Bridging Adaptivity and Safety:
Learning Agile Collision-Free Locomotion Across Varied Physics
Abstract
Real-world legged locomotion systems often need to reconcile agility and safety for different scenarios. Moreover, the underlying dynamics are often unknown and time-variant (e.g., payload, friction). In this paper, we introduce BAS (Bridging Adaptivity and Safety), which builds upon the pipeline of prior work Agile But Safe (ABS) (he2024agilesafelearningcollisionfree) and is designed to provide adaptive safety even in dynamic environments with uncertainties. BAS involves an agile policy to avoid obstacles rapidly and a recovery policy to prevent collisions, a physical parameter estimator that is concurrently trained with agile policy, and a learned control-theoretic RA (reach-avoid) value network that governs the policy switch. Also, the agile policy and RA network are both conditioned on physical parameters to make them adaptive. To mitigate the distribution shift issue, we further introduce an on-policy fine-tuning phase for the estimator to enhance its robustness and accuracy. The simulation results show that BAS achieves 50% better safety than baselines in dynamic environments while maintaining a higher speed on average. In real-world experiments, BAS shows its capability in complex environments with unknown physics (e.g., slippery floors with unknown frictions, unknown payloads up to 8kg), while baselines lack adaptivity, leading to collisions or degraded agility. As a result, BAS achieves a 19.8% increase in speed and gets a 2.36 times lower collision rate than ABS in the real world. Videos: https://adaptive-safe-locomotion.github.io.
keywords:
Reinforcement Learning, Adaptive Safe Control, Legged Locomotion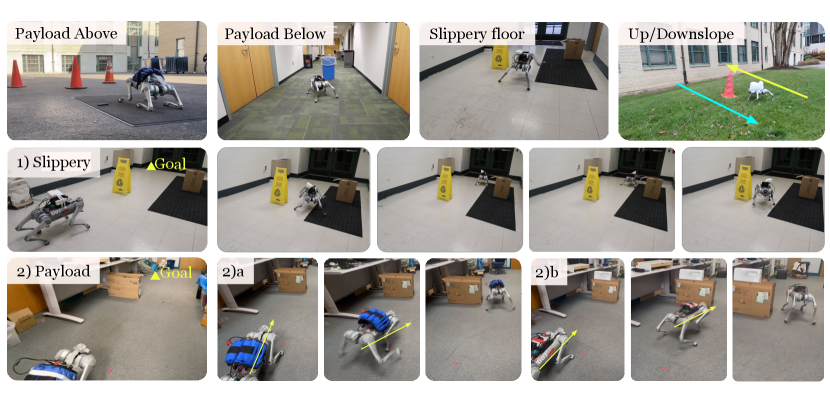
1 INTRODUCTION
Legged robot locomotion in cluttered and dynamic environments requires adaptivity to varying physics and environmental changes while simultaneously ensuring agility for efficient navigation and safety for reliable deployment. And such adaptivity to varied environments is claimed to be crucial for real-world tasks such as disaster response in forests