Does Character-level Information Always Improve
DRS-based Semantic Parsing?
Abstract
Even in the era of massive language models, it has been suggested that character-level representations improve the performance of neural models. The state-of-the-art neural semantic parser for Discourse Representation Structures uses character-level representations, improving performance in the four languages (i.e., English, German, Dutch, and Italian) in the Parallel Meaning Bank dataset. However, how and why character-level information improves the parser’s performance remains unclear. This study provides an in-depth analysis of performance changes by order of character sequences. In the experiments, we compare F1-scores by shuffling the order and randomizing character sequences after testing the performance of character-level information. Our results indicate that incorporating character-level information does not improve the performance in English and German. In addition, we find that the parser is not sensitive to correct character order in Dutch. Nevertheless, performance improvements are observed when using character-level information.
1 Introduction
Character-level information is sometimes helpful in grasping the meanings of words for humans. Previous studies have suggested that character-level information helps to improve the performance of neural models on various NLP tasks (Cherry et al., 2018; Zhang et al., 2015). In multilingual NLP systems, character-level information contributes to performance improvements on Named Entity Recognition tasks (Lample et al., 2016; Yu et al., 2018) and semantic parsing tasks (van Noord et al., 2020). However, due to the black-box nature of neural models, it is still unclear how and why character-level information contributes to model performance.
The rapid developments of neural models have led to a growing interest in investigating the extent to which these models understand natural language. Recent works have indicated that pre-trained language models are insensitive to word order on permuted English datasets on language understanding tasks Sinha et al. (2021a, b); Pham et al. (2021); Hessel and Schofield (2021). Meanwhile, other works have shown controversial results regarding inductive biases for word order Abdou et al. (2022), especially in different languages Ravfogel et al. (2019); White and Cotterell (2021).
In this work, we explore the extent to which neural models capture character order. By focusing on character order rather than word order, we present an in-depth analysis of the capacity of models to capture syntactic structures across languages. To analyze whether the importance of character order information differs across languages, we investigate multilingual Discourse Representation Structure (DRS; Kamp and Reyle (1993)) parsing models. van Noord et al. (2020) proposed an encoder-decoder DRS parsing model incorporating character-level representations. The study concluded that incorporating character-level representations contributes to performance improvements of the model across languages. However, the underlying mechanism remains unclear.
We examine the influence of character-level information on DRS-based semantic parsing tasks using the state-of-the-art model (van Noord et al., 2020). We analyze whether the model is sensitive to the order of character sequences in various units of granularity (i.e., characters, words, and sentences) across the languages. In addition, we investigate whether the amount of information per character-level token affects the model performance. Our data will be publicly available at https://github.com/ynklab/character_order_analysis.
Sentence | Brad Pitt is an actor. |
---|---|
Correct order (unigrams) | ^^^ b r a d ||| ^^^ p i t t ||| i s ||| a n ||| a c t o r ||| . |
UNI | a a a a a a a a a a a a a a a a a a a a a a a a a |
SHF (word-level) | d a r ^^^ b ||| t ^^^ p t i ||| i s ||| a n ||| o t c a r ||| . |
SHF (sentence-level) | c t r r i i . ||| a ||| d a t ||| b p ||| s t ||| ^^^ o n a ^^^ |
RND | " i c v , t 9 d j : l ’ n 6 0 b 0 1 q w ! j w u q |
Bigrams | ^^^b br ra ad d||| |||^^^ ^^^p pi it tt t||| |||i is s||| |
|||a an n||| |||a ac ct to or r||| |||. |
2 Background
Multilingual DRS corpus
The Parallel Meaning Bank (PMB; Abzianidze et al. (2017)) is a multilingual corpus annotated with DRSs. The PMB contains sentences for four languages (English, German, Dutch, and Italian) with three levels of DRS annotation: gold (fully manually checked), silver (partially manually corrected), and bronze (without manual correction). The PMB also provides semantic tags, which are linguistic annotations for producing DRSs Abzianidze and Bos (2017).
Neural DRS parsing models
There have been various attempts to improve the performance of neural DRS parsing models, such as by using graph formats Fancellu et al. (2019); Poelman et al. (2022), stack LSTMs Evang (2019), and sequence labeling models Shen and Evang (2022). van Noord et al. (2020) proposed a sequence-to-sequence model with neural encoders and an attention mechanism (Vaswani et al., 2017). In the study, the number and type of encoders and the type of embeddings of the pre-trained language models, including BERT (Devlin et al., 2019), were changed to evaluate the model. Moreover, linguistic features and character-level representations were added to the model, concluding that character-level representations contribute to the performance improvements in all four languages, compared to using only BERT embeddings as input.
Sensitivity to word order
Several studies have analyzed whether generic language models understand word order Sinha et al. (2021a, b); Pham et al. (2021); Hessel and Schofield (2021); Abdou et al. (2022). However, these studies have focused on text classification benchmarks, such as GLUE Wang et al. (2019), rather than semantic parsing tasks, such as DRS parsing. In addition, these studies did not investigate whether models are sensitive to character order.
3 Experimental Setup
We explore whether character-level information influences the predictions of the state-of-the-art DRS parsing model using character representations (van Noord et al., 2020) across languages. This section introduces the common experimental setup.
Dataset
Models
We focus on two types of architectures: English BERT with semantic tags (BERT + sem) for English and multilingual BERT (mBERT) for the other languages, achieving the highest F1-scores on the PMB release 3.0.0 in the original study (van Noord et al., 2020). These setups use a single bi-LSTM encoder for BERT (or mBERT) embeddings and semantic tags (only English), in the previous study. Whereas the original model used their trigram-based tagger and predicted semantic tags for English, we use the gold semantic tags in the PMB to exclude performance changes based on the accuracy of the tagger. Although PMB also has gold semantic tags for non-English languages, we adopt them only for English to compare with van Noord et al. (2020). We define BERT + sem + char for English and mBERT + char for the other languages with an additional bi-LSTM encoder for character-level representations as the default setting 2-enc + char.
Evaluation metrics
To evaluate model performance precisely, we report averaged micro F1-scores of 15 runs, which are more than those on the settings of the original study (five runs). We use Counter and Referee (van Noord et al., 2018a, b) to calculate the micro F1-score. See Appendix A.1 for further details.
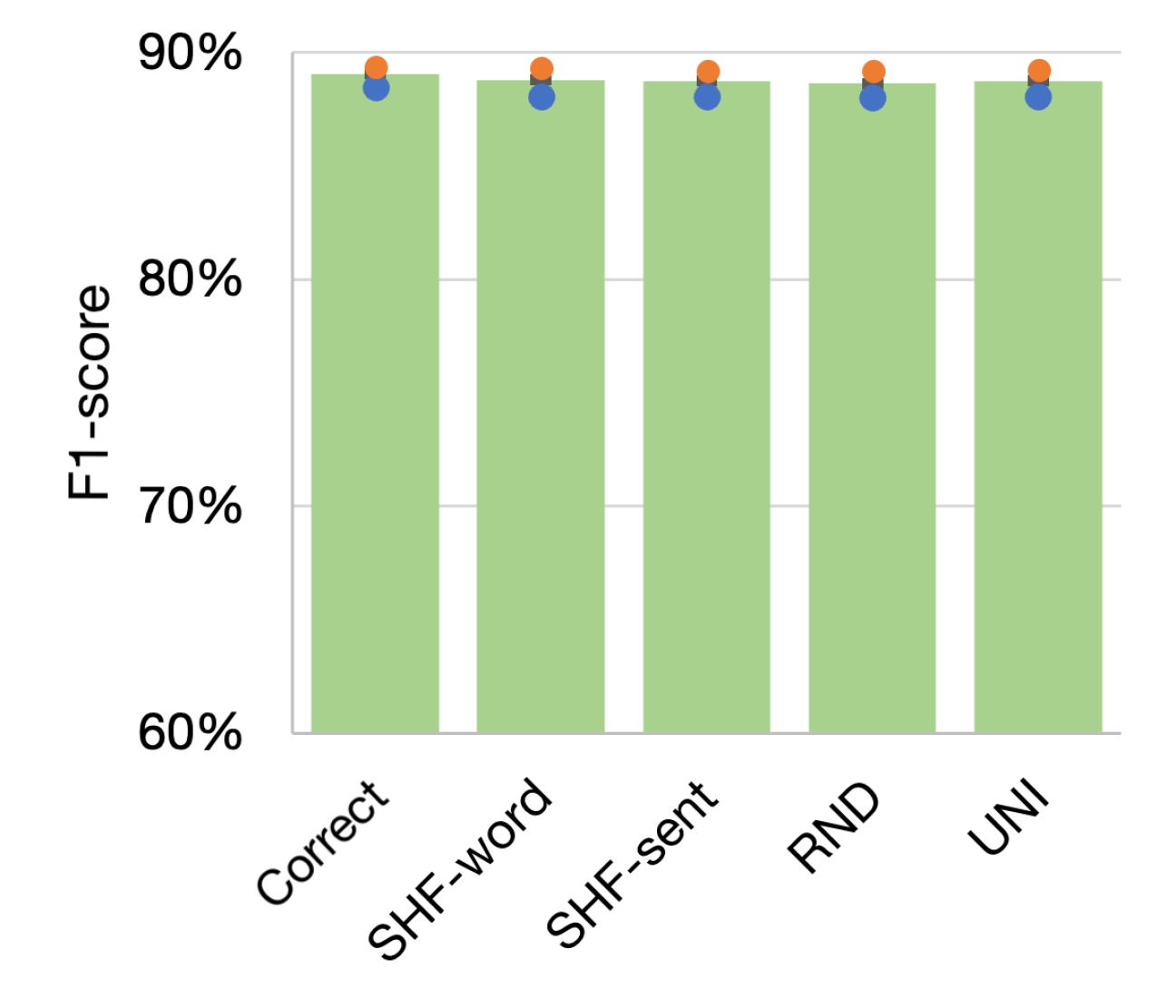
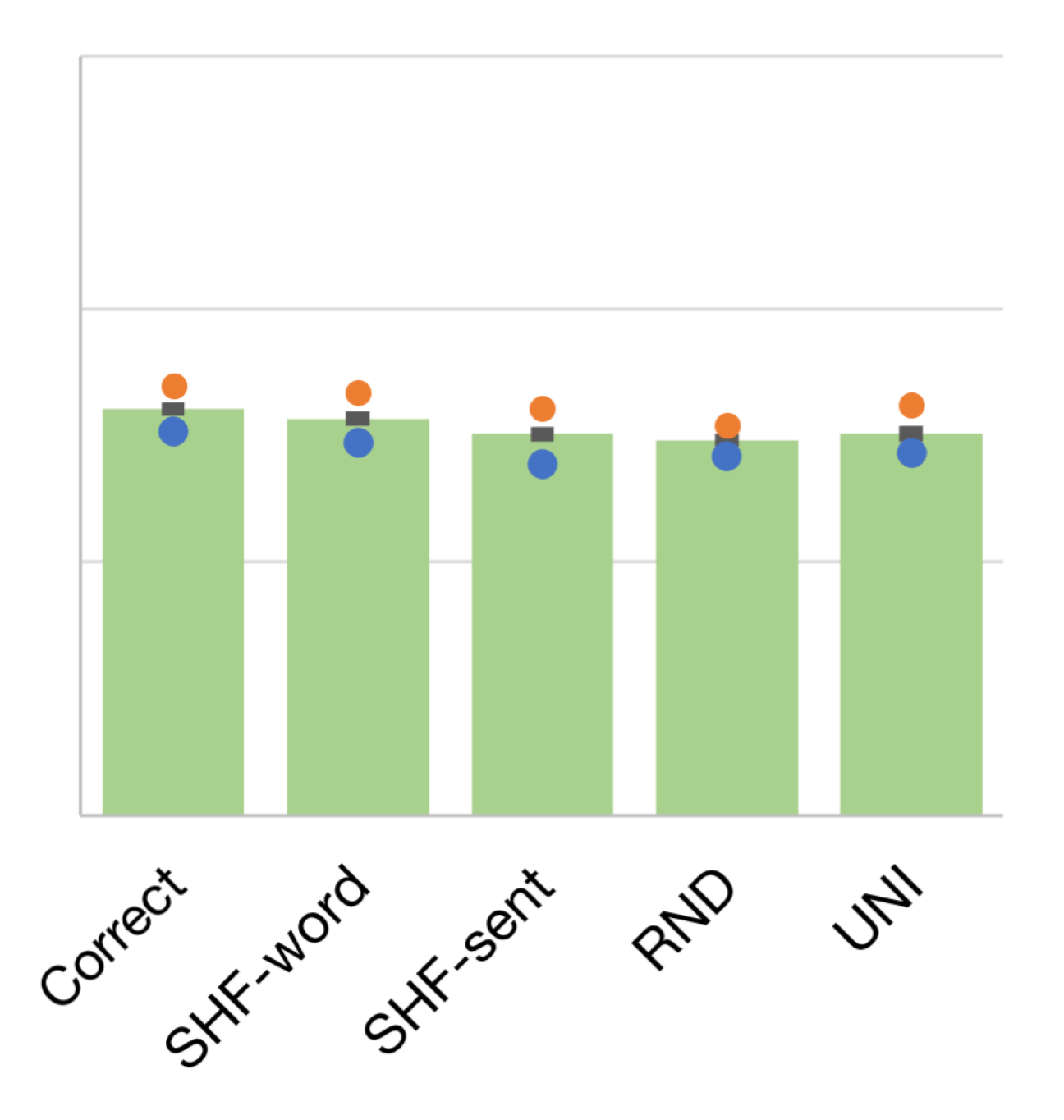
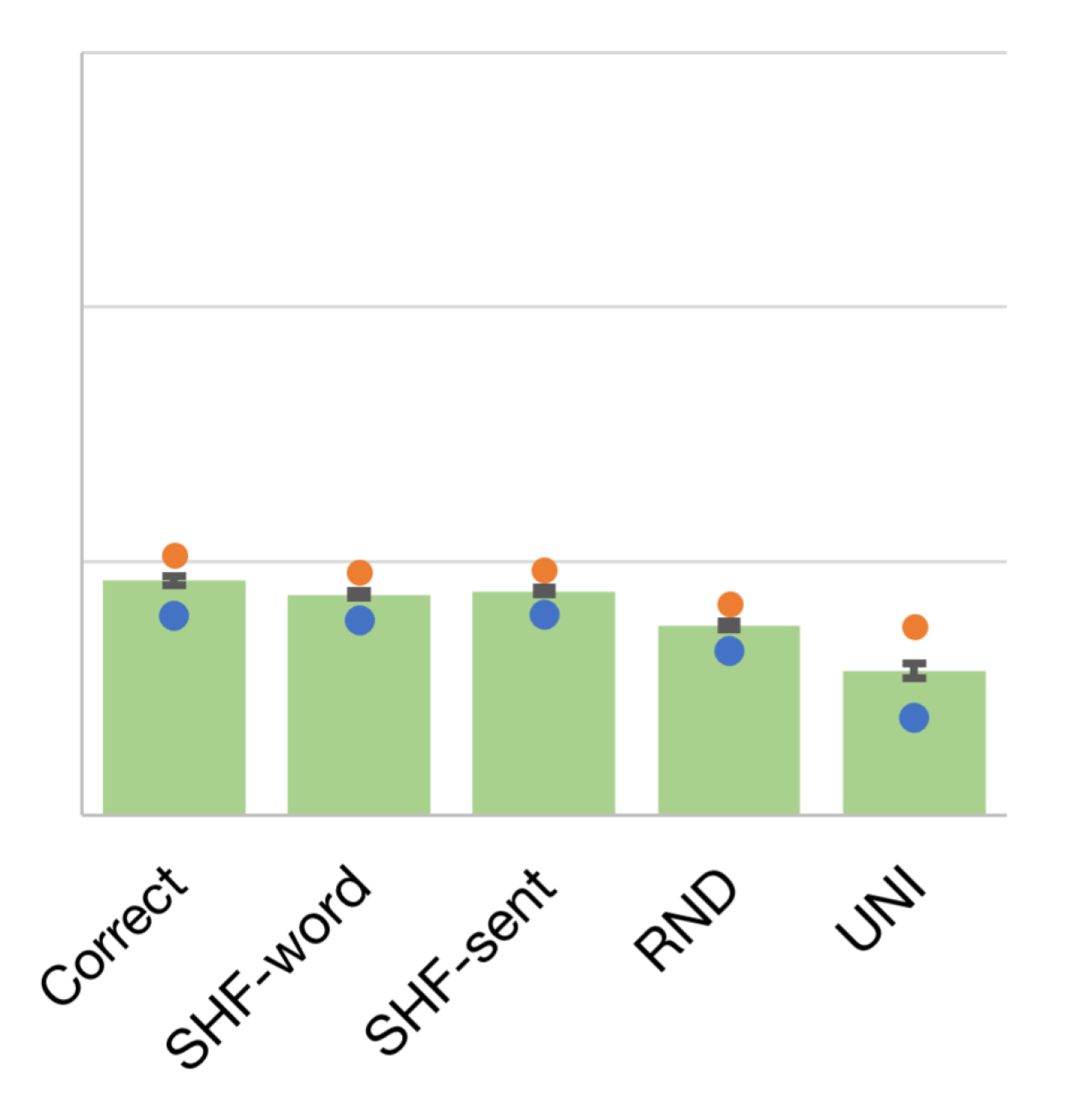
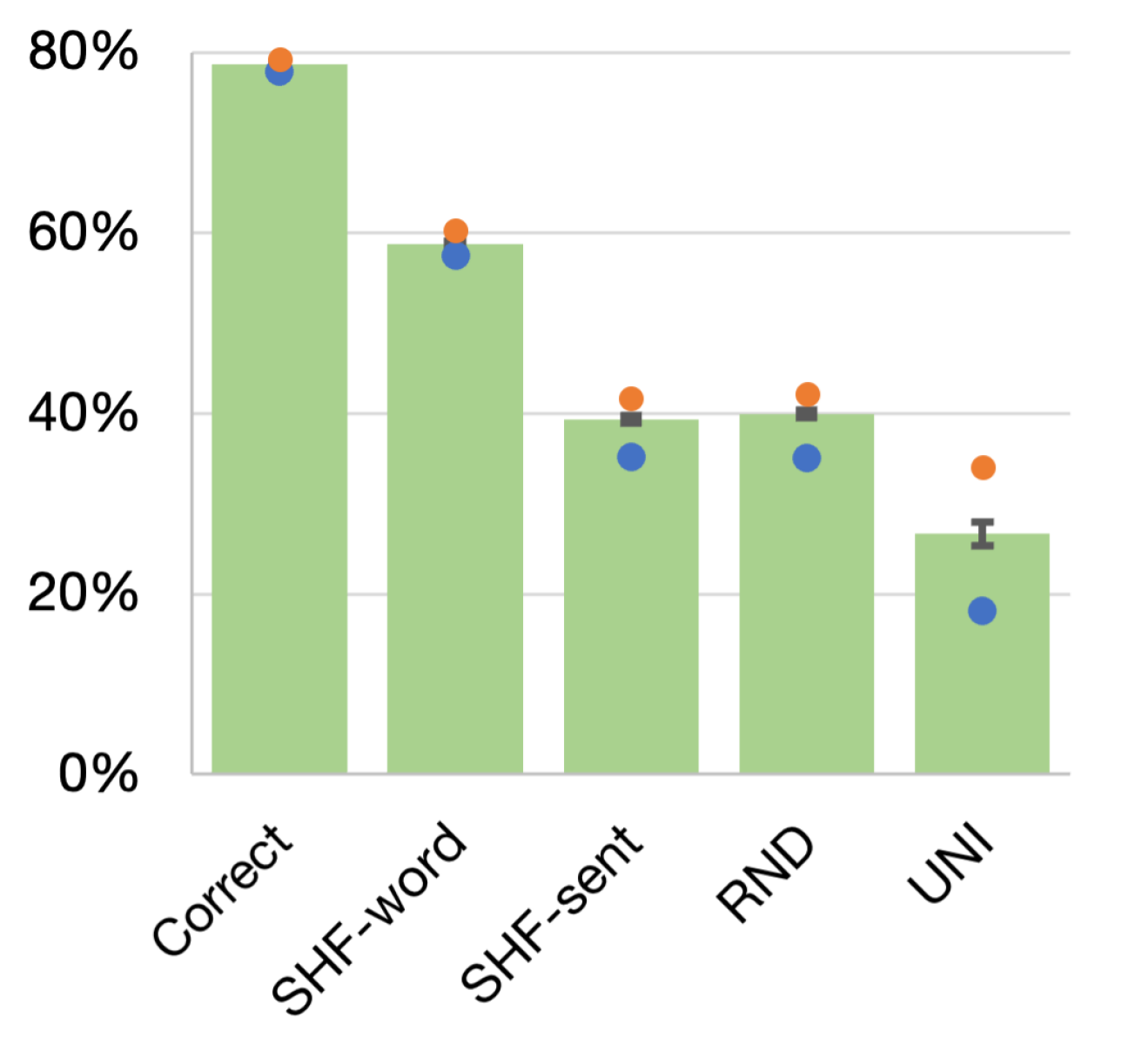
4 Method
We provide multiple methods to reanalyze whether the DRS parsing models van Noord et al. (2020) are sensitive to character-level information across languages in a more fine-grained way. First, we reexamine whether character-level information benefits the model in terms of character sequences compared to the setup without an encoder for characters. Second, we examine whether the model trained with correct character order predicts correct DRSs even with incorrect character sequences obtained using techniques such as shuffling. In the above two methods, we prepare models trained with correct character sequences and evaluate the performance when incorrect character order is input to them. Third, we explore the capacity of the models to understand character-level information using unigrams or bigrams of characters as character tokens. By using unigrams, we mean one character at a time, and by using bigrams, we mean two characters at a time.
4.1 Do models use characters as a clue?
Before examining whether the model is sensitive to character order, we have to reveal whether incorporating character sequences is useful or not for the model. To test this, we prepare the models trained on correct character order and evaluate them using unified character sequences (UNI). Note that our method is a more detailed analysis of van Noord et al. (2020) in claiming whether character-level information is useful (or not). UNI consists of a single character a (see Table 1). As this type of sequences is entirely irrelevant to the input sentences, the model should perform almost the same as setups without an encoder for character-level information. Additionally, we reproduce to compare the values of the no char setups.
4.2 Are models sensitive to character order?
For languages in which the usefulness of character-level information is confirmed (Section 4.1), we analyze whether the model understands correct character order across languages. We create two types of incorrect character sequences by (i) shuffling the order of the character sequences and (ii) randomizing the sequences (see Table 1). If the model is sensitive to correct character order during training, it should fail to predict the correct DRSs with incorrect order.
Shuffled (SHF)
We shuffle the sequences on two levels, word-level and sentence-level. A word-level shuffled character sequence is obtained by shuffling character order within each word (separated by “| | |”, see Table 1). In contrast, a sentence-level shuffled sequence can be created by rearranging the characters in the entire sentence, including spaces. By comparing the performance of these two shuffling levels, we investigate the extent to which the model is confused, depending on the extent of disturbance in the character order.
Randomized (RND)
We provide an additional types of character sequences, randomized character sequences. The randomized sequences consist of characters randomly selected from the PMB in each language.
4.3 Can models be improved performance by extended character sequences?
The original model uses a unigram character as the character token. Typically, the amount of information per character-level token is increased by using bigrams instead of unigrams. Also, the four languages in the PMB consist of alphabets, and the number of letters is limited, unlike several Asian languages such as Chinese and Japanese. Thus we provide bigram sequences other than unigram sequences, treated them as extended character sequences, and train the models using them. In the bigram sequence settings (bigrams), as illustrated in the bottom line of Table 1, the models can obtain not only character order but also the connections of characters from character tokens. If an encoder for character-level representations affects the model performance, the use of bigram sequences is expected to improve the model performance.
5 Results and Discussion
Character contribution for models
Table 2 shows the micro averaged F1-scores with their standard errors. The values in the no char column are F1-scores of the setups without character encoders. The stander errors corresponding to English and German showed significant differences. However, these differences suggest that character-level information is not crucial in DRS parsing. On the other hand, we can see effectiveness in the other languages: Dutch and Italian. In particular, an F1-score change of more than 50% can be observed in Italian. However, values of UNI are far lower than ones of no char in Dutch and Italian. This tendency suggests that providing incorrect character-level information decreases scores critically when incorporating character-level information is effective.
Models’ sensitivity to character order
Figure 1 shows the micro averaged, maximum, and minimum F1-scores for each type of character-level information: correct, SHF-word (word-level SHF), SHF-sent (sentence-level SHF), RND, and UNI (for comparison). In English (Figure 1) and German (Figure 1), only minor changes (1%) were observed in the averaged F1-scores for all types of characters. This observation supports less effectiveness of incorporating character-level information for these two languages. We also experimented with the 2-enc + char model without semantic tags in English and obtained similar trends (see Appendix D).
In Dutch (Figure 1), even though we can see a slight performance decrease from correct to RND, shuffling the character order does not affect the performance of the models. These results indicate that DRS parsing models are not sensitive to character order for Dutch.
For Italian (Figure 1), we can see that the correct character order contributes to the performance of the model. Shuffling the characters within each word decreased the model’s performance by 20% (from 79% to 59%). The performance decreased by another 20% (from 59% to 39%) when shuffling in a whole sentence, compared with SHF-word. One of the possible reasons that the Italian model is significantly sensitive to the character-level information is the existence of the accented characters specific to Italian (e.g., é), especially the loss of it by shuffling characters within sentences (SHF-word SHF-sent). For example, the character é plays the role of an auxiliary verb in Italian by itself. When characters are lost by shuffling them within words (correct SHF-word), shuffled character sequences within words appear to affect the incorrect prediction of words. Further investigation into differences between languages is needed, which is left as future work.
Correct | UNI | No char | |
---|---|---|---|
English | 89.05 0.06 | 88.76 0.09 | 88.89 0.08 |
German | 76.07 0.12 | 75.09 0.17 | 75.33 0.14 |
Dutch | 69.23 0.18 | 65.69 0.30 | 68.81 0.13 |
Italian | 78.75 0.10 | 26.66 1.30 | 77.54 0.09 |
No char | Unigrams | Bigrams | |
---|---|---|---|
English | 88.89 0.08 | 88.99 0.08 | 89.10 0.07 |
German | 75.33 0.14 | 75.94 0.11 | 76.96 0.11 |
Dutch | 68.81 0.13 | 69.22 0.18 | 69.62 0.11 |
Italian | 77.54 0.09 | 78.73 0.11 | 79.46 0.08 |
Extending character tokens improves model performance
Table 3 shows the averaged F1-scores and standard errors obtained using character-level information (bigrams, unigrams, and no char). We observe no significant differences in the overall setups in English. In contrast, in German, Dutch, and Italian, we can find performance improvements in extensions from unigrams to bigrams and from no character-level information to unigrams. In particular, the model achieves the largest improvements by incorporating unigrams as character-level information in Italian and by extending from unigrams to bigrams in German, respectively. These results indicate that although models are not usually sensitive to character order, character-level information helps performance improvements in German, Dutch, and Italian.
One of the reasons models cannot achieve any improvements in English, while improvements are observed in non-English languages, is the quantity and quality of data in the PMB. As noted in the statistics of PMB 3.0.0 (Appendix 1 and Table 4), we can use over 6.6k English gold training data. In addition, nearly 100k sliver cases are available. In contrast, the German dataset only contains 1.2k gold and 5.3k silver cases, and there is no gold case in both Dutch and Italian.
6 Conclusion and Future Work
In this study, we carried out a further exploration of the extent to which character-level representations contribute to the performance improvements of multilingual DRS parsing models. We found that character-level information provided little performance improvement in English and German but improved performance in Dutch and Italian. However, we find that the model is sensitive to character order in Italian but not in Dutch. The take-away message from our investigation is that the importance of character-level information in DRS-based semantic parsing depends on the language and syntactic structures of the sentences.
In future work, we will analyze in more detail the significant differences between the four languages, especially Italian, and other languages. Another direction of our future work is to investigate the relationship between the neural models and humans in reading performance for incorrect character order. It would be interesting to analyze whether the results on DRS parsing tasks are consistent with those of these studies (Ferreira et al., 2002; Gibson et al., 2013; Traxler, 2014).
Limitations
In this study, we focus on DRS parsing tasks, and do not consider other representation formats for semantic parsing tasks.
Acknowledgements
We thank the three anonymous reviewers for their helpful comments and suggestions, which improved this paper. We also thank our colleagues, Aman Jain and Anirudh Reddy Kondapally, for proofreading and providing many comments on our paper. This work was supported by JST, PRESTO grant number JPMJPR21C8, Japan.
References
- Abdou et al. (2022) Mostafa Abdou, Vinit Ravishankar, Artur Kulmizev, and Anders Søgaard. 2022. Word order does matter and shuffled language models know it. In Proceedings of the 60th Annual Meeting of the Association for Computational Linguistics (Volume 1: Long Papers), pages 6907–6919, Dublin, Ireland. Association for Computational Linguistics.
- Abzianidze et al. (2017) Lasha Abzianidze, Johannes Bjerva, Kilian Evang, Hessel Haagsma, Rik van Noord, Pierre Ludmann, Duc-Duy Nguyen, and Johan Bos. 2017. The Parallel Meaning Bank: Towards a multilingual corpus of translations annotated with compositional meaning representations. In Proceedings of the 15th Conference of the European Chapter of the Association for Computational Linguistics: Volume 2, Short Papers, pages 242–247, Valencia, Spain. Association for Computational Linguistics.
- Abzianidze and Bos (2017) Lasha Abzianidze and Johan Bos. 2017. Towards universal semantic tagging. In Proceedings of the 12th International Conference on Computational Semantics (IWCS), Montpellier, France. Association for Computational Linguistics.
- Cherry et al. (2018) Colin Cherry, George Foster, Ankur Bapna, Orhan Firat, and Wolfgang Macherey. 2018. Revisiting character-based neural machine translation with capacity and compression. In Proceedings of the 2018 Conference on Empirical Methods in Natural Language Processing, pages 4295–4305, Brussels, Belgium. Association for Computational Linguistics.
- Devlin et al. (2019) Jacob Devlin, Ming-Wei Chang, Kenton Lee, and Kristina Toutanova. 2019. BERT: Pre-training of deep bidirectional transformers for language understanding. In Proceedings of the 2019 Conference of the North American Chapter of the Association for Computational Linguistics: Human Language Technologies, Volume 1 (Long and Short Papers), pages 4171–4186, Minneapolis, Minnesota. Association for Computational Linguistics.
- Evang (2019) Kilian Evang. 2019. Transition-based DRS parsing using stack-LSTMs. In Proceedings of the IWCS Shared Task on Semantic Parsing, pages 16–23, Gothenburg, Sweden. Association for Computational Linguistics.
- Fancellu et al. (2019) Federico Fancellu, Sorcha Gilroy, Adam Lopez, and Mirella Lapata. 2019. Semantic graph parsing with recurrent neural network DAG grammars. In Proceedings of the 2019 Conference on Empirical Methods in Natural Language Processing and the 9th International Joint Conference on Natural Language Processing (EMNLP-IJCNLP), pages 2769–2778, Hong Kong, China. Association for Computational Linguistics.
- Ferreira et al. (2002) Fernanda Ferreira, Karl G. D. Bailey, and Vittoria Ferraro. 2002. Good-enough representations in language comprehension. Current Directions in Psychological Science, 11(1):11–15.
- Gibson et al. (2013) Edward Gibson, Leon Bergen, and Steven T. Piantadosi. 2013. Rational integration of noisy evidence and prior semantic expectations in sentence interpretation. Proceedings of the National Academy of Sciences, 110(20):8051–8056.
- Hessel and Schofield (2021) Jack Hessel and Alexandra Schofield. 2021. How effective is BERT without word ordering? implications for language understanding and data privacy. In Proceedings of the 59th Annual Meeting of the Association for Computational Linguistics and the 11th International Joint Conference on Natural Language Processing (Volume 2: Short Papers), pages 204–211, Online. Association for Computational Linguistics.
- Kamp and Reyle (1993) Hans Kamp and Uwe Reyle. 1993. From Discourse to Logic. Springer, Dordrecht.
- Lample et al. (2016) Guillaume Lample, Miguel Ballesteros, Sandeep Subramanian, Kazuya Kawakami, and Chris Dyer. 2016. Neural architectures for named entity recognition. In Proceedings of the 2016 Conference of the North American Chapter of the Association for Computational Linguistics: Human Language Technologies, pages 260–270, San Diego, California. Association for Computational Linguistics.
- Pham et al. (2021) Thang Pham, Trung Bui, Long Mai, and Anh Nguyen. 2021. Out of order: How important is the sequential order of words in a sentence in natural language understanding tasks? In Findings of the Association for Computational Linguistics: ACL-IJCNLP 2021, pages 1145–1160, Online. Association for Computational Linguistics.
- Poelman et al. (2022) Wessel Poelman, Rik van Noord, and Johan Bos. 2022. Transparent semantic parsing with Universal Dependencies using graph transformations. In Proceedings of the 29th International Conference on Computational Linguistics, pages 4186–4192, Gyeongju, Republic of Korea. International Committee on Computational Linguistics.
- Ravfogel et al. (2019) Shauli Ravfogel, Yoav Goldberg, and Tal Linzen. 2019. Studying the inductive biases of RNNs with synthetic variations of natural languages. In Proceedings of the 2019 Conference of the North American Chapter of the Association for Computational Linguistics: Human Language Technologies, Volume 1 (Long and Short Papers), pages 3532–3542, Minneapolis, Minnesota. Association for Computational Linguistics.
- Shen and Evang (2022) Minxing Shen and Kilian Evang. 2022. DRS parsing as sequence labeling. In Proceedings of the 11th Joint Conference on Lexical and Computational Semantics, pages 213–225, Seattle, Washington. Association for Computational Linguistics.
- Sinha et al. (2021a) Koustuv Sinha, Robin Jia, Dieuwke Hupkes, Joelle Pineau, Adina Williams, and Douwe Kiela. 2021a. Masked language modeling and the distributional hypothesis: Order word matters pre-training for little. In Proceedings of the 2021 Conference on Empirical Methods in Natural Language Processing, pages 2888–2913, Online and Punta Cana, Dominican Republic. Association for Computational Linguistics.
- Sinha et al. (2021b) Koustuv Sinha, Prasanna Parthasarathi, Joelle Pineau, and Adina Williams. 2021b. UnNatural Language Inference. In Proceedings of the 59th Annual Meeting of the Association for Computational Linguistics and the 11th International Joint Conference on Natural Language Processing (Volume 1: Long Papers), pages 7329–7346, Online. Association for Computational Linguistics.
- Traxler (2014) Matthew J. Traxler. 2014. Trends in syntactic parsing: anticipation, bayesian estimation, and good-enough parsing. Trends in Cognitive Sciences, 18(11):605–611.
- van Noord et al. (2018a) Rik van Noord, Lasha Abzianidze, Hessel Haagsma, and Johan Bos. 2018a. Evaluating scoped meaning representations. In Proceedings of the Eleventh International Conference on Language Resources and Evaluation (LREC 2018), Miyazaki, Japan. European Language Resources Association (ELRA).
- van Noord et al. (2018b) Rik van Noord, Lasha Abzianidze, Antonio Toral, and Johan Bos. 2018b. Exploring neural methods for parsing discourse representation structures. Transactions of the Association for Computational Linguistics, 6:619–633.
- van Noord et al. (2020) Rik van Noord, Antonio Toral, and Johan Bos. 2020. Character-level representations improve DRS-based semantic parsing even in the age of BERT. In Proceedings of the 2020 Conference on Empirical Methods in Natural Language Processing (EMNLP), pages 4587–4603, Online. Association for Computational Linguistics.
- Vaswani et al. (2017) Ashish Vaswani, Noam Shazeer, Niki Parmar, Jakob Uszkoreit, Llion Jones, Aidan N Gomez, Łukasz Kaiser, and Illia Polosukhin. 2017. Attention is all you need. In Advances in Neural Information Processing Systems, volume 30. Curran Associates, Inc.
- Wang et al. (2019) Alex Wang, Amanpreet Singh, Julian Michael, Felix Hill, Omer Levy, and Samuel R. Bowman. 2019. GLUE: A multi-task benchmark and analysis platform for natural language understanding. In Proceedings of the Seventh International Conference on Learning Representations, New Orleans, Louisiana. International Conference on Learning Representations.
- White and Cotterell (2021) Jennifer C. White and Ryan Cotterell. 2021. Examining the inductive bias of neural language models with artificial languages. In Proceedings of the 59th Annual Meeting of the Association for Computational Linguistics and the 11th International Joint Conference on Natural Language Processing (Volume 1: Long Papers), pages 454–463, Online. Association for Computational Linguistics.
- Yu et al. (2018) Xiaodong Yu, Stephen Mayhew, Mark Sammons, and Dan Roth. 2018. On the strength of character language models for multilingual named entity recognition. In Proceedings of the 2018 Conference on Empirical Methods in Natural Language Processing, pages 3073–3077, Brussels, Belgium. Association for Computational Linguistics.
- Zhang et al. (2015) Xiang Zhang, Junbo Zhao, and Yann LeCun. 2015. Character-level convolutional networks for text classification. In Advances in Neural Information Processing Systems, volume 28. Curran Associates, Inc.
Appendix A DRS Parsing Task
DRS parsing is a task to convert natural language sentences into DRS-based meaning representations. In van Noord et al. (2020) and this study, the outputs of the models are clausal forms with relative naming for the variables. See van Noord et al. (2018b) for the further details.
A.1 Evaluation
This study follows micro F1-scores based on matching clauses between predicted and gold DRSs adopted by van Noord et al. (2020). The tool for calculating the values is Counter (van Noord et al., 2018a), which searches for the best mapping of variables between two DRSs and calculates the values based on the number of clauses. Referee (van Noord et al., 2018b) verifies whether an output DRS is well-formed. An output DRS is ill-formed (i.e., not well-formed) when it has illegal clauses or the tool fails to solve variable references.
Appendix B Dataset Settings
We use PMB release 3.0.0 and the same setup as that in the previous study (van Noord et al., 2020). As pre-training datasets, we use a merged set of the gold and the silver training sets for English, a merged set of all training sets (gold, silver, and bronze) for German111We also experiment on the setup described in van Noord et al. (2020). See Appendix E.2, and combined sets of silver and bronze training sets for Dutch and Italian. As datasets for fine-tuning, we use the gold training set for English, a combined set of the gold and silver training sets for German, and the silver training sets for Dutch and Italian. Table 4 shows data statistics of the PMB release 3.0.0.
Gold | Silver | Bronze | |||
---|---|---|---|---|---|
Train | Dev | Test | Train | Train | |
English | 6,620 | 885 | 898 | 97,598 | 146,371 |
German | 1,159 | 417 | 403 | 5,250 | 121,111 |
Dutch | 0 | 529 | 483 | 1,301 | 21,550 |
Italian | 0 | 515 | 547 | 2,772 | 64,305 |
Appendix C Numerical Results
Avg | SE | Min | Max | Avg values per pre-train | |
---|---|---|---|---|---|
Correct | 89.05 | 0.06 | 88.47 | 89.39 | 89.04, 88.95, 89.17 |
SHF-word | 88.80 | 0.09 | 88.03 | 89.34 | 88.80, 88.75, 88.87 |
SHF-sent | 88.75 | 0.09 | 88.04 | 89.20 | 88.79, 88.53, 88.93 |
RND | 88.65 | 0.09 | 88.01 | 89.19 | 88.74, 88.48, 88.74 |
UNI | 88.76 | 0.09 | 88.04 | 89.25 | 88.62, 88.61, 89.05 |
Avg | SE | Min | Max | Avg values per pre-train | |
---|---|---|---|---|---|
Correct | 76.07 | 0.12 | 75.21 | 77.02 | 76.24, 76.24, 75.74 |
SHF-word | 75.68 | 0.13 | 74.76 | 76.75 | 75.69, 75.88, 75.46 |
SHF-sent | 75.07 | 0.13 | 73.90 | 76.09 | 74.89, 75.28, 75.03 |
RND | 74.81 | 0.11 | 74.22 | 75.46 | 74.72, 74.83, 74.88 |
UNI | 75.09 | 0.17 | 74.34 | 76.26 | 75.02, 75.25, 74.99 |
Avg | SE | Min | Max | Avg values per pre-train | |
---|---|---|---|---|---|
Correct | 69.23 | 0.18 | 67.89 | 70.26 | 69.41, 69.33, 68.95 |
SHF-word | 68.69 | 0.13 | 67.70 | 69.60 | 68.94, 68.68, 68.46 |
SHF-sent | 68.82 | 0.13 | 67.95 | 69.68 | 69.31, 68.59, 68.55 |
RND | 67.47 | 0.14 | 66.52 | 68.34 | 67.65, 67.50, 67.26 |
UNI | 65.69 | 0.30 | 63.90 | 67.47 | 65.68, 65.76, 65.64 |
Avg | SE | Min | Max | Avg values per pre-train | |
---|---|---|---|---|---|
Correct | 78.75 | 0.10 | 77.99 | 79.29 | 78.97, 78.53, 78.75 |
SHF-word | 58.84 | 0.20 | 57.59 | 60.30 | 58.74, 58.34, 59.43 |
SHF-sent | 39.37 | 0.42 | 35.22 | 41.78 | 39.37, 38.08, 40.66 |
RND | 39.95 | 0.46 | 35.08 | 42.26 | 39.83, 40.30, 39.73 |
UNI | 26.66 | 1.30 | 18.23 | 34.16 | 28.06, 30.14, 21.77 |
Appendix D Results in English without Semantic Tags
Figure 2 and Table 6 show the results of the 2-enc + char model without semantic tags in English. Compared with 2-enc + char (Figure 1), we can observe slightly larger but minor changes in the averaged F1-scores. Thus, regardless of the existence of semantic tags, our experimental results indicate that the model is not sensitive to the order of character sequences in English.
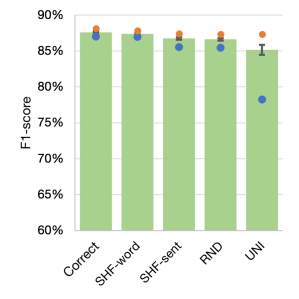
Avg | SE | Min | Max | |
---|---|---|---|---|
Correct | 87.58 | 0.10 | 87.01 | 88.14 |
SHF-word | 87.39 | 0.08 | 86.97 | 87.84 |
SHF-sent | 86.73 | 0.16 | 85.54 | 87.40 |
RND | 86.61 | 0.17 | 85.48 | 87.34 |
UNI | 85.15 | 0.70 | 78.25 | 87.34 |
Appendix E Additional Analysis
E.1 Score change by character-level information per case
We look at the performance changes in individual cases. Figure 3 shows scatter diagrams of the four languages. In these diagrams, we plot the averaged F1-score changes of 15 runs by adding (i.e., from no char to unigrams) and extending (i.e., from unigrams to bigrams) character-level information. We observe many cases whose averaged F1-score increases with the addition and extension of character-level information (plotted in the first quadrant). However, these numbers are lower than those in the second and fourth quadrants, indicating that the improvement works only by either adding or extending the information. Moreover, we observed cases whose scores decrease in both aspects, plotted in the third quadrant. These trends are observed for all languages, even though the overall scores improved for all languages except English.
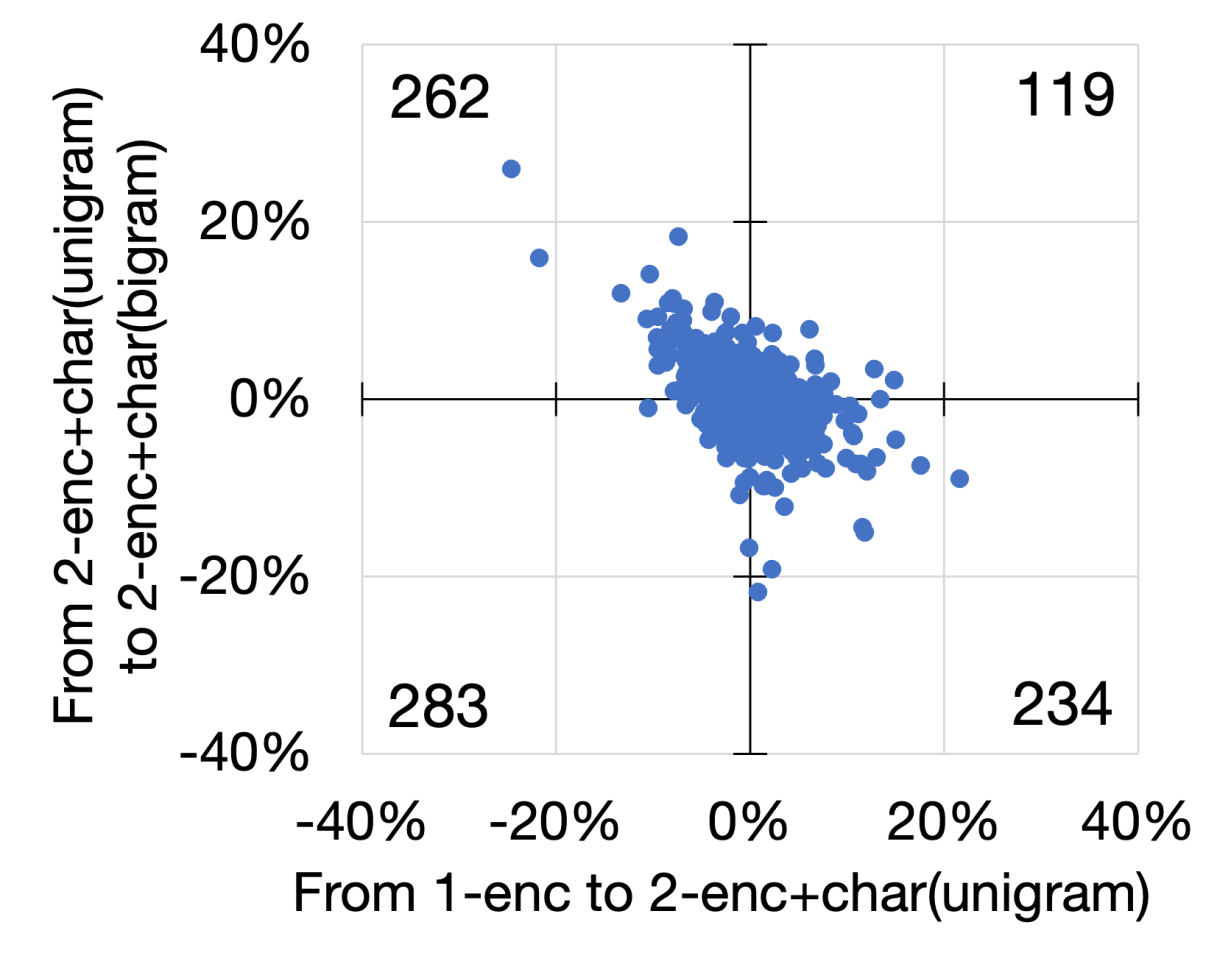
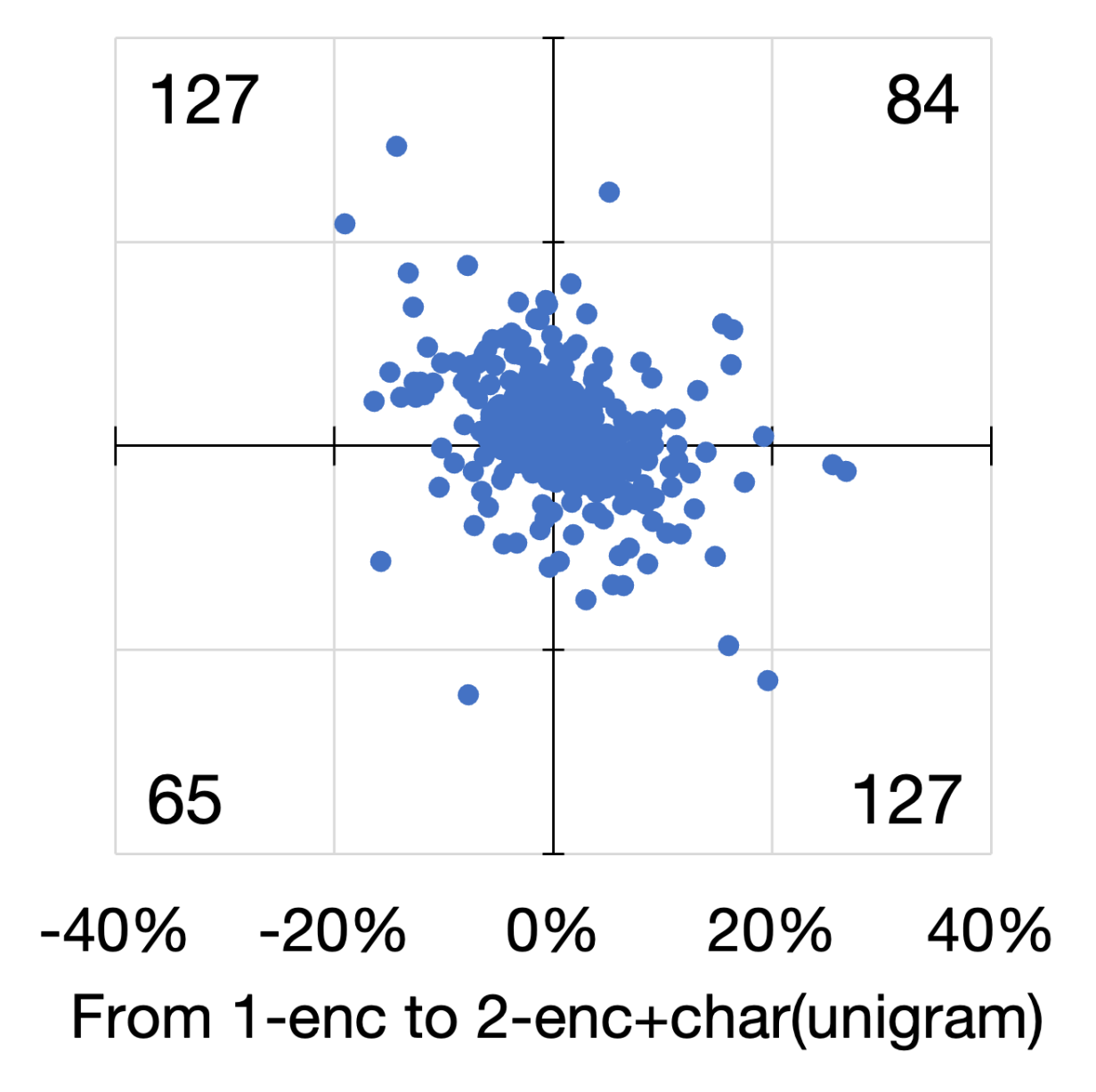
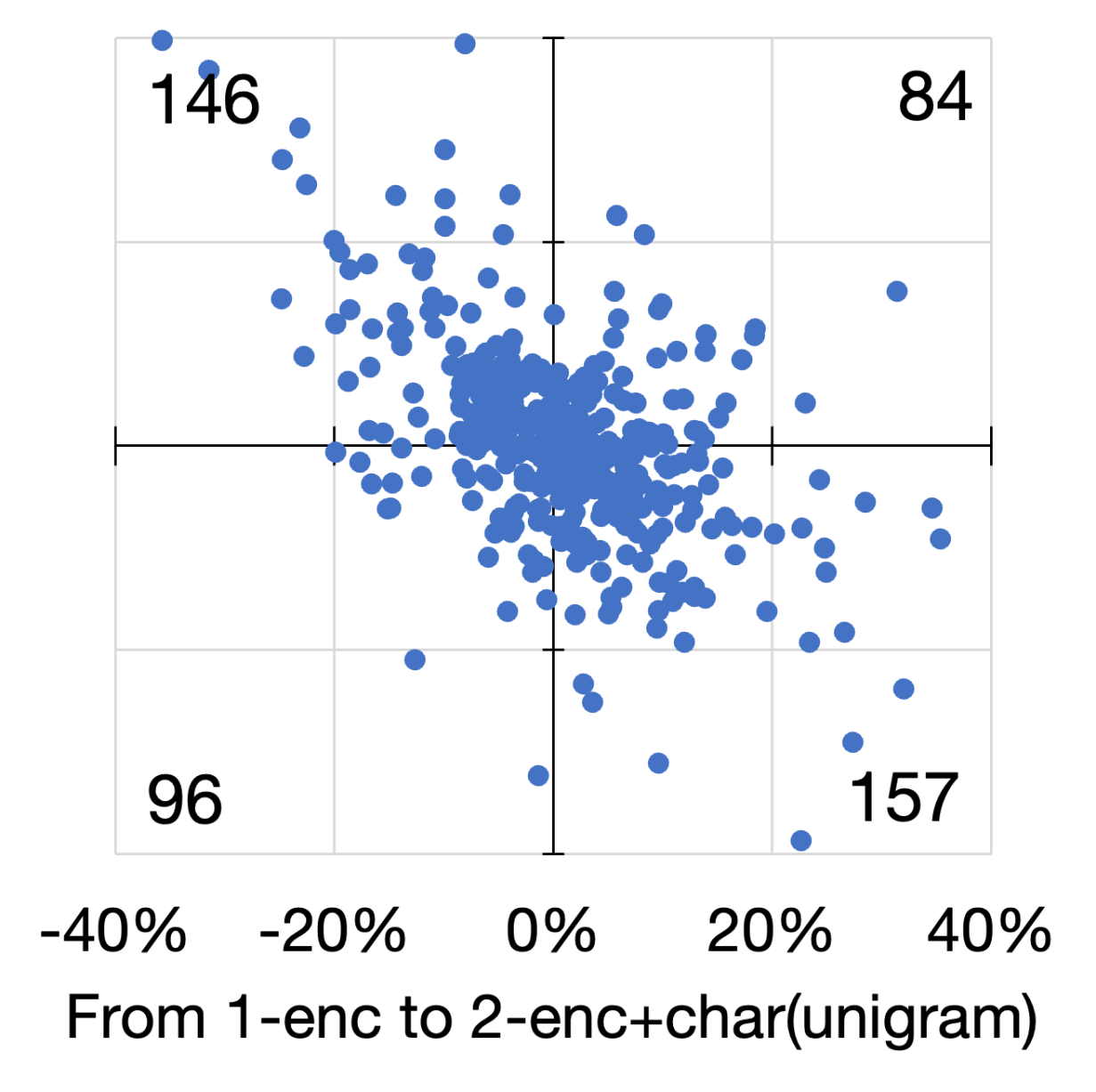
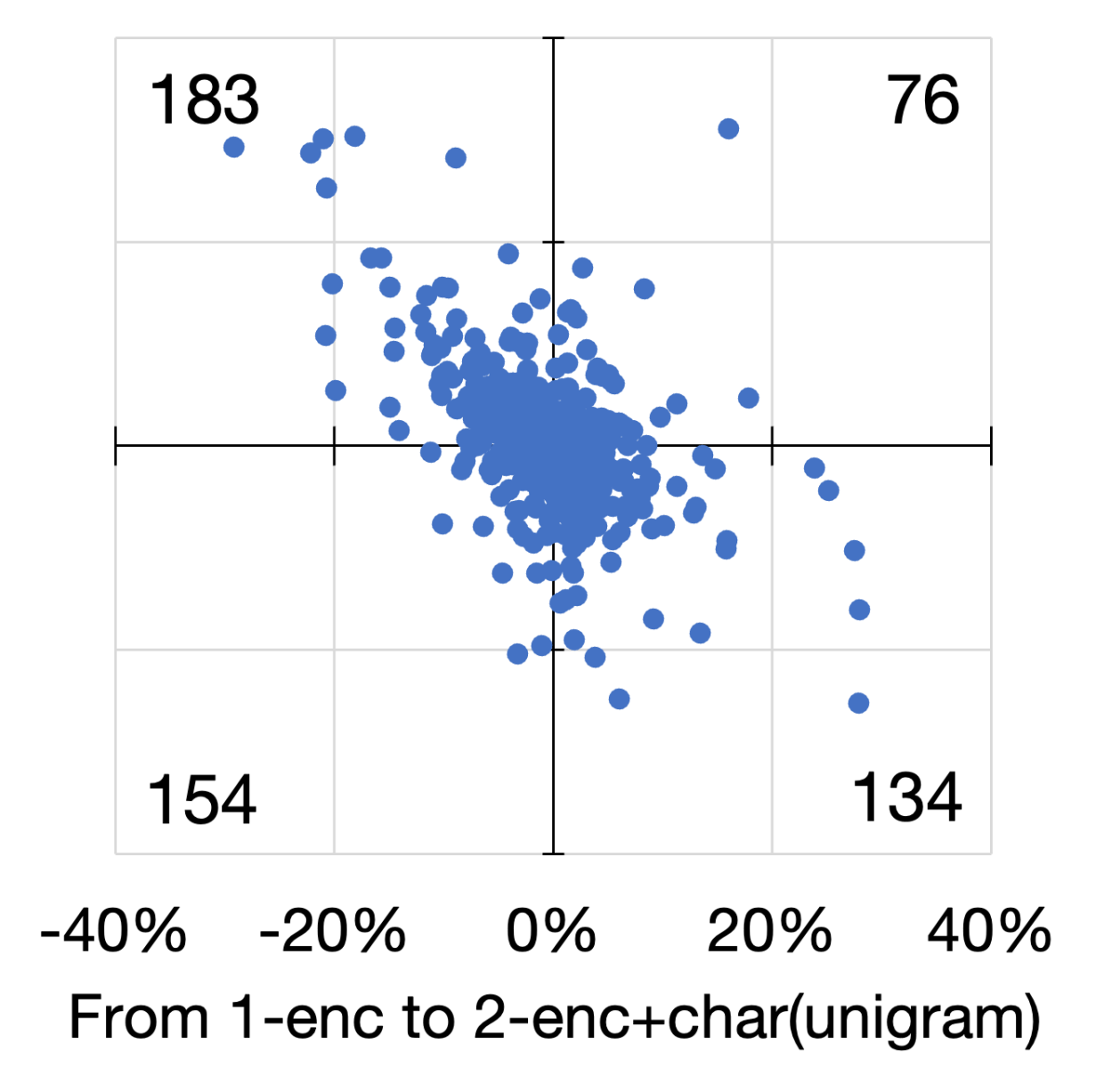
E.2 Why do our values deviate from van Noord et al. (2020)?
The values reported in this study are lower than those from the previous study van Noord et al. (2020), especially in German. We follow nearly all the setups reported in van Noord et al. (2020), but the values are still low.
van Noord et al. (2020) reports that they only used the gold and silver data if gold (train) data is available in a certain language. The German data in PMB release 3.0.0 has the gold train data comprising 1,159 documents. Therefore, we experiment with the model pre-trained on the merged set of the gold and silver data and fine-tuned on the gold data only. We reported an averaged value of five runs in Table 7 with one from van Noord et al. (2020). A large deviation between the two F1-scores can be observed.
Average | All values | |
---|---|---|
van Noord et al. (2020) | 82.0 | N/A |
Our replication | 68.52 | 68.54, 67.95, 69.38, 68.61, 68.10 |