Controllable Text-to-3D Generation via Surface-Aligned Gaussian Splatting
Abstract
While text-to-3D and image-to-3D generation tasks have received considerable attention, one important but under-explored field between them is controllable text-to-3D generation, which we mainly focus on in this work. To address this task, 1) we introduce Multi-view ControlNet (MVControl), a novel neural network architecture designed to enhance existing pre-trained multi-view diffusion models by integrating additional input conditions, such as edge, depth, normal, and scribble maps. Our innovation lies in the introduction of a conditioning module that controls the base diffusion model using both local and global embeddings, which are computed from the input condition images and camera poses. Once trained, MVControl is able to offer 3D diffusion guidance for optimization-based 3D generation. And, 2) we propose an efficient multi-stage 3D generation pipeline that leverages the benefits of recent large reconstruction models and score distillation algorithm. Building upon our MVControl architecture, we employ a unique hybrid diffusion guidance method to direct the optimization process. In pursuit of efficiency, we adopt 3D Gaussians as our representation instead of the commonly used implicit representations. We also pioneer the use of SuGaR, a hybrid representation that binds Gaussians to mesh triangle faces. This approach alleviates the issue of poor geometry in 3D Gaussians and enables the direct sculpting of fine-grained geometry on the mesh. Extensive experiments demonstrate that our method achieves robust generalization and enables the controllable generation of high-quality 3D content. The source code is available at our website: https://lizhiqi49.github.io/MVControl.
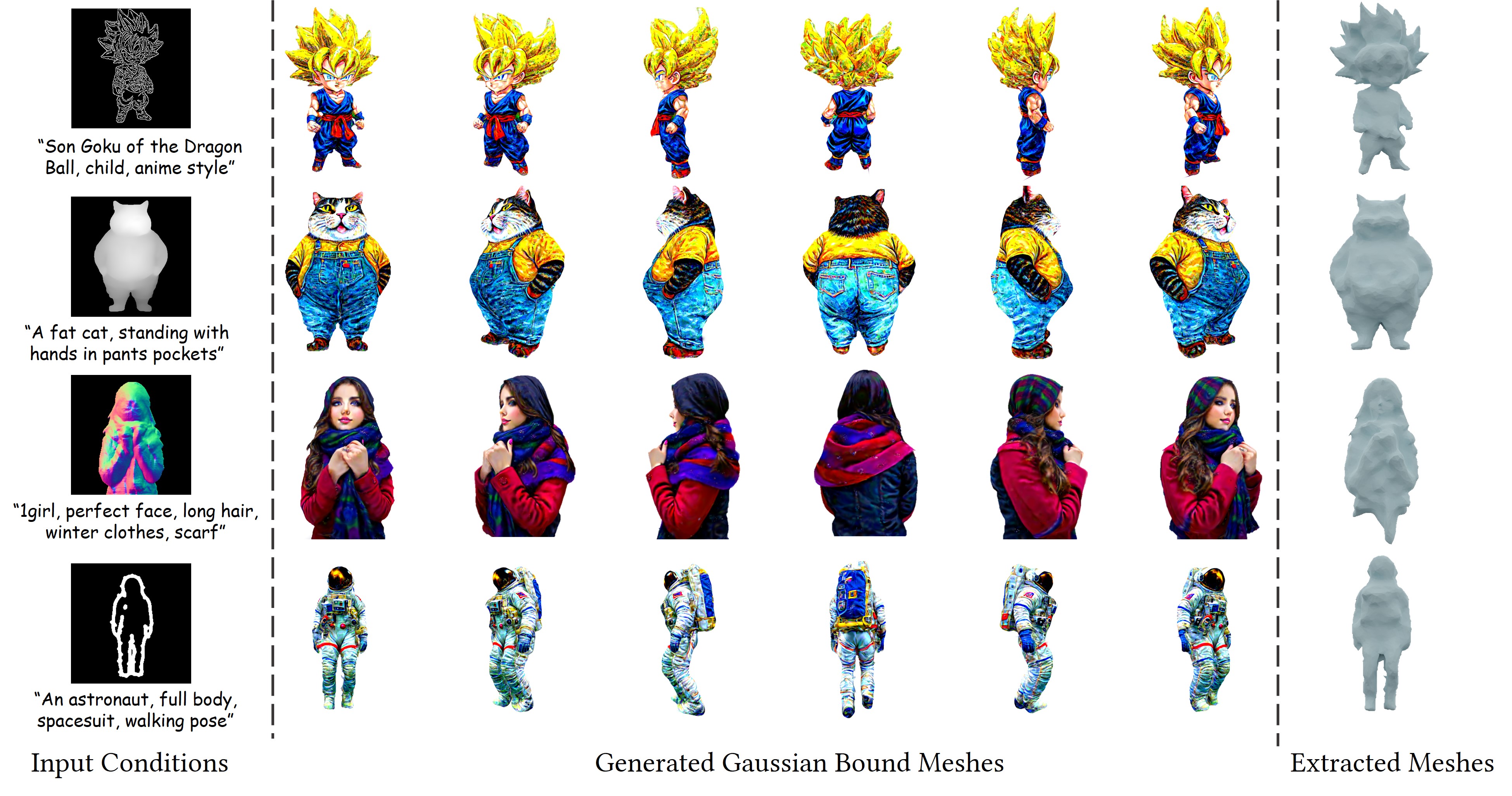
1 Introduction
Remarkable progress has recently been achieved in the field of 2D image generation, which has subsequently propelled research in 3D generation tasks. This progress is attributed to the favorable properties of image diffusion models [44, 30] and differentiable 3D representations [37, 59, 48, 24]. In particular, recent methods based on score distillation optimization (SDS) [41] have attempted to distill 3D knowledge from pre-trained large text-to-image generative models [44, 30, 49], leading to impressive results [41, 28, 55, 11, 36, 62, 54].
Several approaches aim to enhance generation quality, such as applying multiple optimization stages [28, 11], optimizing the diffusion prior with 3D representations simultaneously [62, 51], refining score distillation algorithms [22, 67], and improving pipeline details [20, 4, 69]. Another focus is on addressing view-consistency issues by incorporating multi-view knowledge into pre-trained diffusion models [30, 49, 31, 26, 42, 33]. However, achieving high-quality 3D assets often requires a combination of these techniques, which can be time-consuming. To mitigate this, recent work aims to train 3D generation networks to produce assets rapidly [39, 21, 8, 19, 25, 60, 56]. While efficient, these methods often produce lower quality and less complex shapes due to limitations in training data.
While many works focus on text- or image-to-3D tasks, an important yet under-explored area lies in controllable text-to-3D generation—a gap that this work aims to address. In this work, we propose a new highly efficient controllable 3D generation pipeline that leverages the advantages of both lines of research mentioned in the previous paragraph. Motivated by the achievements of 2D ControlNet [68], an integral component of Stable-Diffusion [44], we propose MVControl, a multi-view variant. Given the critical role of multi-view capabilities in 3D generation, MVControl is designed to extend the success of 2D ControlNet into the multi-view domain. We adopt MVDream [49], a newly introduced multi-view diffusion network, as our foundational model. MVControl is subsequently crafted to collaborate with this base model, facilitating controllable text-to-multi-view image generation. Similar to the approach in [68], we freeze the weights of MVDream and solely focus on training the MVControl component. However, the conditioning mechanism of 2D ControlNet, designed for single image generation, does not readily extend to the multi-view scenario, making it challenging to achieve view-consistency by directly applying its control network to interact with the base model. Additionally, MVDream is trained on an absolute camera system conflicts with the practical need for relative camera poses in our application scenario. To address these challenges, we introduce a simple yet effective conditioning strategy.
After training MVControl, we can leverage it to establish 3D priors for controllable text-to-3D asset generation. To address the extended optimization times of SDS-based methods, which can largely be attributed to the utilization of NeRF[37]-based implicit representations, we propose employing a more efficient explicit 3D representation, 3D Gaussian[24]. Specifically, we propose a multi-stage pipeline for handling textual prompts and condition images: 1) Initially, we employ our MVControl to generate four multi-view images, which are then inputted into LGM[56], a recently introduced large Gaussian reconstruction model. This step yields a set of coarse 3D Gaussians. 2) Subsequently, the coarse Gaussians undergo optimization using a hybrid diffusion guidance approach, combining our MVControl with a 2D diffusion model. We introduce SuGaR [17] regularization terms in this stage to improve the Gaussians’ geometry. 3) The optimized Gaussians are then transformed into a coarse Gaussian-bound mesh, for further refinement of both texture and geometry. Finally, a high-quality textured mesh is extracted from the refined Gaussian-bound mesh.
In summary, our main contributions are as follows:
-
•
We introduce a novel network architecture designed for controllable fine-grain text-to-multi-view image generation. The model is evaluated across various condition types (edge, depth, normal, and scribble), demonstrating its generalization capabilities;
-
•
We develop a multi-stage yet efficient 3D generation pipeline that combines the strengths of large reconstruction models and score distillation. This pipeline optimizes a 3D asset from coarse Gaussians to SuGaR, culminating in a mesh. Importantly, we are the first to explore the potential of a Gaussian-Mesh hybrid representation in the realm of 3D generation;
-
•
Extensive experimental results showcase the ability of our method to produce high-fidelity multi-view images and 3D assets. These outputs can be precisely controlled using an input condition image and text prompt.
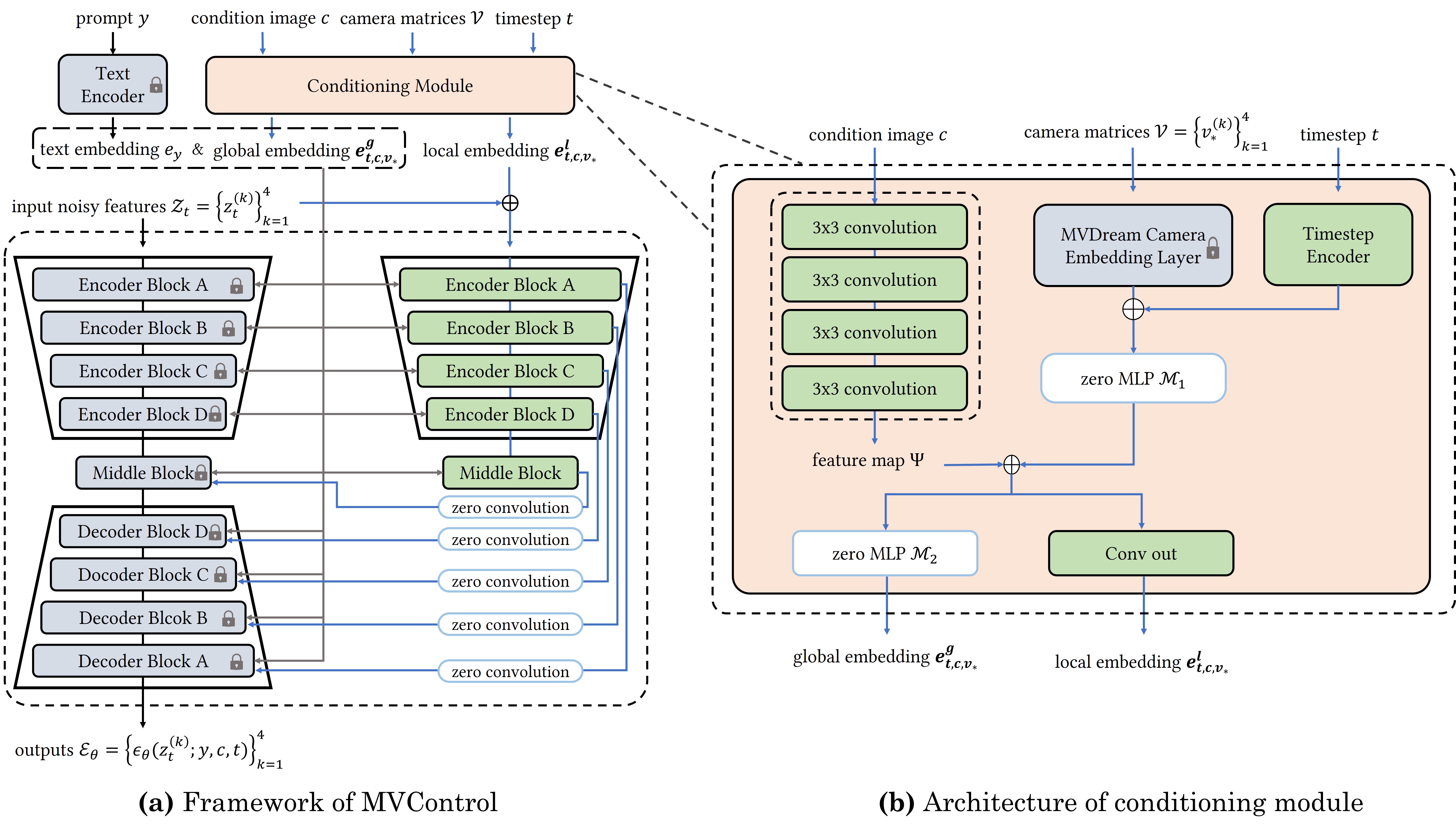
2 Related Work
Multi-view Diffusion Models. The success of text-to-image generation via large diffusion models inspires the development of multi-view image generation. Commonly adopted approach is to condition on a diffusion model by an additional input image and target pose [30, 33, 31]. Unlike those methods, Chan et al. recently proposed to learn 3D scene representation from a single or multiple input images and then exploit a diffusion model for target novel view image synthesis [10]. Instead of generating a single target view image, MVDiffusion [57] proposes to generate multi-view consistent images in one feed-forward pass. They build upon a pre-trained diffusion model to have better generalization capability. MVDream [49] introduces a method for generating consistent multi-view images from a text prompt. They achieve this by fine-tuning a pre-trained diffusion model using a 3D dataset. The trained model is then utilized as a 3D prior to optimize the 3D representation through Score Distillation Sampling. Similar work ImageDream[58] substitutes the text condition with an image. While prior works can generate impressive novel/multi-view consistent images, fine-grained control over the generated text-to-multi-view images is still difficult to achieve, as what ControlNet [68] has achieved for text-to-image generation. Therefore, we propose a multi-view ControlNet (i.e. MVControl) in this work to further advance diffusion-based multi-view image generation.
3D Generation Tasks. The exploration of generating 3D models can typically be categorized into two approaches. The first is SDS-based optimization method, initially proposed by DreamFusion[41], which aims to extract knowledge for 3D generation through the utilization of pre-trained large image models. SDS-based method benefits from not requiring expansive 3D datasets and has therefore been extensively explored in subsequent works[28, 11, 62, 51, 54, 69, 65, 42]. These works provide insights of developing more sophisticated score distillation loss functions [62, 42, 51], refining optimization strategies [69, 28, 11, 51, 54], and employing better 3D representations [11, 62, 51, 54, 65], thereby further enhancing the quality of the generation. Despite the success achieved by these methods in generating high-fidelity 3D assets, they usually require hours to complete the text-to-3D generation process. On the contrary, feed-forward 3D native methods can produce 3D assets within seconds after training on extensive 3D datasets[14]. Researchers have explored various 3D representations to achieve improved results, such as volumetric representation[6, 15, 63, 27], triangular mesh[53, 16, 13, 66], point cloud[2, 1], implicit neural representation[40, 35, 12, 47, 9, 61, 25, 19], as well as the recent 3D Gaussian[56]. While some methods efficiently generate 3D models that meet input conditions, 3D generative methods, unlike image generative modeling, struggle due to limited 3D training assets. This scarcity hinders their ability to produce high-fidelity and diverse 3D objects. Our method merges both approaches: generating a coarse 3D object with a feed-forward method conditioned on MVControl’s output, then refining it using SDS loss for the final representation.
Optimization-based Mesh Generation. The current single-stage mesh generation method, such as MeshDiffusion[32], struggles to produce high-quality mesh due to its highly complex structures. To achieve high grade mesh in both geometry and texture, researchers often turn to multi-stage optimization-based methods[28, 11, 51]. These methods commonly use non-mesh intermediate representations that are easy to process, before transforming them back into meshes with mesh reconstruction methods, which can consume a long optimization time. DreamGaussian[54] refer to a more efficient representation, 3D Gaussians, to effectively reduce the training time. However, extracting meshes from millions of unorganized tiny 3D Gaussians remains challenging. LGM[56] presents a new mesh extraction method for 3D Gaussians but still relies on implicit representation. In contrast, we adopt a fully explicit representation, a hybrid of mesh and 3D Gaussians as proposed by SuGaR[17]. This approach enables us to achieve high-quality mesh generation within reasonable optimization time.
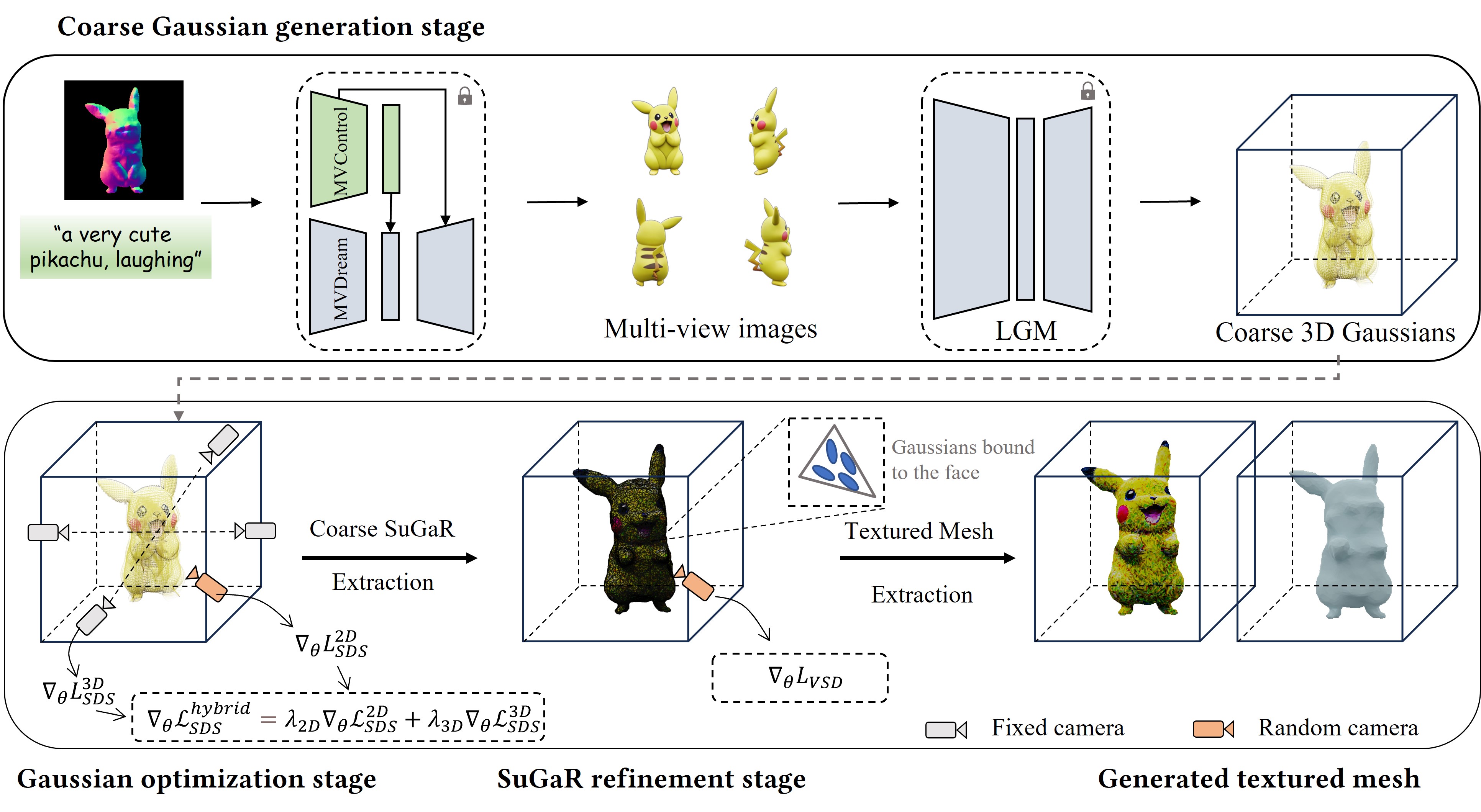
3 Method
We first review relevant methods, including the 2D ControlNet [68], score distillation sampling [41], Gaussian Splatting [24] and SuGaR [17] in Section 3.1. Then, we analyze the strategy of introducing additional spatial conditioning to MVDream by training a multi-view ControlNet in Section 3.2. Finally in Section 3.3, based on the trained multi-view ControlNet, we propose an efficient 3D generation pipeline, to realize the controllable text-to-3D generation via Gaussian-binded mesh and further textured mesh.
3.1 Preliminary
Score Distillation Sampling. Score distillation sampling (SDS) [41, 28] utilizes a pretrained text-to-image diffusion model as a prior to guide the generation of text-conditioned 3D assets. Specifically, given a pretrained diffusion model , SDS optimizes the parameters of a differentiable 3D representation (e.g., neural radiance field) using the gradient of the loss with respect to :
(1) |
where is an image rendered by under a camera pose , is a weighting function dependent on the timestep , and is the noisy image input to the diffusion model obtained by adding Gaussian noise to corresponding to the -th timestep. The primary insight is to enforce the rendered image of the learnable 3D representation to adhere to the distribution of the pretrained diffusion model. In practice, the values of the timestep and the Gaussian noise are randomly sampled at every optimization step.
Gaussian Splatting and SuGaR. Gaussian Splatting [24] represents the scene as a collection of 3D Gaussians, where each Gaussian is characterized by its center and covariance . The covariance is parameterized by a scaling factor and a rotation quaternion . Additionally, each Gaussian maintains opacity and color features for rendering via splatting. Typically, color features are represented using spherical harmonics to model view-dependent effects. During rendering, the 3D Gaussians are projected onto the 2D image plane as 2D Gaussians, and color values are computed through alpha composition of these 2D Gaussians in front-to-back depth order. While the vanilla Gaussian Splatting representation may not perform well in geometry modeling, SuGaR [17] introduces several regularization terms to enforce flatness and alignment of the 3D Gaussians with the object surface. This facilitates extraction of a mesh from the Gaussians through Poisson reconstruction [23]. Furthermore, SuGaR offers a hybrid representation by binding Gaussians to mesh faces, allowing joint optimization of texture and geometry through backpropagation.
3.2 Multi-view ControlNet
Inspired by ControlNet in controlled text-to-image generation and recently released text-to-multi-view image diffusion model (e.g. MVDream), we aim to design a multi-view version of ControlNet (i.e. MVControl) to achieve controlled text-to-multi-view generation. As shown in Fig. 2, we follow similar architecture style as ControlNet, i.e. a locked pre-trained MVDream and a trainable control network. The main insight is to preserve the learned prior knowledge of MVDream, while training the control network to learn the inductive bias with a small amount of data. The control network consists of a conditioning module and a copy of the encoder network of MVDream. Our main contribution lies at the conditioning module and we will detail it as follows.
The conditioning module (Fig. 2b) receives the condition image , four camera matrices and timestep as input, and outputs four local control embeddings and global control embeddings . The local embedding is then added with the input noisy latent features as the input to the control network, and the global embedding is injected to each layer of MVDream and MVControl to globally control generation.
The condition image (i.e. edge map, depth map etc.) is processed by four convolution layers to obtain a feature map . Instead of using the absolute camera pose matrices embedding of MVDream, we move the embedding into the conditioning module. To make the network better understand the spatial relationship among different views, the relative camera poses with respect to the condition image are used. The experimental results also validate the effectiveness of the design. The camera matrices embedding is combined with the timestep embedding, and is then mapped to have the same dimension as the feature map by a zero-initialized module . The sum of these two parts is projected to the local embedding through a convolution layer.
While MVDream is pretrained with absolute camera poses, the conditioning module exploits relative poses as input. We experimentally find that the network hardly converges due to the mismatch of both coordinate frames. We therefore exploit an additional network to learn the transformation and output a global embedding to replace the original camera matrix embedding of MVDream and add on timestep embeddings of both MVDream and MVControl part, so that semantical and view-dependent features are injected globally.
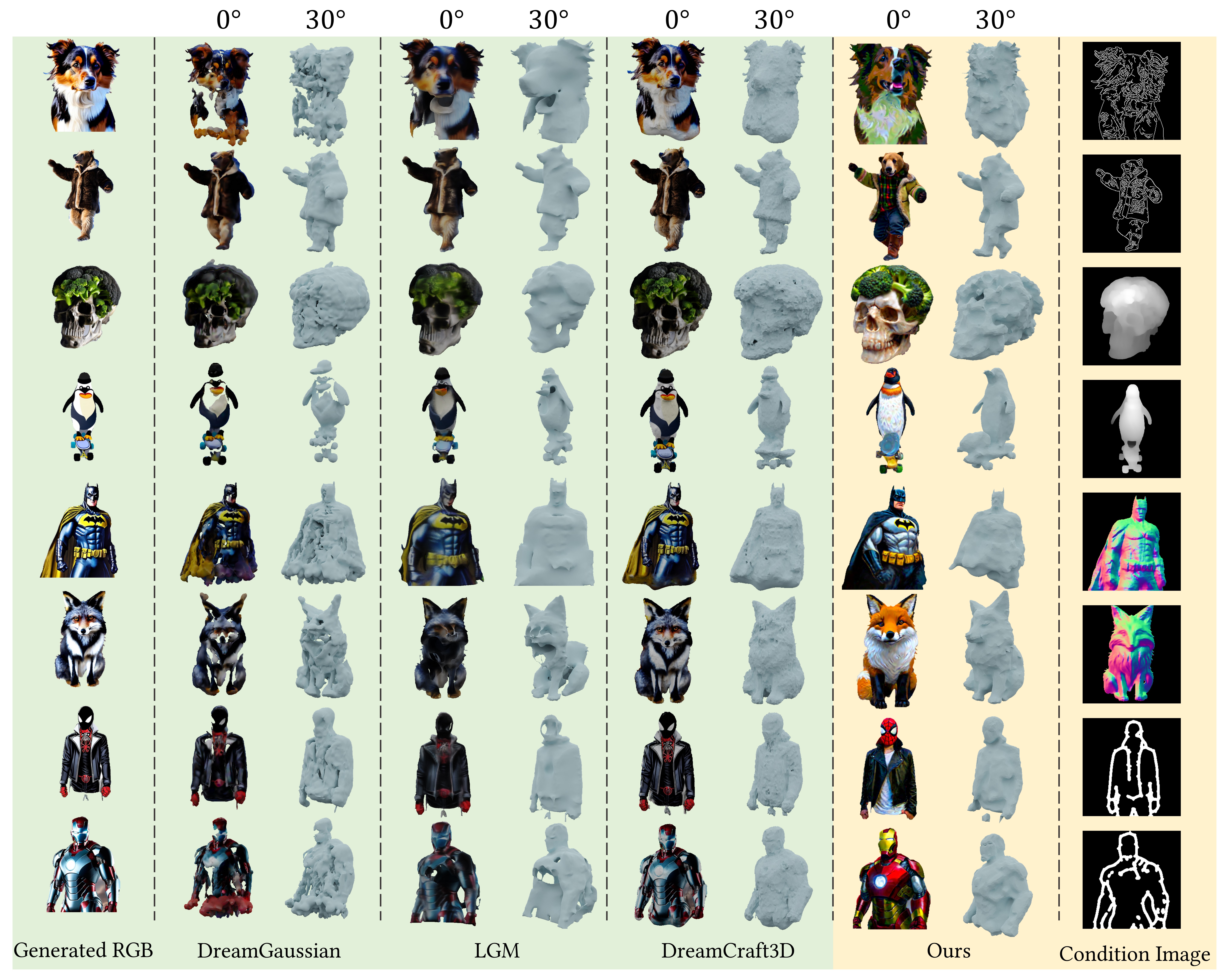
3.3 Controllable 3D Textured Mesh Generation
In this section, we introduce our highly efficient multi-stage textured mesh generation pipeline: Given a condition image and corresponding description prompt, we first generate a set of coarse 3D Gaussians using LGM [56] with four multi-view images generated by our trained MVControl. Subsequently, the coarse Gaussians undergo refinement utilizing a hybrid diffusion prior, supplemented with several regularization terms aimed at enhancing geometry and facilitating coarse SuGaR mesh extraction. Both the texture and geometry of the extracted coarse SuGaR mesh are refined using 2D diffusion guidance under high resolution, culminating in the attainment of a textured mesh. The overall pipeline is illustrated in Fig. 3.
Coarse Gaussians Initialization. Thanks to the remarkable performance of LGM [56], the images generated by our MVControl model can be directly inputted into LGM to produce a set of 3D Gaussians. However, owing to the low quality of the coarse Gaussians, transferring them directly to mesh, as done in the original paper, does not yield a satisfactory result. Instead, we further apply an optimization stage to refine the coarse Gaussians, with the starting point of optimization either initialized with all the coarse Gaussians’ features or solely their positions.
Gaussian-to-SuGaR Optimization. In this stage, we incorporate a hybrid diffusion guidance from a 2D diffusion model and our MVControl to enhance the optimization of coarse Gaussians . MVControl offers robust and consistent geometry guidance across four canonical views , while the 2D diffusion model contributes fine geometry and texture sculpting under other randomly sampled views . Here, we utilize the DeepFloyd-IF base model [3] due to its superior performance in refining coarse geometry. Given a text prompt and a condition image , the hybrid SDS gradient can be calculated as:
(2) | |||
where and are the strength of 2D and 3D prior respectively. To enhance the learning of geometry during the Gaussians optimization stage, we employ a Gaussian rasterization engine capable of rendering depth and alpha values [5]. Specifically, in addition to color images, depth and alpha of the scene are also rendered, and we estimate the surface normal by taking the derivative of . Consequently, the total variation (TV) regularization terms [45] on these components, denoted as and , are calculated and incorporated into the hybrid SDS loss. Furthermore, as the input conditions are invariably derived from existing images, a foreground mask is generated during the intermediate process. Therefore, we compute the mask loss to ensure the sparsity of the scene. Thus, the total loss for Gaussian optimization is expressed as:
(3) |
where are the weights of depth TV loss, normal TV loss and mask loss respectively. Following the approach in [11], we alternately utilize RGB images or normal maps as input to the diffusion models when calculating SDS gradients. After a certain number of optimization steps , we halt the split and pruning of Gaussians. Subsequently, we introduce SuGaR regularization terms [17] as new loss terms to to ensure that the Gaussians become flat and aligned with the object surface. This process continues for an additional steps, after which we prune all points whose opacity is below a threshold .
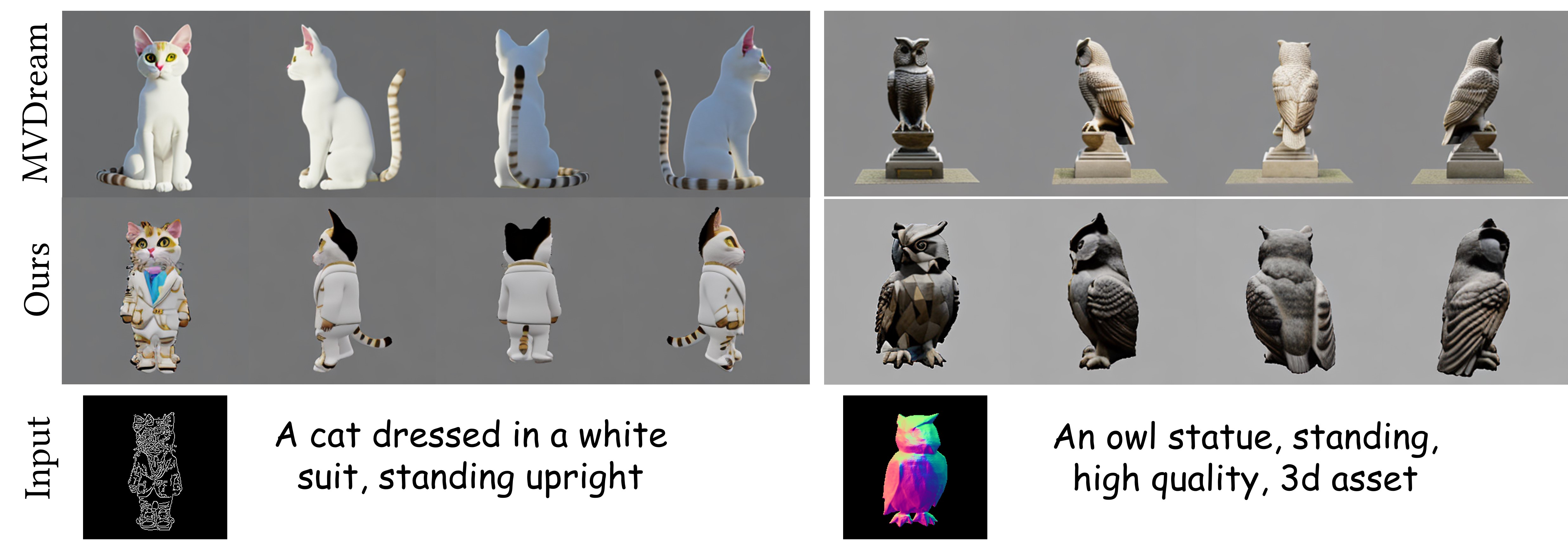
SuGaR Refinement. Following the official pipeline of [17], we transfer the optimized Gaussians to a coarse mesh. For each triangle face, a set of new flat Gaussians is bound. The color of these newly bound Gaussians is initialized with the colors of the triangle vertices. The positions of the Gaussians are initialized with predefined barycentric coordinates, and rotations are defined as 2D complex numbers to constrain the Gaussians within the corresponding triangles. Different from the original implementation, we initialize the learnable opacities of the Gaussians with a large number, specifically 0.9, to facilitate optimization at the outset. Given that the geometry of the coarse mesh is nearly fixed, we replace the hybrid diffusion guidance with solely 2D diffusion guidance computed using Stable Diffusion [44] to achieve higher optimization resolution. Additionally, we employ Variational Score Distillation (VSD) [62] due to its superior performance in texture optimization. Similarly, we render the depth and alpha through the bound Gaussians. However, in contrast, we can directly render the normal map using mesh face normals. With these conditions, we calculate the TV losses, and , and the mask loss similarly to the previous section. The overall loss for SuGaR refinement is computed as:
(4) |
where represent the weights of the different loss terms, respectively.
4 Experiments
4.1 Qualitative Comparisons
Multi-view Image Generation. To assess the controlling capacity of our MVControl, we conduct experiments on MVDream both with and without MVControl attached as shown in Fig. 5. In the first case, MVDream fails to generate the correct contents according to the given prompt, producing a squatting cat without clothes, which contradicts the prompt. In contrast, it successfully generates the correct contents with the assistance of MVControl. The second case also demonstrates that our MVControl effectively controls the generation of MVDream, resulting in highly view-consistent multi-view images.
3D Gaussian-based Mesh Generation. Given that our 3D generation pipeline aims to produce textured mesh from 3D Gaussians, we compare our method with recent Gaussian-based mesh generation approaches, DreamGaussian [54] and LGM [56], both of which can be conditioned on RGB images. Moreover, we also take the state-of-the-art image-to-3D generation method, DreamCraft3D [51] into comparison. For fair comparison, we generate 2D RGB images without cherry picking using the pre-trained 2D ControlNet as the input for the compared methods. As illustrated in Fig. 4, DreamGaussian struggles to generate the geometry for most of the examples, resulting in many broken and hollow areas in the generated meshes. LGM performs better than DreamGaussian, however, its extracted meshes lack details and still contain broken areas in some cases. Although DreamCraft3D can produce unbroken shapes, it still suffer from unsmoothed surface in the meshes. In contrast, our method produces fine-grain meshes with more delicate textures, even without an RGB condition. Due to space limitations, the textual prompts are not provided in Fig. 4, and we will include them in our appendix.
Optimiztion Stage | CLIP-T | CLIP-I | |
---|---|---|---|
DreamGaussian | GSMesh | 0.200 | 0.847 |
LGM | GSMesh | 0.228 | 0.872 |
DreamCraft3D | NeRFNeuSDMTet | 0.275 | 0.884 |
MVControl(Ours) | GSSuGaR | 0.279 | 0.909 |
4.2 Quantitative Comparisons
In this section, we adopt CLIP-score [38] to evaluate the compared methods and our method. We calculate both image-text and image-image similarities. For each object, we uniformly render 36 surrounding views. The image-text similarity, denoted as CLIP-T, is computed by averaging the similarities between each view and the given prompt.Similarly, the image-image similarity, referred to as CLIP-I, is the mean similarity between each view and the reference view. The results, calculated for a set of 60 objects, are reported in Table 1. When employing our method, the condition type used for each object is randomly sampled from edge, depth, normal, and scribble map. Additionally, the RGB images for DreamGaussian and LGM are generated using 2D ControlNet with the same condition image and prompt. Our method achieves the best performance in terms of both the CLIP-T and CLIP-I score.
4.3 Ablation Study
CLIP-T | CLIP-I | |
---|---|---|
Stage 2 w/o | 0.245 | 0.866 |
Stage 2 w/o normal losses | 0.263 | 0.876 |
Full stage 2 | 0.267 | 0.882 |
Stage 1 only | 0.230 | 0.859 |
Full method | 0.279 | 0.909 |
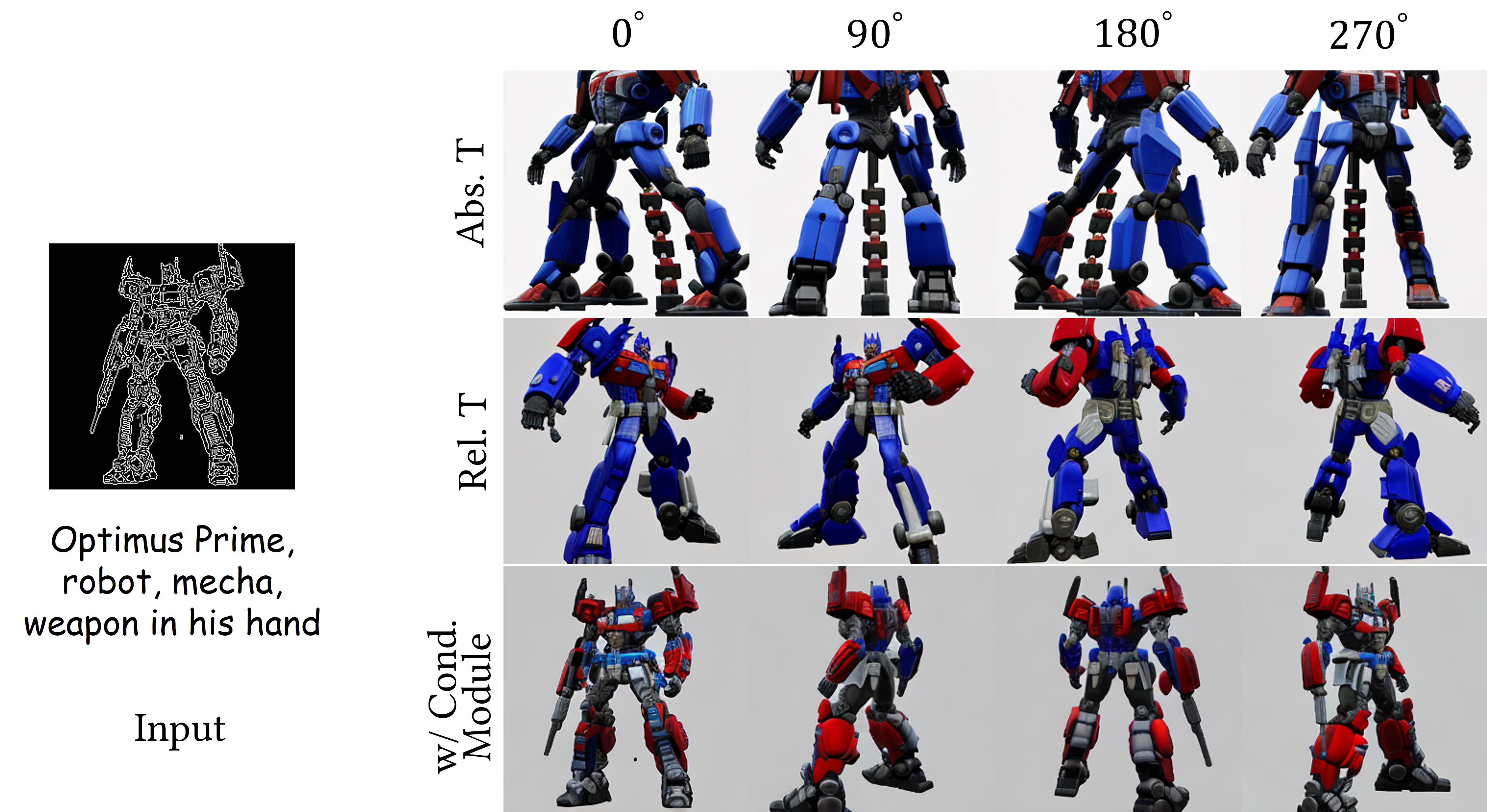
Conditioning Module of MVControl. We evaluate the training of our model under three different settings to introduce camera condition: 1) we utilize the absolute (world) camera system (i.e., Abs. T) as MVDream [49] does, without employing our designed conditioning module (retaining the same setup as 2D ControlNet); 2) we adopt the relative camera system without employing the conditioning module; 3) we employ the complete conditioning module. The experimental results, depicted in Fig. 6, demonstrate that only the complete conditioning module can accurately generate view-consistent multi-view images that adhere to the descriptions provided by the condition image.
Hybrid Diffusion Guidance. We conduct ablation study on hybrid diffusion guidance utilized in the Gaussian optimization stage. As illustrated in Fig. 7 (top right), when excluding provided by our MVControl, the generated 3D Gaussians lack texture details described in the given condition edge map. For instance, the face of the rabbit appears significantly blurrier without . The quantitative evaluation is provided in Table 2 (line 1 and 3).
Losses on Rendered Normal Maps. The normal-related losses in our method are alternately calculated using SDS loss with the normal map as input in stage 2, and the normal TV regularization term. We conduct experiments by dropping all of them in stage 2, and the results are illustrated in Fig. 7 (bottom left). Compared to our full method, the surface normal of 3D Gaussians deteriorates without the normal-related losses. The corresponding quantitative results are provided in Table 2 (line 2 and 3).
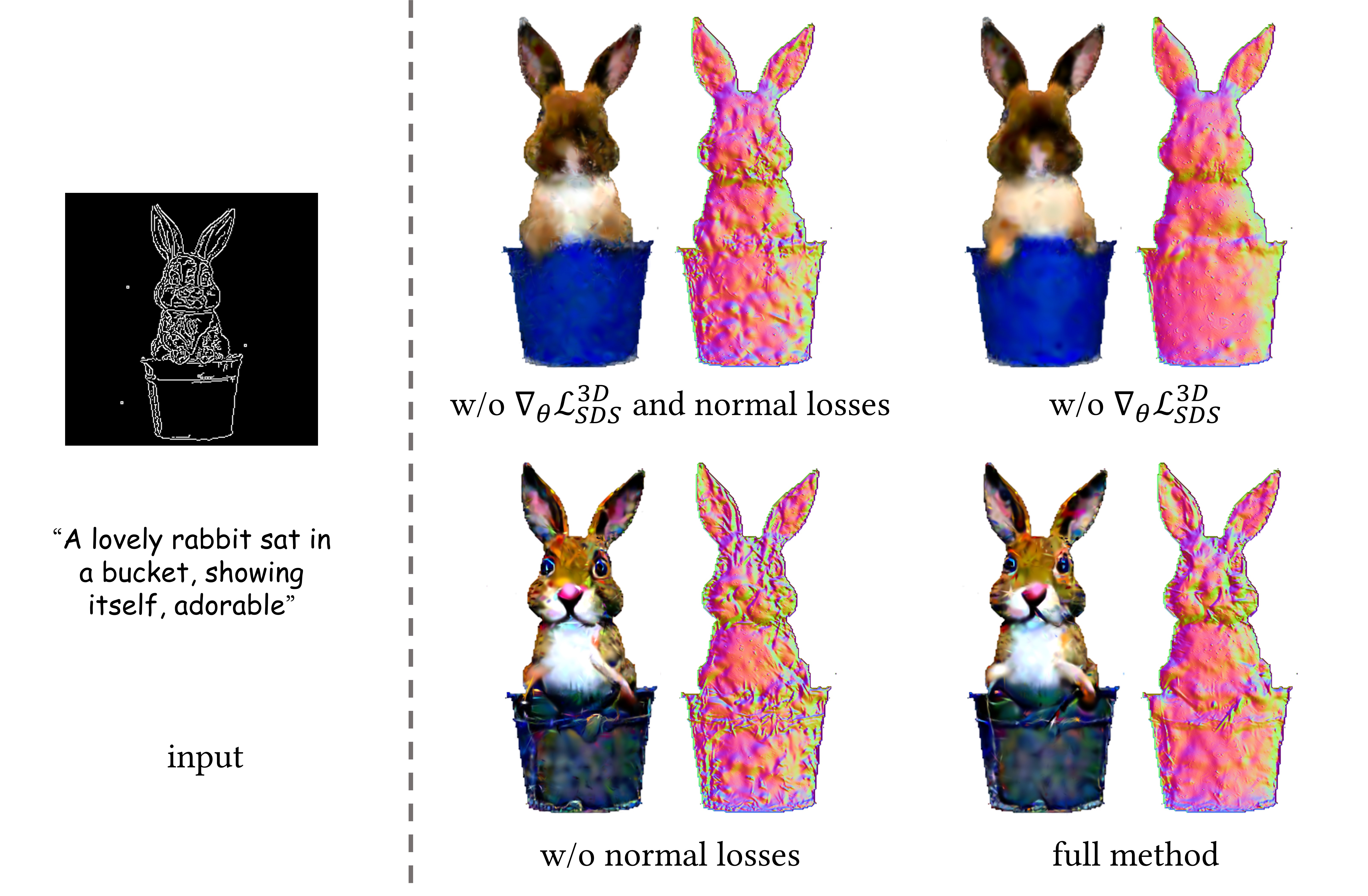
Multi-stage Optimization. We also assess the impact of different optimization stages, as shown in Fig. 8. Initially, in stage 1, the coarse Gaussians exhibit poor geometry consistency. However, after the Gaussian optimization stage, they become view-consistent, albeit with blurry texture. Finally, in the SuGaR refinement stage, the texture of the 3D model becomes fine-grained and of high quality. We have also provided the quantitative evaluation on different optimization stages in Table 2 (the lower 3 lines).
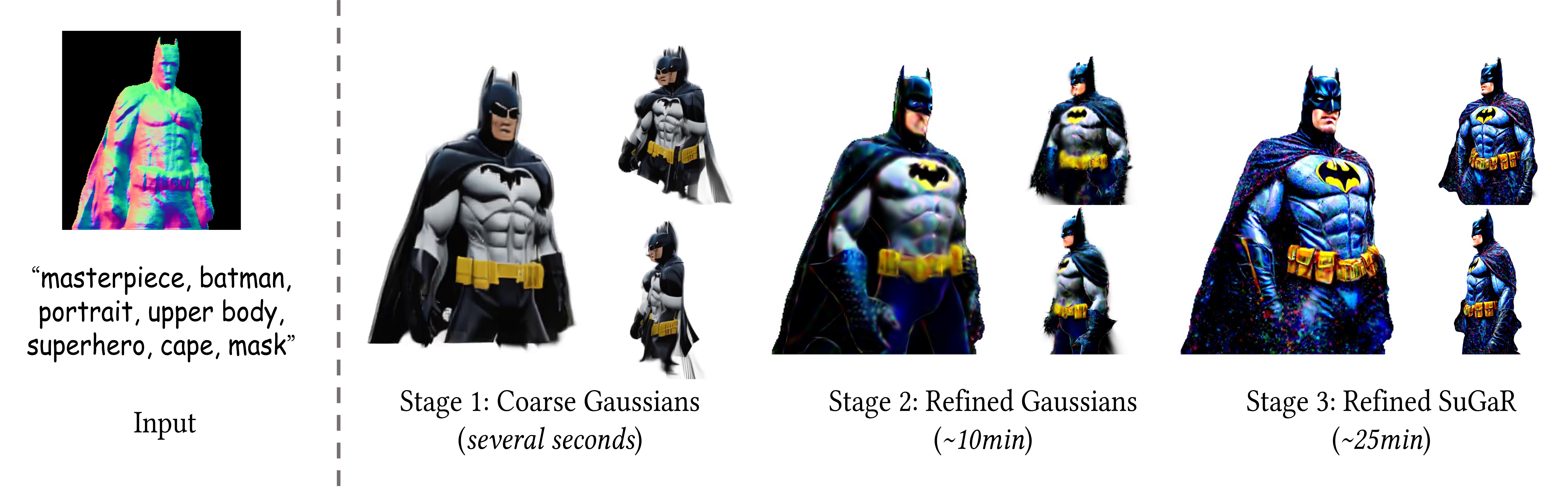
5 Conclusion
In this work, we delve into the important yet under-explored field of controllable 3D generation. We present a novel network architecture, MVControl, for controllable text-to-multiview image generation. Our approach features a trainable control network that interacts with the base image diffusion model to enable controllable multi-view image generation. Once trained, our network offers 3D diffusion guidance for controllable text-to-3D generation using a hybrid SDS gradient alongside another 2D diffusion model. We propose an efficient multi-stage 3D generation pipeline using both feed-forward and optimization-based methods. Our pioneering use of SuGaR—an explicit representation blending mesh and 3D Gaussians—outperforms previous Gaussian-based mesh generation approaches. Experimental results demonstrate our method’s ability to produce controllable, high-fidelity text-to-multiview images and text-to-3D assets. Furthermore, tests across various conditions show our method’s generalization capabilities. We believe our network has broader applications in 3D vision and graphics beyond controllable 3D generation via SDS optimization.
Acknowledgement
This work was supported in part by NSFC under Grant 62202389, in part by a grant from the Westlake University-Muyuan Joint Research Institute, and in part by the Westlake Education Foundation.
References
- Achlioptas et al. [2018] Panos Achlioptas, Olga Diamanti, Ioannis Mitliagkas, and Leonidas Guibas. Learning representations and generative models for 3d point clouds. In International conference on machine learning, pages 40–49. PMLR, 2018.
- Albert Pumarola and Ferrari [2020] Francesc Moreno-Noguer Albert Pumarola, Stefan Popov and Vittorio Ferrari. C-Flow: Conditional generative flow models for images and 3D point clouds. In CVPR, 2020.
- Alex et al. [2023] Shonenkov Alex, Konstantinov Misha, Bakshandaeva Daria, Schuhmann Christoph, Ivanova Ksenia, and Klokova Nadiia. Deepfloyd if: A modular cascaded diffusion model. https://github.com/deep-floyd/IF/tree/develop, 2023.
- Armandpour et al. [2023] Mohammadreza Armandpour, Huangjie Zheng, Ali Sadeghian, Amir Sadeghian, and Mingyuan Zhou. Re-imagine the negative prompt algorithm: Transform 2d diffusion into 3d, alleviate janus problem and beyond. arXiv preprint arXiv:2304.04968, 2023.
- ashawkey [2023] ashawkey. Differential gaussian rasterization. https://github.com/ashawkey/diff-gaussian-rasterization, 2023.
- Brock et al. [2016] Andrew Brock, Theodore Lim, James M Ritchie, and Nick Weston. Generative and discriminative voxel modeling with convolutional neural networks. arXiv preprint arXiv:1608.04236, 2016.
- Canny [1986] John Canny. A computational approach to edge detection. IEEE Transactions on pattern analysis and machine intelligence, (6):679–698, 1986.
- Cao et al. [2023] Ziang Cao, Fangzhou Hong, Tong Wu, Liang Pan, and Ziwei Liu. Large-vocabulary 3d diffusion model with transformer. arXiv preprint arXiv:2309.07920, 2023.
- Chan et al. [2022] Eric R. Chan, Connor Z. Lin, Matthew A. Chan, Koki Nagano, Boxiao Pan, Shalini De Mello, Orazio Gallo, Leonidas Guibas, Jonathan Tremblay, Sameh Khamis, Tero Karras, and Gordon Wetzstein. Efficient Geometry Aware 3D Generative Adversarial Networks. In CVPR, 2022.
- Chan et al. [2023] Eric R. Chan, Koki Nagano, Matthew A. Chan, Alexander W. Bergman, Jeong Joon Park, Axel Levy, Miika Aittala, Shalini De Mello, Tero Karras, and Gordon Wetzstein. Generative novel view synthesis with 3D aware diffusion models. In ICCV, 2023.
- Chen et al. [2023] Rui Chen, Yongwei Chen, Ningxin Jiao, and Kui Jia. Fantasia3d: Disentangling geometry and appearance for high-quality text-to-3d content creation. arXiv preprint arXiv:2303.13873, 2023.
- Chen and Zhang [2019] Zhiqin Chen and Hao Zhang. Learning implicit fields for generative shape modeling. In CVPR, 2019.
- Dario Pavllo and Lucchi [2021] Thomas Hofmann Dario Pavllo, Jonas Kohler and Aurelien Lucchi. Learning generative models of textured 3D meshes from real-world images. In ICCV, 2021.
- Deitke et al. [2023] Matt Deitke, Dustin Schwenk, Jordi Salvador, Luca Weihs, Oscar Michel, Eli VanderBilt, Ludwig Schmidt, Kiana Ehsani, Aniruddha Kembhavi, and Ali Farhadi. Objaverse: A universe of annotated 3d objects. In Proceedings of the IEEE/CVF Conference on Computer Vision and Pattern Recognition, pages 13142–13153, 2023.
- Gadelha et al. [2017] Matheus Gadelha, Subhransu Maji, and Rui Wang. 3d shape induction from 2d views of multiple objects. In 2017 International Conference on 3D Vision (3DV), pages 402–411. IEEE, 2017.
- Gao et al. [2019] Lin Gao, Jie Yang, Tong Wu, Yujie Yuan, Hongbo Fu, Yukun Lai, and Hao Zhang. SDM-Net: Deep generative network for structured deformable mesh. In ACM TOG, 2019.
- Guédon and Lepetit [2023] Antoine Guédon and Vincent Lepetit. Sugar: Surface-aligned gaussian splatting for efficient 3d mesh reconstruction and high-quality mesh rendering. arXiv preprint arXiv:2311.12775, 2023.
- Guo et al. [2023] Yuan-Chen Guo, Ying-Tian Liu, Ruizhi Shao, Christian Laforte, Vikram Voleti, Guan Luo, Chia-Hao Chen, Zi-Xin Zou, Chen Wang, Yan-Pei Cao, and Song-Hai Zhang. threestudio: A unified framework for 3d content generation. https://github.com/threestudio-project/threestudio, 2023.
- Hong et al. [2023] Yicong Hong, Kai Zhang, Jiuxiang Gu, Sai Bi, Yang Zhou, Difan Liu, Feng Liu, Kalyan Sunkavalli, Trung Bui, and Hao Tan. Lrm: Large reconstruction model for single image to 3d. arXiv preprint arXiv:2311.04400, 2023.
- Huang et al. [2023] Yukun Huang, Jianan Wang, Yukai Shi, Xianbiao Qi, Zheng-Jun Zha, and Lei Zhang. Dreamtime: An improved optimization strategy for text-to-3d content creation. arXiv preprint arXiv:2306.12422, 2023.
- Jun and Nichol [2023] Heewoo Jun and Alex Nichol. Shap-e: Generating conditional 3d implicit functions. arXiv preprint arXiv:2305.02463, 2023.
- Katzir et al. [2023] Oren Katzir, Or Patashnik, Daniel Cohen-Or, and Dani Lischinski. Noise-free score distillation. arXiv preprint arXiv:2310.17590, 2023.
- Kazhdan et al. [2006] Michael Kazhdan, Matthew Bolitho, and Hugues Hoppe. Poisson surface reconstruction. In Proceedings of the fourth Eurographics symposium on Geometry processing, page 0, 2006.
- Kerbl et al. [2023] Bernhard Kerbl, Georgios Kopanas, Thomas Leimkühler, and George Drettakis. 3d gaussian splatting for real-time radiance field rendering. ACM Transactions on Graphics (ToG), 42(4):1–14, 2023.
- Li et al. [2023a] Jiahao Li, Hao Tan, Kai Zhang, Zexiang Xu, Fujun Luan, Yinghao Xu, Yicong Hong, Kalyan Sunkavalli, Greg Shakhnarovich, and Sai Bi. Instant3d: Fast text-to-3d with sparse-view generation and large reconstruction model. arXiv preprint arXiv:2311.06214, 2023a.
- Li et al. [2023b] Weiyu Li, Rui Chen, Xuelin Chen, and Ping Tan. Sweetdreamer: Aligning geometric priors in 2d diffusion for consistent text-to-3d. arXiv preprint arXiv:2310.02596, 2023b.
- Li et al. [2019] Xiao Li, Yue Dong, Pieter Peers, and Xin Tong. Synthesizing 3d shapes from silhouette image collections using multi-projection generative adversarial networks. In Proceedings of the IEEE/CVF Conference on Computer Vision and Pattern Recognition, pages 5535–5544, 2019.
- Lin et al. [2023a] Chen-Hsuan Lin, Jun Gao, Luming Tang, Towaki Takikawa, Xiaohui Zeng, Xun Huang, Karsten Kreis, Sanja Fidler, Ming-Yu Liu, and Tsung-Yi Lin. Magic3d: High-resolution text-to-3d content creation. In Proceedings of the IEEE/CVF Conference on Computer Vision and Pattern Recognition, pages 300–309, 2023a.
- Lin et al. [2023b] Shanchuan Lin, Bingchen Liu, Jiashi Li, and Xiao Yang. Common diffusion noise schedules and sample steps are flawed. arXiv preprint arXiv:2305.08891, 2023b.
- Liu et al. [2023a] Ruoshi Liu, Rundi Wu, Basile Van Hoorick, Pavel Tokmakov, Sergey Zakharov, and Carl Vondrick. Zero-1-to-3: Zero-shot one image to 3d object. In Proceedings of the IEEE/CVF International Conference on Computer Vision, pages 9298–9309, 2023a.
- Liu et al. [2023b] Yuan Liu, Cheng Lin, Zijiao Zeng, Xiaoxiao Long, Lingjie Liu, Taku Komura, and Wenping Wang. Syncdreamer: Generating multiview-consistent images from a single-view image. arXiv preprint arXiv:2309.03453, 2023b.
- Liu et al. [2023c] Zhen Liu, Yao Feng, Michael J Black, Derek Nowrouzezahrai, Liam Paull, and Weiyang Liu. Meshdiffusion: Score-based generative 3d mesh modeling. arXiv preprint arXiv:2303.08133, 2023c.
- Long et al. [2023] Xiaoxiao Long, Yuan-Chen Guo, Cheng Lin, Yuan Liu, Zhiyang Dou, Lingjie Liu, Yuexin Ma, Song-Hai Zhang, Marc Habermann, Christian Theobalt, et al. Wonder3d: Single image to 3d using cross-domain diffusion. arXiv preprint arXiv:2310.15008, 2023.
- Luo et al. [2023] Tiange Luo, Chris Rockwell, Honglak Lee, and Justin Johnson. Scalable 3d captioning with pretrained models. arXiv preprint arXiv:2306.07279, 2023.
- Mescheder et al. [2019] Lars Mescheder, Michael Oechsle, Michael Niemeyer, Sebastuan Nowozin, and Andreas Geiger. Occupancy Networks: Learning 3D reconstruction in function space. In CVPR, 2019.
- Metzer et al. [2023] Gal Metzer, Elad Richardson, Or Patashnik, Raja Giryes, and Daniel Cohen-Or. Latent-nerf for shape-guided generation of 3d shapes and textures. In Proceedings of the IEEE/CVF Conference on Computer Vision and Pattern Recognition, pages 12663–12673, 2023.
- Mildenhall et al. [2021] Ben Mildenhall, Pratul P Srinivasan, Matthew Tancik, Jonathan T Barron, Ravi Ramamoorthi, and Ren Ng. Nerf: Representing scenes as neural radiance fields for view synthesis. Communications of the ACM, 65(1):99–106, 2021.
- Mohammad Khalid et al. [2022] Nasir Mohammad Khalid, Tianhao Xie, Eugene Belilovsky, and Tiberiu Popa. Clip-mesh: Generating textured meshes from text using pretrained image-text models. pages 1–8, 2022.
- Nichol et al. [2022] Alex Nichol, Heewoo Jun, Prafulla Dhariwal, Pamela Mishkin, and Mark Chen. Point-e: A system for generating 3d point clouds from complex prompts. arXiv preprint arXiv:2212.08751, 2022.
- Park et al. [2019] Jeong Joon Park, Peter Florence, Julian Straub, Richard Newcombe, and Steven Lovegrove. DeepSDF: Learning continuous signed distance functions for shape representation. In CVPR, 2019.
- Poole et al. [2023] Ben Poole, Ajay Jain, Jonathan T Barron, and Ben Mildenhall. Dreamfusion: Text-to-3d using 2d diffusion. In ICLR, 2023.
- Qian et al. [2023] Guocheng Qian, Jinjie Mai, Abdullah Hamdi, Jian Ren, Aliaksandr Siarohin, Bing Li, Hsin-Ying Lee, Ivan Skorokhodov, Peter Wonka, Sergey Tulyakov, et al. Magic123: One image to high-quality 3d object generation using both 2d and 3d diffusion priors. arXiv preprint arXiv:2306.17843, 2023.
- Ranftl et al. [2021] René Ranftl, Alexey Bochkovskiy, and Vladlen Koltun. Vision transformers for dense prediction. In Proceedings of the IEEE/CVF international conference on computer vision, pages 12179–12188, 2021.
- Rombach et al. [2022] Robin Rombach, Andreas Blattmann, Dominik Lorenz, Patrick Esser, and Björn Ommer. High-resolution image synthesis with latent diffusion models. In Proceedings of the IEEE/CVF conference on computer vision and pattern recognition, pages 10684–10695, 2022.
- Rudin and Osher [1994] Leonid I Rudin and Stanley Osher. Total variation based image restoration with free local constraints. In Proceedings of 1st international conference on image processing, pages 31–35. IEEE, 1994.
- Schuhmann et al. [2022] Christoph Schuhmann, Romain Beaumont, Richard Vencu, Cade Gordon, Ross Wightman, Mehdi Cherti, Theo Coombes, Aarush Katta, Clayton Mullis, Mitchell Wortsman, et al. Laion-5b: An open large-scale dataset for training next generation image-text models. Advances in Neural Information Processing Systems, 35:25278–25294, 2022.
- Schwarz et al. [2022] Katja Schwarz, Axel Sauer, Michael Niemeyer, Yiyi Liao, , and Andreas Geiger. VoxGRAF: Fast 3D-aware image synthesis with sparse voxel grids. 2022.
- Shen et al. [2021] Tianchang Shen, Jun Gao, Kangxue Yin, Ming-Yu Liu, and Sanja Fidler. Deep marching tetrahedra: a hybrid representation for high-resolution 3d shape synthesis. Advances in Neural Information Processing Systems, 34:6087–6101, 2021.
- Shi et al. [2023] Yichun Shi, Peng Wang, Jianglong Ye, Mai Long, Kejie Li, and Xiao Yang. Mvdream: Multi-view diffusion for 3d generation. arXiv preprint arXiv:2308.16512, 2023.
- Song et al. [2020] Jiaming Song, Chenlin Meng, and Stefano Ermon. Denoising diffusion implicit models. arXiv preprint arXiv:2010.02502, 2020.
- Sun et al. [2023a] Jingxiang Sun, Bo Zhang, Ruizhi Shao, Lizhen Wang, Wen Liu, Zhenda Xie, and Yebin Liu. Dreamcraft3d: Hierarchical 3d generation with bootstrapped diffusion prior. arXiv preprint arXiv:2310.16818, 2023a.
- Sun et al. [2023b] Qinghong Sun, Yangguang Li, ZeXiang Liu, Xiaoshui Huang, Fenggang Liu, Xihui Liu, Wanli Ouyang, and Jing Shao. Unig3d: A unified 3d object generation dataset. arXiv preprint arXiv:2306.10730, 2023b.
- Tan et al. [2018] Qingyang Tan, Lin Gao, Yukun Lai, and Shihong Xia. Variational autoencoders for deforming 3D mesh models. In CVPR, 2018.
- Tang et al. [2023a] Jiaxiang Tang, Jiawei Ren, Hang Zhou, Ziwei Liu, and Gang Zeng. Dreamgaussian: Generative gaussian splatting for efficient 3d content creation. arXiv preprint arXiv:2309.16653, 2023a.
- Tang et al. [2023b] Junshu Tang, Tengfei Wang, Bo Zhang, Ting Zhang, Ran Yi, Lizhuang Ma, and Dong Chen. Make-it-3d: High-fidelity 3d creation from a single image with diffusion prior. arXiv preprint arXiv:2303.14184, 2023b.
- Tang et al. [2024] Jiaxiang Tang, Zhaoxi Chen, Xiaokang Chen, Tengfei Wang, Gang Zeng, and Ziwei Liu. Lgm: Large multi-view gaussian model for high-resolution 3d content creation. arXiv preprint arXiv:2402.05054, 2024.
- Tang et al. [2023c] Shitao Tang, Fuyang Zhang, Jiacheng Chen, Peng Wang, and Yasutaka Furukawa. Mvdiffusion: Enabling holistic multi-view image generation with correspondence-aware diffusion, 2023c.
- Wang and Shi [2023] Peng Wang and Yichun Shi. Imagedream: Image-prompt multi-view diffusion for 3d generation. arXiv preprint arXiv:2312.02201, 2023.
- Wang et al. [2021] Peng Wang, Lingjie Liu, Yuan Liu, Christian Theobalt, Taku Komura, and Wenping Wang. Neus: Learning neural implicit surfaces by volume rendering for multi-view reconstruction. arXiv preprint arXiv:2106.10689, 2021.
- Wang et al. [2023a] Peng Wang, Hao Tan, Sai Bi, Yinghao Xu, Fujun Luan, Kalyan Sunkavalli, Wenping Wang, Zexiang Xu, and Kai Zhang. Pf-lrm: Pose-free large reconstruction model for joint pose and shape prediction. arXiv preprint arXiv:2311.12024, 2023a.
- Wang et al. [2023b] Tengfei Wang, Bo Zhang, Ting Zhang, Shuyang Gu, Jianmin Bao, Tadas Baltrusaitis, Jingjing Shen, Dong Chen, Fang Wen, Qifeng Chen, and Baining Guo. Rodin: A generative model for sculpting 3D digital Avatars using diffusion. In CVPR, 2023b.
- Wang et al. [2023c] Zhengyi Wang, Cheng Lu, Yikai Wang, Fan Bao, Chongxuan Li, Hang Su, and Jun Zhu. Prolificdreamer: High-fidelity and diverse text-to-3d generation with variational score distillation. arXiv preprint arXiv:2305.16213, 2023c.
- Wu et al. [2016] Jiajun Wu, Chengkai Zhang, Tianfan Xue, Bill Freeman, and Josh Tenenbaum. Learning a probabilistic latent space of object shapes via 3d generative-adversarial modeling. Advances in neural information processing systems, 29, 2016.
- Xie and Tu [2015] Saining Xie and Zhuowen Tu. Holistically-nested edge detection. In Proceedings of the IEEE international conference on computer vision, pages 1395–1403, 2015.
- Yi et al. [2023] Taoran Yi, Jiemin Fang, Guanjun Wu, Lingxi Xie, Xiaopeng Zhang, Wenyu Liu, Qi Tian, and Xinggang Wang. Gaussiandreamer: Fast generation from text to 3d gaussian splatting with point cloud priors. arXiv preprint arXiv:2310.08529, 2023.
- Youwang et al. [2022] Kim Youwang, Kim Ji-Yeon, and Tae-Hyun Oh. CLIP-Actor: text driven recommendation and stylization for animating human meshes. In ECCV, 2022.
- Yu et al. [2023] Xin Yu, Yuan-Chen Guo, Yangguang Li, Ding Liang, Song-Hai Zhang, and Xiaojuan Qi. Text-to-3d with classifier score distillation. arXiv preprint arXiv:2310.19415, 2023.
- Zhang et al. [2023] Lvmin Zhang, Anyi Rao, and Maneesh Agrawala. Adding conditional control to text-to-image diffusion models. In Proceedings of the IEEE/CVF International Conference on Computer Vision, pages 3836–3847, 2023.
- Zhu et al. [2023] Junzhe Zhu, Peiye Zhuang, and Sanmi Koyejo. Hifa: High-fidelity text-to-3d generation with advanced diffusion guidance. In The Twelfth International Conference on Learning Representations, 2023.
Supplementary Material
Appendix A Introduction
In this supplementary material, we offer additional details regarding our experimental setup and implementation. Subsequently, we present more qualitative results showcasing the performance and diversity of our method with various types of condition images as input.
Appendix B Implementation Detail
B.1 Training Data
Multi-view Images Dataset. We employ the multi-view renderings from the publicly available large 3D dataset, Objaverse [14], to train our MVControl. Initially, we preprocess the dataset by removing all samples with a CLIP-score lower than 22, based on the labeling criteria from [52]. This filtering results in approximately 400k remaining samples. For each retained sample, we first normalize its scene bounding box to a unit cube centered at the world origin. Subsequently, we sample a random camera setting by uniformly selecting the camera distance between 1.4 and 1.6, the angle of Field-of-View (FoV) between 40 and 60 degrees, the degree of elevation between 0 and 30 degrees, and the starting azimuth angle between 0 and 360 degrees. Under the random camera setting, multi-view images are rendered at a resolution of 256256 under 4 canonical views at the same elevation starting from the sampled azimuth. We repeat this procedure three times for each object. During training, one of these views is chosen as the reference view corresponding to the condition image. Instead of utilizing the names and tags of the 3D assets, we employ the captions from [34] as text descriptions for our retained objects.
Canny Edges. We apply the Canny edge detector [7] with random thresholds to all rendered images to obtain the Canny edge conditions. The lower threshold is randomly selected from the range [50, 125], while the upper threshold is chosen from the range [175, 250].
Depth Maps. We use the pre-trained depth estimator, Midas [43], to estimate the depth maps of rendered images.
Normal Maps. We compute normal map estimations of all rendered images by computing normal-from-distance on the depth values predicted by Midas.
B.2 Training Details of MVControl
While our base model, MVDream [49], is fine-tuned from Stable Diffusion v2.1 [44], we train our multi-view ControlNet models from publicly available 2D ControlNet checkpoints††https://huggingface.co/thibaud adapted to Stable Diffusion v2.1 for consistency. The models are trained on an 8A100 node, where we have 160 (404) images on each GPU. With a gradient accumulation of 2 steps, we achieve a total batch size of 2560 images. The model undergoes 50000 steps of training under a constant learning rate of with 1000 steps of warm-up. Similar to the approach in [68], we randomly drop the text prompt as empty with a 50% chance during training to facilitate classifier-free learning and enhance the model’s understanding of input condition images. Moreover, we also employ 2D-3D joint training following [49]. Specifically, we randomly sample images from the AES v2 subset of LAION [46] with a 30% probability during training to ensure the network retains its learned 2D image priors.
B.3 Implementation Details of 3D Generation
Multi-view Image Generation . In our coarse Gaussian generation stage, the multi-view images are generated with our MVControl attached to MVDream using a 30-step DDIM sampler [50] with a guidance scale of 9 and a negative prompt ”ugly, blurry, pixelated obscure, unnatural colors, poor lighting, dull, unclear, cropped, lowres, low quality, artifacts, duplicate”.
Gaussian Optimization Stage . This stage comprises a total of 3000 steps. During the initial 1500 steps, we perform simple 3D Gaussian optimization with split and prune every 300 steps. After step 1500, we cease densification and prune, and instead introduce SuGaR [17] regularization terms to refine the scene. In the end of the stage, we prune all Gaussians with opacity below . The 3D SDS () is computed with a guidance scale of 50 using the CFG rescale trick [29], and is computed with a guidance scale of 20. We use and for 2D and 3D diffusion guidance, with resolutions of 512512 and 256256 respectively.
SuGaR Refinement Stage . This stage has totally 5000 steps of optimiztion. The in the SuGaR refinement stage is computed with a guidance scale of 7.5. The training is under resolution 512512 for rendering.
All score distillation terms also incorporate the aforementioned negative prompt. Our implementation is based on the threestudio project [18]. All testing images for condition image extraction are downloaded from civitai.com.
Appendix C Additional Qualitative Results
C.1 Diversity of MVControl
Similar to 2D ControlNet [68], our MVControl can generate diverse multi-view images with the same condition image and prompt. Please refer to our project page for some of the results.
C.2 Textured Meshes
We also provide additional generated textured mesh. Please refer to our project page for video and interactive mesh results.
Appendix D Textual Prompts for 3D Comparison
Here we provide the missing textual prompts in Fig. 4 of our main paper as below:
1. ”RAW photo of A charming long brown coat dog, border collie, head of the dog, upper body, dark brown fur on the back,shelti,light brown fur on the chest,ultra detailed, brown eye”
2. ”Wild bear in a sheepskin coat and boots, open-armed, dancing, boots, patterned cotton clothes, cinematic, best quality”
3. ”Skull, masterpiece, a human skull made of broccoli”
4. ”A cute penguin wearing smoking is riding skateboard, Adorable Character, extremely detailed”
5. ”Masterpiece, batman, portrait, upper body, superhero, cape, mask”
6. ”Ral-chrome, fox, with brown orange and white fur, seated, full body, adorable”
7. ”Spiderman, mask, wearing black leather jacket, punk, absurdres, comic book”
8. ”Marvel iron man, heavy armor suit, futuristic, very cool, slightly sideways, portrait, upper body”