Asymptotic expansion of the hard-to-soft edge transition
Abstract
By showing that the symmetrically transformed Bessel kernel admits a full asymptotic expansion for the large parameter, we establish a hard-to-soft edge transition expansion. This resolves a conjecture recently proposed by Bornemann.
1 Introduction and statement of results
Consideration in this paper is a universal phenomenon arising from the random matrix theory, namely, the hard-to-soft edge transition [8]. As a concrete example, let and be two , , random matrices, whose element is chosen to be an independent normal random variable. The complex Wishart matrix or the Laguerre unitary ensemble (LUE), which plays an important role in statistics and signal processing (cf. [27, 30] and the references therein), is defined to be
where the superscript ∗ stands for the operation of conjugate transpose. As with fixed, the smallest eigenvalue of accumulates near the hard-edge . After proper scaling, the limiting process is a determinantal point process characterized by the Bessel kernel [15, 18]
(1.1) |
where is the Bessel function of the first kind of order (cf. [24]). If the parameter grows simultaneously with in such a way that approaches a positive constant, it comes out that the smallest eigenvalue is pushed away from the origin, creating a soft-edge. The fluctuation around the soft-edge, however, is given by the Airy point process [15, 18], which is determined by the Airy kernel
(1.2) |
where is the standard Airy function. One encounters the same limiting process by considering the scaled cumulative distribution of largest eigenvalues for large random Hermitian matrices with complex Gaussian entries, which is also known as the Tracy-Widom distribution [28].
Besides the above explanation of the hard-to-soft edge transition, the present work is also highly motivated by its connection with distribution of the length of longest increasing subsequences. Let be the set of all permutations on . Given , we denote by the length of longest increasing subsequences, which is defined as the maximum of all such that with . Equipped with the uniform measure, the question of the distribution of discrete random variable for large was posed by Ulam in the early 1960s [29]. After the efforts of many people (cf. [1, 26] and the references therein), Baik, Deift and Johansson finally answered this question in a celebrated work [2] by showing
(1.3) |
where
(1.4) |
is the aforementioned Tracy-Widom distribution with being the Airy kernel in (1.2).
To establish (1.3), a key ingredient of the proof is to introduce the exponential generating function of defined by
which is known as Hammersley’s Poissonization of the random variable . The quantity itself can be interpreted as the cumulative distribution of – the length of longest up/right path from to with nodes chosen randomly according to a Poisson process of intensity ; cf. [3, Chapter 2]. By representing as a Toeplitz determinant, it was proved in [2] that
(1.5) |
This, together with Johansson’s de-Poissonization lemma [20], will lead to (1.3).
Alternatively, one has (see [8, 16])
(1.6) |
where
(1.7) |
with being the Bessel kernel defined in (1.1) is the scaled hard-edge gap probability of LUE over . Thus, by showing the hard-to-soft transition
(1.8) |
An interesting question now is to improve (1.3) and (1.5) by establishing the first few finite-size correction terms or the asymptotic expansion. This is also known as edgeworth expansions in the literature, and we particularly refer to [7, 10, 13, 14, 19, 25] for the relevant results of Laguerre ensembles. In the context of the distribution for the length of longest increasing subsequences, the relationship (1.6) plays an important role in a recent work of Bornemann [5] among various studies toward this aim [4, 6, 17]. Instead of working on the Fredholm determinant directly, the idea in [5] is to establish an expansion between the Bessel kernel and the Airy kernel, which can be lifted to trace class operators. It is the aim of this paper to resolve some conjectures posed therein.
To proceed, we set
(1.9) |
and define, as in [5], the symmetrically transformed Bessel kernel
(1.10) |
where
(1.11) |
Our main result is stated as follows.
Theorem 1.1.
With defined in (1.10), we have, for any ,
(1.12) |
uniformly valid for with being any fixed real number. Preserving uniformity, the expansion can be repeatedly differentiated w.r.t. the variable and . Here, is the Airy kernel given in (1.2) and
(1.13) |
with being polynomials in and . Moreover, we have
(1.14) |
and
(1.15) |
Based on a uniform version of transient asymptotic expansion of Bessel functions [23], the above theorem is stated in [5] under the condition that , where the upper bound is obtained through a numerical inspection. It is conjectured therein that (1.12) is valid without such a restriction, Theorem 1.1 thus gives a confirm answer to this conjecture.
As long as the Bessel kernel admits an expansion of the form (1.12), it is generally believed that one can lift the expansion to the associated Fredholm determinants. By carefully estimating trace norms in terms of kernel bounds, this is rigorously established in [5, Theorem 2.1] for the perturbed Airy kernel determinants, which allows us to obtain the following hard-to-soft edge transition expansion with the aid of Theorem 1.1.
Corollary 1.2.
Again the above result is stated in [5] under a restriction on the number of summation but with explicit expressions of and in terms of the derivatives of . The expansion (1.16) serves as a preparatory step in establishing the expansion of the limit law (1.3) in [5]. Finally, we also refer to [9] for exponential moments, central limit theorems and rigidity of the hard-to-soft edge transition.
The rest of this paper is devoted to the proof of Theorem 1.1. The difficulty of using transient asymptotic expansion of the Bessel functions for large order to prove Theorem 1.1 lies in checking the divisibility of a certain sequence of polynomials. Indeed, it was commented in [5] that one probably needs some hidden symmetry of the coefficients in the expansion. The approach we adopt here, however, is based on a Riemann-Hilbert (RH) characterization of the Bessel kernel, as described in Section 2. By performing a Deift-Zhou nonlinear steepest descent analysis [12] to the associated RH problem in Section 3, the initial RH problem will be transformed into a small norm problem, for which one can find a uniform estimate. The proof of Theorem 1.1 is an outcome of our asymptotic analysis, which is presented in Section 4.
Notations
Throughout this paper, the following notations are frequently used.
-
•
If is a matrix, then stands for its -th entry. We use to denote a identity matrix.
-
•
As usual, the three Pauli matrices are defined by
(1.17)
2 An RH characterization of the Bessel kernel
Our starting point is the following Bessel parametrix [21], which particularly characterizes the Bessel kernel in (1.1).
For and , we set
(2.1) |
where and denote the modified Bessel functions of order (see [24]) and define
(2.2) |
Then satisfies the following RH problem.
RH problem 2.1.
- (a)
-
(b)
For , , the limiting values of exist and satisfy the jump condition
(2.4) - (c)
-
(d)
As , we have, for ,
(2.6) and for ,
(2.7) where is analytic at and we choose principal branches for and .
The Bessel kernel then admits the following representation:
(2.8) |
where the sign means taking limits from the upper half-plane.
3 Asymptotic analysis of the RH problem for with large
In this section, we will perform a Deift-Zhou steepest descent analysis [11] for the RH problem for . It consists of a series of explicit and invertible transformations and the final goal is to arrive at an RH problem tending to the identity matrix as . Without loss of generality, we may assume that in what follows.
3.1 First transformation:
Due to the scaled variable (1.11), the first transformation is a scaling. In addition, we multiply some constant matrices from the left to simplify the asymptotic behavior at infinity.
Define the matrix-valued function
(3.1) |
It is then readily seen from RH problem 2.1 that satisfies the following RH problem.
RH problem 3.1.
-
(a)
is defined and analytic for , where , , is defined in (2.3).
-
(b)
satisfies the jump condition
(3.2) -
(c)
As , we have
(3.3) where we take the principal branch for fractional exponents.
3.2 Second transformation:
In the second transformation we apply contour deformations. The rays and emanating from the origin are replaced by their parallel lines and emanating from . Let I and II be two regions bounded by and , respectively; see Figure 2 for an illustration. We now define
(3.4) |
It is easily seen the following RH problem for .
RH problem 3.2.
- (a)
-
(b)
satisfies the jump condition
(3.7) -
(c)
As , we have
(3.8)
3.3 Third transformation:
In order to normalize the behavior at infinity, we apply the third transformation by introducing the so-called -function:
(3.9) |
As before, we take a cut along for . The following proposition of is immediate from its definition.
Proposition 3.3.
-
(i)
The function is analytic in .
-
(ii)
For , we have
(3.10) -
(iii)
For , we have
(3.11) -
(iv)
For , we have
(3.12) -
(v)
As , we have
(3.13)
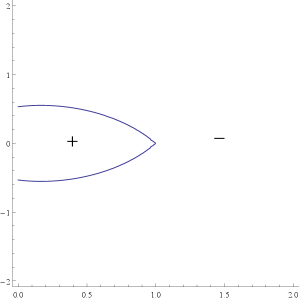
By setting
(3.14) |
it is readily seen from Proposition 3.3 and RH problem 3.2 that satisfies the RH problem as follows.
RH problem 3.4.
-
(a)
is defined and analytic in , where the contour is defined in (3.5).
-
(b)
satisfies the jump condition
(3.15) -
(c)
As , we have
(3.16)
3.4 Global parametrix
As , from the image of depicted in Figure 3, we conclude that all the jump matrices of tend to exponentially fast except for that along . Ignoring the exponential small terms in the jump matrices for , we come to the following global parametrix.
RH problem 3.5.
-
(a)
is defined and analytic in .
-
(b)
satisfies the jump condition
(3.17) -
(c)
As , we have
(3.18)
An explicit solution to the RH problem for is given by
(3.19) |
3.5 Local parametrix
Since the jump matrices for and are not uniformly close to each other near the point , we next construct local parametrix near this point. In a disc centered at 1 with certain fixed radius , we seek a matrix-valued function satisfying an RH problem as follows.
RH problem 3.6.
This local parametrix can be constructed by using the Airy parametrix introduced in Appendix A. To do this, we introduce the function:
(3.22) |
It is easily obtained that
(3.23) |
We then set
(3.24) |
with
(3.25) |
Proof.
We first show the prefactor is analytic near . According to its definition in (3.25), the only possible jump is on . It follows from (3.17) and (3.5) that, if ,
(3.26) |
since for . Thus, is analytic in . Note that, as , by using (3.5),
(3.27) |
with given in (1.9), we conclude that is a removable singularity. The jump conditions of in (3.20) follows directly from the analyticity of and (A.1). Finally, as , we apply (A.2) and obtain after a straightforward computation that
(3.28) |
This completes the proof of Proposition 3.7. ∎
3.6 Final transformation
We define the final transformation
(3.29) |
It is then readily seen that satisfies the following RH problem.
RH problem 3.8.
- (a)
-
(b)
satisfies the jump condition
(3.30) where
(3.31) -
(c)
As , we have
(3.32)
For , we have the estimate
(3.33) |
for some constant .
For , substituting the full expansion (A.4) of into (3.24) and (3.31), we have
(3.34) |
where
(3.35) |
with and , given in (3.5) and (A.5), respectively. By a standard argument [11, 12], we conclude that, as ,
(3.36) |
uniformly for . A combination of (3.36) and RH problem 3.8 shows that satisfies
RH problem 3.9.
-
(a)
is defined and analytic in .
-
(b)
satisfies the jump condition
(3.37) where
(3.38) -
(c)
As , we have .
From the local behavior of near given in (3.5), we obtain that
(3.39) |
By Cauchy’s residue theorem, we have
(3.40) |
Similarly, satisfies the following RH problem.
RH problem 3.10.
-
(a)
is defined and analytic in .
- (b)
-
(c)
As , we have .
From (3.6), (3.38), (3.42) and Cauchy’s residue theorem, it follows that
(3.43) |
is a diagonal matrix. For general , the functions are analytic in with asymptotic behavior as , and satisfy
(3.44) |
where the functions are given in (3.35). By Cauchy’s residue theorem, we have
(3.45) |
One can check that, by the structure of and mathematical induction, each takes the following structure:
(3.46) |
This, together with (3.36), gives us
(3.47) |
We are now ready to prove our main result.
4 Proof of Theorem 1.1
Recall the RH characterization of the Bessel kernel given in (2.8), we then follow the series of transformations in (3.1) (3.4) and (3.14) to obtain that
(4.1) |
where is given in (3.9). In what follows, we split our discussions into different cases based on different ranges of and .
If and , applying the final transformation (3.29) and (3.24) shows that
(4.2) |
where the functions and are given in (3.25), (3.29) and (3.19), respectively. By (3.11), it is readily seen that
(4.3) |
Thus, for ,
(4.4) |
and other terms in (4) are bounded for large positive , which follow from (3.19), (3.25) and (3.36). By taking
(4.5) |
in (4), where
(4.6) |
with being any fixed real number, we have, for any ,
(4.7) |
Here, is defined in (1.11) and the error term in the first inequality comes from the estimate [24, Formula 9.7.15]
(4.8) |
where is an arbitrary polynomial and the constant only depends on . As a consequence, the transformed Bessel kernel (1.10) will be absorbed into the error term of (1.12) in this case. As for the expansion terms in (1.12), note that is large as , we obtain again from [24, Formula 9.7.15] that, for any arbitrary polynomials ,
(4.9) |
and
(4.10) |
This, together with the estimate (4.8) for and , implies that the expansion terms in (1.12) is also absorbed into the error term, which shows that (1.12) is valid under the condition (4.6).
A similar argument holds if and , which implies (1.12) for and .
If , we obtain from (3.29) that
(4.11) |
From (4.3), it follows that
(4.12) |
and other terms in (4) are bounded for large positive , as can be seen from their definitions in (3.19) and (3.36). Substituting (4.5) into (4) with
(4.13) |
we have, for any ,
(4.14) |
The transformed Bessel kernel is again absorbed into the error term completely in this case. The removability of the singularities at comes from the symmetric structure of the expansion (4). Since the Airy functions in the expansion (1.12) related to and are superexponential decay as (see (4.9) and (4.10)), we conclude (1.12) under the condition (4.13).
It remains to consider the final case, namely, , which corresponds to
(4.15) |
through (4.5). To proceed, we again observe from (3.29) and (3.24) that
(4.16) |
Inserting (4.5) into the above formula gives us that
(4.17) |
We now show expansions of different parts on the right-hand side of the above formula. According to [5, Lemma 3.1], one has
(4.18) |
where each is certain polynomial of degree .
Next, it is observed that if ,
(4.19) |
This, together with (3.6) and the fact that is an analytic function in , implies that, as ,
(4.20) |
where are certain matrices with all the entries being polynomials in and . Indeed, by (3.27), (3.6) and (3.6), it follows that, as ,
(4.21) | ||||
(4.22) |
Thus, we have
(4.23) |
Then, we consider the parts involving the Airy functions. From the expansion of near given in (3.5), we have
(4.24) |
where are certain polynomials of degree with
(4.25) |
Moreover, by writing
(4.26) |
it is easily seen that
(4.27) |
for . We then obtain from the analyticity of Airy function and (4.26) that
(4.28) |
where denotes the -th derivative of with respect to . From the differential equation
(4.29) |
satisfied by Airy function, a direct calculation gives us
(4.30) |
where and are polynomials with and . They satisfy the recurrence relations (cf. [22])
(4.31) |
With the aids of the expansions (4.28) and (4.30), we obtain
(4.32) |
where
(4.33) | |||
(4.34) |
and
(4.35) |
are polynomials in and . Since the polynomials and are anti-symmetric in , , they must have the form
(4.36) |
We would like to show the polynomials admit the same structure. In other words, we want to show
(4.37) |
or equivalently,
(4.38) |
To prove the above equality, we need the following lemma.
Lemma 4.1.
With polynomials and defined through (4.30), we have
(4.39) |
Proof.
We use the method of mathematical induction to prove the above identity. It is clear that (4.39) holds for . Assume that (4.39) is valid for , i.e.,
(4.40) |
After taking derivative on both sides with respected to , it follows that
Applying the recurrence relations (4.31) to the above formula, we arrive at
which is (4.39) with . ∎
Using(4.27) and (4.39), we could rewrite the left-hand side of (4) as
(4.41) |
as required. Since the polynomials and all take the same structure (4.36), we obtain from (4) and the estimates of Airy functions in (4.8) that, for any ,
(4.42) |
where , and are certain polynomials in and .
Combining (4.18), (4.20) and (4) together gives us that, under the condition (4.15),
(4.43) |
as , where , , are polynomials in and . Additionally, it is worth noting that even though the calculations involved are inherently complicated, it is possible to derive precise formulaes for utilizing the explicit expressions (3.5), (3.27), (3.6) and (4.18). The first few polynomials are hereby presented:
and
Finally, since all the terms , , and are analytic functions for , all the expansions can repeatedly be differentiated w.r.t. the variables and while preserving the uniformity, which holds for the kernel expansion.
This completes the proof of Theorem 1.1. ∎
Appendix A The Airy parametrix
The Airy parametrix is the unique solution of the following RH problem.
RH problem A.1.
-
(a)
is analytic in , where the contours , , are indicated in Figure 5.
-
(b)
satisfies the jump condition
(A.1) -
(c)
As , we have
(A.2) -
(d)
is bounded near the origin.
Denote , the unique solution is given by (cf. [11])
(A.3) |
Furthermore, applying the asymptotics of and in [24, Chapter 9], we have, as ,
(A.4) |
where and
(A.5) |
Acknowledgements
We thank Folkmar Bornemann for helpful comments on the preliminary version of this paper. This work was partially supported by National Natural Science Foundation of China under grant numbers 12271105, 11822104, and “Shuguang Program” supported by Shanghai Education Development Foundation and Shanghai Municipal Education Commission.
References
- [1] D. Aldous and P. Diaconis, Longest increasing subsequences: from patience sorting to the Baik-Deift-Johansson theorem, Bull. Amer. Math. Soc. 36 (1999), 413–432.
- [2] J. Baik, P. Deift and K. Johansson, On the distribution of the length of the longest increasing subsequence of random permutations, J. Amer. Math. Soc. 12 (1999), 1119–1178.
- [3] J. Baik, P. Deift and T. Suidan, Combinatorics and Random Matrix Theory, Amer. Math. Soc., Providence, 2016.
- [4] J. Baik and R. Jenkins, Limiting distribution of maximal crossing and nesting of Poissonized random matchings, Ann. Probab. 41 (2013), 4359–4406.
- [5] F. Bornemann, Asymptotic expansions relating to the distribution of the length of longest increasing subsequences, preprint arXiv:2301.02022v5.
- [6] F. Bornemann, A Stirling-type formula for the distribution of the length of longest increasing subsequences, Found. Comput. Math. (2023).
- [7] F. Bornemann, A note on the expansion of the smallest eigenvalue distribution of the LUE at the hard edge, Ann. Appl. Probab. 26 (2016), 1942–1946.
- [8] A. Borodin and P. J. Forrester, Increasing subsequences and the hard-to-soft edge transition in matrix ensembles, J. Phys. A 36 (2003), 2963–2981.
- [9] C. Charlier and J. Lenells, The hard-to-soft edge transition: exponential moments, central limit theorems and rigidity, J. Approx. Theory 285 (2023), 105833, 50pp.
- [10] L. N. Choup, Edgeworth expansion of the largest eigenvalue distribution function of GUE and LUE, Int. Math. Res. Not. 2006 (2006), 61049, 32pp.
- [11] P. Deift, Orthogonal Polynomials and Random Matrices: A Riemann-Hilbert Approach, Courant Lecture Notes, vol. 3, New York University, 1999.
- [12] P. Deift and X. Zhou, A steepest descent method for oscillatory Riemann-Hilbert problems. Asymptotics for the MKdV equation, Ann. Math. 137 (1993), 295–368.
- [13] A. Edelman, A. Guionnet and S. Péché, Beyond universality in random matrix theory, Ann. Appl. Probab. 26 (2016), 1659–1697.
- [14] N. El Karoui, A rate of convergence result for the largest eigenvalue of complex white Wishart matrices, Ann. Probab. 34 (2006), 2077–2117.
- [15] P. J. Forrester, The spectrum edge of random matrix ensembles, Nucl. Phys. B 402 (1993), 709–728.
- [16] P. J. Forrester and T. D. Hughes, Complex Wishart matrices and conductance in mesoscopic systems: exact results, J. Math. Phys. 35 (1994), 6736–6747.
- [17] P. J. Forrester and A. Mays, Finite size corrections relating to distributions of the length of longest increasing subsequences, Adv. Appl. Math. 145 (2023), 102482, 33pp.
- [18] P. J. Forrester and T. Nagao, Asymptotic correlations at the spectrum edge of random matrices, Nuclear Phys. B 435 (1995), 401–420.
- [19] P. J. Forrester and A. K. Trinh, Finite-size corrections at the hard edge for the Laguerre ensemble, Stud. Appl. Math. 143 (2019), 315–336.
- [20] K. Johansson, The longest increasing subsequence in a random permutation and a unitary random matrix model, Math. Res. Lett. 5 (1988), 63–82.
- [21] A. B. J. Kuijlaars, K. T. -R. McLaughlin, W. Van Assche and M. Vanlessen, The Riemann-Hilbert approach to strong asymptotics for orthogonal polynomials on , Adv. Math. 188 (2004), 337–398.
- [22] B. J. Laurenzi, Polynomials associated with the gigher derivatives of the Airy functions and , preprint arXiv:1110.2025.
- [23] F. W. J. Olver, Some new asymptotic expansions for Bessel functions of large orders, Proc. Cambridge Philos. Soc. 48 (1952), 414–427.
- [24] F. W. J. Olver, A. B. Olde Daalhuis, D. W. Lozier, B. I. Schneider, R. F. Boisvert, C. W. Clark, B. R. Miller, B. V. Saunders, H. S. Cohl, and M. A. McClain, eds, NIST Digital Library of Mathematical Functions, http://dlmf.nist.gov/, Release 1.1.10 of 2023-06-15.
- [25] A. Perret and G. Schehr, Finite corrections to the limiting distribution of the smallest eigenvalue of Wishart complex matrices, Random Matrices Theory Appl. 5 (2016), 1650001, 27pp.
- [26] D. Romik, The Surprising Mathematics of Longest Increasing Subsequences, Cambridge Univ. Press, New York, 2015.
- [27] J. W. Silverstein and P. L. Combettes, Signal detection via spectral theory of large dimensional random matrices, IEEE Trans. Signal Processing 40 (1992), 2100–2105.
- [28] C. A. Tracy and H. Widom, Level-spacing distributions and the Airy kernel, Commun. Math. Phys. 159 (1994), 151–174.
- [29] S. M. Ulam, Monte Carlo calculations in problems of mathematical physics. In: Modern mathematics for the engineer: Second series, 261–281, McGraw-Hill, New York, 1961.
- [30] J. Wishart, The generalized product moment distribution in samples from a normal multivariate population, Biometrika A 20 (1928), 32–52.