DUAL LEARNING FOR LARGE VOCABULARY ON-DEVICE ASR
Abstract
Dual learning is a paradigm for semi-supervised machine learning that seeks to leverage unsupervised data by solving two opposite tasks at once. In this scheme, each model is used to generate pseudo-labels for unlabeled examples that are used to train the other model. Dual learning has seen some use in speech processing by pairing ASR and TTS as dual tasks. However, these results mostly address only the case of using unpaired examples to compensate for very small supervised datasets, and mostly on large, non-streaming models. Dual learning has not yet been proven effective for using unsupervised data to improve realistic on-device streaming models that are already trained on large supervised corpora. We provide this missing piece though an analysis of an on-device-sized streaming conformer trained on the entirety of Librispeech, showing relative WER improvements of without an LM and with an LM.
1 INTRODUCTION
The high cost of supervision in speech datasets has yielded a rich literature on the use of unpaired audio and text to improve ASR performance. The conventional setting of this problem involves use of unpaired data to enable a working ASR system to be trained on a small enough amount of paired data that ASR would otherwise have been unfeasible. Work on this setting of the problem dates back to long before deep neural networks became the dominant approach to speech processing [1, 2, 3].
In the last several years, progress on this problem has advanced to the point where reasonably strong ASR systems can be trained with only tens of hours of supervised data. Methods for doing so fall broadly into two categories: generative methods that model the data distribution itself, and contrastive methods that model the likelihood of a sample given some context.
Generative methods are premised originally on the autoencoder [4], which when applied in the speech domain has been shown to yield strong representations [5]. \sayPredictive coding extends the autoencoder by seeking to predict a sample some number of steps in the future, and can be done autoregressively [6] or non-autoregressively [7]. The success of masked language modeling in the language domain (e.g. [8]) motivated the application of masked prediction [9, 10] and discretization [11] to generative speech pretraining, yielding strong results.
Contrastive methods, on the other hand, are premised on \saycontrastive predictive coding (CPC) [12], in which positive examples and distractors are together used to model how much more or less likely an audio sample is given some context. In principle, this relieves the system from modeling irrelevant details of the data distribution. The Wav2Vec line of papers [13, 14, 15] demonstrated the viability of CPC features for ASR, eventually combining CPC with generative methods to achieve strong performance on Librispeech using as little as ten minutes of supervised audio. WavLM [16] iterated on these ideas and demonstrated usefulness on an array of speech tasks beyond ASR.
While these ideas have enjoyed great success, applications have strongly emphasized the low resource setting and generally involve very large, full-context models. That is, while we’ve seen that we can use unlabeled speech to build an ASR model given very little supervised data, it is not yet clear that we can use it to overcome the challenges inherent in on-device systems on high resource languages. We offer an alternative framing of the problem, in which a large amount of supervised data is available, but the ASR system must operate under the real-world constraints of being able to stream results and being sufficiently small so as to fit on a smartphone. We ask how unsupervised audio and text may be used to improve performance in this setting.
Dual learning is a method in semi-supervised learning in which two opposite tasks are learned simultaneously, with each model providing supervision for the other. This idea has been successful in machine translation in the form of \saybacktranslation [17, 18]. In the speech domain, TTS has been used as the \saydual task to ASR and combined with audio and text reconstruction tasks to some success [19, 20, 21, 22]. However, these results are constrained to the low-resource setting, with non-streaming models and usually with short utterance lengths. The TTS4ASR line of work [23, 24, 25] uses an approach similar to dual learning, in which a TTS model is used to provide supervision for unpaired audio before training. These models have been proven to work with large supervised datasets; [25] in particular yields gains on top of a strong Librispeech baseline. However, even these models do not address the on-device setting, as they are very large ([25] has 600M parameters) and are non-streaming.
In this paper, we seek to fill what we see is a missing piece in the literature and demonstrate the viability of dual learning in the large vocabulary, on-device setting. We show consistent improvements over a supervised streaming conformer optimized for on-device inference trained on all 960 hours of Librispeech.
2 METHODS
In this section, we describe our implementation of ASR pretraining based on dual learning.
2.1 ARCHITECTURE
In order to perform ASR, TTS, and reconstruction in both domains, our implementation must include encoders and decoders for both audio and text. Imitating [26], we implement streaming with an architecture that can emit a provisional hypothesis immediately and then revise it after a short delay. In order to improve the likelihood of success on the two more difficult tasks (ASR and TTS), we adapt these components from existing ASR and TTS architectures. Our audio encoders and text decoder are adapted from conformer [27, 26]. Our text encoder and audio decoder are adapted from Tacotron 2 [28].
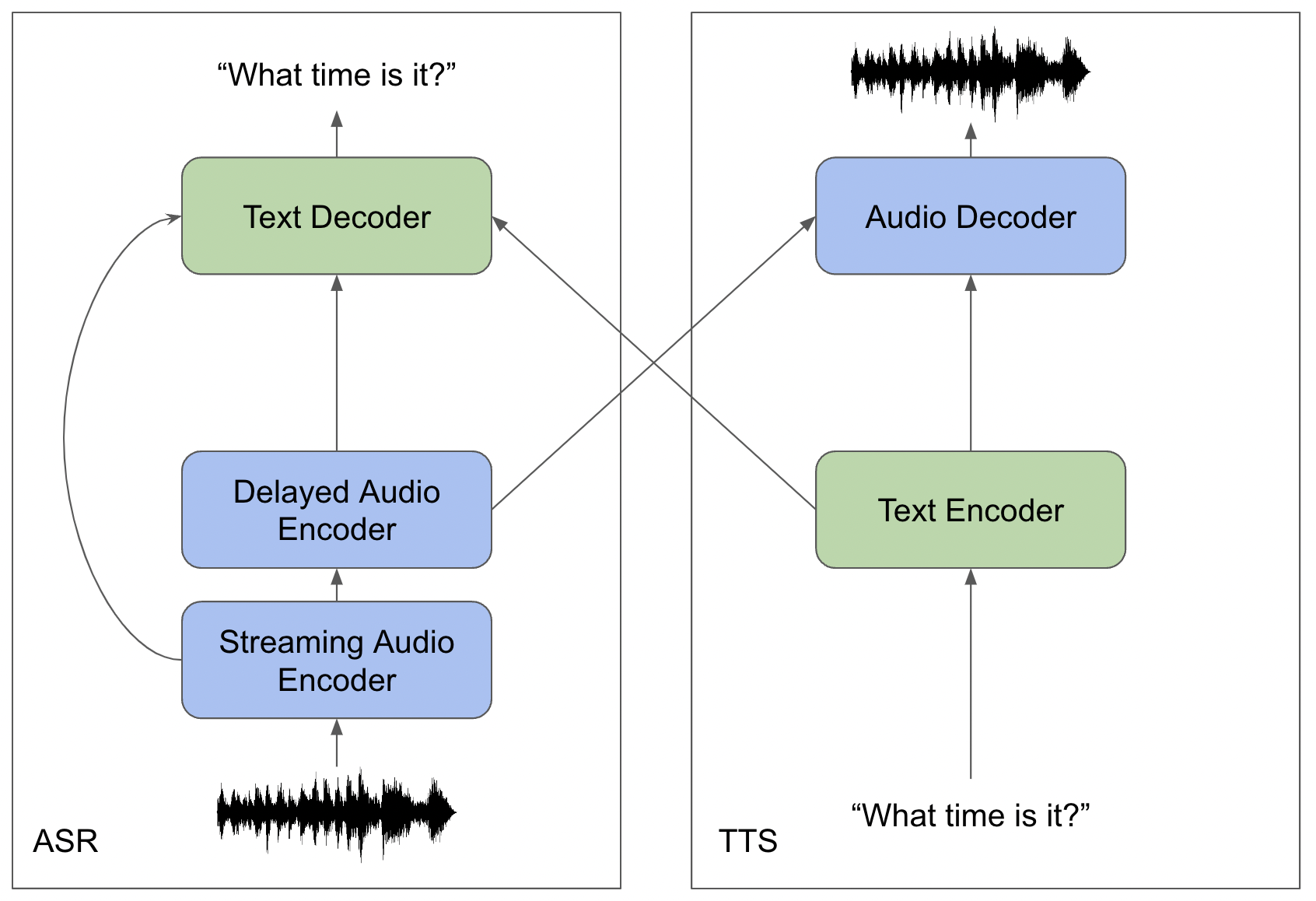
Formally, we frame our problem around three datasets. First, consists of paired text and audio examples , where is an audio sample of length and is the corresponding text transcript of length . Second, gives unpaired text examples , and finally gives unpaired audio examples . We then define the components of the model as functions. We define the audio encoders , which has only left-context and , which has 900ms of right-context. We also define the text encoder , the decoders and , and the linear transformations which maps an audio embedding to a text embedding and which maps a text embedding to an audio embedding.
We may then proceed to define the model’s objectives.
2.1.1 Supervised ASR and TTS
We follow [26] to build a model capable of emitting an ASR hypothesis in real time while streaming finalized predictions with 900ms latency. To this end, we split ASR training into two losses. For , the immediate streaming task is given by:
while the delayed task is given by:
where denotes function composition. That is, the streaming task uses only the first, left-context encoder while the delayed task adds a further encoder with 900ms of right-context. Here we have defined as the cross-entropy loss over text units.
Since this work focuses on pretraining for ASR systems, we do not seek to stream the TTS task. Instead we define the single full-context task:
where we have defined as the mean squared error loss over continuous audio features.
2.1.2 Unsupervised ASR and TTS
Dual learning for speech and text involves the use of the TTS system to provide pseudo-labels for the ASR system and visa versa. Specifically, for an unpaired text example we derive the pseudo-label by beam search over the outputs of the ASR model. We may then define the objectives:
and
Similarly, for an unpaired audio example we may derive the pseudo-label by performing inference in the TTS system. We may then define the objective:
2.1.3 Reconstruction
To perform text reconstruction, we must pass representations from the ASR encoder to the TTS decoder and visa versa. We find that when initializing using pre-trained ASR and TTS systems, the components struggle to adapt to each-other and the model fails to converge. We find that this problem is alleviated simply by placing a single linear transformation between the audio encoder and audio decoder, and another transformation between the text encoder and text decoder. With this in mind, we define the text reconstruction task:
for , with defined analogously for . We similarly define the audio reconstruction task:
for , with defined analogously for .
2.2 Training
We might naively seek to train the above tasks together by alternating tasks across sequences of batches. We find that such a training scheme fails to achieve convergence, as each task is forgotten during the training of the others. Instead, we combine all the above tasks in a single batch. Each batch is split in thirds, the first coming from , the second from , and the last from . For the first third, we jointly optimize the supervised tasks:
for the second third, we optimize the unsupervised audio tasks:
for the last third, we optimize the unsupervised text tasks:
We find that this method achieves convergence, so long as we initialize the model’s components from an ASR and TTS system trained on . Otherwise, the model generates incorrect pseudo-labels early in training, preventing progress.
2.3 Language Modeling
Since dual learning involves the incorporation of unpaired text at training time, we naturally want to compare our method to the incorporation of unpaired text at inference time. To this end we evaluate our models with shallow fusion [29] with a pretrained LM. We also use a Hybrid Autoregressive Transducer (HAT) [30] text decoder, which permits the factorization of our models’ internal LM. Ultimately, at inference time for audio sample we seek:
where gives our acoustic model posterior, gives the likelihood of a transcript in the external LM, gives the likelihood of the transcript in the internal LM (as formulated in HAT), and and are hyperparameters.
Model | Baseline | Shallow Fusion | Internal LM |
---|---|---|---|
BASELINE | 8.4 | 6.3 | 5.8 |
E-ALL | 7.5 | 5.6 | 5.5 |
E-DL | 8.1 | 6.2 | 6 |
E-RECON | 10.3 | 7.4 | 7.2 |
Model | Baseline | Shallow Fusion | Internal LM |
---|---|---|---|
BASELINE | 22.9 | 19.5 | 18.3 |
E-ALL | 20.4 | 16.3 | 16.2 |
E-DL | 21.9 | 18.3 | 17.9 |
E-RECON | 27 | 22.5 | 21.9 |
3 EXPERIMENTAL SETUP
In this section, we give the details of our experimental setup.
3.1 Model
The ASR branch of our model is a cascading conformer adapted from [26], specifically sized to be realistic for an on-device streaming application. The streaming encoder is small to ensure fast inference. It consists first of 3 convolutional layers followed by 7 conformer layers with a 512-dimensional representation for a total of 56M parameters. The delayed encoder is larger, and is parameterized by 10 conformer layers with a 640-dimensional representation for a total of 99M parameters. Following [31], the HAT decoder consists of an embedding network and joint network, contributing another 9M parameters.
The TTS branch of our model is adapted from Tacotron 2 [28]. The text encoder first maps wordpieces into a 512-dimensional embedding space, followed by three convolutional layers and a single bidirectional LSTM layer, totalling 8M parameters. The audio decoder consumes the audio sample autoregressively through a \saypre-net, which consists of two fully-connected layers with 50% dropout at each layer. We find that this aggressive dropout is critical to convergence, since during multi-task training with teacher forcing the TTS decoder has a strong tendency to rely entirely on the autoregressive signal instead of the encoder representation. This yields poor performance at inference, which in turn creates poor pseudo-labels for unpaired text. Scheduled sampling [32] was investigated as an alternative, but was found to be less effective than simple dropout. The audio sample is then passed to two LSTM layers, which also consume the encoder representation via cross-attention. After the LSTM has generated an audio prediction, that result is further processed by a full-context \saypost-net consisting of five convolutional layers. In total, the TTS decoder has about 26M parameters.
3.2 Data
We use 960 hours of supervised audio from Librispeech as , 60k hours of unsupervised audio from Librilight [33] as , and 80M transcripts from the Librispeech LM set as . We process the audio into a 128-dimensional log-mel feature per 10ms of audio. We stack every third such feature with the three features before it, yielding 512-dimensional features at 30ms intervals. We then apply SpecAugment [34] with mask parameter and ten time masks, as in [27]. This forms the inputs to the audio encoder.
3.3 Evaluation
We evaluate our models using a beam search with a beam size of 8. For fusion experiments, we use an external language model trained on . The LM is a causal transformer [35] with 8 layers, 16 attention heads, and a model dimension of 1024, totaling about 100M parameters.
4 RESULTS
We evaluate our model relative to a baseline ASR system trained on 960 hours of Librispeech (BASELINE). The difference between our baseline WER and those reported in full-context works like [16] reflect the added difficulty of streaming results as well as the reduced model size. We contrast this with a model trained on all tasks defined above (E-ALL). We perform ablations by also training a model on only the supervised and dual learning tasks (E-DL, excluding , , , and ) and another only on the supervised and reconstruction tasks (E-RECON, excluding , , and ). Results are given in Table 1.
We find our method to improve performance on the test-clean/test-other test sets by without an LM and with an LM included via shallow fusion. Interestingly, we find that while dual learning alone yields improvements, reconstruction alone (E-RECON) does not. Nevertheless, the combination of dual learning and reconstruction (E-ALL) yields better results than either alone. This suggests that reconstruction itself distracts from the ASR tasks but synergizes with dual learning. This may reflect the fact that on the multi-speaker, long utterances of Librispeech, a joint model benefits from extra exposure to the unsupervised training data in order to produce strong pseduo-labels for dual learning.
4.1 Effect of the Language Model
We note with interest that while the application of shallow fusion preserves the gains yielded by our method, further subtracting out the internal language model via HAT only partially preserves those gains. That is, subtracting out the internal language model substantially closes the gap between the baseline and our method. Figure 2 illustrates this effect by plotting WER for a parameter sweep of external LM interpolation (shallow fusion) weights and internal LM interpolation (HAT) weights. Quantitatively, subtracting out the internal LM with a factor of from a model with shallow fusion improves BASELINE by while only improving E-ALL by .
This result suggests that our method largely benefits the internal language representation of the ASR system’s decoder. This explanation is consistent with other methods of incorporating unsupervised text into a model at training time such as deep fusion [36] and cold fusion [37], but without adding any additional parameters to the ASR model at inference time. That is, unlike conventional language model fusion, our method bakes knowledge of that data into the parameters of the decoder, providing much the same effect as combining the ASR system with a pretrained language model with no modifications to the architecture.
This result also suggests that the improvements due to our method come mostly, but not entirely, from the unsupervised text data, as opposed to the unsupervised audio. This is consistent with our design; unsupervised text yields pseudo-labeled examples for the ASR task, while unsupervised audio yields pseudo-labeled examples for the TTS task.
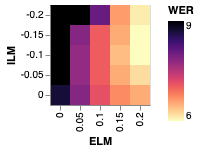
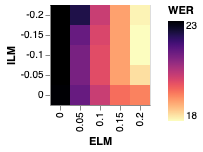
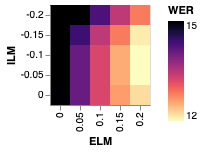
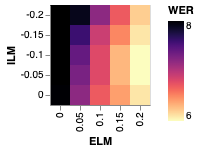
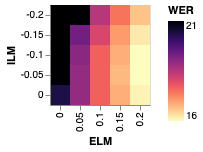
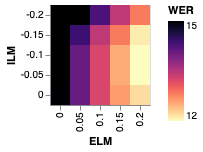
4.2 Tail Analysis
Since unsupervised data is often used to address parts of the data distribution that are absent from the supervised training set, we seek to understand the effect of our method on \saytail words, which we define as words that are underrepresented in the training data relative to their frequency in the language as a whole. To this end, we draw inspiration from [38] and generate a test set of examples containing words that are not represented in but are represented in . Specifically, for some parameter we sample transcripts from containing at least one unigram that occurs with frequency less than in but greater than in . We set and generate a test set of 10k transcripts. We then synthesize audio transcripts using a Tacotron TTS system as in [28]. Results on this tail test set are given in Table 1, and measurements of internal and external LM integration are included in Figure 2.
Model | Baseline | Shallow Fusion | Internal LM |
---|---|---|---|
BASELINE | 16.1 | 13.8 | 12.9 |
E-ALL | 15.3 | 12.6 | 12.4 |
E-DL | 15.5 | 13.1 | 12.8 |
E-RECON | 17.4 | 14.5 | 14.2 |
As above, we find that our method yields improvements across the board. However, we note that the improvements are smaller than they are on Librispeech test sets. In particular, without an LM we improve by , with shallow fusion we improve by , and with internal language model subtraction we improve by .
5 CONCLUSION
In this paper, we demonstrate that dual learning is an effective method for pretraining an on-device, streaming ASR model using both unsupervised audio and text data. We provide an analysis that suggests that the majority of improvements due to the method are attributable to a refinement of the ASR decoder’s internal language representation. We believe that this work motivates exploration in how proven methods for modeling unsupervised audio such as discretization and masked language modeling could further improve these results.
References
- [1] Long Nguyen and Bing Xiang, “Light supervision in acoustic model training,” in 2004 IEEE International Conference on Acoustics, Speech, and Signal Processing.
- [2] Lori Lamel, Jean-Luc Gauvain, and Gilles Adda, “Lightly supervised and unsupervised acoustic model training,” .
- [3] Jeff Ma and Richard Schwartz, “Unsupervised versus supervised training of acoustic models.,” in Interspeech, 2008.
- [4] Lukasz Kaiser and Samy Bengio, “Discrete autoencoders for sequence models,” in International Conference on Learning Representations (ICLR), 2018.
- [5] Jan Chorowski, Ron J. Weiss, Samy Bengio, and Aäron van den Oord, “Unsupervised speech representation learning using wavenet autoencoders,” in IEEE Transactions on Audio, Speech, and Language Processing, 2019.
- [6] Yu-An Chung, Hao Tang, and James Glass, “Vector-quantized autoregressive predictive coding,” in Interspeech, 2020.
- [7] Alexander H. Liu, Yu-An Chung, and James R. Glass, “Non-autoregressive predictive coding for learning speech representations from local dependencies,” in Interspeech, 2021.
- [8] Jacob Devlin, Ming-Wei Chang, Kenton Lee, and Kristina Toutanova, “BERT: pre-training of deep bidirectional transformers for language understanding,” in Annual Conference of the North American Chapter of the Association for Computational Linguistics (NAACL), 2018.
- [9] Dongwei Jiang, Xiaoning Lei, Wubo Li, Ne Luo, Yuxuan Hu, Wei Zou, and Xiangang Li, “Improving transformer-based speech recognition using unsupervised pre-training,” in Interspeech, 2020.
- [10] Andy T. Liu, Shu wen Yang, Po-Han Chi, Po chun Hsu, and Hung yi Lee, “Mockingjay: Unsupervised speech representation learning with deep bidirectional transformer encoders,” in IEEE International Conference on Acoustics, Speech and Signal Processing (ICASSP), 2020.
- [11] Alexei Baevski, Michael Auli, and Abdelrahman Mohamed, “Effectiveness of self-supervised pre-training for speech recognition,” in IEEE International Conference on Acoustics, Speech and Signal Processing (ICASSP), 2020.
- [12] Aäron van den Oord, Yazhe Li, and Oriol Vinyals, “Representation learning with contrastive predictive coding,” in Advancements in Neural Information Processing Systems (NeurIPS), 2018.
- [13] Steffen Schneider, Alexei Baevski, Ronan Collobert, and Michael Auli, “wav2vec: Unsupervised pre-training for speech recognition,” 2019.
- [14] Alexei Baevski, Steffen Schneider, and Michael Auli, “vq-wav2vec: Self-supervised learning of discrete speech representations,” 2020.
- [15] Alexei Baevski, Henry Zhou, Abdelrahman Mohamed, and Michael Auli, “wav2vec 2.0: A framework for self-supervised learning of speech representations,” 2020.
- [16] Sanyuan Chen, Chengyi Wang, Zhengyang Chen, Yu Wu, Shujie Liu, Zhuo Chen, Jinyu Li, Naoyuki Kanda, Takuya Yoshioka, Xiong Xiao, Jian Wu, Long Zhou, Shuo Ren, Yanmin Qian, Yao Qian, Jian Wu, Michael Zeng, and Furu Wei, “Wavlm: Large-scale self-supervised pre-training for full stack speech processing,” 2022.
- [17] Di He, Yingce Xia, Tao Qin, Liwei Wang, Nenghai Yu, Tie-Yan Liu, and Wei-Ying Ma, “Dual learning for machine translation,” in Advances in Neural Information Processing Systems (NeurIPS), 2016.
- [18] Vu Cong Duy Hoang, Philipp Koehn, Gholamreza Haffari, and Trevor Cohn, “Iterative back-translation for neural machine translation,” in Proceedings of the 2nd Workshop on Neural Machine Translation and Generation, 2018.
- [19] Andros Tjandra, Sakriani Sakti, and Satoshi Nakamura, “Listening while speaking: Speech chain by deep learning,” in IEEE Automatic Speech Recognition and Understanding Workshop (ASRU), 2017.
- [20] Yi Ren, Xu Tan, Tao Qin, Sheng Zhao, Zhou Zhao, and Tie-Yan Liu, “Almost unsupervised text to speech and automatic speech recognition,” in International Conference on Machine Learning (ICML), 2019.
- [21] Jin Xu, Xu Tan, Yi Ren, Tao Qin, Jian Li, Sheng Zhao, and Tie-Yan Liu, “Lrspeech: Extremely low-resource speech synthesis and recognition,” in Knowledge Discovery and Data Mining (KDD), 2020.
- [22] Takaaki Hori, Ramón Fernandez Astudillo, Tomoki Hayashi, Yu Zhang, Shinji Watanabe, and Jonathan Le Roux, “Cycle-consistency training for end-to-end speech recognition,” in IEEE International Conference on Acoustics, Speech and Signal Processing (ICASSP), 2019.
- [23] Gary Wang, Andrew Rosenberg, Zhehuai Chen, Yu Zhang, Bhuvana Ramabhadran, Yonghui Wu, and Pedro Moreno, “Improving speech recognition using consistent predictions on synthesized speech,” in IEEE International Conference on Acoustics, Speech and Signal Processing (ICASSP), 2020.
- [24] Zhehuai Chen, Yu Zhang, Andrew Rosenberg, Bhuvana Ramabhadran, Gary Wang, and Pedro Moreno, “Injecting text in self-supervised speech pretraining,” in IEEE Automatic Speech Recognition and Understanding Workshop (ASRU), 2021.
- [25] Zhehuai Chen, Yu Zhang, Andrew Rosenberg, Bhuvana Ramabhadran, Pedro Moreno, and Gary Wang, “Tts4pretrain 2.0: Advancing the use of text and speech in asr pretraining with consistency and contrastive losses,” in IEEE International Conference on Acoustics, Speech and Signal Processing (ICASSP), 2022.
- [26] Arun Narayanan, Tara N. Sainath, Ruoming Pang, Jiahui Yu, Chung-Cheng Chiu, Rohit Prabhavalkar, Ehsan Variani, and Trevor Strohman, “Cascaded encoders for unifying streaming and non-streaming asr,” 2021.
- [27] Anmol Gulati, James Qin, Chung-Cheng Chiu, Niki Parmar, Yu Zhang, Jiahui Yu, Wei Han, Shibo Wang, Zhengdong Zhang, Yonghui Wu, and Ruoming Pang, “Conformer: Convolution-augmented transformer for speech recognition,” in Interspeech, 2020.
- [28] Jonathan Shen, Ruoming Pang, Ron J. Weiss, Mike Schuster, Navdeep Jaitly, Zongheng Yang, Zhifeng Chen, Yu Zhang, Yuxuan Wang, R. J. Skerry-Ryan, Rif A. Saurous, Yannis Agiomyrgiannakis, and Yonghui Wu, “Natural TTS synthesis by conditioning wavenet on mel spectrogram predictions,” in IEEE International Conference on Acoustics, Speech and Signal Processing (ICASSP), 2018.
- [29] Çaglar Gülçehre, Orhan Firat, Kelvin Xu, Kyunghyun Cho, Loïc Barrault, Huei-Chi Lin, Fethi Bougares, Holger Schwenk, and Yoshua Bengio, “On using monolingual corpora in neural machine translation,” CoRR, vol. abs/1503.03535, 2015.
- [30] Ehsan Variani, David Rybach, Cyril Allauzen, and Michael Riley, “Hybrid autoregressive transducer (hat),” in IEEE International Conference on Acoustics, Speech and Signal Processing (ICASSP), 2020.
- [31] Rami Botros, Tara N. Sainath, Robert David, Emmanuel Guzman, Wei Li, and Yanzhang He, “Tied & reduced RNN-T decoder,” in Interspeech, 2021.
- [32] Samy Bengio, Oriol Vinyals, Navdeep Jaitly, and Noam Shazeer, “Scheduled sampling for sequence prediction with recurrent neural networks,” in Advances in Neural Information Processing Systems, 2015.
- [33] Jacob Kahn, Morgane Rivière, Weiyi Zheng, Evgeny Kharitonov, Qiantong Xu, Pierre-Emmanuel Mazaré, Julien Karadayi, Vitaliy Liptchinsky, Ronan Collobert, Christian Fuegen, Tatiana Likhomanenko, Gabriel Synnaeve, Armand Joulin, Abdelrahman Mohamed, and Emmanuel Dupoux, “Libri-light: A benchmark for ASR with limited or no supervision,” in IEEE International Conference on Acoustics, Speech and Signal Processing (ICASSP), 2020.
- [34] Daniel S. Park, William Chan, Yu Zhang, Chung-Cheng Chiu, Barret Zoph, Ekin D. Cubuk, and Quoc V. Le, “SpecAugment: A simple data augmentation method for automatic speech recognition,” in Interspeech, 2019.
- [35] Ashish Vaswani, Noam Shazeer, Niki Parmar, Jakob Uszkoreit, Llion Jones, Aidan N. Gomez, Lukasz Kaiser, and Illia Polosukhin, “Attention is all you need,” in Advancements in Neural Information Processing Systems (NeurIPS), 2017.
- [36] Çaglar Gülçehre, Orhan Firat, Kelvin Xu, Kyunghyun Cho, Loïc Barrault, Huei-Chi Lin, Fethi Bougares, Holger Schwenk, and Yoshua Bengio, “On using monolingual corpora in neural machine translation,” ArXiv, vol. abs/1503.03535, 2015.
- [37] Anuroop Sriram, Heewoo Jun, Sanjeev Satheesh, and Adam Coates, “Cold fusion: Training seq2seq models together with language models,” in Interspeech, 2018.
- [38] Cal Peyser, Sepand Mavandadi, Tara N. Sainath, James Apfel, Ruoming Pang, and Shankar Kumar, “Improving tail performance of a deliberation e2e asr model using a large text corpus,” in Interspeech, 2020.