A Multi-Institutional Open-Source Benchmark Dataset for Breast Cancer Clinical Decision Support using Synthetic Correlated Diffusion Imaging Data
Abstract
Recently, a new form of magnetic resonance imaging (MRI) called synthetic correlated diffusion (CDIs) imaging was introduced and showed considerable promise for clinical decision support for cancers such as prostate cancer when compared to current gold-standard MRI techniques. However, the efficacy for CDIs for other forms of cancers such as breast cancer has not been as well-explored nor have CDIs data been previously made publicly available. Motivated to advance efforts in the development of computer-aided clinical decision support for breast cancer using CDIs, we introduce Cancer-Net BCa, a multi-institutional open-source benchmark dataset of volumetric CDIs imaging data of breast cancer patients. Cancer-Net BCa contains CDIs volumetric images from a pre-treatment cohort of 253 patients across ten institutions, along with detailed annotation metadata (the lesion type, genetic subtype, longest diameter on the MRI (MRLD), the Scarff-Bloom-Richardson (SBR) grade, and the post-treatment breast cancer pathologic complete response (pCR) to neoadjuvant chemotherapy). We further examine the demographic and tumour diversity of the Cancer-Net BCa dataset to gain deeper insights into potential biases. Cancer-Net BCa is publicly available as a part of a global open-source initiative dedicated to accelerating advancement in machine learning to aid clinicians in the fight against cancer.
1 Introduction
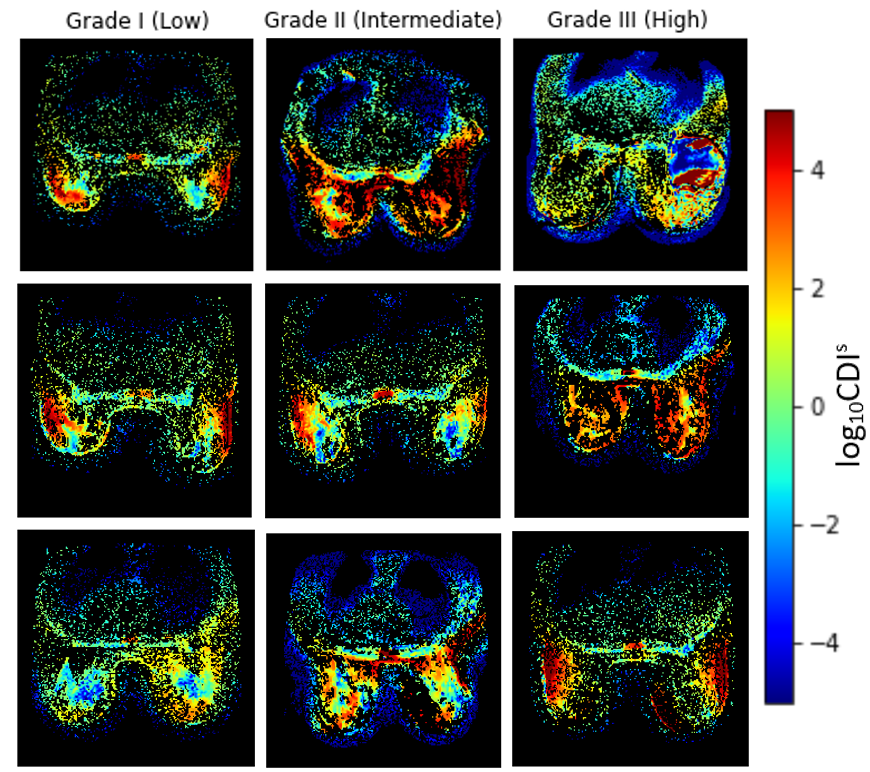
A new form of magnetic resonance imaging (MRI) called synthetic correlated diffusion (CDIs) imaging was recently introduced and showed considered promise for clinical decision support for cancers such as prostate cancer when compared to current gold-standard MRI techniques such as T2-weighted (T2w) imaging, diffusion-weighted imaging (DWI), and dynamic contrast-enhanced (DCE) imaging [1]. However, the efficacy for CDIs for other forms of cancer such as breast cancer has not been as well-explored nor have CDIs data been previously made publicly available. The development of computer-aided clinical decision support for breast cancer using CDIs has begun to be analyzed and shown to have superior results compared to other gold-standard imaging for the prediction of breast cancer patient response from neoadjuvant chemotherapy prior to treatment [2]. Motivated to advance efforts in the development of computer-aided clinical decision support for breast cancer using CDIs for diagnosis, prognosis/grading, treatment planning and more, we introduce Cancer-Net BCa, a multi-institutional open-source benchmark dataset of volumetric CDIs imaging data of breast cancer patients with detailed annotation metadata for each patient. We further examine the demographic and grade diversity of the Cancer-Net BCa dataset to gain deeper insights into potential biases. The Cancer-Net BCa benchmark dataset has been made publicly available 111https://www.kaggle.com/datasets/amytai/cancernet-bca as a part of a global open-source initiative dedicated to accelerating advancement in machine learning to aid clinicians in the fight against cancer.
2 Methodology
To construct the Cancer-Net BCa benchmark dataset, we produced CDIs acquisitions for a pre-treatment (T0) patient cohort of 253 patient cases across 10 institutions via the American College of Radiology Imaging Network (ACRIN) 6698/I-SPY2 study [3, 4, 5, 6]. More specifically, acquisitions were conducted with a four b-value imaging protocol (0 s/mm2, 100 s/mm2, 600 s/mm2, 800 s/mm2, 3-direction) on a 1.5 or 3.0 Tesla scanner using a dedicated breast radiofrequency coil. The pixel spacing for the acquisitions ranged from 0.83 mm to 2.08 mm with a median of 1.29 mm, with both slice thickness and spacing between slices ranged from 4.0 to 5.0 mm with a median of 4.0. The native and synthetic signals produced via a signal synthesizer were mixed together to obtain a final CDIs signal [1]. Each patient case is also associated with one of three possible SBR grades: I (Low), II (Intermediate), and III (High). Example images from each SBR type is shown in Fig. 1. The pCR state after neoadjuvant chemotherapy (No pCR/pCR) is also provided for each patient, with an example of each pCR state shown in Fig. 2.
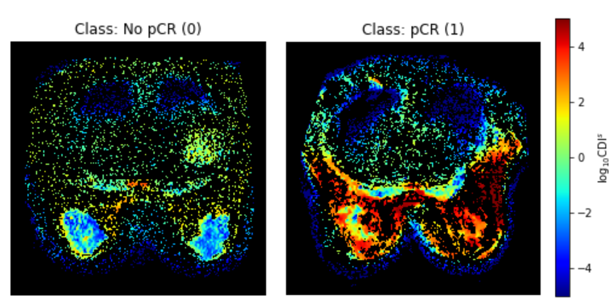
Race | Percentage |
---|---|
White | 70.8% |
Black | 10.7% |
Asian | 6.3% |
Unknown | 11.1% |
Multiple Races | 0.4% |
Native Hawaiian or other Pacific Islander | 0.4% |
American Indian or Alaska Native | 0.4% |
3 Results and Discussion
The demographics of the Cancer-Net BCa dataset is shown in Table 1. It can be seen that the White race dominates the data, comprising of 70.8% of the patients in the dataset, illustrating a severe race bias towards White patients. Additionally, Fig. 3 (top), it can be seen that the majority of the patients are between 30 to 70 years old (95.7%), indicating that very young patients ( 29) and very old patients ( 70) could be underrepresented in the dataset. On the other hand, the genetic subtype in the dataset is more fairly distributed with each subtype represented in at least 10% of the patients whereas the lesion type is more biased towards multiple masses and single mass as seen in Fig. 4 upper left and right respectively. In addition, the longest diameter on the MRI (MRLD) is also biased towards the range of 2 to 4 cm with less representation from patients in the other diameter ranges as seen in Fig. 3 (bottom).
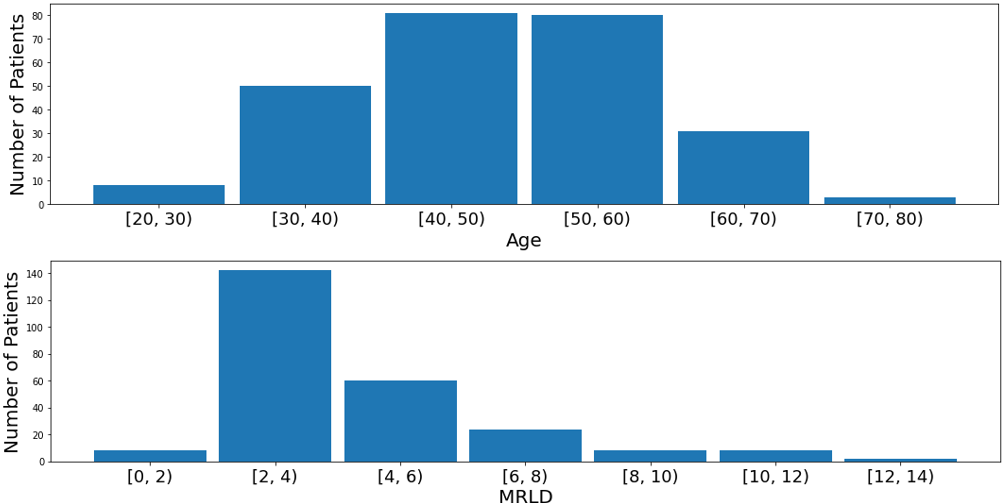
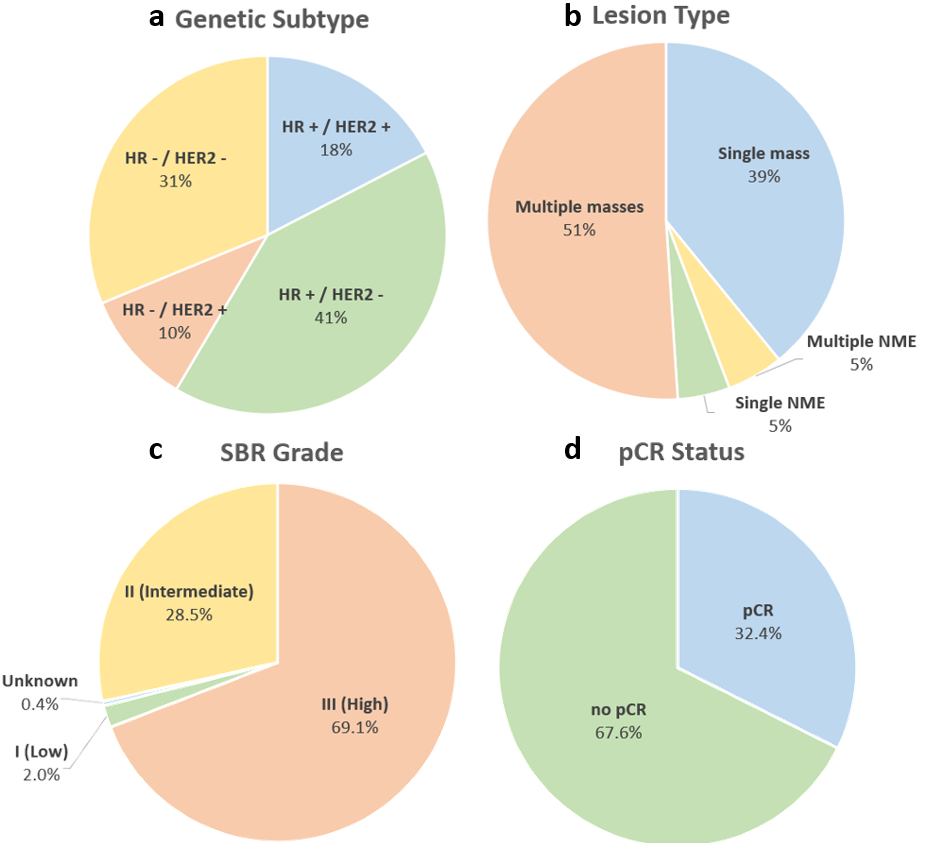
The grade distribution and pCR division are shown in bottom half of Fig. 4, indicating an uneven distribution in SBR grade, significantly skewed towards Grade III (High) and shows that more patients with no pCR (67.6%) compared to those who achieved pCR after neoadjuvant chemotherapy (32.4%). Noting the demographic, grade, and pCR imbalances, it is recommended to use algorithms and strategies that account for the imbalanced dataset such as data sampling, re-balancing of the classes, and balanced loss functions. Furthermore, these imbalances should be considered when evaluating systems developed on this dataset such as with balanced metrics such as per-class precision and recall.
References
- Wong et al. [2022] Alexander Wong, Hayden Gunraj, Vignesh Sivan, and Masoom A. Haider. Synthetic correlated diffusion imaging hyperintensity delineates clinically significant prostate cancer. Scientific Reports, 12(3376), 2022. URL https://doi.org/10.1038/s41598-022-06872-7.
- Tai et al. [2022] Chi-en Amy Tai, Nedim Hodzic, Nic Flanagan, Hayden Gunraj, and Alexander Wong. Cancer-net bca: Breast cancer pathologic complete response prediction using volumetric deep radiomic features from synthetic correlated diffusion imaging. In Conference and Workshop on Neural Information Processing Systems (NeurIPS), Medical Imaging Meets NeurIPS Workshop (MED-NeurIPS), 2022. URL https://arxiv.org/abs/2211.05308.
- Partridge et al. [2018] S. C. Partridge, Z. Zhang, D. C. Newitt, J. E. Gibbs, T. L. Chenevert, M. A. Rosen, P. J. Bolan, H. S. Marques, J. Romanoff, L. Cimino, B. N. Joe, H. R. Umphrey, H. Ojeda-Fournier, B. Dogan, K. Oh, H. Abe, J. S. Drukteinis, L. J. Esserman, and N. M. Hylton. Diffusion-weighted mri findings predict pathologic response in neoadjuvant treatment of breast cancer: The acrin 6698 multicenter trial. Radiology, 289(3):618–627, 2018.
- Newitt et al. [2018] D. C. Newitt, Z. Zhang, J. E. Gibbs, S. C. Partridge, T. L. Chenevert, M. A. Rosen, P. J. Bolan, H. S. Marques, S. Aliu, W. Li, L. Cimino, B. N. Joe, H. Umphrey, H. Ojeda‐Fournier, B. Dogan, H. Abe K. Oh, J. Drukteinis, and L. J. Esserman. Test–retest repeatability and reproducibility of adc measures by breast dwi: Results from the acrin 6698 trial. Journal of Magnetic Resonance Imaging, 49(6):1617–1628, 2018.
- Newitt et al. [2021] D. C. Newitt, S. C. Partridge, T. Chenevert Z. Zhang, J. Gibbs, M. Rosen, P. Bolan, H. Marques, J. Romanoff, L. Cimino, B. N. Joe, H. Umphrey, H. Ojeda-Fournier, B. Dogan, K. Y. Oh, H. Abe, J. Drukteinis, L. J. Esserman, and N. M. Hylton. Acrin 6698/i-spy2 breast dwi [data set]. The Cancer Imaging Archive, 2021.
- Clark et al. [2013] K. Clark, B. Vendt, K. Smith, J. Freymann, J. Kirby, P. Koppel, S. Moore, S. Phillips, D. Maffitt, M. Pringle, L. Tarbox, and F. Prior. The cancer imaging archive (tcia): Maintaining and operating a public information repository. Journal of Digital Imaging, 26(6):1045–1057, 2013.