COVIDx CT-3: A Large-scale, Multinational, Open-Source Benchmark Dataset for Computer-aided COVID-19 Screening from Chest CT Images
Abstract
Computed tomography (CT) has been widely explored as a COVID-19 screening and assessment tool to complement RT-PCR testing. To assist radiologists with CT-based COVID-19 screening, a number of computer-aided systems have been proposed. However, many proposed systems are built using CT data which is limited in both quantity and diversity. Motivated to support efforts in the development of machine learning-driven screening systems, we introduce COVIDx CT-3, a large-scale multinational benchmark dataset for detection of COVID-19 cases from chest CT images. COVIDx CT-3 includes 431,205 CT slices from 6,068 patients across at least 17 countries, which to the best of our knowledge represents the largest, most diverse dataset of COVID-19 CT images in open-access form. Additionally, we examine the data diversity and potential biases of the COVIDx CT-3 dataset, finding that significant geographic and class imbalances remain despite efforts to curate data from a wide variety of sources.
1 Introduction
CT has shown great potential for detection of COVID-19, and in particular machine learning-based systems for detection of COVID-19 from CT images have been widely explored. Machine learning techniques allow for the visual characteristics of COVID-19 to be learned directly from CT images, which may aid clinicians in differentiating COVID-19 pneumonia from pneumonia of other etiology. However, even the largest studies in research literature have been limited in terms of quantity and/or diversity of patients, with many limited to single-nation cohorts [1, 2, 3, 4, 5, 6, 7, 8, 9, 10]. Multinational patient cohorts have been leveraged in several studies, but have typically been limited to few patients or few countries[11, 12, 13]. In this study, we introduce COVIDx CT-3, a large-scale multinational benchmark dataset for detection of COVID-19 cases from chest CT images comprising 431,205 CT slices from 6,068 patients across at least 17 countries. COVIDx CT-3 builds upon COVIDx CT-2 [14], and includes more than twice as many images. Additionally, we examine the data diversity and potential biases of the COVIDx CT-3 dataset, finding that significant geographic and class imbalances remain despite efforts to curate data from a wide variety of CT data sources.
2 Methods
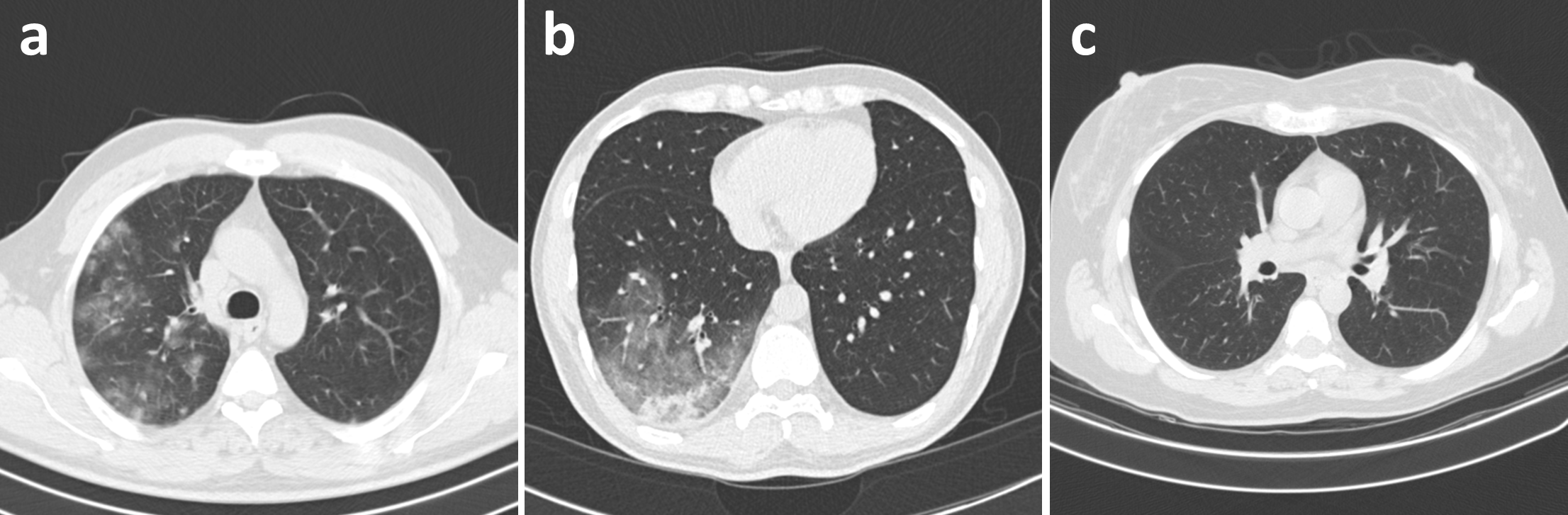
In this study, we carefully processed and curated CT images from several data sources from around the world which were collected using a variety of CT scanners and protocols. The resulting patient cohort consists of patient cases collected by the following organizations and initiatives: (1) China National Center for Bioinformation (CNCB) [3], (2) National Institutes of Health Intramural Targeted Anti-COVID-19 (ITAC) Program [15, 16], (3) COVID-CTset [4], (4) Integrative CT Images and Clinical Features for COVID-19 (iCTCF) [5], (5) COVID-19 CT Lung and Infection Segmentation initiative (COVID-19-CT-Seg) [17], (6) Lung Image Database Consortium and Image Database Resource Initiative (LIDC-IDRI) [16, 18, 19], (7) Radiopaedia collection [20], (8) MosMedData [21], (9) Stony Brook University [16, 22], (10) Study of Thoracic CT in COVID-19 (STOIC) [10], and (11) COVID-CT-MD [23].
Each patient is associated with one of three possible infection types: (a) COVID-19, (b) community-acquired pneumonia (CAP), or (c) normal controls, with Figure 1 illustrating an example of each infection type. For CT volumes which were not labelled at the image level, labels were obtained in one of three ways: (1) segmentation-based labelling, where ground-truth infection masks were used to identify abnormal CT slices, (2) non-expert manual labelling, where non-experts manually labelled CT slices with obvious abnormalities, or (3) model-based automatic labelling, where a pre-trained model [14] was used to identify CT slices with high CAP or COVID-19 confidence. For all three methods, the selected CT slices were assigned the same labels as their respective patients.
3 Results
3.1 Demographics
Table 1 shows the demographics of the COVIDx CT-3 dataset. Examining the countries of origin, we see that Chinese patients dominate the data, accounting for 42.2% of the patient cohort. Additionally, we see that the vast majority of patients (85.9%) were imaged in one of four countries (China, France, Russia, or Iran), illustrating a severe geographical bias towards Asian and European patients. Additionally, we see that age and sex are unknown for more than half of the patient cohort. Of the patients with known ages, the majority (94.3%, or 45.9% of all patients) fall within the age range 30-89, indicating that young patients ( 29) and very old patients ( 90) may be underrepresented. Of the patients with known sexes, there is a reasonably even split between male and female patients (27.0% and 22.1%, respectively).
Country | Age | ||||
Unknown | 702 (11.6%) | Scotland | 1 (0.02%) | Unknown | 3120 (51.4%) |
China | 2563 (42.2%) | Peru | 1 (0.02%) | 0-9 | 17 (0.3%) |
France | 1176 (19.4%) | Lebanon | 1 (0.02%) | 10-19 | 24 (0.4%) |
Russia | 756 (12.5%) | England | 1 (0.02%) | 20-29 | 114 (1.9%) |
Iran | 718 (11.8%) | Turkey | 1 (0.02%) | 30-39 | 428 (7.1%) |
USA | 129 (2.1%) | Belgium | 1 (0.02%) | 40-49 | 467 (7.7%) |
Australia | 7 (0.12%) | Azerbaijan | 1 (0.02%) | 50-59 | 576 (9.5%) |
Algeria | 5 (0.08%) | Afghanistan | 1 (0.02%) | 60-69 | 606 (10.0%) |
Italy | 3 (0.05%) | Ukraine | 1 (0.02%) | 70-79 | 403 (6.6%) |
Sex | 80-89 | 302 (5.0%) | |||
Unknown | 3091 (50.9%) | 90-99 | 11 (0.2%) | ||
Male | 1639 (27.0%) | ||||
Female | 1338 (22.1%) |
3.2 Data Splits
Table 2 shows the distribution of CT slices and patients amongst the training, validation and test sets. The data is split into training, validation, and test sets with fractions of approximately 84%, 8%, and 8%, respectively. This is due to the fact that data labelled automatically or by non-experts is included solely in the training set, while the validation and test sets were both labelled manually by experts. Additionally, we observe a class imbalance where COVID-19 images represent 73.4% of the data and normal and pneumonia images represent 16.6% and 10.0% of the data, respectively. Notably, this imbalance is primarily seen in the training set, while the validation and test sets both have ratios of approximately 2.2:1:1 between Normal, CAP, and COVID-19 images, respectively.
Infection Type | ||||
Data Split | Normal | CAP | COVID-19 | Total |
Training | 35,996 (321) | 26,970 (592) | 300,733 (4,092) | 363,699 (5,005) |
Validation | 17,570 (164) | 8,008 (202) | 8,147 (194) | 33,725 (560) |
Test | 17,922 (164) | 7,965 (138) | 7,894 (201) | 33,781 (503) |
Total | 71,488 (649) | 42,943 (932) | 316,774 (4,487) | 431,205 (6,068) |
3.3 Benchmarks
Table 3 provides performance measures for a variety of network architectures on the COVIDx CT-3 test dataset, as previously presented in [14]. These results provide reference performance targets for new networks trained on the COVIDx CT-3 dataset.
Network | Param. (M) | FLOPs (G) | Acc. (%) | Sens. (%) | PPV (%) |
---|---|---|---|---|---|
SqueezeNet [24] | 0.74 | 8.09 | 98.7 | 97.7 | 98.1 |
MobileNetV2 [25] | 2.23 | 3.33 | 99.0 | 98.5 | 98.0 |
EfficientNet-B0 [26] | 4.05 | 4.07 | 99.0 | 99.1 | 98.0 |
NASNet-A-Mobile [27] | 4.29 | 5.94 | 98.8 | 98.7 | 96.9 |
COVID-Net CT L [2] | 1.40 | 4.18 | 98.4 | 98.1 | 96.1 |
COVID-Net CT S [14] | 0.45 | 1.94 | 98.3 | 97.3 | 96.3 |
4 Discussion
Given the demographic imbalances of the COVIDx CT-3 dataset, algorithms and strategies accounting for such imbalances (e.g., balanced loss functions, data sampling and re-balancing methods, etc.) may be an interesting area of exploration. Additionally, due to the large number of patients with unknown ages or sexes, it is difficult to ascertain the true age and sex distributions.
Systems trained on the COVIDx CT-3 dataset may require careful re-balancing of the classes in order to address the class imbalance in the training set. Additionally, evaluation of such systems should take into account the imbalances in the validation and test sets by examining balanced metrics, such as per-class precision and recall.
Potential Negative Societal Impact
While the motivation behind the release of this large-scale benchmark dataset is to support researchers, clinicians, and citizen data scientists in advancing this field, a potential negative societal impact from this release of is the misuse of the collected data. More specifically, misuse of collected data can occur if the benchmark dataset is used to build machine learning algorithms for the purpose of forecasting future medical expenses for individual patients, which insurance companies may use to adjust insurance premiums.
Furthermore, use of this dataset to build predictive models for clinical use can have a negative impact if such models are not properly validated. In particular, the use of automated and non-expert labelling methods in the construction of this dataset inevitably introduces a degree of label noise which may affect the performance and behaviour of any developed models. To avoid this, models built using this dataset should be validated on real-world clinical data, should not be used in clinical settings without expert oversight, and should not be used to make final diagnostic decisions.
Acknowledgements
We would like to thank the Canada Research Chairs program and the the Natural Sciences and Engineering Research Council of Canada (NSERC).
References
- Mei et al. [2020] Xueyan Mei, Hao-Chih Lee, Kai-yue Diao, Mingqian Huang, Bin Lin, Chenyu Liu, Zongyu Xie, Yixuan Ma, Philip Robson, Michael Chung, Adam Bernheim, Venkatesh Mani, Claudia Calcagno, Kunwei Li, Shaolin Li, Hong Shan, Jian Lv, Tongtong Zhao, Junli Xia, and Yang Yang. Artificial intelligence–enabled rapid diagnosis of patients with covid-19. Nature Medicine, 26:1224–1228, 2020. doi: 10.1038/s41591-020-0931-3.
- Gunraj et al. [2020] Hayden Gunraj, Linda Wang, and Alexander Wong. COVIDNet-CT: A tailored deep convolutional neural network design for detection of COVID-19 cases from chest CT images. Frontiers in Medicine, 7:1025, 2020. doi: 10.3389/fmed.2020.608525.
- Zhang et al. [2020] Kang Zhang, Xiaohong Liu, Jun Shen, Zhihuan Li, Ye Sang, Xingwang Wu, Yunfei Zha, Wenhua Liang, Chengdi Wang, Ke Wang, Linsen Ye, Ming Gao, Zhongguo Zhou, Liang Li, Jin Wang, Zehong Yang, Huimin Cai, Jie Xu, Lei Yang, Wenjia Cai, Wenqin Xu, Shaoxu Wu, Wei Zhang, Shanping Jiang, Lianghong Zheng, Xuan Zhang, Li Wang, Liu Lu, Jiaming Li, Haiping Yin, Winston Wang, Oulan Li, Charlotte Zhang, Liang Liang, Tao Wu, Ruiyun Deng, Kang Wei, Yong Zhou, Ting Chen, Johnson Yiu-Nam Lau, Manson Fok, Jianxing He, Tianxin Lin, Weimin Li, and Guangyu Wang. Clinically applicable AI system for accurate diagnosis, quantitative measurements, and prognosis of covid-19 pneumonia using computed tomography. Cell, 18(6):1423–1433, 2020. doi: 10.1016/j.cell.2020.04.045.
- Rahimzadeh et al. [2020] Mohammad Rahimzadeh, Abolfazl Attar, and Seyed Mohammad Sakhaei. A fully automated deep learning-based network for detecting COVID-19 from a new and large lung CT scan dataset. medRxiv, 2020. doi: 10.1101/2020.06.08.20121541. URL https://www.medrxiv.org/content/early/2020/06/12/2020.06.08.20121541.
- Ning et al. [2020] Wanshan Ning, Shijun Lei, Jingjing Yang, Yukun Cao, Peiran Jiang, Qianqian Yang, Jiao Zhang, Xiaobei Wang, Fenghua Chen, Zhi Geng, Liang Xiong, Hongmei Zhou, Yaping Guo, Yulan Zeng, Heshui Shi, Lin Wang, Yu Xue, and Zheng Wang. Open resource of clinical data from patients with pneumonia for the prediction of COVID-19 outcomes via deep learning. Nature Biomedical Engineering, 4:1197–1207, 2020. doi: 10.1038/s41551-020-00633-5.
- Xu et al. [2020] Xiaowei Xu, Xiangao Jiang, Chunlian Ma, Peng Du, Xukun Li, Shuangzhi Lv, Liang Yu, Qin Ni, Yanfei Chen, Junwei Su, Guanjing Lang, Yongtao Li, Hong Zhao, Jun Liu, Kaijin Xu, Lingxiang Ruan, Jifang Sheng, Yunqing Qiu, Wei Wu, Tingbo Liang, and Lanjuan Li. A deep learning system to screen novel coronavirus disease 2019 pneumonia. Engineering, 6(10):1122–1129, 2020. doi: 10.1016/j.eng.2020.04.010.
- Ardakani et al. [2020] Ali Abbasian Ardakani, Alireza Rajabzadeh Kanafi, U. Rajendra Acharya, Nazanin Khadem, and Afshin Mohammadi. Application of deep learning technique to manage covid-19 in routine clinical practice using ct images: Results of 10 convolutional neural networks. Computers in Biology and Medicine, 121:103795, 2020. ISSN 0010-4825. doi: 10.1016/j.compbiomed.2020.103795.
- Javaheri et al. [2021] Tahereh Javaheri, Morteza Homayounfar, Zohreh Amoozgar, Reza Reiazi, Fatemeh Homayounieh, Engy Abbas, Azadeh Laali, Amir Reza Radmard, Mohammad Hadi Gharib, Seyed Ali Javad Mousavi, Omid Ghaemi, Rosa Babaei, Hadi Karimi Mobin, Mehdi Hosseinzadeh, Rana Jahanban-Esfahlan, Khaled Seidi, Mannudeep K. Kalra, Guanglan Zhang, L. T. Chitkushev, Benjamin Haibe-Kains, Reza Malekzadeh, and Reza Rawassizadeh. CovidCTNet: an open-source deep learning approach to diagnose covid-19 using small cohort of CT images. NPJ Digital Medicine, 4(1):29, Feb. 2021.
- Wu et al. [2020] Xiangjun Wu, Hui Hui, Meng Niu, Liang Li, Li Wang, Bingxi He, Xin Yang, Li Li, Hongjun Li, Jie Tian, and Yunfei Zha. Deep learning-based multi-view fusion model for screening 2019 novel coronavirus pneumonia: A multicentre study. European Journal of Radiology, 128:109041, Jul. 2020.
- Revel et al. [2021] Marie-Pierre Revel, Samia Boussouar, Constance de Margerie-Mellon, Inès Saab, Thibaut Lapotre, Dominique Mompoint, Guillaume Chassagnon, Audrey Milon, Mathieu Lederlin, Souhail Bennani, Sébastien Molière, Marie-Pierre Debray, Florian Bompard, Severine Dangeard, Chahinez Hani, Mickaël Ohana, Sébastien Bommart, Carole Jalaber, Mostafa El Hajjam, Isabelle Petit, Laure Fournier, Antoine Khalil, Pierre-Yves Brillet, Marie-France Bellin, Alban Redheuil, Laurence Rocher, Valérie Bousson, Pascal Rousset, Jules Grégory, Jean-François Deux, Elisabeth Dion, Dominique Valeyre, Raphael Porcher, Léa Jilet, and Hendy Abdoul. Study of thoracic CT in COVID-19: The STOIC project. Radiology, 301(1):E361–E370, 2021. doi: 10.1148/radiol.2021210384. PMID: 34184935.
- Hasan et al. [2020] Ali M. Hasan, Mohammed M. AL-Jawad, Hamid A. Jalab, Hadil Shaiba, Rabha W. Ibrahim, and Ala’a R. AL-Shamasneh. Classification of COVID-19 coronavirus, pneumonia and healthy lungs in CT scans using Q-deformed entropy and deep learning features. Entropy, 22(5), 2020. doi: 10.3390/e22050517.
- Harmon et al. [2020] Stephanie A. Harmon, Thomas H. Sanford, et al. Artificial intelligence for the detection of covid-19 pneumonia on chest ct using multinational datasets. Nature Communications, 11(4080), 2020. doi: 10.1038/s41467-020-17971-2.
- Jin et al. [2020] Cheng Jin, Weixiang Chen, Yukun Cao, Zhanwei Xu, Zimeng Tan, Xin Zhang, Lei Deng, Chuansheng Zheng, Jie Zhou, Heshui Shi, and Jianjiang Feng. Development and evaluation of an ai system for covid-19 diagnosis. medRxiv, 2020. doi: 10.1101/2020.03.20.20039834.
- Gunraj et al. [2022] Hayden Gunraj, Ali Sabri, David Koff, and Alexander Wong. COVID-Net CT-2: Enhanced deep neural networks for detection of COVID-19 from chest CT images through bigger, more diverse learning. Frontiers in Medicine, 8:729287, 2022. doi: 10.3389/fmed.2021.729287.
- An et al. [2020] Peng An, Sheng Xu, Stephanie A. Harmon, Evrim B. Turkbey, Thomas H. Sanford, Amel Amalou, Michael Kassin, Nicole Varble, Maxime Blain, Victoria Anderson, Francesca Patella, Gianpaolo Carrafiello, Baris Turkbey, and Bradford J. Wood. CT images in COVID-19 [data set]. The Cancer Imaging Archive, 2020. URL https://doi.org/10.7937/tcia.2020.gqry-nc81.
- Clark et al. [2013] Kenneth Clark, Bruce Vendt, Kirk Smith, John Freymann, Justin Kirby, Paul Koppel, Stephen Moore, Stanley Phillips, David Maffitt, Michael Pringle, Lawrence Tarbox, , and Fred Prior. The Cancer Imaging Archive (TCIA): maintaining and operating a public information repository. Journal of Digital Imaging, 26(6):1045–1057, 2013.
- Ma et al. [2021] Jun Ma, Yixin Wang, Xingle An, Cheng Ge, Ziqi Yu, Jianan Chen, Qiongjie Zhu, Guoqiang Dong, Jian He, Zhiqiang He, Tianjia Cao, Yuntao Zhu, Ziwei Nie, and Xiaoping Yang. Toward data-efficient learning: A benchmark for COVID-19 CT lung and infection segmentation. Medical Physics, 48(3):1197–1210, 2021. doi: 10.1002/mp.14676. URL https://aapm.onlinelibrary.wiley.com/doi/abs/10.1002/mp.14676.
- III et al. [2015] Samuel G Armato III, Geoffrey McLennan, Luc Bidaut, Michael F. McNitt-Gray, Charles R. Meyer, Anthony P. Reeves, Binsheng Zhao, Denise R. Aberle, Claudia I. Henschke, Eric A. Hoffman, Ella A. Kazerooni, Heber MacMahon, Edwin J. R. van Beeke, David Yankelevitz, Alberto M. Biancardi, Peyton H. Bland, Matthew S. Brown, Roger M. Engelmann, Gary E. Laderach, Daniel Max, Richard C. Pais, David P. Y. Qing, Rachael Y. Roberts, Amanda R. Smith, Adam Starkey, Poonam Batrah, Philip Caligiuri, Ali Farooqi, Gregory W. Gladish, C. Matilda Jude, Reginald F. Munden, Iva Petkovska, Leslie E. Quint, Lawrence H. Schwartz, Baskaran Sundaram, Lori E. Dodd, Charles Fenimore, David Gur, Nicholas Petrick, John Freymann, Justin Kirby, Brian Hughes, Alessi Vande Casteele, Sangeeta Gupte, Maha Sallamm, Michael D. Heath, Michael H. Kuhn, Ekta Dharaiya, Richard Burns, David S. Fryd, Marcos Salganicoff, Vikram Anand, Uri Shreter, Stephen Vastagh, Barbara Y. Croft, and Laurence P. Clarke. Data from LIDC-IDRI [data set]. The Cancer Imaging Archive, 2015. doi: 10.7937/K9/TCIA.2015.LO9QL9SX.
- III et al. [2011] Samuel G Armato III, Geoffrey McLennan, Luc Bidaut, Michael F. McNitt-Gray, Charles R. Meyer, Anthony P. Reeves, Binsheng Zhao, Denise R. Aberle, Claudia I. Henschke, Eric A. Hoffman, Ella A. Kazerooni, Heber MacMahon, Edwin J. R. van Beeke, David Yankelevitz, Alberto M. Biancardi, Peyton H. Bland, Matthew S. Brown, Roger M. Engelmann, Gary E. Laderach, Daniel Max, Richard C. Pais, David P. Y. Qing, Rachael Y. Roberts, Amanda R. Smith, Adam Starkey, Poonam Batrah, Philip Caligiuri, Ali Farooqi, Gregory W. Gladish, C. Matilda Jude, Reginald F. Munden, Iva Petkovska, Leslie E. Quint, Lawrence H. Schwartz, Baskaran Sundaram, Lori E. Dodd, Charles Fenimore, David Gur, Nicholas Petrick, John Freymann, Justin Kirby, Brian Hughes, Alessi Vande Casteele, Sangeeta Gupte, Maha Sallamm, Michael D. Heath, Michael H. Kuhn, Ekta Dharaiya, Richard Burns, David S. Fryd, Marcos Salganicoff, Vikram Anand, Uri Shreter, Stephen Vastagh, and Barbara Y. Croft. The Lung Image Database Consortium (LIDC) and Image Database Resource Initiative (IDRI): a completed reference database of lung nodules on CT scans. Medical Physics, 38(2):915–931, 2011.
- [20] COVID-19. Radiopaedia. URL https://radiopaedia.org/articles/covid-19-4.
- Morozov et al. [2020] Sergey P. Morozov, Anna E. Andreychenko, Ivan A. Blokhin, Pavel B. Gelezhe, Anna P. Gonchar, Alexander E. Nikolaev, Nikolay A. Pavlov, Valeria Yu. Chernina, and Victor A. Gombolevskiy. MosMedData: data set of 1110 chest CT scans performed during the COVID-19 epidemic. Digital Diagnostics, 1(1):49–59, 2020.
- Saltz et al. [2021] Joel Saltz, Mary Saltz, Prateek Prasanna, Richard Moffitt, Janos Hajagos, Erich Bremer, Joseph Balsamo, and Tahsin Kurc. Stony Brook University COVID-19 positive cases [data set]. The Cancer Imaging Archive, 2021. doi: 10.7937/TCIA.BBAG-2923.
- Afshar et al. [2021] Parnian Afshar, Shahin Heidarian, Nastaran Enshaei, Farnoosh Naderkhani, Moezedin Javad Rafiee, Anastasia Oikonomou, Faranak Babaki Fard, Kaveh Samimi, Konstantinos N. Plataniotis, and Arash Mohammadi. COVID-CT-MD, COVID-19 computed tomography scan dataset applicable in machine learning and deep learning. Scientific Data, 8(1):121, 2021.
- Iandola et al. [2016] Forrest N. Iandola, Song Han, Matthew W. Moskewicz, Khalid Ashraf, William J. Dally, and Kurt Keutzer. Squeezenet: Alexnet-level accuracy with 50x fewer parameters and <0.5mb model size. 2016.
- Sandler et al. [2018] Mark Sandler, Andrew Howard, Menglong Zhu, Andrey Zhmoginov, and Liang-Chieh Chen. MobileNetV2: Inverted residuals and linear bottlenecks. In 2018 IEEE/CVF Conference on Computer Vision and Pattern Recognition, pages 4510–4520, 2018. doi: 10.1109/CVPR.2018.00474.
- Tan and Le [2019] Mingxing Tan and Quoc Le. EfficientNet: Rethinking Model Scaling for Convolutional Neural Networks. In 2019 International Conference on Machine Learning (ICML), 2019.
- Zoph et al. [2018] B. Zoph, V. Vasudevan, J. Shlens, and Q. V. Le. Learning Transferable Architectures for Scalable Image Recognition. In 2018 IEEE/CVF Conference on Computer Vision and Pattern Recognition, pages 8697–8710, 2018. doi: 10.1109/CVPR.2018.00907.