CiMaTe: Citation Count Prediction
Effectively Leveraging the Main Text
Abstract
Prediction of the future citation counts of papers is increasingly important to find interesting papers among an ever-growing number of papers. Although a paper’s main text is an important factor for citation count prediction, it is difficult to handle in machine learning models because the main text is typically very long; thus previous studies have not fully explored how to leverage it. In this paper, we propose a BERT-based citation count prediction model, called CiMaTe, that leverages the main text by explicitly capturing a paper’s sectional structure. Through experiments with papers from computational linguistics and biology domains, we demonstrate the CiMaTe’s effectiveness, outperforming the previous methods in Spearman’s rank correlation coefficient; 5.1 points in the computational linguistics domain and 1.8 points in the biology domain.
CiMaTe: Citation Count Prediction
Effectively Leveraging the Main Text
Jun Hirako Ryohei Sasano Koichi Takeda Graduate School of Informatics, Nagoya University [email protected] {sasano,takedasu}@i.nagoya-u.ac.jp
1 Introduction
The number of academic papers has increased dramatically in various fields. Accordingly, techniques to automatically estimate the quality of papers are becoming increasingly important for finding intersting papers among the vast number of papers. In this paper, we adopt the citation count as an approximate indicator of a paper’s quality, following previous studies Chubin and Garfield (1979); Aksnes (2006), and we tackle the task of predicting paper’s future citation counts.
In predicting citation counts, the use of a paper’s main text has the potential to improve performance. However, most existing studies (e.g., Ibáñez et al., 2009; Ma et al., 2021) use only titles and abstracts as the textual information. A major reason for this is that the papers’ main texts are very long and therefore difficult to handle in machine learning models. In particular, the quadratic computational costs of Transformer Vaswani et al. (2017)-based models such as BERT Devlin et al. (2019) make it difficult to process long documents. Approaches to overcome this problem include improvement in the Transformer structure (e.g., Kitaev et al., 2020; Beltagy et al., 2020) and processing of long documents by dividing them into several parts (e.g., Pappagari et al., 2019; Afkanpour et al., 2022), but it is unclear whether these approaches are effective or not in citation count prediction.
In this paper, we propose CiMaTe, a simple and strong Citation count prediction model that leverages the Main Text. CiMaTe explicitly captures a paper’s sectional structure by encoding each section with BERT and then aggregating the encoded section representations to predict the citation count. We demonstrate CiMaTe’s effectiveness by performing comparative experiments with several methods on two sets of papers collected from arXiv and bioRxiv, respectively.
2 Related Work
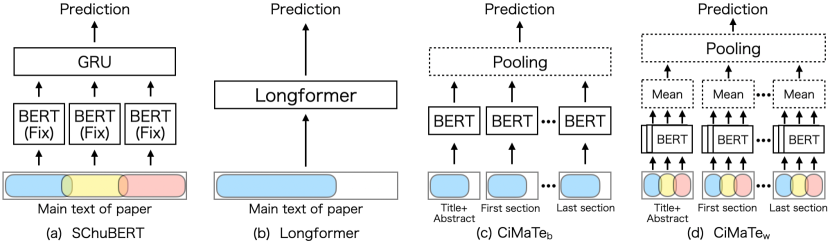
2.1 Citation Count Prediction
Existing methods for predicting citation counts include those that use textual information and those that do not. Among methods that do not use textual information, those of Castillo et al. (2007), Davletov et al. (2014), and Pobiedina and Ichise (2015) represent the citation relationships of papers as a graph. Abrishami and Aliakbary (2018) proposed a method that predicts the long-term citation count from short-term citation counts by using an RNN.
As for methods that use textual information, Ibáñez et al. (2009) proposed a method that uses a bag-of-words with abstracts, Fu and Aliferis (2008) proposed a method that uses frequency-based weighting, and Yan et al. (2011) and Chakraborty et al. (2014) proposed methods that use topics estimated by LDA. In recent years, there has been research on methods to capture the semantic features of papers’ textual information through deep learning. Ma et al. (2021) proposed a method that encodes the title and abstract with Doc2Vec Le and Mikolov (2014), and van Dongen et al. (2020) proposed a method that uses a paper’s main text via a pre-trained model. Hirako et al. (2023) proposed a BERT-based method that captures the latest research trends for newly-published papers.
2.2 Long Document Processing
There are two major approaches to processing long documents in a Transformer-based model. The first approach modifies the Transformer structure to efficiently process long sequences Kitaev et al. (2020); Beltagy et al. (2020); Zaheer et al. (2020). These methods mainly modify the self-attention module and improve the computational complexity. The second approach processes long documents by dividing them up. This approach includes methods that encode the divided text with BERT and aggregate it with simple techniques such as attention or an RNN Afkanpour et al. (2022); van Dongen et al. (2020). The second approach also includes hierarchical methods in which a BERT-encoded representation is further input to a Transformer Pappagari et al. (2019); Xue et al. (2021).
3 Leveraging the Main Text
3.1 Existing Approaches
SChuBERT van Dongen et al. (2020) is an existing method for predicting citation counts from the whole main text of papers. As shown in Figure 1 (a), it predicts the citation count by dividing the main text into overlapping chunks, encoding each of them with BERT, and aggregating them with a GRU Cho et al. (2014). As SChuBERT does not fine-tune BERT, it can leverage the whole main text with a relatively small computational cost. However, it divides the main text with a fixed number of characters and does not explicitly capture a paper’s structure.
Longformer Beltagy et al. (2020), a pre-trained language model for processing long sequences, can be used to predict citation counts leveraging the main text. As shown in Figure 1 (b), this method inputs the beginning of the main text by truncating it to a maximum input length of 4096 tokens. While this truncation is commonly used Xie et al. (2020), much of a paper’s main text may be truncated, and Longformer thus cannot consider the whole paper equally. For example, it truncated about 46% of the main text on average for the dataset used in our experiments (see Section 4.2).
3.2 CiMaTe
Our proposed method, CiMaTe predicts citation counts by encoding each section with BERT and then pooling the encoded section representations. We introduce two versions of CiMaTe: CiMaTe, which leverages only the beginning of each section, and CiMaTe, which divides each section to leverage the whole main text. Figure 1 (c) shows an overview of CiMaTe. This method inputs the section title as the first sentence and the content as the second sentence into BERT to generate section representations. The input content is truncated to not exceed 512 tokens, BERT’s maximum input length. While this method mitigates the increase in computational cost, only limited content is taken into account for each section.
Figure 1 (d) shows an overview of CiMaTe. First, this method divides the content of each section into chunks; then it encodes each chunk with BERT. The chunk representations are then pooled with their means to generate section representations. Following previous studies Pappagari et al. (2019); van Dongen et al. (2020), each content is divided into chunks that overlap by 50 tokens each. In encoding each chunk, the section title is input as the first sentence, and the chunk, as the second sentence, even if it is the second or later chunk. While this method can take the whole content into account, it increases the computational cost.
These two methods predict citation counts by pooling section representations. For a more expressive pooling method, we use Transformers besides the means. In Transformer pooling, each section representation is input to a one-layer Transformer as a sequence, and then the output is averaged.
4 Experiments
4.1 Task Setting
To validate the effectiveness of the CiMaTe, we compared several methods on the realistic citation count prediction task for newly published papers proposed by Hirako et al. (2023). In this task, the information used for training is strictly restricted to that available at the time of the target paper’s publication, which allows for a more realistic evaluation of the prediction method. The target of the prediction is the citation counts one year after publication. While the citation count one year after publication is available for papers published more than one year before the target paper, it is not available for papers published less than one year before the target paper. Thus, we used the method proposed by Hirako et al. (2023) to complement the citation counts for these papers. The specific target of the prediction is the logarithm rather than the citation count , reflecting the intuition that the difference between 0 and 10 cases is more significant than between 1,000 and 1,010 cases.
4.2 Dataset
As with Hirako et al. (2023), we constructed two datasets from the preprint server papers: a CL dataset from papers in the computational linguistics field, and a Bio dataset from the biology field. The reason for using papers from the preprint server was that while a large number of papers are published, some papers are not peer-reviewed and their quality is not guaranteed, so it was considered highly important to predict citation counts.
The CL dataset was constructed from papers submitted to the cs.CL category of arXiv111https://arxiv.org/ between June 2014 and June 2020. Citation information for calculating the citation counts was collected from Semantic Scholar.222https://www.semanticscholar.org/ The main texts of papers were collected by parsing their HTML versions.333https://ar5iv.labs.arxiv.org/ Papers for which HTML versions could not be collected and parsed were excluded from the dataset. We created 13 subsets of the CL dataset. Specifically, starting with each month between June 2019 and June 2020 inclusive, we created 13 subsets comprising papers that had been submitted in the previous 5 years before each of those months. The papers submitted in the last month of each subset were used for evaluation, while the other papers in the subset were used for training. Among these subsets, the one using papers published in June 2019 for evaluation was used as the development set, while the remaining subsets were used to calculate the evaluation scores. The average numbers of papers for training and evaluation were 11,383 and 449, respectively.
The Bio dataset was constructed from papers submitted to the Biochemistry, Plant Biology, and Pharmacology and Toxicology categories of bioRxiv444https://www.biorxiv.org/ between April 2015 and April 2021. The main texts were collected by parsing the papers’ HTML versions on bioRxiv. As with the CL dataset, we created 13 subsets of the Bio dataset. Papers submitted in each month from April 2020 to April 2021 inclusive were used for evaluation, and the subset with the oldest papers for evaluation was again used as the development set. The average numbers of papers for training and evaluation were 5,737 and 256, respectively.
4.3 Evaluation
For evaluation metrics, we used Spearman’s rank correlation coefficient (), the mean squared error (MSE), and a metric defined as the percentage of the actual top n% of papers in the top k% of the output (n%@k%). We calculated the evaluation scores as follows. First, for each subset, we trained a model and use that model to predict the citation count for each paper. We then compiled the predictions of citation counts for the 12 subsets and calculated an evaluation score on the whole. In all settings, we experimented with three different random seeds and calculated the means and standard deviations of the evaluation scores.
For certain methods, we found in our experiments that the predictions tend to be slightly larger than the correct values, which had a negative impact on the appropriate evaluation using MSE. To mitigate this, we also used MSE*, a variant of the MSE, as an evaluation metrics. This metric calculates the MSE by subtracting the difference between the average of the predictions and the average of the correct values for the development set from the predictions at test time.
Dataset | Method | Pooling | MSE | MSE* | 5%@5% | 5%@25% | 10%@10% | 10%@50% | |
---|---|---|---|---|---|---|---|---|---|
CL | BERT | - | 37.0±0.2 | 1.295±.016 | 1.082±.014 | 28.8±0.8 | 69.2±1.0 | 34.1±1.9 | 83.9±0.4 |
BERT | - | 38.5±0.8 | 1.246±.047 | 1.028±.010 | 29.0±2.1 | 71.5±1.5 | 34.6±1.7 | 86.0±1.0 | |
SChuBERT | - | 37.5±0.9 | 0.983±.015 | 0.987±.014 | 24.7±3.3 | 62.3±2.1 | 29.0±1.4 | 82.2±1.3 | |
Longformer | - | 37.9±0.1 | 1.245±.027 | 1.046±.014 | 27.9±1.4 | 74.3±1.6 | 34.6±0.3 | 85.8±0.3 | |
CiMaTe | mean | 42.6±0.1 | 1.184±.025 | 0.955±.006 | 33.1±1.7 | 74.0±2.0 | 38.3±0.4 | 86.7±0.9 | |
CiMaTe | Transformer | 43.6±0.6 | 1.228±.051 | 0.976±.015 | 33.6±0.4 | 76.7±1.3 | 38.4±0.7 | 88.1±1.1 | |
CiMaTe | mean | 42.9±0.5 | 1.157±.005 | 0.958±.009 | 32.7±1.1 | 75.4±0.6 | 37.3±0.5 | 87.3±0.9 | |
CiMaTe | Transformer | 43.2±1.2 | 1.170±.013 | 0.985±.019 | 34.2±0.9 | 74.0±0.8 | 37.8±1.7 | 87.8±1.0 | |
Bio | BERT | - | 34.7±0.5 | 0.517±.012 | 0.518±.012 | 48.8±0.4 | 82.1±0.8 | 47.4±1.3 | 86.0±1.0 |
BERT | - | 38.4±0.7 | 0.484±.010 | 0.489±.009 | 49.5±1.0 | 85.8±2.3 | 51.5±.0 | 87.8±2.1 | |
SChuBERT | - | 27.2±4.8 | 0.663±.015 | 0.613±.023 | 32.2±2.5 | 65.6±2.3 | 35.5±2.3 | 74.4±3.5 | |
Longformer | - | 39.1±0.2 | 0.479±.005 | 0.484±.007 | 50.3±1.7 | 84.7±2.6 | 49.6±0.2 | 86.4±0.7 | |
CiMaTe | mean | 39.4±0.7 | 0.450±.008 | 0.448±.008 | 51.4±2.7 | 86.5±0.4 | 51.4±0.7 | 90.2±1.2 | |
CiMaTe | Transformer | 40.9±0.5 | 0.452±.006 | 0.453±.007 | 52.7±1.0 | 87.8±1.0 | 51.4±0.5 | 90.8±0.2 | |
CiMaTe | mean | 38.5±0.0 | 0.467±.006 | 0.461±.009 | 51.0±1.3 | 86.7±1.0 | 49.6±0.7 | 88.2±1.4 | |
CiMaTe | Transformer | 40.7±0.9 | 0.456±.001 | 0.457±.001 | 52.1±1.5 | 89.1±1.5 | 50.3±0.8 | 91.0±1.0 | |
4.4 Comparison Methods
In addition to the methods described in Section 3, we compared two baseline methods using BERT. First, BERT was a method to predict citation counts by inputting the title as the first sentence and the abstract as the second sentence into BERT. Second, BERT, was a method to predict citation counts by inputting the beginning of the main text into BERT, similarly to Longformer, but this method used only a maximum of 512 tokens, whereas Longformer allows a maximum of 4096 tokens to be input.
4.5 Experimental Settings
We used the vector representation corresponding to the [CLS]
token as the output of BERT and Longformer; then, they input the model’s final vector output to a fully connected layer to predict citation counts.
In CiMaTe, to somewhat mitigate the computational cost, the content of each section was divided into a maximum of eight chunks, and the remaining text was truncated.
The loss function was the MSE of the predictions and the correct values, and we applied dropout Srivastava et al. (2014) to the final output vector during training.
For pre-trained weights, we used ‘bert-base-uncased’ for BERT and ‘allenai/longformer-base-4096’ for Longformer, both of which are publicly available on the Transformers Wolf et al. (2020). Also, there is no Longformer variant that was pre-trained on the scientific domain corpus. To make a fair comparison, we used only BERT that was pre-trained on the general domain corpus and did not use models pre-trained on the scientific domain corpus, such as SciBERT Beltagy et al. (2019).
All methods except SChuBERT were trained with a batch size of 32, the AdamW Loshchilov and Hutter (2019) optimizer, and a learning rate schedule that warm-up to 10% of the total training steps and then decayed linearly over the remaining steps. For the number of epochs and the learning rate, we performed grid search with values of {3, 4} for the number of epochs and {2e-5, 3e-5, 5e-5} for the learning rate, and we then used the values that yielded the highest rank correlation coefficient on the development set. For SChuBERT, only the number of epochs was determined by hyperparameter search, while the remaining settings were trained as in previous studies. The search range for the number of epochs was set to {20, 30, 40} according to the values in previous studies.
4.6 Results
The experimental results are summarized in Table 1. Except for SChuBERT, the methods that leverage a paper’s main text outperformed BERT on both datasets; thus we confirmed the effectiveness of leveraging the main text in predicting citation counts. Among the methods that leverage the main text, CiMaTe, which explicitly captures a paper’s sectional structure, achieved the highest performance. Specifically, in terms of the rank correlation coefficient, the model that scored best among the CiMaTe variants outperformed the other methods by at least 5.1 points on the CL dataset and at least 1.8 points on the Bio dataset. In terms of the MSE, MSE*, and n%@k%, it also outperformed the other methods in most cases, which demonstrates the general effectiveness of CiMaTe on sets of papers from different fields, namely, computational linguistics and biology.
Between the two CiMaTe versions, CiMaTe achieved the same or better performance as CiMaTe, and we thus found that by using only the beginning of each section, it was possible to achieve high prediction performance while mitigating the increase in computational cost. We also confirmed that Transformer pooling performed better overall than using means in the pooling of section representations. Although SChuBERT showed an improved MSE and MSE* on the CL dataset, its performance was inferior to that of BERT in other cases. We speculate that this was because SChuBERT did not fine-tune BERT to accept the whole main text as input with a relatively small computational cost Pappagari et al. (2019).
5 Conclusion
We have proposed CiMaTe, a citation count prediction method that effectively leverages a paper’s main text, and demonstrated its effectiveness through experimental comparison with several other methods on sets of papers from different fields, namely, computational linguistics and biology. In particular, we confirmed that CiMaTe can achieve high performance while mitigating the increase in computational cost by using only the beginning of each section. For future work, we plan to build a model that simultaneously considers information besides a paper’s text, such as the information available from the figures and tables, author information, and citation graphs.
References
- Abrishami and Aliakbary (2018) Alireza Abrishami and Sadegh Aliakbary. 2018. Predicting citation counts based on deep neural network learning techniques. J. Informetrics, 13:485–499.
- Afkanpour et al. (2022) Arash Afkanpour, Shabir Adeel, Hansenclever Bassani, Arkady Epshteyn, Hongbo Fan, Isaac Jones, Mahan Malihi, Adrian Nauth, Raj Sinha, Sanjana Woonna, Shiva Zamani, Elli Kanal, Mikhail Fomitchev, and Donny Cheung. 2022. BERT for long documents: A case study of automated ICD coding. In Proceedings of the 13th International Workshop on Health Text Mining and Information Analysis (LOUHI 2022), pages 100–107.
- Aksnes (2006) Dagfinn W. Aksnes. 2006. Citation rates and perceptions of scientific contribution. J. Assoc. Inf. Sci. Technol., 57:169–185.
- Beltagy et al. (2019) Iz Beltagy, Kyle Lo, and Arman Cohan. 2019. SciBERT: A pretrained language model for scientific text. In Proceedings of the 2019 Conference on Empirical Methods in Natural Language Processing and the 9th International Joint Conference on Natural Language Processing (EMNLP-IJCNLP 2019), pages 3615–3620.
- Beltagy et al. (2020) Iz Beltagy, Matthew E. Peters, and Arman Cohan. 2020. Longformer: The long-document transformer. arXiv, abs/2004.05150.
- Castillo et al. (2007) Carlos Castillo, Debora Donato, and A. Gionis. 2007. Estimating number of citations using author reputation. In String Processing and Information Retrieval: 14th International Symposium (SPIRE 2007).
- Chakraborty et al. (2014) Tanmoy Chakraborty, Suhansanu Kumar, Pawan Goyal, Niloy Ganguly, and Animesh Mukherjee. 2014. Towards a stratified learning approach to predict future citation counts. IEEE/ACM Joint Conference on Digital Libraries, pages 351–360.
- Cho et al. (2014) Kyunghyun Cho, Bart van Merriënboer, Caglar Gulcehre, Dzmitry Bahdanau, Fethi Bougares, Holger Schwenk, and Yoshua Bengio. 2014. Learning phrase representations using RNN encoder–decoder for statistical machine translation. In Proceedings of the 2014 Conference on Empirical Methods in Natural Language Processing (EMNLP 2014), pages 1724–1734.
- Chubin and Garfield (1979) Daryl E. Chubin and Eugene Garfield. 1979. Is citation analysis a legitimate evaluation tool? Scientometrics, 2:91–94.
- Davletov et al. (2014) Feruz Davletov, Ali Aydin, and Ali Cakmak. 2014. High impact academic paper prediction using temporal and topological features. In Proceedings of the 23rd ACM International Conference on Conference on Information and Knowledge Management (CIKM 2014), page 491–498.
- Devlin et al. (2019) Jacob Devlin, Ming-Wei Chang, Kenton Lee, and Kristina Toutanova. 2019. BERT: Pre-training of deep bidirectional transformers for language understanding. In Proceedings of the 2019 Conference of the North American Chapter of the Association for Computational Linguistics: Human Language Technologies, Volume 1 (NAACL 2019), pages 4171–4186.
- Fu and Aliferis (2008) Lawrence D. Fu and Constantin F. Aliferis. 2008. Models for predicting and explaining citation count of biomedical articles. AMIA … Annual Symposium proceedings. AMIA Symposium vol. 2008 (AMIA 2008), pages 222–226.
- Hirako et al. (2023) Jun Hirako, Ryohei Sasano, and Koichi Takeda. 2023. Realistic citation count prediction task for newly published papers. In Findings of the Association for Computational Linguistics: EACL 2023 (EACL 2023 Findings), pages 1131–1141.
- Ibáñez et al. (2009) Alfonso Ibáñez, Pedro Larrañaga, and Concha Bielza. 2009. Predicting citation count of bioinformatics papers within four years of publication. Bioinformatics, 25 24:3303–9.
- Kitaev et al. (2020) Nikita Kitaev, Lukasz Kaiser, and Anselm Levskaya. 2020. Reformer: The efficient transformer. In Proceedings of the 8th International Conference on Learning Representations (ICLR 2020).
- Le and Mikolov (2014) Quoc V. Le and Tomás Mikolov. 2014. Distributed representations of sentences and documents. In Proceedings of the 31th International Conference on Machine Learning (ICML 2014), pages 1188–1196.
- Loshchilov and Hutter (2019) Ilya Loshchilov and Frank Hutter. 2019. Decoupled weight decay regularization. In Proceedings of the 7th International Conference on Learning Representations (ICLR 2020).
- Ma et al. (2021) Anqi Ma, Yu Liu, Xiujuan Xu, and Tao Dong. 2021. A deep-learning based citation count prediction model with paper metadata semantic features. Scientometrics, 126:6803 – 6823.
- Pappagari et al. (2019) R. Pappagari, Piotr Żelasko, Jesús Villalba, Yishay Carmiel, and Najim Dehak. 2019. Hierarchical transformers for long document classification. IEEE Automatic Speech Recognition and Understanding Workshop (ASRU), pages 838–844.
- Pobiedina and Ichise (2015) Nataliia Pobiedina and Ryutaro Ichise. 2015. Citation count prediction as a link prediction problem. Applied Intelligence, 44:252 – 268.
- Srivastava et al. (2014) Nitish Srivastava, Geoffrey Hinton, Alex Krizhevsky, Ilya Sutskever, and Ruslan Salakhutdinov. 2014. Dropout: A simple way to prevent neural networks from overfitting. Journal of Machine Learning Research (JMLR 2014), 15(56):1929–1958.
- van Dongen et al. (2020) Thomas van Dongen, Gideon Maillette de Buy Wenniger, and Lambert Schomaker. 2020. SChuBERT: Scholarly document chunks with BERT-encoding boost citation count prediction. In Proceedings of the First Workshop on Scholarly Document Processing (SDP 2020), pages 148–157.
- Vaswani et al. (2017) Ashish Vaswani, Noam Shazeer, Niki Parmar, Jakob Uszkoreit, Llion Jones, Aidan N Gomez, Ł ukasz Kaiser, and Illia Polosukhin. 2017. Attention is all you need. In Proceedings of the 31st Conference on Neural Information Processing Systems (NeurIPS 2017), volume 30, pages 6000–6010.
- Wolf et al. (2020) Thomas Wolf, Lysandre Debut, Victor Sanh, Julien Chaumond, Clement Delangue, Anthony Moi, Pierric Cistac, Tim Rault, Remi Louf, Morgan Funtowicz, Joe Davison, Sam Shleifer, Patrick von Platen, Clara Ma, Yacine Jernite, Julien Plu, Canwen Xu, Teven Le Scao, Sylvain Gugger, Mariama Drame, Quentin Lhoest, and Alexander Rush. 2020. Transformers: State-of-the-art natural language processing. In Proceedings of the 2020 Conference on Empirical Methods in Natural Language Processing (EMNLP 2020): System Demonstrations, pages 38–45.
- Xie et al. (2020) Qizhe Xie, Zihang Dai, Eduard Hovy, Thang Luong, and Quoc Le. 2020. Unsupervised data augmentation for consistency training. In Proceedings of the 34th Conference on Neural Information Processing Systems (NeurIPS 2020), volume 33, pages 6256–6268.
- Xue et al. (2021) Jin Xue, Xiaoyi Tang, and Liyan Zheng. 2021. A hierarchical bert-based transfer learning approach for multi-dimensional essay scoring. IEEE Access, 9:125403–125415.
- Yan et al. (2011) Rui Yan, Jie Tang, Xiaobing Liu, Dongdong Shan, and Xiaoming Li. 2011. Citation count prediction: learning to estimate future citations for literature. In Proceedings of the 20th ACM international conference on Information and knowledge management (CIKM 2011), page 1247–1252.
- Zaheer et al. (2020) Manzil Zaheer, Guru Guruganesh, Kumar Avinava Dubey, Joshua Ainslie, Chris Alberti, Santiago Ontanon, Philip Pham, Anirudh Ravula, Qifan Wang, Li Yang, and Amr Ahmed. 2020. Big Bird: Transformers for longer sequences. In Proceedings of the 34th Conference on Neural Information Processing Systems (NeurIPS 2020), volume 33, pages 17283–17297.