoloransi-red-intensecircus.
[EXP2: Multi-layer word embedding feature extraction] They are \textcoloransi-red-intensenuts that have the Liberal government by the short and curlies, the good news is we see them a d they are being rejected on a global stage! It can’t happen fast enough, the Marxist \textcoloransi-red-intensepigs are a \textcoloransi-red-intensefreak show better \textcoloransi-red-intensesuited for a \textcoloransi-red-intensecircus.
Explanation | Feature | |||
---|---|---|---|---|
EXP1 | POS-Noun | T | C | 0.608 |
EXP2 | MLWE | T | C | 0.999 |
tableQuantitative explanation for example in Figure 3. T is the Toxic label, C is the Clean label.
In the third example, Figure 3, the custom LSTM model has been exploited analyzing the decision-making process that brought it to classify the input document in Figure 3 as Toxic. The most influential features extracted by \XXX are showed in Figures 3 and 3, while the quantitative explanation is reported in Table 3. An analysis process of the explanation reports similar to the one performed in the previous examples can be followed even in this case. The POS analysis identifies the Nouns as more influential part-of-speech for the prediction of the Toxic class label with a nPIR score of 0.608 (EXP1). Moreover, the MLWE feature analysis, finds the cluster of words {nuts, pigs, freak, suited, circus} as a very positively important feature for the prediction of class Toxic with an nPIR score of 0.999 (EXP2). As can be noticed, the two features are quite similar, but the MLWE can find a tiny number of words that together cause the prediction of the class label. Moreover, the MLWE features appear to be significant for the performed prediction, enforcing the trustfulness of the performed prediction. Thus, thanks to the provided explanations it is straightforward to confirm the correctness of the decision-making process performed by the LSTM model for this specific input document, from the moment that \XXX identified as influential specific words that are usually correlated to a toxic language.